Predicting grain protein content in wheat using hyperspectral sensing of in-season crop canopies and partial least squares regression
Article
Article Title | Predicting grain protein content in wheat using hyperspectral sensing of in-season crop canopies and partial least squares regression |
---|---|
ERA Journal ID | 123732 |
Article Category | Article |
Authors | Apan, Armando (Author), Kelly, Rob (Author), Phinn, Stuart (Author), Strong, Wayne (Author), Lester, David (Author), Butler, David (Author) and Robson, Andrew (Author) |
Journal Title | International Journal of Geoinformatics |
Journal Citation | 2 (1), pp. 93-108 |
Year | 2006 |
Place of Publication | Thailand |
ISSN | 1686-6576 |
Web Address (URL) | http://www.j-geoinfo.net/ |
Abstract | [Abstract]: The ability to estimate and map grain protein in cereal crops prior to harvest can benefit Australian grain growers. Segregation of grain by protein content can take advantage of price premiums, as well as to retrospectively assess the effectiveness of nutrient application strategies. This paper explores the relationships between hyperspectral data and grain protein content (GPC) in wheat (Triticum aestivum), with a view of developing predictive regression models. Canopy-scale, ground-based measurements of hyperspectral reflectance were obtained from samples located in the Formatin district of the Darling Downs, Queensland, Australia. Using partial least squares (PLS) regression, we investigated if the raw reflectance spectra, transformed data, and spectral vegetation indices (SVIs) could adequately predict grain protein content. The results showed that there are high correlations (e.g. r2=0.86, r2=0.81, r2=0.80) between reflectance data and grain protein. Cross-validated and tested PLS regression models produced low root mean square error of prediction (RMSEP) values (e.g. 0.5 percent GPC) and high prediction accuracy (e.g. 92%), confirming the usefulness of narrow-band spectral data. Bands in the near infrared (NIR) region were the most significant variables in the prediction. Despite the slightly higher correlation coefficients of SVIs, their predictive power for grain protein estimation was generally comparable with those of the raw spectra when analysed using PLS regression. |
Keywords | grain protein content, hyperspectral sensing, partial least squares regression |
ANZSRC Field of Research 2020 | 401304. Photogrammetry and remote sensing |
Public Notes | No response from publisher to requests for copyright permission. |
Byline Affiliations | Australian Centre for Sustainable Catchments |
Department of Primary Industries, Queensland | |
University of Queensland | |
Department of Primary Industries and Fisheries, Queensland | |
Incitec-Pivot, Australia |
https://research.usq.edu.au/item/9xx6q/predicting-grain-protein-content-in-wheat-using-hyperspectral-sensing-of-in-season-crop-canopies-and-partial-least-squares-regression
Download files
2737
total views1505
total downloads1
views this month0
downloads this month
Export as
Related outputs
Assessing the Spatio-temporal Activity Pattern and Habitat Use of Bengal Tiger (Panthera tigris tigris) Across Three Forest Management Regimes in Nepal
Maharjan, Amir, Maraseni, Tek, Allen, Benjamin L. and Apan, Armando. 2025. "Assessing the Spatio-temporal Activity Pattern and Habitat Use of Bengal Tiger (Panthera tigris tigris) Across Three Forest Management Regimes in Nepal." Biodiversity and Conservation. 34 (2), pp. 643-663. https://doi.org/10.1007/s10531-024-02991-xHydropower and Climate Resilience of Nepal Himalaya: A bottom-up Hydrological Approach
Bhattarai, Utsav, Maraseni, Tek, Devkota, Laxmi Prasad and Apan, Armando. 2024. "Hydropower and Climate Resilience of Nepal Himalaya: A bottom-up Hydrological Approach ." Earth Systems and Environment. https://doi.org/10.1007/s41748-024-00529-3Assessing the Theoretical Scope of Environmental Justice in Contemporary Literature and Developing a Pragmatic Monitoring Framework
Pandey, Hari Prasad, Maraseni, Tek Narayan and Apan, Armando. 2024. "Assessing the Theoretical Scope of Environmental Justice in Contemporary Literature and Developing a Pragmatic Monitoring Framework ." Sustainability. 16 (24). https://doi.org/10.3390/su162410799Insights into Ecological Resettlements and Conservation-led Displacements: A Systematic Review
Pandey, Hari Prasad, Maraseni, Tek Narayan and Apan, Armando. 2024. "Insights into Ecological Resettlements and Conservation-led Displacements: A Systematic Review." Environmental Management (New York): an international journal for decision-makers, scientists and environmental auditors. https://doi.org/10.1007/s00267-024-02097-8Enhancing systematic literature review adapting 'double diamond approach'
Pandey, Hari Prasad, Maraseni, Tek Narayan and Apan, Armando A.. 2024. "Enhancing systematic literature review adapting 'double diamond approach' ." Heliyon. 10 (22). https://doi.org/10.1016/j.heliyon.2024.e40581Unlocking the tapestry of conservation: Navigating ecological resettlement policies in Nepal
Pandey, Hari Prasad, Maraseni, Tek Narayan, Apan, Armando and Aryal, Kishor. 2024. "Unlocking the tapestry of conservation: Navigating ecological resettlement policies in Nepal." Science of the Total Environment. 946. https://doi.org/10.1016/j.scitotenv.2024.174335Decoupling REDD+ understanding of local stakeholders on the onset of materializing carbon credits from forests in Nepal
Pandey, Hari Prasad, Maraseni, Tek Narayan, Apan, Armando and Bhusal, Shreejana. 2024. "Decoupling REDD+ understanding of local stakeholders on the onset of materializing carbon credits from forests in Nepal." Forest Ecosystems. 11. https://doi.org/10.1016/j.fecs.2024.100239Achieving SOC Conservation without Land-Use Changes between Agriculture and Forests
