Uncovering the Hidden Carbon Treasures of the Philippines’ Towering Mountains: A Synergistic Exploration Using Satellite Imagery and Machine Learning
Article
Article Title | Uncovering the Hidden Carbon Treasures of the Philippines’ Towering Mountains: A Synergistic Exploration Using Satellite Imagery and Machine Learning |
---|---|
Article Category | Article |
Authors | Altarez, Richard Dein D., Apan, Armando and Maraseni, Tek |
Journal Title | PFG - Journal of Photogrammetry, Remote Sensing and Geoinformation Science |
Journal Citation | 92 (1), pp. 55-73 |
Number of Pages | 19 |
Year | 2024 |
Publisher | Springer |
Place of Publication | Germany |
ISSN | 2512-2789 |
2512-2819 | |
Digital Object Identifier (DOI) | https://doi.org/10.1007/s41064-023-00264-w |
Web Address (URL) | https://link.springer.com/article/10.1007/s41064-023-00264-w |
Abstract | Tropical montane forests (TMFs) are highly valuable for their above-ground biomass (AGB) and their potential to sequester carbon, but they remain understudied. Sentinel-1, -2, biophysical data and Machine Learning were used to estimate and map the AGB and above-ground carbon (AGC) stocks in Benguet, Philippines. Non-destructive field AGB measurements were collected from 184 plots, revealing that pine forests had 33.57% less AGB than mossy forests (380.33 Mgha−1), whilst the grassland summit had 39.93 Mgha−1. In contrast to the majority of literature, AGB did not decrease linearly with elevation. NDVI, LAI, fAPAR, fCover and elevation were the most effective predictors of field-derived AGB as determined by Random Forest (RF) feature selection in R. WEKA was used to evaluate and validate 26 Machine Learning algorithms. The results show that the Machine Learning K star (K*) (r = 0.213–0.832; RMSE = 106.682 Mgha−1–224.713 Mgha−1) and RF (r = 0.391–0.822; RMSE = 108.226 Mgha−1–175.642 Mgha−1) exhibited high modelling capabilities to estimate AGB across all predictor categories. Consequently, spatially explicit models were carried out in Whitebox Runner software to map the study site’s AGB, demonstrating RF with the highest predictive performance (r = 0.982; RMSE = 53.980 Mgha−1). The study area’s carbon stock map ranged from 0 to 434.94 Mgha−1, highlighting the significance of forests at higher elevations for forest conservation and carbon sequestration. Carbon-rich mountainous regions of the county can be encouraged for carbon sequestration through REDD + interventions. Longer wavelength radar imagery, species-specific allometric equations and soil fertility should be tested in future carbon studies. The produced carbon maps can help policy makers in decision-planning, and thus contribute to conserve the natural resources of the Benguet Mountains. |
Keywords | Tropical montane forest ; Machine learning; Radar remote sensing ; Optical remote sensing ; Biomass |
Related Output | |
Is part of | Machine learning-based assessment of deforestation, successional stages, and carbon stocks in a tropical montane forest using radar and optical satellite imagery |
Contains Sensitive Content | Does not contain sensitive content |
ANZSRC Field of Research 2020 | 410406. Natural resource management |
Public Notes | Files associated with this item cannot be displayed due to copyright restrictions. |
This article is part of a UniSQ Thesis by publication. See Related Output. | |
Byline Affiliations | Institute for Life Sciences and the Environment |
School of Surveying and Built Environment | |
Institute for Life Sciences and the Environment |
https://research.usq.edu.au/item/z78z5/uncovering-the-hidden-carbon-treasures-of-the-philippines-towering-mountains-a-synergistic-exploration-using-satellite-imagery-and-machine-learning
81
total views0
total downloads5
views this month0
downloads this month
Export as
Related outputs
Powering progress: The interplay of energy security and institutional quality in driving economic growth
Azimi, Mohammad Naim, Rahman, Mohammad Mafizur and Maraseni, Tek. 2025. "Powering progress: The interplay of energy security and institutional quality in driving economic growth." Applied Energy. 378 (Part A). https://doi.org/10.1016/j.apenergy.2024.124835Poplar Plantation as an Agroforestry Approach: Economic Benefits and Its Role in Carbon Sequestration in North India
Joshi, Rajeev, Sharma, Bharat, Singh, Hukum, Dhakal, Nabin, Ayer, Santosh and Maraseni, Tek. 2024. "Poplar Plantation as an Agroforestry Approach: Economic Benefits and Its Role in Carbon Sequestration in North India." Journal of Resources and Ecology. 15 (4), pp. 880-888. https://doi.org/10.5814/j.issn.1674-764x.2024.04.009Attitudes towards causes of and solutions to conflict between humans and Asian elephants
Cabral de Mel, Surendranie J., Seneweera, Saman, Dangolla, Ashoka, Weerakoon, Devaka K., King, Rachel, Maraseni, Tek and Allen, Benjamin L.. 2024. "Attitudes towards causes of and solutions to conflict between humans and Asian elephants ." Conservation Science and Practice. https://doi.org/10.1111/csp2.13238Assessing pollinator abundance and services to enhance agricultural sustainability and crop yield optimization in the Qilian Mountains
Yang, Haijiang, Gou, Xiaohua, Niu, Yibo, Shi, Wenwei, Wang, Xinyun, Wei, Yuxin and Maraseni, Tek. 2024. "Assessing pollinator abundance and services to enhance agricultural sustainability and crop yield optimization in the Qilian Mountains." Agricultural Systems. 221. https://doi.org/10.1016/j.agsy.2024.104109Unlocking the tapestry of conservation: Navigating ecological resettlement policies in Nepal
Pandey, Hari Prasad, Maraseni, Tek Narayan, Apan, Armando and Aryal, Kishor. 2024. "Unlocking the tapestry of conservation: Navigating ecological resettlement policies in Nepal." Science of the Total Environment. 946. https://doi.org/10.1016/j.scitotenv.2024.174335Decoupling REDD+ understanding of local stakeholders on the onset of materializing carbon credits from forests in Nepal
Pandey, Hari Prasad, Maraseni, Tek Narayan, Apan, Armando and Bhusal, Shreejana. 2024. "Decoupling REDD+ understanding of local stakeholders on the onset of materializing carbon credits from forests in Nepal." Forest Ecosystems. 11. https://doi.org/10.1016/j.fecs.2024.100239Achieving SOC Conservation without Land-Use Changes between Agriculture and Forests
Pandey, Hari Prasad, Maraseni, Tek Narayan, Apan, Armando and Bhusal, Shreejana. 2024. "Achieving SOC Conservation without Land-Use Changes between Agriculture and Forests." Sustainability. 16 (16). https://doi.org/10.3390/su16167207Technology in farming: Unleashing farmers’ behavioral intention for the adoption of agriculture 5.0
Mishra, Nitesh, Bhandari, Nabin, Maraseni, Tek, Devkota, Niranjan, Khanal, Ghanashyam, Bhusal, Biswash, Basya, Devid Kumar, Paudel, Udaya Raj and Danu, Ranjana Kumari. 2024. "Technology in farming: Unleashing farmers’ behavioral intention for the adoption of agriculture 5.0." PLoS One. 19 (8). https://doi.org/10.1371/journal.pone.0308883Spatiotemporal analysis of the food-related carbon emissions of China: Regional heterogeneity and the urban‒rural divide
Han, Jinyu, Qu, Jiansheng, Maraseni, Tek Narayan, Zeng, Jingjing, Wang, Dai, Ge, Yujie and Wu, Dingye. 2024. "Spatiotemporal analysis of the food-related carbon emissions of China: Regional heterogeneity and the urban‒rural divide ." Journal of Environmental Management. 370. https://doi.org/10.1016/j.jenvman.2024.122441Facilitating sustainable energy transition of Nepal: A best-fit model to prioritize influential socio-economic and climate perception factors on household energy behaviour
Bhattarai, Utsav, Maraseni, Tek, Devkota, Laxmi Prasad and Apan, Armando. 2024. "Facilitating sustainable energy transition of Nepal: A best-fit model to prioritize influential socio-economic and climate perception factors on household energy behaviour." Energy for Sustainable Development: the journal of the international energy initiative. 81. https://doi.org/10.1016/j.esd.2024.101505Carbon emission reduction initiatives: Lessons from the REDD+ process of the Asia and Pacific region
Aryal, Kishor, Maraseni, Tek, Rana, Eak, Subedi, Bhishma Prasad, Laudari, Hari Krishna, Ghimire, Puspa Lal, Khanal, Sudarshan Chandra, Zhang, Han and Timilsina, Ramesh. 2024. "Carbon emission reduction initiatives: Lessons from the REDD+ process of the Asia and Pacific region." Land Use Policy: the international journal covering all aspects of land use. 146. https://doi.org/10.1016/j.landusepol.2024.107321Determinants of minimal soil disturbance adoption over time and in the face of climate vulnerability
Sharna, Shaima Chowdhury, Maraseni, Tek and Radanielson, Ando. 2024. "Determinants of minimal soil disturbance adoption over time and in the face of climate vulnerability." Archives of Agronomy and Soil Science. 70 (1), pp. 1-21. https://doi.org/10.1080/03650340.2024.2398755Multi-stakeholder identification and prioritization of human-tiger conflict reduction measures in Chitwan National Park, Nepal
Dhungana, Rajendra, Maraseni, Tek, Allen, Benjamin L., Kandel, Ram Chandra, Koirala, Pashupati Nath, Pant, Ganesh and Ranabhat, Rishi. 2024. "Multi-stakeholder identification and prioritization of human-tiger conflict reduction measures in Chitwan National Park, Nepal." Oryx: journal of fauna and flora international. https://doi.org/10.1017/S0030605323001734The Interplay of Dietary Habits, Economic Factors, and Globalization: Assessing the Role of Institutional Quality
Azimi, Mohammad Naim, Rahman, Mohammad Mafizur and Maraseni, Tek. 2024. "The Interplay of Dietary Habits, Economic Factors, and Globalization: Assessing the Role of Institutional Quality." Nutrients. 16 (18). https://doi.org/10.3390/nu16183116Modelling Floodplain Vegetation Response to Climate Change, Using the Soil and Water Assessment Tool (SWAT) Model Simulated LAI, Applying Different GCM’s Future Climate Data and MODIS LAI Data
Muhury, Newton, Apan, Armando and Maraseni, Tek. 2024. "Modelling Floodplain Vegetation Response to Climate Change, Using the Soil and Water Assessment Tool (SWAT) Model Simulated LAI, Applying Different GCM’s Future Climate Data and MODIS LAI Data ." Remote Sensing. 16 (7). https://doi.org/10.3390/rs16071204A systematic literature review: Trends and current state of research on Bengal tiger (Panthera tigris tigris), 2010-2022
Maharjan, Amir, Maraseni, Tek, Allen, Benjamin L. and Apan, Armando. 2024. "A systematic literature review: Trends and current state of research on Bengal tiger (Panthera tigris tigris), 2010-2022." Biodiversity and Conservation. 33 (6-7), pp. 1871-1894. https://doi.org/10.1007/s10531-024-02856-3Evaluating four decades of energy policy evolution for sustainable development of a South Asian country-Nepal: A comprehensive review
Bhattarai, Utsav, Maraseni, Tek, Devkota, Laxmi P. and Apan, Armando. 2024. "Evaluating four decades of energy policy evolution for sustainable development of a South Asian country-Nepal: A comprehensive review." Sustainable Development. 32 (6), pp. 6703-6731. https://doi.org/10.1002/sd.3053REDD+ at risk: Emerging ten questions that REDD+ must answer
Aryal, Kishor, Maraseni, Tek, Subedi, Bhishma Prasad, Laudari, Hari Krishna, Ghimire, Puspa Lal, Khanal, Sudarshan Chandra, Zhang, Han and Timilsina, Ramesh. 2024. "REDD+ at risk: Emerging ten questions that REDD+ must answer ." Environmental Science and Policy. 156. https://doi.org/10.1016/j.envsci.2024.103744Unravelling the impact of climate change on honey bees: An ensemble modelling approach to predict shifts in habitat suitability in Queensland, Australia
Tennakoon, Sarasie, Apan, Armando and Maraseni, Tek. 2024. "Unravelling the impact of climate change on honey bees: An ensemble modelling approach to predict shifts in habitat suitability in Queensland, Australia." Ecology and Evolution. 14 (4). https://doi.org/10.1002/ece3.11300Land Use Carbon Emissions or Sink: Research Characteristics, Hotspots and Future Perspectives
Liu, Lina, Qu, Jiansheng, Gao, Feng, Maraseni, Tek Narayan, Wang, Shaojian, Aryal, Suman, Zhang, Zhenhua and Wu, Rong. 2024. "Land Use Carbon Emissions or Sink: Research Characteristics, Hotspots and Future Perspectives." Land. 13 (3). https://doi.org/10.3390/land13030279Enviro-economic and feasibility analysis of industrial hemp value chain: A systematic literature review
Budhathoki, Rajan, Maraseni, Tek and Apan, Armando. 2024. "Enviro-economic and feasibility analysis of industrial hemp value chain: A systematic literature review." GCB Bioenergy. 16 (6). https://doi.org/10.1111/gcbb.13141Pathways towards net zero: Assessment of enablers and barriers in Nepal
Bhusal, Shreejana, Dangol, Mukesh, Bhatta, Manoj, Maraseni, Tek and Mathew, Supriya. 2024. "Pathways towards net zero: Assessment of enablers and barriers in Nepal." Research in Globalization. 8. https://doi.org/10.1016/j.resglo.2024.100226A composite indicator-based method to assess the energy security of Nepal and prospects of cross-border electricity sharing in South Asia
Bhattarai, Utsav, Maraseni, Tek, Devkota, Laxmi and Apan, Armando. 2024. "A composite indicator-based method to assess the energy security of Nepal and prospects of cross-border electricity sharing in South Asia." Environmental Development. 51. https://doi.org/10.1016/j.envdev.2024.101002Comparison of Planted Pine versus Natural Mix Forests in Nepal
Pandey, Hari Prasad, Maraseni, Tek Narayan and Pokhrel, Shila. 2024. "Comparison of Planted Pine versus Natural Mix Forests in Nepal ." Forests. 15 (6). https://doi.org/10.3390/f15061070Identifying human elephant conflict hotspots through satellite remote sensing and GIS to support conflict mitigation