Pandey, Hari Prasad, Maraseni, Tek Narayan, Apan, Armando and Bhusal, Shreejana. 2024. "Achieving SOC Conservation without Land-Use Changes between Agriculture and Forests." Sustainability. 16 (16). https://doi.org/10.3390/su16167207Facilitating sustainable energy transition of Nepal: A best-fit model to prioritize influential socio-economic and climate perception factors on household energy behaviour
Bhattarai, Utsav, Maraseni, Tek, Devkota, Laxmi Prasad and Apan, Armando. 2024. "Facilitating sustainable energy transition of Nepal: A best-fit model to prioritize influential socio-economic and climate perception factors on household energy behaviour." Energy for Sustainable Development: the journal of the international energy initiative. 81. https://doi.org/10.1016/j.esd.2024.101505Modelling Floodplain Vegetation Response to Climate Change, Using the Soil and Water Assessment Tool (SWAT) Model Simulated LAI, Applying Different GCM’s Future Climate Data and MODIS LAI Data
Muhury, Newton, Apan, Armando and Maraseni, Tek. 2024. "Modelling Floodplain Vegetation Response to Climate Change, Using the Soil and Water Assessment Tool (SWAT) Model Simulated LAI, Applying Different GCM’s Future Climate Data and MODIS LAI Data ." Remote Sensing. 16 (7). https://doi.org/10.3390/rs16071204A systematic literature review: Trends and current state of research on Bengal tiger (Panthera tigris tigris), 2010-2022
Maharjan, Amir, Maraseni, Tek, Allen, Benjamin L. and Apan, Armando. 2024. "A systematic literature review: Trends and current state of research on Bengal tiger (Panthera tigris tigris), 2010-2022." Biodiversity and Conservation. 33 (6-7), pp. 1871-1894. https://doi.org/10.1007/s10531-024-02856-3Evaluating four decades of energy policy evolution for sustainable development of a South Asian country-Nepal: A comprehensive review
Bhattarai, Utsav, Maraseni, Tek, Devkota, Laxmi P. and Apan, Armando. 2024. "Evaluating four decades of energy policy evolution for sustainable development of a South Asian country-Nepal: A comprehensive review." Sustainable Development. 32 (6), pp. 6703-6731. https://doi.org/10.1002/sd.3053Unravelling the impact of climate change on honey bees: An ensemble modelling approach to predict shifts in habitat suitability in Queensland, Australia
Tennakoon, Sarasie, Apan, Armando and Maraseni, Tek. 2024. "Unravelling the impact of climate change on honey bees: An ensemble modelling approach to predict shifts in habitat suitability in Queensland, Australia." Ecology and Evolution. 14 (4). https://doi.org/10.1002/ece3.11300Enviro-economic and feasibility analysis of industrial hemp value chain: A systematic literature review
Budhathoki, Rajan, Maraseni, Tek and Apan, Armando. 2024. "Enviro-economic and feasibility analysis of industrial hemp value chain: A systematic literature review." GCB Bioenergy. 16 (6). https://doi.org/10.1111/gcbb.13141A composite indicator-based method to assess the energy security of Nepal and prospects of cross-border electricity sharing in South Asia
Bhattarai, Utsav, Maraseni, Tek, Devkota, Laxmi and Apan, Armando. 2024. "A composite indicator-based method to assess the energy security of Nepal and prospects of cross-border electricity sharing in South Asia." Environmental Development. 51. https://doi.org/10.1016/j.envdev.2024.101002Identifying human elephant conflict hotspots through satellite remote sensing and GIS to support conflict mitigation
Gunawansa, Thakshila D., Perera, Kithsiri, Apan, Armando and Hettiarachchi, Nandita K.. 2024. "Identifying human elephant conflict hotspots through satellite remote sensing and GIS to support conflict mitigation ." Remote Sensing Applications: Society and Environment. 35. https://doi.org/10.1016/j.rsase.2024.101261Uncovering the Hidden Carbon Treasures of the Philippines’ Towering Mountains: A Synergistic Exploration Using Satellite Imagery and Machine Learning
Altarez, Richard Dein D., Apan, Armando and Maraseni, Tek. 2024. "Uncovering the Hidden Carbon Treasures of the Philippines’ Towering Mountains: A Synergistic Exploration Using Satellite Imagery and Machine Learning." PFG - Journal of Photogrammetry, Remote Sensing and Geoinformation Science. 92 (1), pp. 55-73. https://doi.org/10.1007/s41064-023-00264-wModelling future spatial distribution of peanut crops in Australia under climate change scenarios
Haerani, Haerani, Apan, Armando, Nguyen-Huy, Thong and Basnet, Badri. 2024. "Modelling future spatial distribution of peanut crops in Australia under climate change scenarios." Geo-spatial Information Science. 27 (5), pp. 1585-1604. https://doi.org/10.1080/10095020.2022.2155255TIMESAT software for estimating crop seasonality parameter
Haerani, Haerani and Apan, Armando. 2023. "TIMESAT software for estimating crop seasonality parameter." 1st Unhas International Conference on Agricultural Technology (UICAT 2021). Makassar, Indonesia 27 - 28 Oct 2021 United States. AIP Publishing. https://doi.org/10.1063/5.0118791Application of machine learning to assess people's perception of household energy in the developing world: A case of Nepal
Bhattarai, Utsav, Maraseni, Tek, Devkota, Laxmi Prasad and Apan, Armando. 2023. "Application of machine learning to assess people's perception of household energy in the developing world: A case of Nepal." Energy and AI. 14. https://doi.org/10.1016/j.egyai.2023.100303Understanding production possibility frontiers and utility values of ecosystem services in the Himalayas: An analysis of the supply-demand divide