Gunawansa, Thakshila D., Perera, Kithsiri, Apan, Armando and Hettiarachchi, Nandita K.. 2024. "Identifying human elephant conflict hotspots through satellite remote sensing and GIS to support conflict mitigation ." Remote Sensing Applications: Society and Environment. 35. https://doi.org/10.1016/j.rsase.2024.101261Machine learning-based assessment of deforestation, successional stages, and carbon stocks in a tropical montane forest using radar and optical satellite imagery
Altarez, Richard Dein D.. 2024. Machine learning-based assessment of deforestation, successional stages, and carbon stocks in a tropical montane forest using radar and optical satellite imagery. PhD by Publication Doctor of Philosophy. University of Southern Queensland. https://doi.org/10.26192/z78z2Determinants of organic soil fertilization methods use over time and in the face of climate vulnerability
Sharna, Shaima Chowdhury, Maraseni, Tek and Radanielson, Ando Mariot. 2024. "Determinants of organic soil fertilization methods use over time and in the face of climate vulnerability." Soil and Tillage Research. 240. https://doi.org/10.1016/j.still.2024.106066Community forestry in a changing context: A perspective from Nepal's mid-hill
Laudari, Hari Krishna, Sapkota, Lok Mani, Maraseni, Tek, Subedi, Poorneswor, Pariyar, Shiva, Kaini, Tika Raj, Lopchan, Shyam Babu, Weston, Christopher and Volkova, Luba. 2024. "Community forestry in a changing context: A perspective from Nepal's mid-hill." Land Use Policy: the international journal covering all aspects of land use. 138. https://doi.org/10.1016/j.landusepol.2023.107018Does joint land ownership empower rural women socio-economically? Evidence from Eastern Nepal
Ghimire, Puspa Raj, Devkota, Niranjan, Maraseni, Tek, Khanal, Ghanashyam, Deuja, Jagat and Khadka, Umesh. 2024. "Does joint land ownership empower rural women socio-economically? Evidence from Eastern Nepal." Land Use Policy: the international journal covering all aspects of land use. 138. https://doi.org/10.1016/j.landusepol.2024.107052Predicting the current habitat refugia of Himalayan Musk deer (Moschus chrysogaster) across Nepal
Dhami, Bijaya, Chhetri, Nar Bahadur, Neupane, Bijaya, Adhikari, Binaya, Bashyal, Bijay, Maraseni, Tek Maraseni, Thapamagar, Tilak, Dhakal, Yogesh, Tripathi, Aashish and Koju, Narayan Prasad. 2024. "Predicting the current habitat refugia of Himalayan Musk deer (Moschus chrysogaster) across Nepal." Ecology and Evolution. 14 (2). https://doi.org/10.1002/ece3.10949The interplay between the entrepreneurial leadership identity, entrepreneurial leadership competency and venture growth intentions of women in rural Australia
Dhakal, Purushottam, Wiesner, Retha and Maraseni, Tek. 2024. "The interplay between the entrepreneurial leadership identity, entrepreneurial leadership competency and venture growth intentions of women in rural Australia." PLoS One. 19 (2). https://doi.org/10.1371/journal.pone.0296865Questioning the use of ensembles versus individual climate model generated flows in future peak flood predictions: Plausibility and implications
Devkota, Laxmi Prasad, Bhattarai, Utsav, Devkota, Rohini, Maraseni, Tek and Marahatta, Suresh. 2024. "Questioning the use of ensembles versus individual climate model generated flows in future peak flood predictions: Plausibility and implications." Journal of Flood Risk Management. 17 (2). https://doi.org/10.1111/jfr3.12978Knowledge mapping for a secure and sustainable hemp industry: A systematic literature review
Aryal, Kishor, Maraseni, Tek, Kretzschmar, Tobias, Chang, Dennis, Naebe, Maryam, Neary, Liz and Ash, Gavin. 2024. "Knowledge mapping for a secure and sustainable hemp industry: A systematic literature review." Case Studies in Chemical and Environmental Engineering. 9. https://doi.org/10.1016/j.cscee.2023.100550Potential for greenhouse gas (GHG) emissions savings from replacing short motorcycle trips with active travel modes in Vietnam
Tong, Yen Dan, Maraseni, Tek, Nguyen, Phuong-Duy, An-Vo, Duc-Anh, Mancuso Tradenta, Julio and Tran, Thuy Ai Dong. 2024. "Potential for greenhouse gas (GHG) emissions savings from replacing short motorcycle trips with active travel modes in Vietnam." Transportation. 51 (5), pp. 1999-2018. https://doi.org/10.1007/s11116-023-10394-0Welfare impacts associated with using aversive geofencing devices on captive Asian elephants
Cabral de Mel, Surendranie J., Seneweera, Saman, de Mel, Ruvinda K., Dangolla, Ashoka, Weerakoon, Devaka K., Maraseni, Tek and Allen, Benjamin L.. 2023. "Welfare impacts associated with using aversive geofencing devices on captive Asian elephants." Applied Animal Behaviour Science. 265. https://doi.org/10.1016/j.applanim.2023.105991Attitudes towards the Potential Use of Aversive Geofencing Devices to Manage Wild Elephant Movement
Cabral de Mel, Surendranie J., Seneweera, Saman, Dangolla, Ashoka, Weerakoon, Devaka K., Maraseni, Tek and Allen, Benjamin L.. 2023. "Attitudes towards the Potential Use of Aversive Geofencing Devices to Manage Wild Elephant Movement." Animals. 13 (16). https://doi.org/10.3390/ani13162657TIMESAT software for estimating crop seasonality parameter
Haerani, Haerani and Apan, Armando. 2023. "TIMESAT software for estimating crop seasonality parameter." 1st Unhas International Conference on Agricultural Technology (UICAT 2021). Makassar, Indonesia 27 - 28 Oct 2021 United States. AIP Publishing. https://doi.org/10.1063/5.0118791A global systematic literature review on sustainable soil management practices (1994–2022)
Sharna, Shaima Chowdhury, Maraseni, Tek and Radanielson, Ando Mariot. 2023. "A global systematic literature review on sustainable soil management practices (1994–2022)." Soil Use and Management. 39 (4), pp. 1267-1288. https://doi.org/10.1111/sum.12949Assessing the COVID-19 impacts on the coffee industry in Laos: An input–output modelling approach
Phimmavong, Somvang, Keenan, Rodney J., Phimmavong, Viengvilay, Maroulis, Jerry and Maraseni, Tek. 2023. "Assessing the COVID-19 impacts on the coffee industry in Laos: An input–output modelling approach." Cogent Economics and Finance. 11 (2). https://doi.org/10.1080/23322039.2023.2250692Community evaluation of forest and REDD+ governance quality in the democratic Republic of the Congo
Morgan, Edward A., Bush, Glenn, Mandea, Joseph Zambo and Maraseni, Tek. 2023. "Community evaluation of forest and REDD+ governance quality in the democratic Republic of the Congo." Journal of Environmental Management. 328. https://doi.org/10.1016/j.jenvman.2022.116891Accounting for and Comparison of Greenhouse Gas (GHG) Emissions between Crop and Livestock Sectors in China
Han, Jinyu, Qu, Jiansheng, Wang, Dai and Maraseni, Tek Narayan. 2023. "Accounting for and Comparison of Greenhouse Gas (GHG) Emissions between Crop and Livestock Sectors in China." Land. 12 (9). https://doi.org/10.3390/land12091787Application of machine learning to assess people's perception of household energy in the developing world: A case of Nepal
Bhattarai, Utsav, Maraseni, Tek, Devkota, Laxmi Prasad and Apan, Armando. 2023. "Application of machine learning to assess people's perception of household energy in the developing world: A case of Nepal." Energy and AI. 14. https://doi.org/10.1016/j.egyai.2023.100303Understanding production possibility frontiers and utility values of ecosystem services in the Himalayas: An analysis of the supply-demand divide
Aryal, Kishor, Maraseni, Tek and Apan, Armando. 2023. "Understanding production possibility frontiers and utility values of ecosystem services in the Himalayas: An analysis of the supply-demand divide." Journal of Cleaner Production. 423. https://doi.org/10.1016/j.jclepro.2023.138725Greenery change and its impact on human-elephant conflict in Sri Lanka: a model-based assessment using Sentinel-2 imagery
Gunawansa, Thakshila D., Perera, Kithsiri, Apan, Armando and Hettiarachchi, Nandita. 2023. "Greenery change and its impact on human-elephant conflict in Sri Lanka: a model-based assessment using Sentinel-2 imagery." International Journal of Remote Sensing. 44 (16), pp. 5121-5146. https://doi.org/10.1080/01431161.2023.2244644Identification of recycling pathways for secondary aluminum dross with integrated hybrid life cycle assessment
Xiao, Luyin, Wang, Yao, Zheng, Rufeng, Liu, Jingru, Zhao, Jun, Maraseni, Tek and Qian, Guangren. 2023. "Identification of recycling pathways for secondary aluminum dross with integrated hybrid life cycle assessment." Resources, Conservation and Recycling. 193. https://doi.org/10.1016/j.resconrec.2023.106987Attaining multiple sustainable development goals through storage hydropower development amidst community vulnerabilities
Bhattarai, Utsav, Devkota, Rohini, Maraseni, Tek, Devkota, Laxmi and Marahatta, Suresh. 2023. "Attaining multiple sustainable development goals through storage hydropower development amidst community vulnerabilities." Sustainable Development. 31 (5), pp. 3913-3929. https://doi.org/10.1002/sd.2634Carbonated tiger-high above-ground biomass carbon stock in protected areas and corridors and its observed negative relationship with tiger population density and occupancy in the Terai Arc Landscape, Nepal
Thapa, Kanchan, Thapa, Gokarna Jung, Manandhar, Ugan, Dhakal, Maheshwar, Jnawali, Shant Raj and Maraseni, Tek Narayan. 2023. "Carbonated tiger-high above-ground biomass carbon stock in protected areas and corridors and its observed negative relationship with tiger population density and occupancy in the Terai Arc Landscape, Nepal ." PLoS One. 18 (1). https://doi.org/10.1371/journal.pone.0280824Decoding the impacts of space and time on honey bees: GIS based fuzzy AHP and fuzzy overlay to assess land suitability for apiary sites in Queensland, Australia
Mudiyanselage, Sarasie, Apan, Armando, Maraseni, Tek and Altarez, Richard Dein D.. 2023. "Decoding the impacts of space and time on honey bees: GIS based fuzzy AHP and fuzzy overlay to assess land suitability for apiary sites in Queensland, Australia." Applied Geography. 155. https://doi.org/10.1016/j.apgeog.2023.102951Impact of the coronavirus pandemic on financial returns of smallholder coffee plantations in Lao PDR
Phimmavong, Somvang, Maraseni, Tek Narayan, Keenan, Rodney J, Phongoudome, Chanhsamone and Douangphosy, Boonthavy. 2023. "Impact of the coronavirus pandemic on financial returns of smallholder coffee plantations in Lao PDR." Agroforestry Systems. 97 (4), pp. 533-548. https://doi.org/10.1007/s10457-023-00808-4Understanding local ecosystem dynamics in three provinces of the lowlands of Nepal
Pandey, Hari Prasad, Aryal, Kishor, Aryal, Suman and Maraseni, Tek Narayan. 2023. "Understanding local ecosystem dynamics in three provinces of the lowlands of Nepal." Science of the Total Environment. 867. https://doi.org/10.1016/j.scitotenv.2023.161501Local stakeholders’ priorities and perceptions towards forest ecosystem services in the Red panda habitat region of Nepal
Koju, Upama, Karki, Sikha, Shrestha, Anita, Maraseni, Tek, Gautam, Ambika P., Cadman, Tim, Sherpa, Ang Phuri and Lama, Sonam Tashi. 2023. "Local stakeholders’ priorities and perceptions towards forest ecosystem services in the Red panda habitat region of Nepal." Land Use Policy: the international journal covering all aspects of land use. 129. https://doi.org/10.1016/j.landusepol.2023.106657Managing water scarcity via rainwater harvesting system in Kathmandu Valley, Nepal: People's awareness, implementation challenges and way forward
Khanal, Ghanashyam, Maraseni, Tek, Thapa, Anusha, Devkota, Niranjan, Paudel, Udaya Raj and Khanal, Chandra Kanta. 2023. "Managing water scarcity via rainwater harvesting system in Kathmandu Valley, Nepal: People's awareness, implementation challenges and way forward." Environmental Development. 46. https://doi.org/10.1016/j.envdev.2023.100850Ecological factors associated with hispid hare (Caprolagus hispidus) habitat use and conservation threats in the Terai Arc Landscape of Nepal
Dhami, Bijaya, Neupane, Bijaya, K.C., Nishan, Maraseni, Tek, Basyal, Chitra Rekha, Joshi, Laxmi Raj and Adhikari, Hari. 2023. "Ecological factors associated with hispid hare (Caprolagus hispidus) habitat use and conservation threats in the Terai Arc Landscape of Nepal." Global Ecology and Conservation. 43. https://doi.org/10.1016/j.gecco.2023.e02437Virtual fencing of captive Asian elephants fitted with an aversive geofencing device to manage their movement
Cabral de Mel, Surendranie J., Seneweera, Saman, de Mel, Ruvinda K., Medawala, Mihiran, Abeysinghe, Navod, Dangolla, Ashoka, Weerakoon, Devaka K., Maraseni, Tek and Allen, Benjamin L.. 2023. "Virtual fencing of captive Asian elephants fitted with an aversive geofencing device to manage their movement." Applied Animal Behaviour Science. 258. https://doi.org/10.1016/j.applanim.2022.105822Improving soil health for farm profits through organics
Culas, Richard, Anwar, Muhuddin, McGrath, Nick, Southwell, Alison, Diack, James, King, Helen, Maraseni, Tek, Storer, Christine and Godfrey, Sosheel. 2023. "Improving soil health for farm profits through organics." 2023 Soil Science Australia National Conference . Darwin, Australia 25 - 30 Jun 2023 Australia.Forest Governance in Nepal concerning Sustainable Community Forest Management and Red Panda Conservation