Aryal, Kishor, Maraseni, Tek and Apan, Armando. 2023. "Understanding production possibility frontiers and utility values of ecosystem services in the Himalayas: An analysis of the supply-demand divide." Journal of Cleaner Production. 423. https://doi.org/10.1016/j.jclepro.2023.138725Greenery change and its impact on human-elephant conflict in Sri Lanka: a model-based assessment using Sentinel-2 imagery
Gunawansa, Thakshila D., Perera, Kithsiri, Apan, Armando and Hettiarachchi, Nandita. 2023. "Greenery change and its impact on human-elephant conflict in Sri Lanka: a model-based assessment using Sentinel-2 imagery." International Journal of Remote Sensing. 44 (16), pp. 5121-5146. https://doi.org/10.1080/01431161.2023.2244644Decoding the impacts of space and time on honey bees: GIS based fuzzy AHP and fuzzy overlay to assess land suitability for apiary sites in Queensland, Australia
Mudiyanselage, Sarasie, Apan, Armando, Maraseni, Tek and Altarez, Richard Dein D.. 2023. "Decoding the impacts of space and time on honey bees: GIS based fuzzy AHP and fuzzy overlay to assess land suitability for apiary sites in Queensland, Australia." Applied Geography. 155. https://doi.org/10.1016/j.apgeog.2023.102951Rationalizing donations and subsidies: Energy ecosystem development for sustainable renewable energy transition in Nepal
Bhattarai, Utsav, Maraseni, Tek, Apan, Armando and Devkota, Laxmi Prasad. 2023. "Rationalizing donations and subsidies: Energy ecosystem development for sustainable renewable energy transition in Nepal." Energy Policy. 177. https://doi.org/10.1016/j.enpol.2023.113570Preference, perceived change, and professed relationship among ecosystem services in the Himalayas
Aryal, Kishor, Maraseni, Tek and Apan, Armando. 2023. "Preference, perceived change, and professed relationship among ecosystem services in the Himalayas." Journal of Environmental Management. 344. https://doi.org/10.1016/j.jenvman.2023.118522Examining policy− institution− program (PIP) responses against the drivers of ecosystem dynamics. A chronological review (1960–2020) from Nepal
Aryal, Kishor, Maraseni, Tek and Apan, Armando. 2023. "Examining policy− institution− program (PIP) responses against the drivers of ecosystem dynamics. A chronological review (1960–2020) from Nepal." Land Use Policy: the international journal covering all aspects of land use. 132, p. 106789. https://doi.org/10.1016/j.landusepol.2023.106789Spatial dynamics of biophysical trade-offs and synergies among ecosystem services in the Himalayas
Aryal, Kishor, Maraseni, Tek and Apan, Armando. 2023. "Spatial dynamics of biophysical trade-offs and synergies among ecosystem services in the Himalayas." Ecosystem Services: science, policy and pratice. 59. https://doi.org/10.1016/j.ecoser.2022.101503Comparing global and local land cover maps for ecosystem management in the Himalayas
Aryal, Kishor, Apan, Armando and Maraseni, Tek. 2023. "Comparing global and local land cover maps for ecosystem management in the Himalayas." Remote Sensing Applications: Society and Environment. 30. https://doi.org/10.1016/j.rsase.2023.100952The human-elephant conflict in Sri Lanka: history and present status
Gunawansa, Thakshila, Perera, Kithsiri, Apan, Armando and Hettiarachchi, Nandita K.. 2023. "The human-elephant conflict in Sri Lanka: history and present status." Biodiversity and Conservation. 32 (10), pp. 3025-3052. https://doi.org/10.1007/s10531-023-02650-7Convolutional Neural Network-Based Deep Learning Approach for Automatic Flood Mapping Using NovaSAR-1 and Sentinel-1 Data
Apan, Armando, Andrew, Ogbaje, Paudyal, Dev Raj and Perera, Kithsiri. 2023. "Convolutional Neural Network-Based Deep Learning Approach for Automatic Flood Mapping Using NovaSAR-1 and Sentinel-1 Data." ISPRS International Journal of Geo-Information. 12 (194), pp. 1-26. https://doi.org/10.3390/ijgi12050194A call for ‘management authorship’ in community forestry
Aryal, Kishor, Maraseni, Tek and Apan, Armando. 2023. "A call for ‘management authorship’ in community forestry." Environmental Science and Policy. 139, pp. 204-208. https://doi.org/10.1016/j.envsci.2022.10.023Transforming agroforestry in contested landscapes: A win-win solution to trade-offs in ecosystem services in Nepal
Aryal, Kishor, Maraseni, Tek and Apan, Armando. 2023. "Transforming agroforestry in contested landscapes: A win-win solution to trade-offs in ecosystem services in Nepal." Science of the Total Environment. 857 (Part 1). https://doi.org/10.1016/j.scitotenv.2022.159301Deep learning U-Net classification of Sentinel-1 and 2 fusions effectively demarcates tropical montane forest's deforestation
Altarez, Richard Dein D., Apan, Armando and Maraseni, Tek. 2023. "Deep learning U-Net classification of Sentinel-1 and 2 fusions effectively demarcates tropical montane forest's deforestation." Remote Sensing Applications: Society and Environment. 29. https://doi.org/10.1016/j.rsase.2022.100887Student Performance Predictions for Advanced Engineering Mathematics Course With New Multivariate Copula Models
Nguyen-Huy, Thong, Deo, Ravinesh C., Khan, Shahjahan, Devi, Aruna, Adeyinka, Adewuyi Ayodele, Apan, Armando A. and Yaseen, Zaher Mundher. 2022. "Student Performance Predictions for Advanced Engineering Mathematics Course With New Multivariate Copula Models." IEEE Access. 10, pp. 45112 -45136. https://doi.org/10.1109/ACCESS.2022.3168322Assay of renewable energy transition: A systematic literature review
Bhattarai, Utsav, Maraseni, Tek and Apan, Armando. 2022. "Assay of renewable energy transition: A systematic literature review." Science of the Total Environment. 833, pp. 1-18. https://doi.org/10.1016/j.scitotenv.2022.155159Rapid assessment of mine rehabilitation areas with airborne LiDAR and deep learning: bauxite strip mining in Queensland, Australia
Murray, Xavier, Apan, Armando, Deo, Ravinesh and Maraseni, Tek. 2022. "Rapid assessment of mine rehabilitation areas with airborne LiDAR and deep learning: bauxite strip mining in Queensland, Australia." Geocarto International. 37 (26), pp. 11223-11252. https://doi.org/10.1080/10106049.2022.2048902Spaceborne satellite remote sensing of tropical montane forests: a review of applications and future trends
Altarez, Richard Dein D., Apan, Armando and Maraseni, Tek. 2022. "Spaceborne satellite remote sensing of tropical montane forests: a review of applications and future trends." Geocarto International. 37 (26), pp. 11900-11928. https://doi.org/10.1080/10106049.2022.2060330Detection of calcium, magnesium, and chlorophyll variations of wheat genotypes on sodic soils using hyperspectral red edge parameters
Choudhury, Malini Roy, Christopher, Jack, Das, Sumanta, Apan, Armando, Menzies, Neal W., Chapman, Scott, Mellor, Vincent and Dang, Yash P.. 2022. "Detection of calcium, magnesium, and chlorophyll variations of wheat genotypes on sodic soils using hyperspectral red edge parameters." Environmental Technology and Innovation. 27, pp. 1-14. https://doi.org/10.1016/j.eti.2022.102469Identifying and prioritising climate change adaptation actions for greater one-horned rhinoceros (Rhinoceros unicornis) conservation in Nepal
Pant, Ganesh, Maraseni, Tek, Apan, Armando and Allen, Benjamin L.. 2022. "Identifying and prioritising climate change adaptation actions for greater one-horned rhinoceros (Rhinoceros unicornis) conservation in Nepal." PEERJ. 10. https://doi.org/10.7717/peerj.12795How much do we know about trade-offs in ecosystem services? A systematic review of empirical research observations
Aryal, Kishor, Maraseni, Tek N. and Apan, Armando. 2022. "How much do we know about trade-offs in ecosystem services? A systematic review of empirical research observations." Science of the Total Environment. 806, pp. 1-13. https://doi.org/10.1016/j.scitotenv.2021.151229Evaluation of drought tolerance of wheat genotypes in rain-fed sodic soil environments using high-resolution UAV remote sensing techniques
Das, Sumanta, Christopher, Jack, Choudhury, Malini Roy, Apan, Armando, Chapman, Scott, Menzies, Neal W. and Dang, Yash P.. 2022. "Evaluation of drought tolerance of wheat genotypes in rain-fed sodic soil environments using high-resolution UAV remote sensing techniques." Biosystems Engineering. 217, pp. 68-82. https://doi.org/10.1016/j.biosystemseng.2022.03.004Predicted declines in suitable habitat for greater one-horned rhinoceros (Rhinoceros unicornis) under future climate and land use change scenarios
Pant, Ganesh, Maraseni, Tek, Apan, Armando and Allen, Benjamin L.. 2021. "Predicted declines in suitable habitat for greater one-horned rhinoceros (Rhinoceros unicornis) under future climate and land use change scenarios." Ecology and Evolution. 11 (24), pp. 18288-18304. https://doi.org/10.1002/ece3.8421Application of Sentinel-2 Satellite Data to Map Forest Cover in Southeast Sri Lanka through the Random Forest Classifier
Gunawansa, Thakshila, Perera, Kithsiri, Apan, Armando and Hettiarachchi, Nandita. 2022. "Application of Sentinel-2 Satellite Data to Map Forest Cover in Southeast Sri Lanka through the Random Forest Classifier." Journal of Advances in Engineering and Technology. I (I), pp. 049-1 to 049-10.Modelling Floodplain Vegetation Response to Groundwater Variability Using the ArcSWAT Hydrological Model, MODIS NDVI Data, and Machine Learning
Muhury, Newton, Apan, Armando A., Maraseni, Tek N. and Ayele, Gebiaw T.. 2022. "Modelling Floodplain Vegetation Response to Groundwater Variability Using the ArcSWAT Hydrological Model, MODIS NDVI Data, and Machine Learning." Land. 11 (12), pp. 1-23. https://doi.org/10.3390/land11122154Mapping Prominent Cash Crops Employing ALOS PALSAR-2 and Selected Machine Learners
Panuju, Dyah R., Haerani, H., Apan, Armando, Griffin, Amy L., Paull, David J. and Trisasongko, Bambang Hendro. 2022. "Mapping Prominent Cash Crops Employing ALOS PALSAR-2 and Selected Machine Learners." Kumar, Arvind, Kumar, Pavan, Singh, S. S., Trisasongko, Bambang Hendro and Rani, Meenu (ed.) Agriculture, Livestock Production and Aquaculture: Advances for Smallholder Farming Systems. Cham, Switzerland. Springer. pp. 131-146Improving Biomass and Grain Yield Prediction of Wheat Genotypes on Sodic Soil Using Integrated High-Resolution Multispectral, Hyperspectral, 3D Point Cloud, and Machine Learning Techniques
Choudhury, Malini Roy, Das, Sumanta, Christopher, Jack, Apan, Armando, Chapman, Scott, Menzies, Neal W. and Dang, Yash P.. 2021. "Improving Biomass and Grain Yield Prediction of Wheat Genotypes on Sodic Soil Using Integrated High-Resolution Multispectral, Hyperspectral, 3D Point Cloud, and Machine Learning Techniques." Remote Sensing. 13 (17), pp. 1-27. https://doi.org/10.3390/rs13173482UAV-thermal imaging: A technological breakthrough for monitoring and quantifying crop abiotic stress to help sustain productivity on sodic soils – A case review on wheat
Das, Sumanta, Chapman, Scott, Christopher, Jack, Choudhury, Malini Roy, Menzies, Neal W., Apan, Armando and Dang, Yash P.. 2021. "UAV-thermal imaging: A technological breakthrough for monitoring and quantifying crop abiotic stress to help sustain productivity on sodic soils – A case review on wheat." Remote Sensing Applications: Society and Environment. 23, pp. 1-13. https://doi.org/10.1016/j.rsase.2021.100583Improving estimation of in-season crop water use and health of wheat genotypes on sodic soils using spatial interpolation techniques and multi-component metrics