Cadman, Timothy, Maraseni, Tek, Koju, Upama Ashish, Shrestha, Anita and Karki, Sikha. 2023. "Forest Governance in Nepal concerning Sustainable Community Forest Management and Red Panda Conservation." Land. 12 (2). https://doi.org/10.3390/land12020493Rationalizing donations and subsidies: Energy ecosystem development for sustainable renewable energy transition in Nepal
Bhattarai, Utsav, Maraseni, Tek, Apan, Armando and Devkota, Laxmi Prasad. 2023. "Rationalizing donations and subsidies: Energy ecosystem development for sustainable renewable energy transition in Nepal." Energy Policy. 177. https://doi.org/10.1016/j.enpol.2023.113570Preference, perceived change, and professed relationship among ecosystem services in the Himalayas
Aryal, Kishor, Maraseni, Tek and Apan, Armando. 2023. "Preference, perceived change, and professed relationship among ecosystem services in the Himalayas." Journal of Environmental Management. 344. https://doi.org/10.1016/j.jenvman.2023.118522Examining policy− institution− program (PIP) responses against the drivers of ecosystem dynamics. A chronological review (1960–2020) from Nepal
Aryal, Kishor, Maraseni, Tek and Apan, Armando. 2023. "Examining policy− institution− program (PIP) responses against the drivers of ecosystem dynamics. A chronological review (1960–2020) from Nepal." Land Use Policy: the international journal covering all aspects of land use. 132, p. 106789. https://doi.org/10.1016/j.landusepol.2023.106789Spatial dynamics of biophysical trade-offs and synergies among ecosystem services in the Himalayas
Aryal, Kishor, Maraseni, Tek and Apan, Armando. 2023. "Spatial dynamics of biophysical trade-offs and synergies among ecosystem services in the Himalayas." Ecosystem Services: science, policy and pratice. 59. https://doi.org/10.1016/j.ecoser.2022.101503Calibrating Nepal's scientific forest management practices in the measure of forest restoration
Aryal, Kishor, Awasthi, Nripesh, Maraseni, Tek, Laudari, Hari Krishna, Gotame, Pabitra and Bist, Dhan Bahadur. 2023. "Calibrating Nepal's scientific forest management practices in the measure of forest restoration." Land Use Policy: the international journal covering all aspects of land use. 127. https://doi.org/10.1016/j.landusepol.2023.106586Comparing global and local land cover maps for ecosystem management in the Himalayas
Aryal, Kishor, Apan, Armando and Maraseni, Tek. 2023. "Comparing global and local land cover maps for ecosystem management in the Himalayas." Remote Sensing Applications: Society and Environment. 30. https://doi.org/10.1016/j.rsase.2023.100952Climate‐driven decline in the habitat of the endemic spiny babbler (Turdoides nipalensis)
Adhikari, Binaya, Subedi, Suresh C., Bhandari, Shivish, Baral, Kedar, Lamichhane, Sandesh and Maraseni, Tek. 2023. "Climate‐driven decline in the habitat of the endemic spiny babbler (Turdoides nipalensis)." Ecosphere. 14 (6). https://doi.org/10.1002/ecs2.4584The human-elephant conflict in Sri Lanka: history and present status
Gunawansa, Thakshila, Perera, Kithsiri, Apan, Armando and Hettiarachchi, Nandita K.. 2023. "The human-elephant conflict in Sri Lanka: history and present status." Biodiversity and Conservation. 32 (10), pp. 3025-3052. https://doi.org/10.1007/s10531-023-02650-7Convolutional Neural Network-Based Deep Learning Approach for Automatic Flood Mapping Using NovaSAR-1 and Sentinel-1 Data
Apan, Armando, Andrew, Ogbaje, Paudyal, Dev Raj and Perera, Kithsiri. 2023. "Convolutional Neural Network-Based Deep Learning Approach for Automatic Flood Mapping Using NovaSAR-1 and Sentinel-1 Data." ISPRS International Journal of Geo-Information. 12 (194), pp. 1-26. https://doi.org/https://doi.org/10.3390/ijgi12050194Modelling future spatial distribution of peanut crops in Australia under climate change scenarios
Haerani, Haerani, Apan, Armando, Nguyen-Huy, Thong and Basnet, Badri. 2023. "Modelling future spatial distribution of peanut crops in Australia under climate change scenarios." Geo-spatial Information Science. https://doi.org/10.1080/10095020.2022.2155255Factors affecting the occupancy of Chinese pangolins (Manis pentadactyla) suggest a highly specialized ecological niche
Dhami, Bijaya, Neupane, Bijaya, Devkota, Bishnu Prasad, Maraseni, Tek, Sadadev, Bipana Maiya, Bista, Shreyashi, Adhikari, Amit, Chhetri, Nar Bahadur, Panta, Melina and Stewart, Alyssa B.. 2023. "Factors affecting the occupancy of Chinese pangolins (Manis pentadactyla) suggest a highly specialized ecological niche." Ecosphere. 14 (1). https://doi.org/10.1002/ecs2.4356A call for ‘management authorship’ in community forestry
Aryal, Kishor, Maraseni, Tek and Apan, Armando. 2023. "A call for ‘management authorship’ in community forestry." Environmental Science and Policy. 139, pp. 204-208. https://doi.org/10.1016/j.envsci.2022.10.023Transforming agroforestry in contested landscapes: A win-win solution to trade-offs in ecosystem services in Nepal
Aryal, Kishor, Maraseni, Tek and Apan, Armando. 2023. "Transforming agroforestry in contested landscapes: A win-win solution to trade-offs in ecosystem services in Nepal." Science of the Total Environment. 857 (Part 1). https://doi.org/10.1016/j.scitotenv.2022.159301Deep learning U-Net classification of Sentinel-1 and 2 fusions effectively demarcates tropical montane forest's deforestation
Altarez, Richard Dein D., Apan, Armando and Maraseni, Tek. 2023. "Deep learning U-Net classification of Sentinel-1 and 2 fusions effectively demarcates tropical montane forest's deforestation." Remote Sensing Applications: Society and Environment. 29. https://doi.org/10.1016/j.rsase.2022.100887Challenges and opportunities of forest management and silviculture
Baral, Sony, Basnyat, Bijendra, Dangal, Shambhu P., Khatri, Dil B., Poudyal, Bishnu Hari, Baral, Srijana, Maraseni, Tek N., Pokheral, Ridish K., Kafle, Megh Raj and Karna, Akhileswar L.. 2022. Challenges and opportunities of forest management and silviculture. Nepal. Forest Action Nepal.Sustaining ecosystem based adaptation: the lessons from policy and practices in Nepal
Bhattarai, Sushma, Regmi, Bimal Raj, Pant, Basant, Uprety, Dharam Raj and Maraseni, Tek. 2021. "Sustaining ecosystem based adaptation: the lessons from policy and practices in Nepal." Land Use Policy: the international journal covering all aspects of land use. 104, pp. 1-10. https://doi.org/10.1016/j.landusepol.2021.105391Current and Future Approaches to Mitigate Conflict between Humans and Asian Elephants: The Potential Use of Aversive Geofencing Devices
Cabral de Mel, Surendranie Judith, Seneweera, Saman, de Mel, Ruvinda Kasun, Dangolla, Ashoka, Weerakoon, Devaka Keerthi, Maraseni, Tek and Allen, Benjamin Lee. 2022. "Current and Future Approaches to Mitigate Conflict between Humans and Asian Elephants: The Potential Use of Aversive Geofencing Devices." Animals. 12 (21), pp. 1-29. https://doi.org/10.3390/ani12212965Achieving Water, Energy, and Food Security in Nepal Through Nexus Approach to Planning and Development
Upreti, Gopi, Timsina, Jagadish and Maraseni, Tek N.. 2022. "Achieving Water, Energy, and Food Security in Nepal Through Nexus Approach to Planning and Development." Timsina, Jadadish, Maraseni, Tek N., Gauchan, Devendra, Adhikari, Jagannath and Ojha, Hemant (ed.) Agriculture, Natural Resources and Food Security: Lessons from Nepal. Cham, Switzerland. Springer. pp. 397-414Assessing the Potential of Agroforestry in Nepal: Socio-economic and Environmental Perspectives
Dhakal, Arun, Maraseni, Tek N. and Timsina, Jagadish. 2022. "Assessing the Potential of Agroforestry in Nepal: Socio-economic and Environmental Perspectives." Timsina, Jadadish, Maraseni, Tek N., Gauchan, Devendra, Adhikari, Jagannath and Ojha, Hemant (ed.) Agriculture, Natural Resources and Food Security: Lessons from Nepal. Cham, Switzerland. Springer. pp. 375-394An Assessment of Selective Logging Policies and Practices in Nepal
Poudyal, Bishnu H., Maraseni, Tek N., Aryal, Suman and Cockfield, Geoff. 2022. "An Assessment of Selective Logging Policies and Practices in Nepal." Timsina, Jagadish, Maraseni, Tek N., Gauchan, Devendra, Adhikari, Jagannath and Ojha, Hemant (ed.) Agriculture, Natural Resources and Food Security: Lessons from Nepal. Cham, Switzerland. Springer. pp. 357-374Changes in Transhumance Systems in Nepal: Analysing Socio-ecological Impacts Using Driver-Pressure-State-Impact-Response Framework
Aryal, Suman, Maraseni, Tek N. and Cockfield, Geoff. 2022. "Changes in Transhumance Systems in Nepal: Analysing Socio-ecological Impacts Using Driver-Pressure-State-Impact-Response Framework." Timsina, Jadadish, Maraseni, Tek N., Gauchan, Devendra, Adhikari, Jagannath and Ojha, Hemant (ed.) Agriculture, Natural Resources and Food Security: Lessons from Nepal. Cham, Switzerland. Springer. pp. 297-314Introduction and Overview
Timsina, Jagadish, Maraseni, Tek N., Gauchan, Devendra, Adhikari, Jagannath and Ojha, Hemant. 2022. "Introduction and Overview." Timsina, Jadadish, Maraseni, Tek N., Gauchan, Devendra, Adhikari, Jagannath and Ojha, Hemant (ed.) Agriculture, Natural Resources and Food Security: Lessons from Nepal. Cham, Switzerland. Springer. pp. 1-16Evaluating the governance of sustainable development: The quality and legitimacy of the blue economy
Maraseni, Tek, Karki, Sikha, Koju, Upama, Shrestha, Anita and Cadman, Timothy. 2022. "Evaluating the governance of sustainable development: The quality and legitimacy of the blue economy." Cadman, Timothy and Sarkar, Tapan (ed.) De Gruyter Handbook of Sustainable Development and Finance. Germany. Walter de Gruyter. pp. 567-597Vegetation loss and recovery analysis from the 2015 Gorkha earthquake (7.8 Mw) triggered landslides
Pandey, Hari Prasad, Gnyawali, Kaushal, Dahal, Kshitij, Pokhrel, Narayan Prasad and Maraseni, Tek Narayan. 2022. "Vegetation loss and recovery analysis from the 2015 Gorkha earthquake (7.8 Mw) triggered landslides." Land Use Policy: the international journal covering all aspects of land use. 119, pp. 1-10. https://doi.org/10.1016/j.landusepol.2022.106185Student Performance Predictions for Advanced Engineering Mathematics Course With New Multivariate Copula Models
Nguyen-Huy, Thong, Deo, Ravinesh C., Khan, Shahjahan, Devi, Aruna, Adeyinka, Adewuyi Ayodele, Apan, Armando A. and Yaseen, Zaher Mundher. 2022. "Student Performance Predictions for Advanced Engineering Mathematics Course With New Multivariate Copula Models." IEEE Access. 10, pp. 45112 -45136. https://doi.org/10.1109/ACCESS.2022.3168322Assay of renewable energy transition: A systematic literature review
Bhattarai, Utsav, Maraseni, Tek and Apan, Armando. 2022. "Assay of renewable energy transition: A systematic literature review." Science of the Total Environment. 833, pp. 1-18. https://doi.org/10.1016/j.scitotenv.2022.155159Rapid assessment of mine rehabilitation areas with airborne LiDAR and deep learning: bauxite strip mining in Queensland, Australia
Murray, Xavier, Apan, Armando, Deo, Ravinesh and Maraseni, Tek. 2022. "Rapid assessment of mine rehabilitation areas with airborne LiDAR and deep learning: bauxite strip mining in Queensland, Australia." Geocarto International. 37 (26), pp. 11223-11252. https://doi.org/10.1080/10106049.2022.2048902Spaceborne satellite remote sensing of tropical montane forests: a review of applications and future trends
Altarez, Richard Dein D., Apan, Armando and Maraseni, Tek. 2022. "Spaceborne satellite remote sensing of tropical montane forests: a review of applications and future trends." Geocarto International. 37 (26), pp. 11900-11928. https://doi.org/10.1080/10106049.2022.2060330Growth dynamics of Shorea robusta Gaertn in relation to climate change: a case study from tropical region of Nepal
Baral, Sony, Gaire, Narayan Prasad, Giri, Anjana, Maraseni, Tek, Basnyat, Bijendra, Paudel, Ambika, Kunwar, Ripu, Rayamajhi, Santosh, Basnet, Saroj, Sharma, Shiva K., Khadka, Chiranjeewee and Vacik, Harald. 2022. "Growth dynamics of Shorea robusta Gaertn in relation to climate change: a case study from tropical region of Nepal." Trees: structure and function. 36 (4), pp. 1425-1436. https://doi.org/10.1007/s00468-022-02300-5When Entrepreneurial Leadership Identity and Passion Meet Venture Growth Intention
Dhakal, Purushottam, Wiesner, Retha and Maraseni, Tek. 2022. "When Entrepreneurial Leadership Identity and Passion Meet Venture Growth Intention." Sustainability. 14 (20), pp. 1-20. https://doi.org/10.3390/su142013129Navigating policy debates of and discourse coalitions on Nepal's Scientific Forest Management
Aryal, Kishor, Laudari, Hari Krishna, Maraseni, Tek and Pathak, Bhoj Raj. 2022. "Navigating policy debates of and discourse coalitions on Nepal's Scientific Forest Management." Forest Policy and Economics. 141. https://doi.org/10.1016/j.forpol.2022.102768Efficient water management and irrigation on farms
Reardon-Smith, K., Mushtaq, Shahbaz, Scobie, M., Eberhard, J. and Maraseni, T. N.. 2022. "Efficient water management and irrigation on farms." Sims, Ralph E. H. (ed.) Energy-smart farming: efficiency, renewable energy and sustainability. United Kingdom. Burleigh Dodds Science Publishing Limited. pp. 102-134City-Level Determinants of Household CO2 Emissions per Person: An Empirical Study Based on a Large Survey in China
Qu, Jiansheng, Liu, Lina, Zeng, Jingjing, Maraseni, Tek Narayan and Zhang, Zhiqiang. 2022. "City-Level Determinants of Household CO2 Emissions per Person: An Empirical Study Based on a Large Survey in China." Land. 11 (6). https://doi.org/10.3390/land11060925What determines attitude of local people towards tiger and leopard in Nepal?