Choudhury, Malini Roy, Mellor, Vincent, Das, Sumanta, Christopher, Jack, Apan, Armando, Menzies, Neal W., Chapman, Scott and Dang, Yash P.. 2021. "Improving estimation of in-season crop water use and health of wheat genotypes on sodic soils using spatial interpolation techniques and multi-component metrics." Agricultural Water Management. 255. https://doi.org/10.1016/j.agwat.2021.107007Evaluation of water status of wheat genotypes to aid prediction of yield on sodic soils using UAV-thermal imaging and machine learning
Das, Sumanta, Christopher, Jack, Apan, Armando, Choudhury, Malini Roy, Chapman, Scott, Menzies, Neal W. and Dang, Yash P.. 2021. "Evaluation of water status of wheat genotypes to aid prediction of yield on sodic soils using UAV-thermal imaging and machine learning." Agricultural and Forest Meteorology. 307. https://doi.org/10.1016/j.agrformet.2021.108477UAV-thermal imaging and agglomerative hierarchical clustering techniques to evaluate and rank physiological performance of wheat genotypes on sodic soil
Das, Sumanta, Christopher, Jack, Apan, Armando, Choudhury, Malini Roy, Chapman, Scott, Menzies, Neal W. and Dang, Yash P.. 2021. "UAV-thermal imaging and agglomerative hierarchical clustering techniques to evaluate and rank physiological performance of wheat genotypes on sodic soil." ISPRS Journal of Photogrammetry and Remote Sensing. 173, pp. 221-237. https://doi.org/10.1016/j.isprsjprs.2021.01.014Characterizing net primary productivity of forests and non-forest areas in the Philippines using satellite imagery
Apan, Armando and Maraseni, Tek. 2012. "Characterizing net primary productivity of forests and non-forest areas in the Philippines using satellite imagery." 1st National Symposium and Workshop on Environmental Science (IESM 2012): Environmental Science in a Changing Environment. Manila, Philippines 07 - 08 May 2012 Manila, Philippines.Climate change vulnerability of Asia’s most iconic megaherbivore: greater one-horned rhinoceros (Rhinoceros unicornis)
Pant, Ganesh, Maraseni, Tek, Apan, Armando and Allen, Benjamin L.. 2020. "Climate change vulnerability of Asia’s most iconic megaherbivore: greater one-horned rhinoceros (Rhinoceros unicornis)." Global Ecology and Conservation. 23, pp. 1-14. https://doi.org/10.1016/j.gecco.2020.e01180The climate-induced alteration of future geographic distribution of aflatoxin in peanut crops and its adaptation options
Haerani, Haerani, Apan, Armando and Basnet, Badri. 2020. "The climate-induced alteration of future geographic distribution of aflatoxin in peanut crops and its adaptation options." Mitigation and Adaptation Strategies for Global Change. 25 (6), pp. 1149-1175. https://doi.org/10.1007/s11027-020-09927-0Using a Mixed Method Research to Develop Spatial Information Sharing Strategies Across Natural Resource Management Communities
Paudyal, Dev Raj, McDougall, Kevin and Apan, Armando. 2019. "Using a Mixed Method Research to Develop Spatial Information Sharing Strategies Across Natural Resource Management Communities." Inaugural Australasia & Pacific Regional Mixed Methods International Research Association Conference 2019: Expanding Conceptual and Methodological Boundaries (MMIRA 2019). Wellington, New Zealand 04 - 06 Dec 2019 Indianapolis, United States.Intelligent data analytics for time series, trend analysis and drought indices comparison
Dayal, Kavina S., Deo, Ravinesh C. and Apan, Armando A.. 2021. "Intelligent data analytics for time series, trend analysis and drought indices comparison." Deo, Ravinesh C., Samui, Pijush, Kisi, Ozgur and Yaseen, Zaher Mundher (ed.) Intelligent data analytics for decision-support systems in hazard mitigation: theory and practice of hazard mitigation. Singapore. Springer. pp. 151-169Monitoring rice growth status in the Mekong Delta, Vietnam using multitemporal Sentinel-1 data
Phung, Hoang-Phi, Lam-Dao, Nguyen, Nguyen-Huy, Thong, Le-Toan, Thuy and Apan, Armando A.. 2020. "Monitoring rice growth status in the Mekong Delta, Vietnam using multitemporal Sentinel-1 data." Journal of Applied Remote Sensing. 14 (1), pp. 014518-1-014518-23. https://doi.org/10.1117/1.JRS.14.014518Trends and current state of research on greater one-horned rhinoceros (Rhinoceros unicornis): a systematic review of the literature over a period of 33 years (1985–2018)
Pant, Ganesh, Maraseni, Tek, Apan, Armando and Allen, Benjamin L.. 2020. "Trends and current state of research on greater one-horned rhinoceros (Rhinoceros unicornis): a systematic review of the literature over a period of 33 years (1985–2018)." Science of the Total Environment. 710, pp. 1-14. https://doi.org/10.1016/j.scitotenv.2019.136349An assessment of governance quality for community-based forest management systems in Asia: Prioritisation of governance indicators at various scales
Maraseni, Tek Narayan, Bhattarai, Nabin, Karky, Bhaskar Singh, Cadman, Timothy, Timalsina, Niroj, Bhandari, Trishna Singh, Apan, Armando, Ma, Hwan Ok, Rawat, R. S., Verma, Nemit, San, Su Mon, Oo, Thaung Naing, Dorji, Kinley, Dhungana, Sindhu and Poudel, Mohan. 2019. "An assessment of governance quality for community-based forest management systems in Asia: Prioritisation of governance indicators at various scales." Land Use Policy: the international journal covering all aspects of land use. 81, pp. 750-671. https://doi.org/10.1016/j.landusepol.2018.11.044Development of copula statistical drought prediction model using the Standardized Precipitation-Evapotranspiration Index
Dayal, Kavina S., Deo, Ravinesh C. and Apan, Armando A.. 2020. "Development of copula statistical drought prediction model using the Standardized Precipitation-Evapotranspiration Index." Samui, Pijush, Bui, Dieu Tien, Chakraborty, Subrata and Deo, Ravinesh C. (ed.) Handbook of probabilistic models. Oxford, United Kingdom. Elsevier. pp. 141-178Remotely sensed agricultural grassland productivity responses to land use and hydro-climatic drivers under extreme drought and rainfall
Kath, Jarrod, Le Brocque, Andrew, Reardon-Smith, Kathryn and Apan, Armando. 2019. "Remotely sensed agricultural grassland productivity responses to land use and hydro-climatic drivers under extreme drought and rainfall." Agricultural and Forest Meteorology. 268, pp. 11-22. https://doi.org/10.1016/j.agrformet.2019.01.007Tree biomass quantity, carbon stock and canopy correlates in mangrove forest and land uses that replaced mangroves in Honda Bay, Philippines
Castillo, Jose Alan A., Apan, Armando A., Maraseni, Tek Narayan and Salmo, Severino G., III. 2018. "Tree biomass quantity, carbon stock and canopy correlates in mangrove forest and land uses that replaced mangroves in Honda Bay, Philippines." Regional Studies in Marine Science. 24, pp. 174-183. https://doi.org/10.1016/j.rsma.2018.08.006Mapping of peanut crops in Queensland, Australia using time-series PROBA-V 100-m normalized difference vegetation index imagery
Haerani, Haerani, Apan, Armando and Basnet, Badri. 2018. "Mapping of peanut crops in Queensland, Australia using time-series PROBA-V 100-m normalized difference vegetation index imagery." Journal of Applied Remote Sensing. 12 (3), pp. 1-22. https://doi.org/10.1117/1.JRS.12.036005Drought modelling based on artificial intelligence and neural network algorithms: a case study in Queensland, Australia
Dayal, Kavina, Deo, Ravinesh and Apan, Armando A.. 2017. "Drought modelling based on artificial intelligence and neural network algorithms: a case study in Queensland, Australia." Filho, Walter Leal (ed.) Climate change adaptation in Pacific countries:fostering resilience and improving the quality of life. Springer. pp. 177-198Drivers of grassland resistance and recovery to long term drought
Le Brocque, Andrew F, Kath, Jarrod, Reardon-Smith, Kate and Apan, Armando. 2017. "Drivers of grassland resistance and recovery to long term drought." 6th Joint Conference of the Ecological Society of Australia and the New Zealand Ecological Society (EcoTAS 2017). Pokolbin, Australia 26 Nov - 01 Dec 2017Spatio-temporal drought risk mapping approach and its application in the drought-prone region of south-east Queensland, Australia
Dayal, Kavina S., Deo, Ravinesh C. and Apan, Armando A.. 2018. "Spatio-temporal drought risk mapping approach and its application in the drought-prone region of south-east Queensland, Australia." Natural Hazards. 93 (2), pp. 823-847. https://doi.org/10.1007/s11069-018-3326-8Investigating drought duration-severity-intensity characteristics using the Standardized Precipitation-Evapotranspiration Index: case studies in drought-prone Southeast Queensland
Dayal, Kavina S., Deo, Ravinesh C. and Apan, Armando A.. 2018. "Investigating drought duration-severity-intensity characteristics using the Standardized Precipitation-Evapotranspiration Index: case studies in drought-prone Southeast Queensland." Journal of Hydrologic Engineering. 23 (1), p. 05017029. https://doi.org/10.1061/(ASCE)HE.1943-5584.0001593Soil C quantities of mangrove forests, their competing land uses, and their spatial distribution in the coast of Honda Bay, Philippines
Castillo, Jose Alan A., Apan, Armando A., Maraseni, Tek Narayan and Salmo, Severino G., III. 2017. "Soil C quantities of mangrove forests, their competing land uses, and their spatial distribution in the coast of Honda Bay, Philippines." Geoderma. 293, pp. 82-90. https://doi.org/10.1016/j.geoderma.2017.01.025The rate, extent and spatial predictors of forest loss (2000-2012) in the terrestrial protected areas of the Philippines
Apan, Armando, Suarez, Luz Angelica, Maraseni, Tek and Castillo, Jose Alan. 2017. "The rate, extent and spatial predictors of forest loss (2000-2012) in the terrestrial protected areas of the Philippines." Applied Geography. 81, pp. 32-42. https://doi.org/10.1016/j.apgeog.2017.02.007Savanna burning methodology for fire management and emissions reduction: a critical review of influencing factors
Maraseni, Tek Narayan, Reardon‑Smith, Kathryn, Griffiths, Greg and Apan, Armando. 2016. "Savanna burning methodology for fire management and emissions reduction: a critical review of influencing factors." Carbon Balance and Management. 11, pp. 1-11. https://doi.org/10.1186/s13021-016-0067-4Soil greenhouse gas fluxes in tropical mangrove forests and in land uses on deforested mangrove lands
Castillo, Jose Alan A., Apan, Armando A., Maraseni, Tek N. and Salmo, Severino G., III. 2017. "Soil greenhouse gas fluxes in tropical mangrove forests and in land uses on deforested mangrove lands." Catena. 159, pp. 60-69. https://doi.org/10.1016/j.catena.2017.08.005Estimation and mapping of above-ground biomass of mangrove forests and their replacement land uses in the Philippines using Sentinel imagery
Castillo, Jose Alan A., Apan, Armando A., Maraseni, Tek N. and Salmo, Severino G., III. 2017. "Estimation and mapping of above-ground biomass of mangrove forests and their replacement land uses in the Philippines using Sentinel imagery." ISPRS Journal of Photogrammetry and Remote Sensing. 134, pp. 70-85. https://doi.org/10.1016/j.isprsjprs.2017.10.016Hyperspectral sensing to detect the impact of herbicide drift on cotton growth and yield
Suarez, L. A., Apan, Armando and Werth, Jeff. 2016. "Hyperspectral sensing to detect the impact of herbicide drift on cotton growth and yield." ISPRS Journal of Photogrammetry and Remote Sensing. 120, pp. 65-76. https://doi.org/10.1016/j.isprsjprs.2016.08.004Groundwater decline and climatic variability: Implications for groundwater linked agro-ecosystems