Dhungana, Rajendra, Maraseni, Tek, Silwal, Thakur, Aryal, Kishor and Karki, Jhamak Bahadur. 2022. "What determines attitude of local people towards tiger and leopard in Nepal?" Journal for Nature Conservation. 68. https://doi.org/10.1016/j.jnc.2022.126223How will hydro-energy generation of the Nepalese Himalaya vary in the future? A climate change perspective
Bhattarai, Utsav, Devkota, Laxmi Prasad, Marahatta, Suresh, Shrestha, Dibesh and Maraseni, Tek. 2022. "How will hydro-energy generation of the Nepalese Himalaya vary in the future? A climate change perspective." Environmental Research. 214 (1), pp. 1-13. https://doi.org/10.1016/j.envres.2022.113746Stakeholder perspectives on the effectiveness of governance in red panda conservation programmes in Nepal: a comparative analysis
Shrestha, Anita, Karki, Sikha, Koju, Upama, Maraseni, Tek, Gautam, Ambika P., Cadman, Tim and Baral, Sony. 2022. "Stakeholder perspectives on the effectiveness of governance in red panda conservation programmes in Nepal: a comparative analysis." Ecosystems and People. 18 (1), pp. 547-565. https://doi.org/10.1080/26395916.2022.2121762Applying landscape-level principles to koala management in Australia: a comparative analysis
Schlagloth, Rolf, Morgan, Edward A., Cadman, Timothy, Santamaria, Flavia, McGinnis, Gabrielle, Thomson, Hedley, Kerlin, Douglas H., Maraseni, Tek Narayan, Cahir, Fred, Clark, Ian D., Clode, Danielle and Mcewan, Alexandra. 2024. "Applying landscape-level principles to koala management in Australia: a comparative analysis." Journal of Environmental Planning and Management. 67 (3), pp. 542-563. https://doi.org/10.1080/09640568.2022.2124154Detection of calcium, magnesium, and chlorophyll variations of wheat genotypes on sodic soils using hyperspectral red edge parameters
Choudhury, Malini Roy, Christopher, Jack, Das, Sumanta, Apan, Armando, Menzies, Neal W., Chapman, Scott, Mellor, Vincent and Dang, Yash P.. 2022. "Detection of calcium, magnesium, and chlorophyll variations of wheat genotypes on sodic soils using hyperspectral red edge parameters." Environmental Technology and Innovation. 27, pp. 1-14. https://doi.org/10.1016/j.eti.2022.102469Impact of COVID-19 in the forestry sector: A case of lowland region of Nepal
Maraseni, Tek, Poudyal, Bishnu Hari, Aryal, Kishor and Laudari, Hari Krishna. 2022. "Impact of COVID-19 in the forestry sector: A case of lowland region of Nepal." Land Use Policy: the international journal covering all aspects of land use. 120. https://doi.org/10.1016/j.landusepol.2022.106280Sixty-five years of forest restoration in Nepal: Lessons learned and way forward
Laudari, Hari Krishna, Aryal, Kishor, Maraseni, Tek, Pariyar, Shiva, Pant, Bashant, Bhattarai, Sushma, Kaini, Tika Raj, Karki, Gyandendra and Marahattha, Anisha. 2022. "Sixty-five years of forest restoration in Nepal: Lessons learned and way forward." Land Use Policy: the international journal covering all aspects of land use. 115. https://doi.org/10.1016/j.landusepol.2022.106033Identifying and prioritising climate change adaptation actions for greater one-horned rhinoceros (Rhinoceros unicornis) conservation in Nepal
Pant, Ganesh, Maraseni, Tek, Apan, Armando and Allen, Benjamin L.. 2022. "Identifying and prioritising climate change adaptation actions for greater one-horned rhinoceros (Rhinoceros unicornis) conservation in Nepal." PEERJ. 10. https://doi.org/10.7717/peerj.12795How much do we know about trade-offs in ecosystem services? A systematic review of empirical research observations
Aryal, Kishor, Maraseni, Tek N. and Apan, Armando. 2022. "How much do we know about trade-offs in ecosystem services? A systematic review of empirical research observations." Science of the Total Environment. 806, pp. 1-13. https://doi.org/10.1016/j.scitotenv.2021.151229Stakeholder participation in IPBES: connecting local environmental work with global decision making
Krug, Cornelia B., Sterling, Eleanor, Cadman, Timothy, Geschke, Jonas, Schliep, Rainer, Drummond de Castro, Paula F., Osemwegie, Isimemen, Muller-Karger, Frank E. and Maraseni, Tek. 2020. "Stakeholder participation in IPBES: connecting local environmental work with global decision making." Ecosystems and People. 16 (1), pp. 197-211. https://doi.org/10.1080/26395916.2020.1788643Effectiveness of community forest user groups (CFUGs) in responding to the 2015 earthquakes and COVID-19 in Nepal
Gentle, Popular, Maraseni, Tek N., Paudel, Dinesh, Dahal, Ganga R., Kanel, Tara and Pathak, Bharati. 2020. "Effectiveness of community forest user groups (CFUGs) in responding to the 2015 earthquakes and COVID-19 in Nepal." Research in Globalization. 2, pp. 1-5. https://doi.org/10.1016/j.resglo.2020.100025Evaluation of drought tolerance of wheat genotypes in rain-fed sodic soil environments using high-resolution UAV remote sensing techniques
Das, Sumanta, Christopher, Jack, Choudhury, Malini Roy, Apan, Armando, Chapman, Scott, Menzies, Neal W. and Dang, Yash P.. 2022. "Evaluation of drought tolerance of wheat genotypes in rain-fed sodic soil environments using high-resolution UAV remote sensing techniques." Biosystems Engineering. 217, pp. 68-82. https://doi.org/10.1016/j.biosystemseng.2022.03.004Globalisation and traditional social-ecological systems: Understanding impacts of tourism and labour migration to the transhumance systems in the Himalayas
Aryal, Suman, Cockfield, Geoff and Maraseni, Tek Narayan. 2018. "Globalisation and traditional social-ecological systems: Understanding impacts of tourism and labour migration to the transhumance systems in the Himalayas." Environmental Development. 25, pp. 73-84. https://doi.org/10.1016/j.envdev.2017.09.001The sugarcane industry in Nepal: Opportunities and challenges
Neupane, Prem Raj, Maraseni, Tek Narayan and Kohl, Michael. 2017. "The sugarcane industry in Nepal: Opportunities and challenges." Environmental Development. 24, pp. 86-98. https://doi.org/10.1016/j.envdev.2017.02.001Status and Practical Implications of Forest Fire Management in Nepal
Pandey, Hari Prasad, Pokhrel, Narayan Prasad, Thapa, Prakash, Paudel, Naya Sharma and Maraseni, Tek Narayan. 2022. "Status and Practical Implications of Forest Fire Management in Nepal." Journal of Forest and Livelihood. 21 (1), pp. 32-45.Predicted declines in suitable habitat for greater one-horned rhinoceros (Rhinoceros unicornis) under future climate and land use change scenarios
Pant, Ganesh, Maraseni, Tek, Apan, Armando and Allen, Benjamin L.. 2021. "Predicted declines in suitable habitat for greater one-horned rhinoceros (Rhinoceros unicornis) under future climate and land use change scenarios." Ecology and Evolution. 11 (24), pp. 18288-18304. https://doi.org/10.1002/ece3.8421Capacity building at community forestry level for synergistic implementation of NDCs’ adaptation and mitigation commitments
Shivakoti, Binay Raj, Lopez-Casero, Federico, Maraseni, Tek and Pokharel, Krishna. 2021. "Capacity building at community forestry level for synergistic implementation of NDCs’ adaptation and mitigation commitments." APN Science Bulletin. 11 (1), pp. 113-124. https://doi.org/10.30852/sb.2021.1652Application of Sentinel-2 Satellite Data to Map Forest Cover in Southeast Sri Lanka through the Random Forest Classifier
Gunawansa, Thakshila, Perera, Kithsiri, Apan, Armando and Hettiarachchi, Nandita. 2022. "Application of Sentinel-2 Satellite Data to Map Forest Cover in Southeast Sri Lanka through the Random Forest Classifier." Journal of Advances in Engineering and Technology. I (I), pp. 049-1 to 049-10.Modelling Floodplain Vegetation Response to Groundwater Variability Using the ArcSWAT Hydrological Model, MODIS NDVI Data, and Machine Learning
Muhury, Newton, Apan, Armando A., Maraseni, Tek N. and Ayele, Gebiaw T.. 2022. "Modelling Floodplain Vegetation Response to Groundwater Variability Using the ArcSWAT Hydrological Model, MODIS NDVI Data, and Machine Learning." Land. 11 (12), pp. 1-23. https://doi.org/10.3390/land11122154Mapping Prominent Cash Crops Employing ALOS PALSAR-2 and Selected Machine Learners
Panuju, Dyah R., Haerani, H., Apan, Armando, Griffin, Amy L., Paull, David J. and Trisasongko, Bambang Hendro. 2022. "Mapping Prominent Cash Crops Employing ALOS PALSAR-2 and Selected Machine Learners." Kumar, Arvind, Kumar, Pavan, Singh, S. S., Trisasongko, Bambang Hendro and Rani, Meenu (ed.) Agriculture, Livestock Production and Aquaculture: Advances for Smallholder Farming Systems. Cham, Switzerland. Springer. pp. 131-146Improving Biomass and Grain Yield Prediction of Wheat Genotypes on Sodic Soil Using Integrated High-Resolution Multispectral, Hyperspectral, 3D Point Cloud, and Machine Learning Techniques
Choudhury, Malini Roy, Das, Sumanta, Christopher, Jack, Apan, Armando, Chapman, Scott, Menzies, Neal W. and Dang, Yash P.. 2021. "Improving Biomass and Grain Yield Prediction of Wheat Genotypes on Sodic Soil Using Integrated High-Resolution Multispectral, Hyperspectral, 3D Point Cloud, and Machine Learning Techniques." Remote Sensing. 13 (17), pp. 1-27. https://doi.org/10.3390/rs13173482Implementation effect of municipal solid waste mandatory sorting policy in Shanghai
Wang, Yao, Shi, Yu, Zhou, Jizhi, Zhao, Jun, Maraseni, Tek and Qian, Guangren. 2021. "Implementation effect of municipal solid waste mandatory sorting policy in Shanghai." Journal of Environmental Management. 298, pp. 1-7. https://doi.org/10.1016/j.jenvman.2021.113512Ecoefficiency of China's agricultural sector: what are the spatiotemporal characteristics and how are they determined?