Le Brocque, Andrew F., Kath, Jarrod, Reardon-Smith, Kate and Apan, Armando. 2016. "Groundwater decline and climatic variability: Implications for groundwater linked agro-ecosystems." 2016 Conference of the Ecological Society of Australia (ESA 2016). Fremantle, Australia 29 Nov - 02 Dec 2016 Australia.Vulnerability assessment of urban community and critical infrastructures for integrated flood risk management and climate adaptation strategies
Espada, Rudolf, Apan, Armando and McDougall, Kevin. 2017. "Vulnerability assessment of urban community and critical infrastructures for integrated flood risk management and climate adaptation strategies." International Journal of Disaster Resilience in the Built Environment. 8 (4), pp. 375-411. https://doi.org/10.1108/IJDRBE-03-2015-0010Application of hybrid artificial neural network algorithm for the prediction of Standardized Precipitation Index
Dayal, Kavina S., Deo, Ravinesh C. and Apan, Armando A.. 2016. "Application of hybrid artificial neural network algorithm for the prediction of Standardized Precipitation Index." 2016 IEEE Region 10 International Conference: Technologies for Smart Nation (TENCON 2016). Singapore 22 - 25 Nov 2016 Singapore. https://doi.org/10.1109/TENCON.2016.7848588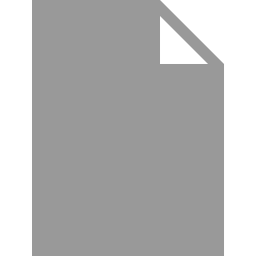
Application of Modis data to assess the latest forest cover changes of Sri Lanka
Perera, Kithsiri, Herath, Srikantha, Apan, Armando and Tateishi, Ryutaro. 2013. "Application of Modis data to assess the latest forest cover changes of Sri Lanka." Ogleby, Cliff (ed.) 22nd Annual Photogrammetry, Remote Sensing and Spatial Information Science Congress (ISPRS 2012): Imaging a Sustainable Future. Melbourne, Australia 25 Aug - 01 Sep 2012 Australia. Copernicus GmbH. https://doi.org/10.5194/isprsannals-I-7-165-2012Detection of phenoxy herbicide dosage in cotton crops through the analysis of hyperspectral data
Suarez, L. A., Apan, Armando and Werth, J.. 2017. "Detection of phenoxy herbicide dosage in cotton crops through the analysis of hyperspectral data." International Journal of Remote Sensing. 38 (23), pp. 6528-6553. https://doi.org/10.1080/01431161.2017.1362128Delineation of management zones using multiple crop yield data
Basnet, Badri, Kelly, Rob, Jensen, Troy, Strong, Wayne, Apan, Armando and Butler, David. 2003. "Delineation of management zones using multiple crop yield data." 16th Triennial Congress of the International Soil Tillage Research Organisation (ISTRO 2003). Brisbane, Australia 13 - 18 Jul 2003 Brisbane, Australia.Correlating high resolution aerial imagery with wheat yield and protein content
Apan, A., Kelly, R., Jensen, T., Strong, W. and Butler, D.. 2004. "Correlating high resolution aerial imagery with wheat yield and protein content." Smith, Richard and Dawbin, Ken (ed.) 12th Australasian Remote Sensing and Photogrammetry Conference (ARSPC 2004): To Measure is to Manage. Fremantle, Australia 18 - 22 Oct 2004 Adelaide, Australia.Spectral discrimination and classification of sugarcane varieties using EO-1 hyperion hyperspectral imagery
Apan, Armando, Held, Alex, Phinn, Stuart and Markley, John. 2004. "Spectral discrimination and classification of sugarcane varieties using EO-1 hyperion hyperspectral imagery." 25th Asian Conference on Remote Sensing (ACRS 2004). Chiang Mai, Thailand 22 - 26 Nov 2004 China.Application of aerial imagery to analyse on-farm trials
Basnet, B., Kelly, R., Jensen, T., Kane, T., Apan, A. and Butler, D.. 2002. "Application of aerial imagery to analyse on-farm trials." 11th Australasian Remote Sensing and Photogrammetry Conference (ARSPC2002): Images to Information. Brisbane, Australia 02 - 06 Sep 2002 Australia.Capturing of remotely sensed images to advance the use of yield maps and other spatial information to improve crop management and forecasting
Jensen, T., Apan, A., Young, F. and Zeller, L.. 2002. "Capturing of remotely sensed images to advance the use of yield maps and other spatial information to improve crop management and forecasting." 11th Australasian Remote Sensing and Photogrammetry Conference (ARSPC2002): Images to Information. Brisbane, Australia 02 - 06 Sep 2002 Adelaide, Australia.Spectral discrimination and separability analysis of agricultural crops and soil attributes using ASTER imagery
Apan, Armando, Kelly, Rob, Jensen, Troy, Butler, David, Strong, Wayne and Basnet, Badri. 2002. "Spectral discrimination and separability analysis of agricultural crops and soil attributes using ASTER imagery." 11th Australasian Remote Sensing and Photogrammetry Conference (ARSPC2002): Images to Information. Brisbane, Australia 02 - 06 Sep 2002 Adelaide, Australia.Quantifying landscape fragmentation in the Lockyer Valley Catchment, Queensland: 1973 – 1997
Apan, Armando A., Raine, Steven R. and Paterson, Mark S.. 2000. "Quantifying landscape fragmentation in the Lockyer Valley Catchment, Queensland: 1973 – 1997." AURISA 2000 Conference (AURISA 2000). Coolum, Australia 20 - 24 Nov 2000Estimating number of resistance genes to root-legion nematodes from synthetic hexaploids wheat lines
Thompson, John P., Zwart, Rebecca S. and Butler, David. 2011. "Estimating number of resistance genes to root-legion nematodes from synthetic hexaploids wheat lines." Wheat Breeding Assembly 2011. Perth, Australia 24 - 26 Aug 2011 Australia.Image analysis techniques for assessing landscape structural change: a case study of the Lockyer Valley Catchment, Queensland
Apan, Armando A., Raine, Steven R. and Paterson, Mark S.. 2000. "Image analysis techniques for assessing landscape structural change: a case study of the Lockyer Valley Catchment, Queensland." 10th Australasian Remote Sensing and Photogrammetry Conference (ARSPC 2000). Adelaide, Australia 21 - 25 Aug 2000Vulnerability assessment and interdependency analysis of critical infrastructures for climate adaptation and flood mitigation
Espada Jr., Rodolfo, Apan, Armando and McDougall, Kevin. 2015. "Vulnerability assessment and interdependency analysis of critical infrastructures for climate adaptation and flood mitigation." International Journal of Disaster Resilience in the Built Environment. 6 (3), pp. 313-346. https://doi.org/10.1108/IJDRBE-02-2014-0019Prioritising carbon sequestration areas in southern Queensland using time series MODIS net primary productivity (NPP) imagery
Apan, A., Suarez Cadavid, L. A., Richardson, L. and Maraseni, T.. 2014. "Prioritising carbon sequestration areas in southern Queensland using time series MODIS net primary productivity (NPP) imagery." Dadhwal, V. K., Seshasai, M. V. R., Hakeem, A., Diwakar, P. G. and Raju, P. L. N. (ed.) Technical Commission 8th Mid-Term Symposium (ISPRS 2014). Hyderabad, India 09 - 12 Dec 2014 Goettingen, Germany. https://doi.org/10.5194/isprsarchives-XL-8-549-2014Spectral discrimination of bulloak (Allocasuarina luehmannii) and associated woodland for habitat mapping of the endangered bulloak jewel butterfly (Hypochrysops piceata) in southern Queensland
Zainol Abdullah, Wan Nor Zanariah, Apan, Armando A., Maraseni, Tek N. and Le Brocque, Andrew F.. 2014. "Spectral discrimination of bulloak (Allocasuarina luehmannii) and associated woodland for habitat mapping of the endangered bulloak jewel butterfly (Hypochrysops piceata) in southern Queensland." Journal of Applied Remote Sensing. 8 (1), pp. 083561-1. https://doi.org/10.1117/1.JRS.8.083561Spatial modelling of natural disaster risk reduction policies with Markov decision processes
Espada Jr., Rodolfo, Apan, Armando and McDougall, Kevin. 2014. "Spatial modelling of natural disaster risk reduction policies with Markov decision processes." Applied Geography. 53, pp. 284-298. https://doi.org/10.1016/j.apgeog.2014.06.021The impact of varying statutory arrangements on spatial data sharing and access in regional NRM bodies
Paudyal, D. R., McDougall, K. and Apan, A.. 2014. "The impact of varying statutory arrangements on spatial data sharing and access in regional NRM bodies." ISPRS Annals of the Photogrammetry, Remote Sensing and Spatial Information Sciences. II (8), pp. 193-197. https://doi.org/10.5194/isprsannals-II-8-193-2014Inheritance of resistance to root-lesion nematodes (Pratylenchus thornei and P. neglectus) in five doubled-haploid populations of wheat
Thompson, John P., Zwart, Rebecca S. and Butler, David. 2012. "Inheritance of resistance to root-lesion nematodes (Pratylenchus thornei and P. neglectus) in five doubled-haploid populations of wheat." Euphytica: international journal on plant breeding. 188 (2), pp. 209-219. https://doi.org/10.1007/s10681-012-0689-xEffects of spatial resolution on measurement of landscape function using the landscape leakiness calculator
Dunwoody, Ernest, Apan, Armando and Liu, Xiaoye. 2013. "Effects of spatial resolution on measurement of landscape function using the landscape leakiness calculator." Surveying and Spatial Sciences Conference (SSSC 2013): Collect, Connect, Capitalise. Canberra, Australia 17 - 19 Apr 2013 Canberra, Australia.Developing spatial information sharing strategies across natural resource management communities
Paudyal, Dev Raj, McDougall, Kevin and Apan, Armando. 2013. "Developing spatial information sharing strategies across natural resource management communities." Onsrud, Harlan and Rajabifard, Abbas (ed.) Spatial Enablement in Support of Economic Development and Poverty Reduction: Research, Development and Education Perspectives. Needham, MA, USA. GSDI Association Press. pp. 141-168Using spatial modelling to develop flood risk and climate adaptation capacity metrics for vulnerability assessments of urban community and critical water supply infrastructure
Espada Jr., Rodolfo, Apan, Armando and McDougall, Kevin. 2013. "Using spatial modelling to develop flood risk and climate adaptation capacity metrics for vulnerability assessments of urban community and critical water supply infrastructure." Featherstone, Jeffrey (ed.) 49th World Congress of the International Society of City and Regional Planners (ISOCARP 2013): Frontiers of Planning: Evolving and Declining Models of City Planning Practice. Brisbane, Australia 01 - 04 Oct 2013 Netherlands.Adaptation and resilience in two flood-prone Queensland communities
King, David, Apan, Armando, Keogh, Diane and Thomas, Melanie. 2013. "Adaptation and resilience in two flood-prone Queensland communities." Boulter, Sarah, Palutikof, Jean, Karoly, David John and Guitart, Daniella (ed.) Natural disasters and adaptation to climate change. New York, NY. United States. Cambridge University Press. pp. 95-106Using spatial modelling to develop flood risk and climate adaptation capacity metrics for assessing urban community and critical electricity infrastructure vulnerability
Espada Jr., R., Apan, A. and McDougall, K.. 2013. "Using spatial modelling to develop flood risk and climate adaptation capacity metrics for assessing urban community and critical electricity infrastructure vulnerability." Piantadosi, J., Anderssen, R. S. and Boland, J. (ed.) 20th International Congress on Modelling and Simulation (MODSIM2013). Adelaide, Australia 01 - 06 Dec 2013 Australia. Modelling and Simulation Society of Australia and New Zealand . https://doi.org/10.36334/modsim.2013.L1.espada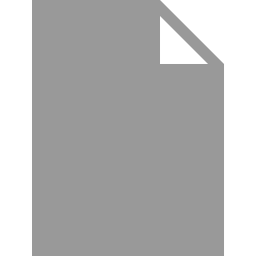