Zeng, Jinjing, Han, Jinyu, Qu, Jiansheng, Maraseni, Tek Narayan, Xu, Li, Li, Hengji and Liu, Lina. 2021. "Ecoefficiency of China's agricultural sector: what are the spatiotemporal characteristics and how are they determined?" Journal of Cleaner Production. 325, pp. 1-11. https://doi.org/10.1016/j.jclepro.2021.129346The effects of tunnel technology on crop productivity and livelihood of smallholder farmers in Nepal
KC, Diwakar, Jamarkattel, Dinesh, Maraseni, Tek, Nandwani, Dilip and Karki, Pratibha. 2021. "The effects of tunnel technology on crop productivity and livelihood of smallholder farmers in Nepal." Sustainability. 13 (14), pp. 1-15. https://doi.org/10.3390/su13147935UAV-thermal imaging: A technological breakthrough for monitoring and quantifying crop abiotic stress to help sustain productivity on sodic soils – A case review on wheat
Das, Sumanta, Chapman, Scott, Christopher, Jack, Choudhury, Malini Roy, Menzies, Neal W., Apan, Armando and Dang, Yash P.. 2021. "UAV-thermal imaging: A technological breakthrough for monitoring and quantifying crop abiotic stress to help sustain productivity on sodic soils – A case review on wheat." Remote Sensing Applications: Society and Environment. 23, pp. 1-13. https://doi.org/10.1016/j.rsase.2021.100583A critical assessment of provincial-level variation in agricultural GHG emissions in China
Han, Jinyu, Qu, Jiansheng, Maraseni, Tek Narayan, Xu, Li, Zeng, Jingjing and Li, Hengji. 2021. "A critical assessment of provincial-level variation in agricultural GHG emissions in China." Journal of Environmental Management. 296, pp. 1-10. https://doi.org/10.1016/j.jenvman.2021.113190COVID-19 lockdown and the forestry sector: insight from Gandaki province of Nepal
Laudari, Hari Krishna, Pariyar, Shiva and Maraseni, Tek. 2021. "COVID-19 lockdown and the forestry sector: insight from Gandaki province of Nepal." Forest Policy and Economics. 131, pp. 1-12. https://doi.org/10.1016/j.forpol.2021.102556Improving estimation of in-season crop water use and health of wheat genotypes on sodic soils using spatial interpolation techniques and multi-component metrics
Choudhury, Malini Roy, Mellor, Vincent, Das, Sumanta, Christopher, Jack, Apan, Armando, Menzies, Neal W., Chapman, Scott and Dang, Yash P.. 2021. "Improving estimation of in-season crop water use and health of wheat genotypes on sodic soils using spatial interpolation techniques and multi-component metrics." Agricultural Water Management. 255. https://doi.org/10.1016/j.agwat.2021.107007Evaluation of water status of wheat genotypes to aid prediction of yield on sodic soils using UAV-thermal imaging and machine learning
Das, Sumanta, Christopher, Jack, Apan, Armando, Choudhury, Malini Roy, Chapman, Scott, Menzies, Neal W. and Dang, Yash P.. 2021. "Evaluation of water status of wheat genotypes to aid prediction of yield on sodic soils using UAV-thermal imaging and machine learning." Agricultural and Forest Meteorology. 307. https://doi.org/10.1016/j.agrformet.2021.108477UAV-thermal imaging and agglomerative hierarchical clustering techniques to evaluate and rank physiological performance of wheat genotypes on sodic soil
Das, Sumanta, Christopher, Jack, Apan, Armando, Choudhury, Malini Roy, Chapman, Scott, Menzies, Neal W. and Dang, Yash P.. 2021. "UAV-thermal imaging and agglomerative hierarchical clustering techniques to evaluate and rank physiological performance of wheat genotypes on sodic soil." ISPRS Journal of Photogrammetry and Remote Sensing. 173, pp. 221-237. https://doi.org/10.1016/j.isprsjprs.2021.01.014Response and resilience of Asian agrifood systems to COVID-19: an assessment across twenty-five countries and four regional farming and food systems
Dixon, John M., Weerahewa, Jeevika, Hellin, Jon, Rola-Rubzen, Maria Fay, Huang, Jikun, Kumar, Shalander, Das, Anup, Qureshi, Muhammad Ejaz, Krupnik, Timothy J., Shideed, Kamil, Jat, Mangi L., Prasad, P. V. Vara, Yadav, Sudhir, Irshad, Aamer, Asanaliev, Abdybek, Abugalieva, Aigul, Karimov, Aziz, Bhattarai, Basundhara, Balgos, Carol Q., ..., Timsina, Jagdish. 2021. "Response and resilience of Asian agrifood systems to COVID-19: an assessment across twenty-five countries and four regional farming and food systems." Agricultural Systems. 193, pp. 1-19. https://doi.org/10.1016/j.agsy.2021.103168Perceived importance and economic valuation of ecosystem services in Ghodaghodi wetland of Nepal
Aryal, Kishor, Ojha, Bhuwan Raj and Maraseni, Tek. 2021. "Perceived importance and economic valuation of ecosystem services in Ghodaghodi wetland of Nepal." Land Use Policy: the international journal covering all aspects of land use. 106, pp. 1-12. https://doi.org/10.1016/j.landusepol.2021.105450Unbalanced status and multidimensional influences of municipal solid waste management in Africa
Shi, Yu, Wang, Yao, Yue, Yang, Zhao, Jun, Maraseni, Tek and Qian, Guangren. 2021. "Unbalanced status and multidimensional influences of municipal solid waste management in Africa." Chemosphere. 281, pp. 1-11. https://doi.org/10.1016/j.chemosphere.2021.130884Estimating the willingness to pay for regulating and cultural ecosystem services from forested Siwalik landscapes: perspectives of disaggregated users
Acharya, Ram Prasad, Maraseni, Tek Narayan and Cockfield, Geoff. 2021. "Estimating the willingness to pay for regulating and cultural ecosystem services from forested Siwalik landscapes: perspectives of disaggregated users." Annals of Forest Science. 78, pp. 1-27. https://doi.org/10.1007/s13595-021-01046-3Who shapes the environmental policy in the global south? Unpacking the reality of Nepal
Aryal, Kishor, Laudari, Hari Krishna, Neupane, Prem Raj and Maraseni, Tek. 2021. "Who shapes the environmental policy in the global south? Unpacking the reality of Nepal." Environmental Science and Policy. 121, pp. 78-88. https://doi.org/10.1016/j.envsci.2021.04.008Reaching over the gap: a review of trends in and status of Red Panda research over 193 years (1827-2020)
Karki, Sikha, Maraseni, Tek, Mackey, Brendan, Bista, Damber, Lama, Sonam Tashi, Gautam, Ambika P., Sherpa, Ang Phuri, Koju, Upama, Shrestha, Anita and Cadman, Tim. 2021. "Reaching over the gap: a review of trends in and status of Red Panda research over 193 years (1827-2020)." Science of the Total Environment. 781, pp. 1-12. https://doi.org/10.1016/j.scitotenv.2021.146659Agriculture: energy use and conservation
Chen, Guangnan and Maraseni, Tek Narayan. 2021. "Agriculture: energy use and conservation." Fath, Brian D., Jorgensen, Sven E. and Cole, Megan (ed.) Managing soils and terrestrial systems. Boca Raton, FL, United States. CRC Press. pp. 9-18Forest Carbon Storage and Species Richness in FSC Certified and Non-certified Community Forests in Nepal
Charmakar, Shambhu, Oli, Bishwa Nath, Joshi, Nabin Raj, Maraseni, Tek Narayan and Atreya, Kishor. 2021. "Forest Carbon Storage and Species Richness in FSC Certified and Non-certified Community Forests in Nepal." Small-Scale Forestry. 20 (2), pp. 199-219. https://doi.org/10.1007/s11842-020-09464-3Species composition, diversity, and carbon stock in trees outside forests in middle hills of Nepal
Bhandari, Shes Kanta, Maraseni, Tek, Timilsina, Yajna Prasad and Parajuli, Rajan. 2021. "Species composition, diversity, and carbon stock in trees outside forests in middle hills of Nepal." Forest Policy and Economics. 125, pp. 1-8. https://doi.org/10.1016/j.forpol.2021.102402Strengthening inter-sectoral coordination and governance for the effective implementation of Nepal's NDCs based on a voluntary national quality-of governance standard for forest sector activities and programmes
Lopez-Casero, Federico, Shivakoti, Binaya Raj, Maraseni, Tek and Pokheral, Krishna. 2021. Strengthening inter-sectoral coordination and governance for the effective implementation of Nepal's NDCs based on a voluntary national quality-of governance standard for forest sector activities and programmes. Japan. Asia-Pacific Network for Global Change Research.Ethnobotany of the Himalayas: The Nepal, Bhutanese, and Tibetan Himalayas
Kunwar, Ripu M., Subedi, Bhishma P., Baral, Sushim R., Maraseni, Tek, LeBoa, Chris, Adhikari, Yagya P. and Bussmann, Rainer W.. 2020. "Ethnobotany of the Himalayas: The Nepal, Bhutanese, and Tibetan Himalayas." Kunwar, R.M., Sher, H. and Bussmann, R.W. (ed.) Ethnobotany of the Himalayas. Cham, Switzerland. Springer. pp. 65-103Key steps in environmental impact assessment: a comparative study of China, Queensland State of Australia and Nepal
Aryal, Suman, Maraseni, Tek, Qu, Jiansheng, Bruyn, Lisa Lobry de, Dhakal, Yub Raj and Zeng, Jingjing. 2020. "Key steps in environmental impact assessment: a comparative study of China, Queensland State of Australia and Nepal." Environmental Monitoring and Assessment. 192, pp. 1-15. https://doi.org/10.1007/s10661-020-8098-4Resident risk attitude analysis in the decision-making management of waste incineration construction
Nie, Yongyou, Wu, Yanjing, Zhao, Jinbu, Zhou, Jizhi, Zhang, Yuhao, Zhao, Jun, Maraseni, Tek and Qian, Guangren. 2020. "Resident risk attitude analysis in the decision-making management of waste incineration construction." Journal of Environmental Management. 258, pp. 1-6. https://doi.org/10.1016/j.jenvman.2019.109946Why is the Private Forest Program Stunted in Nepal?
Aryal, Kishor, Rijal, Arjun, Maraseni, Tek and Parajuli, Manisha. 2020. "Why is the Private Forest Program Stunted in Nepal?" Environmental Management (New York): an international journal for decision-makers, scientists and environmental auditors. 66, pp. 535-548. https://doi.org/10.1007/s00267-020-01343-zHousehold CO2 Emissions: Current Status and Future Perspectives
Liu, Lina, Qu, Jiansheng, Maraseni, Tek Narayan, Niu, Yibo, Zeng, Jingjing, Zhang, Lihua and Xu, Li. 2020. "Household CO2 Emissions: Current Status and Future Perspectives." International Journal of Environmental Research and Public Health. 17 (19), pp. 1-19. https://doi.org/10.3390/ijerph17197077An Ecosystem Services Valuation Research Framework for Policy Integration in Developing Countries: A Case Study from Nepal
Acharya, Ram Prasad, Maraseni, Tek Narayan and Cockfield, Geoff. 2020. "An Ecosystem Services Valuation Research Framework for Policy Integration in Developing Countries: A Case Study from Nepal." Sustainability. 12 (19), pp. 1-15. https://doi.org/10.3390/su12198250Carbon smart agriculture: An integrated regional approach offers significant potential to increase profit and resource use efficiency, and reduce emissions
Maraseni, Tek, An-Vo, Duc-Anh, Mushtaq, Shahbaz and Reardon-Smith, Kate. 2021. "Carbon smart agriculture: An integrated regional approach offers significant potential to increase profit and resource use efficiency, and reduce emissions." Journal of Cleaner Production. 282, pp. 1-14. https://doi.org/10.1016/j.jclepro.2020.124555Assessing the past and adapting to future floods: a hydro-social analysis
Devkota, Rohini, Bhattarai, Utsav, Devkota, Laxmi and Maraseni, Tek Narayan. 2020. "Assessing the past and adapting to future floods: a hydro-social analysis." Climatic Change: an interdisciplinary, international journal devoted to the description, causes and implications of climatic change. 163 (2), pp. 1065-1082. https://doi.org/10.1007/s10584-020-02909-wWhat lessons do the first Nationally Determined Contribution (NDC) formulation process and implementation outcome provide to the enhanced/updated NDC? A reality check from Nepal
Laudari, Hari Krishna, Aryal, Kishor, Bhusal, Shreejana and Maraseni, Tek. 2021. "What lessons do the first Nationally Determined Contribution (NDC) formulation process and implementation outcome provide to the enhanced/updated NDC? A reality check from Nepal." Science of the Total Environment. 759, pp. 1-12. https://doi.org/10.1016/j.scitotenv.2020.143509Characterizing net primary productivity of forests and non-forest areas in the Philippines using satellite imagery
Apan, Armando and Maraseni, Tek. 2012. "Characterizing net primary productivity of forests and non-forest areas in the Philippines using satellite imagery." 1st National Symposium and Workshop on Environmental Science (IESM 2012): Environmental Science in a Changing Environment. Manila, Philippines 07 - 08 May 2012 Manila, Philippines.Climate change vulnerability of Asia’s most iconic megaherbivore: greater one-horned rhinoceros (Rhinoceros unicornis)
Pant, Ganesh, Maraseni, Tek, Apan, Armando and Allen, Benjamin L.. 2020. "Climate change vulnerability of Asia’s most iconic megaherbivore: greater one-horned rhinoceros (Rhinoceros unicornis)." Global Ecology and Conservation. 23, pp. 1-14. https://doi.org/10.1016/j.gecco.2020.e01180The climate-induced alteration of future geographic distribution of aflatoxin in peanut crops and its adaptation options
Haerani, Haerani, Apan, Armando and Basnet, Badri. 2020. "The climate-induced alteration of future geographic distribution of aflatoxin in peanut crops and its adaptation options." Mitigation and Adaptation Strategies for Global Change. 25 (6), pp. 1149-1175. https://doi.org/10.1007/s11027-020-09927-0Using a Mixed Method Research to Develop Spatial Information Sharing Strategies Across Natural Resource Management Communities
Paudyal, Dev Raj, McDougall, Kevin and Apan, Armando. 2019. "Using a Mixed Method Research to Develop Spatial Information Sharing Strategies Across Natural Resource Management Communities." Inaugural Australasia & Pacific Regional Mixed Methods International Research Association Conference 2019: Expanding Conceptual and Methodological Boundaries (MMIRA 2019). Wellington, New Zealand 04 - 06 Dec 2019 Indianapolis, United States.Intelligent data analytics for time series, trend analysis and drought indices comparison
Dayal, Kavina S., Deo, Ravinesh C. and Apan, Armando A.. 2021. "Intelligent data analytics for time series, trend analysis and drought indices comparison." Deo, Ravinesh C., Samui, Pijush, Kisi, Ozgur and Yaseen, Zaher Mundher (ed.) Intelligent data analytics for decision-support systems in hazard mitigation: theory and practice of hazard mitigation. Singapore. Springer. pp. 151-169Assessing the financial contribution and carbon emission pattern of provisioning ecosystem services in Siwalik forests in Nepal: Valuation from the perspectives of disaggregated users
Acharya, Ram Prasad, Maraseni, Tek and Cockfield, Geoff. 2020. "Assessing the financial contribution and carbon emission pattern of provisioning ecosystem services in Siwalik forests in Nepal: Valuation from the perspectives of disaggregated users." Land Use Policy: the international journal covering all aspects of land use. 95, pp. 1-11. https://doi.org/10.1016/j.landusepol.2020.104647Monitoring rice growth status in the Mekong Delta, Vietnam using multitemporal Sentinel-1 data
Phung, Hoang-Phi, Lam-Dao, Nguyen, Nguyen-Huy, Thong, Le-Toan, Thuy and Apan, Armando A.. 2020. "Monitoring rice growth status in the Mekong Delta, Vietnam using multitemporal Sentinel-1 data." Journal of Applied Remote Sensing. 14 (1), pp. 014518-1-014518-23. https://doi.org/10.1117/1.JRS.14.014518Mapping national REDD+ initiatives in the Asia-Pacific region
Maraseni, Tek Narayan, Poudyal, Bishnu Hari, Rana, Eak, Khanal, Sudarshan Chandra, Ghimire, Puspa Lal and Subedi, Bhishma P.. 2020. "Mapping national REDD+ initiatives in the Asia-Pacific region." Journal of Environmental Management. 269, pp. 1-11. https://doi.org/10.1016/j.jenvman.2020.110763Recognition of historical contribution of indigenous peoples and local communities through benefit sharing plans (BSPs) in REDD+
Poudyal, Bishnu Hari, Maraseni, Tek, Cockfield, Geoff and Bhattarai, Bhola. 2020. "Recognition of historical contribution of indigenous peoples and local communities through benefit sharing plans (BSPs) in REDD+." Environmental Science and Policy. 106, pp. 111-114. https://doi.org/10.1016/j.envsci.2020.01.022Trends and current state of research on greater one-horned rhinoceros (Rhinoceros unicornis): a systematic review of the literature over a period of 33 years (1985–2018)
Pant, Ganesh, Maraseni, Tek, Apan, Armando and Allen, Benjamin L.. 2020. "Trends and current state of research on greater one-horned rhinoceros (Rhinoceros unicornis): a systematic review of the literature over a period of 33 years (1985–2018)." Science of the Total Environment. 710, pp. 1-14. https://doi.org/10.1016/j.scitotenv.2019.136349Land use change in Australian mixed crop-livestock systems as a transformative climate change adaptation
Ghahramani, Afshin, Kingwell, Ross S. and Maraseni, Tek Narayan. 2020. "Land use change in Australian mixed crop-livestock systems as a transformative climate change adaptation." Agricultural Systems. 180. https://doi.org/10.1016/j.agsy.2020.102791A postmortem of forest policy dynamics of Nepal
Laudari, Hari Krishna, Aryal, Kishor and Maraseni, Tek. 2020. "A postmortem of forest policy dynamics of Nepal." Land Use Policy: the international journal covering all aspects of land use. 91, pp. 1-13. https://doi.org/10.1016/j.landusepol.2019.104338An assessment of the policies and practices of selective logging and timber utilisation: A case study from natural forests of Tarai Nepal and Queensland Australia
Poudyal, Bishnu Hari, Maraseni, Tek and Cockfield, Geoff. 2020. "An assessment of the policies and practices of selective logging and timber utilisation: A case study from natural forests of Tarai Nepal and Queensland Australia." Land Use Policy: the international journal covering all aspects of land use. 91, pp. 1-12. https://doi.org/10.1016/j.landusepol.2019.104422Global trend of forest ecosystem services valuation – An analysis of publications
Acharya, Ram Prasad, Maraseni, Tek and Cockfield, Geoff. 2019. "Global trend of forest ecosystem services valuation – An analysis of publications." Ecosystem Services: science, policy and pratice. 39, pp. 1-11. https://doi.org/10.1016/j.ecoser.2019.100979Scientific Forest Management Practice in Nepal: Critical Reflections from Stakeholders' Perspectives
Poudyal, Bishnu Hari, Maraseni, Tek and Cockfield, Geoff. 2019. "Scientific Forest Management Practice in Nepal: Critical Reflections from Stakeholders' Perspectives." Forests. 11 (1), pp. 1-20. https://doi.org/10.3390/f11010027Financial returns from collaborative investment models of Eucalyptus agroforestry plantations in Lao PDR
Phimmavong, Somvang, Maraseni, Tek Narayan, Keenan, Rodney J. and Cockfield, Geoff. 2019. "Financial returns from collaborative investment models of Eucalyptus agroforestry plantations in Lao PDR." Land Use Policy: the international journal covering all aspects of land use. 87, pp. 1-11. https://doi.org/10.1016/j.landusepol.2019.104060An assessment of governance quality for community-based forest management systems in Asia: Prioritisation of governance indicators at various scales
Maraseni, Tek Narayan, Bhattarai, Nabin, Karky, Bhaskar Singh, Cadman, Timothy, Timalsina, Niroj, Bhandari, Trishna Singh, Apan, Armando, Ma, Hwan Ok, Rawat, R. S., Verma, Nemit, San, Su Mon, Oo, Thaung Naing, Dorji, Kinley, Dhungana, Sindhu and Poudel, Mohan. 2019. "An assessment of governance quality for community-based forest management systems in Asia: Prioritisation of governance indicators at various scales." Land Use Policy: the international journal covering all aspects of land use. 81, pp. 750-671. https://doi.org/10.1016/j.landusepol.2018.11.044The role of fiscal instruments in encouraging the private sector and smallholders to reduce emissions from deforestation and forest degradation: Evidence from Indonesia
Cadman, Tim, Sarker, Tapan, Muttaqin, Zahrul, Nurfatriani, Firti and Maraseni, Tek. 2019. "The role of fiscal instruments in encouraging the private sector and smallholders to reduce emissions from deforestation and forest degradation: Evidence from Indonesia." Forest Policy and Economics. 108, pp. 1-10. https://doi.org/10.1016/j.forpol.2019.04.017Effectiveness of Gravity Goods Ropeways in market participation of smallholder farmers in uplands
KC, Diwakar, Maraseni, Tek, Jamir, Chubamenla, Thapa Magar, Ritendra and Tuladhar, Florencia. 2020. "Effectiveness of Gravity Goods Ropeways in market participation of smallholder farmers in uplands." Transportation. 47 (3), pp. 1393-1414. https://doi.org/10.1007/s11116-018-9970-8Cost analysis of FSC forest certification and opportunities to cover the costs a case study of Quang Tri FSC group in Central Vietnam
Hoang, Hai Thi, Hoshino, Satoshi, Onitsuka, Kenichiro and Maraseni, Tek. 2019. "Cost analysis of FSC forest certification and opportunities to cover the costs a case study of Quang Tri FSC group in Central Vietnam." Journal of Forest Research. 24 (3), pp. 137-142. https://doi.org/10.1080/13416979.2019.1610993Implications of selective harvesting of natural forests for forest product recovery and forest carbon emissions: cases from Tarai Nepal and Queensland Australia
Poudyal, Bishnu Hari, Maraseni, Tek Narayan and Cockfield, Geoff. 2019. "Implications of selective harvesting of natural forests for forest product recovery and forest carbon emissions: cases from Tarai Nepal and Queensland Australia." Forests. 10 (8), pp. 1-19. https://doi.org/10.3390/f10080693Improved prediction of farm nitrous oxide emission through an understanding of the interaction among climate extremes, soil nitrogen dynamics and irrigation water
Maraseni, Tek and Kodur, Shreevatsa. 2019. "Improved prediction of farm nitrous oxide emission through an understanding of the interaction among climate extremes, soil nitrogen dynamics and irrigation water." Journal of Environmental Management. 248, pp. 1-7. https://doi.org/10.1016/j.jenvman.2019.109278Monthly rainfall forecasting with Markov Chain Monte Carlo simulations integrated with statistical bivariate copulas
Ali, Mumtaz, Deo, Ravinesh C., Downs, Nathan J. and Maraseni, Tek. 2020. "Monthly rainfall forecasting with Markov Chain Monte Carlo simulations integrated with statistical bivariate copulas." Samui, Pijush, Bui, Dieu Tien, Chakraborty, Subrata and Deo, Ravinesh C. (ed.) Handbook of probabilistic models. Oxford, United Kingdom. Elsevier. pp. 89-105Development of copula statistical drought prediction model using the Standardized Precipitation-Evapotranspiration Index
Dayal, Kavina S., Deo, Ravinesh C. and Apan, Armando A.. 2020. "Development of copula statistical drought prediction model using the Standardized Precipitation-Evapotranspiration Index." Samui, Pijush, Bui, Dieu Tien, Chakraborty, Subrata and Deo, Ravinesh C. (ed.) Handbook of probabilistic models. Oxford, United Kingdom. Elsevier. pp. 141-178Spatial and temporal variations of embodied carbon emissions in China’s infrastructure
Bai, Jing, Qu, Jiansheng, Maraseni, Tek Narayan, Wu, Jinjia, Xu, Li and Fan, Yujie. 2019. "Spatial and temporal variations of embodied carbon emissions in China’s infrastructure." Sustainability. 11 (3), pp. 1-17. https://doi.org/10.3390/su11030749Rescuing forests from the carbon trap
Ojha, Hemant, Maraseni, Tek, Nightingale, Andrea, Bhattarai, Basundhara and Khatri, Dil. 2019. "Rescuing forests from the carbon trap." Forest Policy and Economics. 101, pp. 15-18. https://doi.org/10.1016/j.forpol.2019.01.007Impacts of forest management on tree species richness and composition: Assessment of forest management regimes in Tarai landscape Nepal
Poudyal, Bishnu Hari, Maraseni, Tek Narayan and Cockfield, Geoff. 2019. "Impacts of forest management on tree species richness and composition: Assessment of forest management regimes in Tarai landscape Nepal." Applied Geography. 111, pp. 1-11. https://doi.org/10.1016/j.apgeog.2019.102078Improving SPI-derived drought forecasts incorporating synoptic-scale climate indices in multi-phase multivariate empirical mode decomposition model hybridized with simulated annealing and kernel ridge regression algorithms
Ali, Mumtaz, Deo, Ravinesh C., Maraseni, Tek and Downs, Nathan J.. 2019. "Improving SPI-derived drought forecasts incorporating synoptic-scale climate indices in multi-phase multivariate empirical mode decomposition model hybridized with simulated annealing and kernel ridge regression algorithms." Journal of Hydrology. 576, pp. 164-184. https://doi.org/10.1016/j.jhydrol.2019.06.032Local users and other stakeholders’ perceptions of the identification and prioritization of ecosystem services in fragile mountains: a case study of Chure Region of Nepal
Acharya, Ram Prasad, Maraseni, Tek Narayan and Cockfield, Geoff. 2019. "Local users and other stakeholders’ perceptions of the identification and prioritization of ecosystem services in fragile mountains: a case study of Chure Region of Nepal." Forests. 10 (5), pp. 1-20. https://doi.org/10.3390/f10050421Meeting national emissions reduction obligations: a case study of Australia
Maraseni, Tek and Reardon-Smith, Kathryn. 2019. "Meeting national emissions reduction obligations: a case study of Australia." Energies. 12 (3), pp. 1-13. https://doi.org/10.3390/en12030438Incorporating forests, agriculture, and energy consumption in the framework of the Environmental Kuznets Curve: a dynamic panel data approach
Parajuli, Rajan, Joshi, Omkar and Maraseni, Tek. 2019. "Incorporating forests, agriculture, and energy consumption in the framework of the Environmental Kuznets Curve: a dynamic panel data approach." Sustainability. 11 (9), pp. 1-11. https://doi.org/10.3390/su11092688The impact of income on household CO2 emissions in China based on a large sample survey
Qu, Jiansheng, Liu, Lina, Zeng, Jingjing, Zhang, Zhiqiang, Wang, Jinping, Pei, Huijuan, Dong, Liping, Liao, Qin and Maraseni, Tek. 2019. "The impact of income on household CO2 emissions in China based on a large sample survey." Science Bulletin. 64 (6), pp. 351-353. https://doi.org/10.1016/j.scib.2019.02.001Environmental and economic impacts and trade-offs from simultaneous management of soil constraints, nitrogen and water
Kodur, Shreevatsa, Shrestha, Uttam Babu, Maraseni, Tek Narayan and Deo, Ravinesh C.. 2019. "Environmental and economic impacts and trade-offs from simultaneous management of soil constraints, nitrogen and water." Journal of Cleaner Production. 222, pp. 960-970. https://doi.org/10.1016/j.jclepro.2019.03.079Weekly soil moisture forecasting with multivariate sequential, ensemble empirical mode decomposition and Boruta-random forest hybridizer algorithm approach
Prasad, Ramendra, Deo, Ravinesh C., Li, Yan and Maraseni, Tek. 2019. "Weekly soil moisture forecasting with multivariate sequential, ensemble empirical mode decomposition and Boruta-random forest hybridizer algorithm approach." Catena. 177, pp. 149-166. https://doi.org/10.1016/j.catena.2019.02.012Remotely sensed agricultural grassland productivity responses to land use and hydro-climatic drivers under extreme drought and rainfall
Kath, Jarrod, Le Brocque, Andrew, Reardon-Smith, Kathryn and Apan, Armando. 2019. "Remotely sensed agricultural grassland productivity responses to land use and hydro-climatic drivers under extreme drought and rainfall." Agricultural and Forest Meteorology. 268, pp. 11-22. https://doi.org/10.1016/j.agrformet.2019.01.007Tree biomass quantity, carbon stock and canopy correlates in mangrove forest and land uses that replaced mangroves in Honda Bay, Philippines
Castillo, Jose Alan A., Apan, Armando A., Maraseni, Tek Narayan and Salmo, Severino G., III. 2018. "Tree biomass quantity, carbon stock and canopy correlates in mangrove forest and land uses that replaced mangroves in Honda Bay, Philippines." Regional Studies in Marine Science. 24, pp. 174-183. https://doi.org/10.1016/j.rsma.2018.08.006Is the finer the better for municipal solid waste (MSW) classification in view of recyclable constituents? A comprehensive social, economic and environmental analysis
Nie, Yongyou, Wu, Yanjing, Zhao, Jinbu, Zhao, Jun, Chen, Xiaojing, Maraseni, Tek and Qian, Guangren. 2018. "Is the finer the better for municipal solid waste (MSW) classification in view of recyclable constituents? A comprehensive social, economic and environmental analysis." Waste Management. 79, pp. 472-480. https://doi.org/10.1016/j.wasman.2018.08.016Transhumance, livestock mobility and mutual benefits between crop and livestock production
Aryal, Suman, Maraseni, Tek, Cockfield, Geoff and de Bruyn, Lisa Lobry. 2018. "Transhumance, livestock mobility and mutual benefits between crop and livestock production." Lichtfouse, Eric (ed.) Sustainable agriculture reviews 31: biocontrol. Switzerland. Springer. pp. 25-39Representing whose access and allocation interests? Stakeholder perceptions and interests representation in climate governance
Cadman, Timothy, Maraseni, Tek, Breakey, Hugh and Ma, Hwan-ok. 2019. "Representing whose access and allocation interests? Stakeholder perceptions and interests representation in climate governance." Edmondson, Beth and Levy, Stuart (ed.) Transformative climates and accountable governance. Cham, Switzerland. Palgrave Macmillan. pp. 223-248Monitoring REDD+ governance: criteria and indicators-based approach to sustainable forest management in Nepal
Cadman, T., Maraseni, T. and Lopez-Casero, F.. 2018. "Monitoring REDD+ governance: criteria and indicators-based approach to sustainable forest management in Nepal." Dhungana, Sindhu, Poudel, Mohan and Bhandari, Trishna Singh (ed.) REDD+ in Nepal: experiences from the REDD Readiness Phase. Kathmandu, Nepal. Government of Nepal, Ministry of Forests and Environment. pp. 101-109Mapping of peanut crops in Queensland, Australia using time-series PROBA-V 100-m normalized difference vegetation index imagery
Haerani, Haerani, Apan, Armando and Basnet, Badri. 2018. "Mapping of peanut crops in Queensland, Australia using time-series PROBA-V 100-m normalized difference vegetation index imagery." Journal of Applied Remote Sensing. 12 (3), pp. 1-22. https://doi.org/10.1117/1.JRS.12.036005Carbon stock and its relationships with tree diversity and density in community forests in Nepal
Aryal, S., Shrestha, S., Maraseni, T., Wagle, P. C and Gaire, N. P.. 2018. "Carbon stock and its relationships with tree diversity and density in community forests in Nepal." The International Forestry Review. 20 (3), pp. 263-273. https://doi.org/10.1505/146554818824063069Cotton yield prediction with Markov Chain Monte Carlo-based simulation model integrated with genetic programing algorithm: a new hybrid copula-driven approach
Ali, Mumtaz, Deo, Ravinesh C., Downs, Nathan J. and Maraseni, Tek. 2018. "Cotton yield prediction with Markov Chain Monte Carlo-based simulation model integrated with genetic programing algorithm: a new hybrid copula-driven approach." Agricultural and Forest Meteorology. 263, pp. 428-448. https://doi.org/10.1016/j.agrformet.2018.09.002Financial returns for different actors in a teak timber value chain in Paklay District, Lao PDR
Maraseni, Tek Narayan, Phimmavong, Somvang, Keenan, Rodney J., Vongkhamsao, Vongvilay, Cockfield, Geoff and Smith, Hilary. 2018. "Financial returns for different actors in a teak timber value chain in Paklay District, Lao PDR." Land Use Policy: the international journal covering all aspects of land use. 75, pp. 145-154. https://doi.org/10.1016/j.landusepol.2018.03.037Multi-stage committee based extreme learning machine model incorporating the influence of climate parameters and seasonality on drought forecasting
Ali, Mumtaz, Deo, Ravinesh C., Downs, Nathan J. and Maraseni, Tek. 2018. "Multi-stage committee based extreme learning machine model incorporating the influence of climate parameters and seasonality on drought forecasting." Computers and Electronics in Agriculture. 152, pp. 149-165. https://doi.org/10.1016/j.compag.2018.07.013Multi-stage hybridized online sequential extreme learning machine integrated with Markov Chain Monte Carlo copula-Bat algorithm for rainfall forecasting
Ali, Mumtaz, Deo, Ravinesh C., Downs, Nathan J. and Maraseni, Tek. 2018. "Multi-stage hybridized online sequential extreme learning machine integrated with Markov Chain Monte Carlo copula-Bat algorithm for rainfall forecasting." Atmospheric Research. 213, pp. 450-464. https://doi.org/10.1016/j.atmosres.2018.07.005Flood risk management under climate change: a hydro-economic perspective
Devkota, Rohini P. and Maraseni, Tek. 2018. "Flood risk management under climate change: a hydro-economic perspective." Water Science and Technology: Water Supply. 18 (5), pp. 1832-1840. https://doi.org/10.2166/ws.2018.003Evolutionary dynamics of selective logging in the tropics: a systematic review of impact studies and their effectiveness in sustainable forest management
Poudyal, Bishnu Hari, Maraseni, Tek and Cockfield, Geoff. 2018. "Evolutionary dynamics of selective logging in the tropics: a systematic review of impact studies and their effectiveness in sustainable forest management." Forest Ecology and Management. 430, pp. 166-175. https://doi.org/10.1016/j.foreco.2018.08.006Selection of representative feature training sets with self-organized maps for optimized time series modeling and prediction: application to forecasting daily drought conditions with ARIMA and neural network models
McCarthy, Elizabeth, Deo, Ravinesh C., Li, Yan and Maraseni, Tek. 2018. "Selection of representative feature training sets with self-organized maps for optimized time series modeling and prediction: application to forecasting daily drought conditions with ARIMA and neural network models." Kim, Dookie, Roy, Sanjiban Sekhar, Lansivaara, Tim, Deo, Ravinesh C. and Samui, Pijush (ed.) Handbook of research on predictive modeling and optimization methods in science and engineering. Hershey, United States. IGI Global. pp. 446-464Soil moisture forecasting by a hybrid machine learning technique: ELM integrated with ensemble empirical mode decomposition
Prasad, Ramendra, Deo, Ravinesh C., Li, Yan and Maraseni, Tek. 2018. "Soil moisture forecasting by a hybrid machine learning technique: ELM integrated with ensemble empirical mode decomposition." Geoderma. 330, pp. 136-161. https://doi.org/10.1016/j.geoderma.2018.05.035Solar, wind and geothermal energy applications in agriculture: back to the future?
Bundschuh, Jochen, Chen, Guangnan, Tomaszewska, Barbara, Ghaffour, Noreddine, Mushtaq, Shahbaz, Hamawand, Ihsan, Reardon-Smith, Kathryn, Maraseni, Tek, Banhazi, Thomas, Mahmoudi, Hacene, Goosen, Mattheus and Antille, Diogones L.. 2017. "Solar, wind and geothermal energy applications in agriculture: back to the future?" Bundschuh, Jochen, Chen, Guangnan, Chandrasekharam, D. and Piechocki, Janusz (ed.) Geothermal, wind and solar energy applications in agriculture and aquaculture. Leiden, Netherlands. CRC Press. pp. 1-32Drought modelling based on artificial intelligence and neural network algorithms: a case study in Queensland, Australia
Dayal, Kavina, Deo, Ravinesh and Apan, Armando A.. 2017. "Drought modelling based on artificial intelligence and neural network algorithms: a case study in Queensland, Australia." Filho, Walter Leal (ed.) Climate change adaptation in Pacific countries:fostering resilience and improving the quality of life. Springer. pp. 177-198Drivers of grassland resistance and recovery to long term drought
Le Brocque, Andrew F, Kath, Jarrod, Reardon-Smith, Kate and Apan, Armando. 2017. "Drivers of grassland resistance and recovery to long term drought." 6th Joint Conference of the Ecological Society of Australia and the New Zealand Ecological Society (EcoTAS 2017). Pokolbin, Australia 26 Nov - 01 Dec 2017Re-imagining standard timescales in forecasting precipitation events for Queensland’s grazing enterprises
McCarthy, E., Deo, R. C., Li, Y. and Maraseni, T.. 2017. "Re-imagining standard timescales in forecasting precipitation events for Queensland’s grazing enterprises." Syme, G., Hatton MacDonald, D., Fulton, B. and Piantadosi, J. (ed.) 22nd International Congress on Modelling and Simulation (MODSIM2017). Hobart, Australia 03 - 08 Dec 2017 Australia. Modelling and Simulation Society of Australia and New Zealand . https://doi.org/10.36334/modsim.2017.L1.mccarthyAn ensemble-ANFIS based uncertainty assessment model for forecasting multi-scalar standardized precipitation index
Ali, Mumtaz, Deo, Ravinesh C., Downs, Nathan J. and Maraseni, Tek. 2018. "An ensemble-ANFIS based uncertainty assessment model for forecasting multi-scalar standardized precipitation index." Atmospheric Research. 207, pp. 155-180. https://doi.org/10.1016/j.atmosres.2018.02.024Ensemble committee-based data intelligent approach for generating soil moisture forecasts with multivariate hydro-meteorological predictors
Prasad, Ramendra, Deo, Ravinesh C., Li, Yan and Maraseni, Tek. 2018. "Ensemble committee-based data intelligent approach for generating soil moisture forecasts with multivariate hydro-meteorological predictors." Soil and Tillage Research. 181, pp. 63-81. https://doi.org/10.1016/j.still.2018.03.021Spatio-temporal drought risk mapping approach and its application in the drought-prone region of south-east Queensland, Australia
Dayal, Kavina S., Deo, Ravinesh C. and Apan, Armando A.. 2018. "Spatio-temporal drought risk mapping approach and its application in the drought-prone region of south-east Queensland, Australia." Natural Hazards. 93 (2), pp. 823-847. https://doi.org/10.1007/s11069-018-3326-8Investigating drought duration-severity-intensity characteristics using the Standardized Precipitation-Evapotranspiration Index: case studies in drought-prone Southeast Queensland
Dayal, Kavina S., Deo, Ravinesh C. and Apan, Armando A.. 2018. "Investigating drought duration-severity-intensity characteristics using the Standardized Precipitation-Evapotranspiration Index: case studies in drought-prone Southeast Queensland." Journal of Hydrologic Engineering. 23 (1), p. 05017029. https://doi.org/10.1061/(ASCE)HE.1943-5584.0001593Multi-layer perceptron hybrid model integrated with the firefly optimizer algorithm for windspeed prediction of target site using a limited set of neighboring reference station data
Deo, Ravinesh C., Ghorbani, Mohammad Ali, Samadianfard, Saeed, Maraseni, Tek, Bilgili, Mehmet and Biazar, Mustafa. 2018. "Multi-layer perceptron hybrid model integrated with the firefly optimizer algorithm for windspeed prediction of target site using a limited set of neighboring reference station data." Renewable Energy. 116 (Part A), pp. 309-323. https://doi.org/10.1016/j.renene.2017.09.078The financial benefits of forest certification: case studies of acacia growers and a furniture company in Central Vietnam
Maraseni, Tek Narayan, Son, Hoang Lien, Cockfield, Geoff, Duy, Hung Vu and Nghia, Tran Dai. 2017. "The financial benefits of forest certification: case studies of acacia growers and a furniture company in Central Vietnam." Land Use Policy: the international journal covering all aspects of land use. 69, pp. 56-63. https://doi.org/10.1016/j.landusepol.2017.09.011Analysing foregone costs of communities and carbon benefits in small scale community based forestry practice in Nepal
Pandey, Shiva Shankar, Maraseni, Tek Narayan, Reardon-Smith, Kathryn and Cockfield, Geoff. 2017. "Analysing foregone costs of communities and carbon benefits in small scale community based forestry practice in Nepal." Land Use Policy: the international journal covering all aspects of land use. 69, pp. 160-166. https://doi.org/10.1016/j.landusepol.2017.09.007Segregated assessment of total carbon stocks by the mode of origin and ecological functions of forests: implication on restoration potential
Neupane, P. R., Gauli, A., Maraseni, T., Kubler, D., Mundhenk, P. and Dang, M. V.. 2017. "Segregated assessment of total carbon stocks by the mode of origin and ecological functions of forests: implication on restoration potential." The International Forestry Review. 19 (S4), pp. 1-28. https://doi.org/10.1505/146554817822330579An international comparison of rice consumption behaviours and greenhouse gas emissions from rice production
Maraseni, Tek Narayan, Deo, Ravinesh C., Qu, Jiansheng, Gentle, Popular and Neupane, Prem Raj. 2018. "An international comparison of rice consumption behaviours and greenhouse gas emissions from rice production." Journal of Cleaner Production. 172, pp. 2288-2300. https://doi.org/10.1016/j.jclepro.2017.11.182Comparing the financial returns from acacia plantations with different plantation densities and rotation ages in Vietnam
Maraseni, Tek Narayan, Son, Hoang Lien, Cockfield, Geoff, Duy, Hung Vu and Nghia, Tran Dai. 2017. "Comparing the financial returns from acacia plantations with different plantation densities and rotation ages in Vietnam." Forest Policy and Economics. 83, pp. 80-87. https://doi.org/10.1016/j.forpol.2017.06.010Input selection and performance optimization of ANN-based streamflow forecasts in the drought-prone Murray Darling Basin region using IIS and MODWT algorithm
Prasad, Ramendra, Deo, Ravinesh C., Li, Yan and Maraseni, Tek. 2017. "Input selection and performance optimization of ANN-based streamflow forecasts in the drought-prone Murray Darling Basin region using IIS and MODWT algorithm." Atmospheric Research. 197, pp. 42-63. https://doi.org/10.1016/j.atmosres.2017.06.014Soil C quantities of mangrove forests, their competing land uses, and their spatial distribution in the coast of Honda Bay, Philippines
Castillo, Jose Alan A., Apan, Armando A., Maraseni, Tek Narayan and Salmo, Severino G., III. 2017. "Soil C quantities of mangrove forests, their competing land uses, and their spatial distribution in the coast of Honda Bay, Philippines." Geoderma. 293, pp. 82-90. https://doi.org/10.1016/j.geoderma.2017.01.025The rate, extent and spatial predictors of forest loss (2000-2012) in the terrestrial protected areas of the Philippines
Apan, Armando, Suarez, Luz Angelica, Maraseni, Tek and Castillo, Jose Alan. 2017. "The rate, extent and spatial predictors of forest loss (2000-2012) in the terrestrial protected areas of the Philippines." Applied Geography. 81, pp. 32-42. https://doi.org/10.1016/j.apgeog.2017.02.007Sweet success: virtual world tools enhance real world decision making in the Australian sugar industry
Reardon-Smith, Kate, Farley, Helen, Cliffe, Neil, Mushtaq, Shahbaz, Stone, Roger, Doyle, Joanne, Martin, Neil, Ostini, Jenny, Maraseni, Tek, Marcussen, Torben, Loch, Adam and Lindesay, Janette. 2014. "Sweet success: virtual world tools enhance real world decision making in the Australian sugar industry." ICELW 2014: International Conference on e-Learning in the Workplace. New York, United States 11 - 13 Jun 2014Household and community responses to impacts of climate change in the rural hills of Nepal
Gentle, Popular, Thwaites, Rik, Race, Digby, Alexander, Kim and Maraseni, Tek. 2018. "Household and community responses to impacts of climate change in the rural hills of Nepal." Climatic Change: an interdisciplinary, international journal devoted to the description, causes and implications of climatic change. 147 (1-2), pp. 267-282. https://doi.org/10.1007/s10584-017-2124-8Savanna burning methodology for fire management and emissions reduction: a critical review of influencing factors
Maraseni, Tek Narayan, Reardon‑Smith, Kathryn, Griffiths, Greg and Apan, Armando. 2016. "Savanna burning methodology for fire management and emissions reduction: a critical review of influencing factors." Carbon Balance and Management. 11, pp. 1-11. https://doi.org/10.1186/s13021-016-0067-4Comparative evaluation of zero deforestation governance
Cadman, Tim, Maraseni, Tek, Sarker, Tapan and Ma, Hwan Ok. 2017. "Comparative evaluation of zero deforestation governance." EFTRN News. 58, pp. 161-168.Soil greenhouse gas fluxes in tropical mangrove forests and in land uses on deforested mangrove lands
Castillo, Jose Alan A., Apan, Armando A., Maraseni, Tek N. and Salmo, Severino G., III. 2017. "Soil greenhouse gas fluxes in tropical mangrove forests and in land uses on deforested mangrove lands." Catena. 159, pp. 60-69. https://doi.org/10.1016/j.catena.2017.08.005Estimation and mapping of above-ground biomass of mangrove forests and their replacement land uses in the Philippines using Sentinel imagery
Castillo, Jose Alan A., Apan, Armando A., Maraseni, Tek N. and Salmo, Severino G., III. 2017. "Estimation and mapping of above-ground biomass of mangrove forests and their replacement land uses in the Philippines using Sentinel imagery." ISPRS Journal of Photogrammetry and Remote Sensing. 134, pp. 70-85. https://doi.org/10.1016/j.isprsjprs.2017.10.016Opportunities of adopting renewable energy for the nursery industry in Australia
Chen, Guangnan, Schmidt, Erik, Maraseni, Tek, Bundschuh, Jochen, Banhazi, Thomas and Antille, Diogones L.. 2017. "Opportunities of adopting renewable energy for the nursery industry in Australia." Bundschuh, Jochen, Chen, Guangnan, Chandrasekharam, D. and Piechocki, Janusz (ed.) Geothermal, wind and solar energy applications in agriculture and aquaculture. Leiden, Netherlands. CRC Press. pp. 115-126Hyperspectral sensing to detect the impact of herbicide drift on cotton growth and yield
Suarez, L. A., Apan, Armando and Werth, Jeff. 2016. "Hyperspectral sensing to detect the impact of herbicide drift on cotton growth and yield." ISPRS Journal of Photogrammetry and Remote Sensing. 120, pp. 65-76. https://doi.org/10.1016/j.isprsjprs.2016.08.004Dynamism of household carbon emissions (HCEs) from rural and urban regions of northern and southern China
Maraseni, Tek Narayan, Qu, Jiansheng, Yue, Bian, Zeng, Jingjing and Maroulis, Jerry. 2016. "Dynamism of household carbon emissions (HCEs) from rural and urban regions of northern and southern China." Environmental Science and Pollution Research. 23 (20), pp. 20553-20566. https://doi.org/10.1007/s11356-016-7237-5An assessment of carbon sequestration potential of riparian zone of Condamine Catchment, Queensland, Australia
Maraseni, T. N. and Mitchell, C.. 2016. "An assessment of carbon sequestration potential of riparian zone of Condamine Catchment, Queensland, Australia." Land Use Policy: the international journal covering all aspects of land use. 54, pp. 139-146. https://doi.org/10.1016/j.landusepol.2016.02.013Do you think it is time to consider legume-based cropping systems again?
Maraseni, Tek. 2014. "Do you think it is time to consider legume-based cropping systems again? " Journal of Pollution Effects and Control. 2 (1), pp. 1-2. https://doi.org/10.4172/2375-4397.1000e109Groundwater decline and climatic variability: Implications for groundwater linked agro-ecosystems
Le Brocque, Andrew F., Kath, Jarrod, Reardon-Smith, Kate and Apan, Armando. 2016. "Groundwater decline and climatic variability: Implications for groundwater linked agro-ecosystems." 2016 Conference of the Ecological Society of Australia (ESA 2016). Fremantle, Australia 29 Nov - 02 Dec 2016 Australia.An international comparison of agricultural nitrous oxide emissions
Maraseni, Tek Narayan and Qu, Jiansheng. 2016. "An international comparison of agricultural nitrous oxide emissions." Journal of Cleaner Production. 135, pp. 1256-1266. https://doi.org/10.1016/j.jclepro.2016.07.035Vulnerability assessment of urban community and critical infrastructures for integrated flood risk management and climate adaptation strategies
Espada, Rudolf, Apan, Armando and McDougall, Kevin. 2017. "Vulnerability assessment of urban community and critical infrastructures for integrated flood risk management and climate adaptation strategies." International Journal of Disaster Resilience in the Built Environment. 8 (4), pp. 375-411. https://doi.org/10.1108/IJDRBE-03-2015-0010Sustainability of transhumance grazing systems under socio-economic threats in Langtang, Nepal
Aryal, Suman, Maraseni, Tek Narayan and Cockfield, Geoff. 2014. "Sustainability of transhumance grazing systems under socio-economic threats in Langtang, Nepal." Journal of Mountain Science. 11 (4), pp. 1023-1034. https://doi.org/10.1007/s11629-013-2684-7Spatial variations and determinants of per capita household CO2 emissions (PHCEs) in China
Liu, Lina, Qu, Jiansheng, Clarke-Sather, Afton, Maraseni, Tek Narayan and Pang, Jiaxing. 2017. "Spatial variations and determinants of per capita household CO2 emissions (PHCEs) in China." Sustainability. 9 (7). https://doi.org/10.3390/su9071277Business, organised labour and climate policy: forging a role at the negotiation table
Glynn, Peter J., Cadman, Tomothy and Maraseni, Tek Narayan. 2017. Business, organised labour and climate policy: forging a role at the negotiation table. Cheltenham, United Kingdom. Edward Elgar Publishing.Viability of weather index insurance in managing drought risk in Australia
Adeyinka, A. A., Krishnamurti, C., Maraseni, T. and Chantarat, S.. 2013. "Viability of weather index insurance in managing drought risk in Australia." Actuaries Summit 2013. Sydney, Australia 20 - 21 May 2013Application of hybrid artificial neural network algorithm for the prediction of Standardized Precipitation Index
Dayal, Kavina S., Deo, Ravinesh C. and Apan, Armando A.. 2016. "Application of hybrid artificial neural network algorithm for the prediction of Standardized Precipitation Index." 2016 IEEE Region 10 International Conference: Technologies for Smart Nation (TENCON 2016). Singapore 22 - 25 Nov 2016 Singapore. https://doi.org/10.1109/TENCON.2016.7848588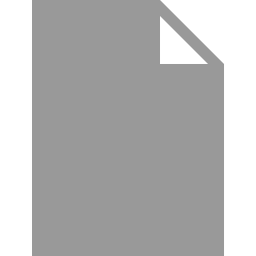
Application of Modis data to assess the latest forest cover changes of Sri Lanka
Perera, Kithsiri, Herath, Srikantha, Apan, Armando and Tateishi, Ryutaro. 2013. "Application of Modis data to assess the latest forest cover changes of Sri Lanka." Ogleby, Cliff (ed.) 22nd Annual Photogrammetry, Remote Sensing and Spatial Information Science Congress (ISPRS 2012): Imaging a Sustainable Future. Melbourne, Australia 25 Aug - 01 Sep 2012 Australia. Copernicus GmbH. https://doi.org/10.5194/isprsannals-I-7-165-2012Perceived changes in climatic variables and impacts on the transhumance system in the Himalayas
Aryal, Suman, Cockfield, Geoff and Maraseni, Tek Narayan. 2016. "Perceived changes in climatic variables and impacts on the transhumance system in the Himalayas." Climate and Development. 8 (5), pp. 435-446. https://doi.org/10.1080/17565529.2015.1040718Detection of phenoxy herbicide dosage in cotton crops through the analysis of hyperspectral data
Suarez, L. A., Apan, Armando and Werth, J.. 2017. "Detection of phenoxy herbicide dosage in cotton crops through the analysis of hyperspectral data." International Journal of Remote Sensing. 38 (23), pp. 6528-6553. https://doi.org/10.1080/01431161.2017.1362128Delineation of management zones using multiple crop yield data
Basnet, Badri, Kelly, Rob, Jensen, Troy, Strong, Wayne, Apan, Armando and Butler, David. 2003. "Delineation of management zones using multiple crop yield data." 16th Triennial Congress of the International Soil Tillage Research Organisation (ISTRO 2003). Brisbane, Australia 13 - 18 Jul 2003 Brisbane, Australia.Correlating high resolution aerial imagery with wheat yield and protein content
Apan, A., Kelly, R., Jensen, T., Strong, W. and Butler, D.. 2004. "Correlating high resolution aerial imagery with wheat yield and protein content." Smith, Richard and Dawbin, Ken (ed.) 12th Australasian Remote Sensing and Photogrammetry Conference (ARSPC 2004): To Measure is to Manage. Fremantle, Australia 18 - 22 Oct 2004 Adelaide, Australia.Spectral discrimination and classification of sugarcane varieties using EO-1 hyperion hyperspectral imagery
Apan, Armando, Held, Alex, Phinn, Stuart and Markley, John. 2004. "Spectral discrimination and classification of sugarcane varieties using EO-1 hyperion hyperspectral imagery." 25th Asian Conference on Remote Sensing (ACRS 2004). Chiang Mai, Thailand 22 - 26 Nov 2004 China.Application of aerial imagery to analyse on-farm trials
Basnet, B., Kelly, R., Jensen, T., Kane, T., Apan, A. and Butler, D.. 2002. "Application of aerial imagery to analyse on-farm trials." 11th Australasian Remote Sensing and Photogrammetry Conference (ARSPC2002): Images to Information. Brisbane, Australia 02 - 06 Sep 2002 Australia.Capturing of remotely sensed images to advance the use of yield maps and other spatial information to improve crop management and forecasting
Jensen, T., Apan, A., Young, F. and Zeller, L.. 2002. "Capturing of remotely sensed images to advance the use of yield maps and other spatial information to improve crop management and forecasting." 11th Australasian Remote Sensing and Photogrammetry Conference (ARSPC2002): Images to Information. Brisbane, Australia 02 - 06 Sep 2002 Adelaide, Australia.Spectral discrimination and separability analysis of agricultural crops and soil attributes using ASTER imagery
Apan, Armando, Kelly, Rob, Jensen, Troy, Butler, David, Strong, Wayne and Basnet, Badri. 2002. "Spectral discrimination and separability analysis of agricultural crops and soil attributes using ASTER imagery." 11th Australasian Remote Sensing and Photogrammetry Conference (ARSPC2002): Images to Information. Brisbane, Australia 02 - 06 Sep 2002 Adelaide, Australia.Quantifying landscape fragmentation in the Lockyer Valley Catchment, Queensland: 1973 – 1997
Apan, Armando A., Raine, Steven R. and Paterson, Mark S.. 2000. "Quantifying landscape fragmentation in the Lockyer Valley Catchment, Queensland: 1973 – 1997." AURISA 2000 Conference (AURISA 2000). Coolum, Australia 20 - 24 Nov 2000Governance values in the climate change regime: stakeholder perceptions of REDD+ legitimacy at the national level
Cadman, Timothy, Maraseni, Tek, Breakey, Hugh, Lopez-Casero, Federoico and Ma, Hwan Ok. 2016. "Governance values in the climate change regime: stakeholder perceptions of REDD+ legitimacy at the national level." Forests. 7 (10), pp. 212-228. https://doi.org/10.3390/f7100212Image analysis techniques for assessing landscape structural change: a case study of the Lockyer Valley Catchment, Queensland
Apan, Armando A., Raine, Steven R. and Paterson, Mark S.. 2000. "Image analysis techniques for assessing landscape structural change: a case study of the Lockyer Valley Catchment, Queensland." 10th Australasian Remote Sensing and Photogrammetry Conference (ARSPC 2000). Adelaide, Australia 21 - 25 Aug 2000Five years of REDD+ governance: the use of market mechanisms as a response to anthropogenic climate change
Cadman, Timothy, Maraseni, Tek, Ok Ma, Hwan and Lopez-Casero, Federico. 2017. "Five years of REDD+ governance: the use of market mechanisms as a response to anthropogenic climate change." Forest Policy and Economics. 79, pp. 8-16. https://doi.org/10.1016/j.forpol.2016.03.008An assessment of direct on-farm energy use for high value grain crops grown under different farming practices in Australia
Maraseni, Tek, Chen, Guangnan, Banhazi, Thomas, Bundschuh, Jochen and Yusaf, Talal. 2015. "An assessment of direct on-farm energy use for high value grain crops grown under different farming practices in Australia." Energies. 8 (11), pp. 13033-13046. https://doi.org/10.3390/en81112353Predicting the distributions of predator (snow leopard) and prey (blue sheep) under climate change in the Himalaya
Aryal, Achyut, Shrestha, Uttam Babu, Ji, Weihong, Ale, Som B., Shrestha, Sujata, Ingty, Tenzing, Maraseni, Tek, Cockfield, Geoff and Raubenheimer, David. 2016. "Predicting the distributions of predator (snow leopard) and prey (blue sheep) under climate change in the Himalaya." Ecology and Evolution. 6 (12), pp. 1-11. https://doi.org/10.1002/ece3.2196An analysis of magnitudes and trends of household carbon emissions in China between 1995 and 2011
Maraseni, T. N., Qu, J., Zeng, J. and Liu, L.. 2016. "An analysis of magnitudes and trends of household carbon emissions in China between 1995 and 2011." International Journal of Environmental Research. 10 (1), pp. 179-192.Trade-offs and synergies between water and energy use in rural Australia
Mushtaq, Shahbaz, Maraseni, Tek N. and Reardon-Smith, Kathryn. 2015. "Trade-offs and synergies between water and energy use in rural Australia." Pittock, Jamie, Hussey, Karen and Dovers, Stephen (ed.) Climate, energy and water: managing trade-offs, seizing opportunities. New York, USA. Cambridge University Press. pp. 123-140Developing sustainable governance systems at the regional level: the case of emissions trading
Cadman, Tim, Hume, Margee, Maraseni, Tek and Lopez-Casero, Federico. 2015. "Developing sustainable governance systems at the regional level: the case of emissions trading." Thomas, Ken D. (ed.) Handbook of research on sustainable development and economics. United States. IGI Global. pp. 248-266Agriculture: alternative energy sources
Chen, Guangnan, Maraseni, Tek, Bundschuh, Jochen and Zare, Dariush. 2015. "Agriculture: alternative energy sources." Anwar, Sohail (ed.) Encyclopedia of energy engineering and technology. United States. Taylor & Francis. pp. 1-6On-farm energy use in the grain and horticultural industries
Chen, Guangnan, Maraseni, Tek, Banhazi, Thomas and Bundschuh, Jochen. 2015. "On-farm energy use in the grain and horticultural industries." 1st Australian Life Cycle Assessment Conference 2015 (AgriFoodLCA): Growth Through Sustainability. Melbourne, Australia 23 - 24 Nov 2015 Australia.On-farm energy use in the dairy industries
Chen, Guangnan, Maraseni, Tek, Banhazi, Thomas and Bundschuh, Jochen. 2015. "On-farm energy use in the dairy industries." 1st Australian Life Cycle Assessment Conference 2015 (AgriFoodLCA): Growth Through Sustainability. Melbourne, Australia 23 - 24 Nov 2015 Australia.Deriving an index of adoption rate and assessing factors affecting adoption of an agroforestry-based farming system in Dhanusha District, Nepal
Dhakal, Arun, Cockfield, Geoff and Maraseni, Tek Narayan. 2015. "Deriving an index of adoption rate and assessing factors affecting adoption of an agroforestry-based farming system in Dhanusha District, Nepal." Agroforestry Systems. 89 (4), pp. 645-661. https://doi.org/10.1007/s10457-015-9802-1The Political economy of sustainable development: policy instruments and market mechanisms
Cadman, Tim, Eastwood, Lauren, Lopez-Casero Michaelis, Federico, Maraseni, Tek, Pittock, Jamie and Sarker, Tapan. 2015. The Political economy of sustainable development: policy instruments and market mechanisms. Cheltenham, Northampton,United Kingdom. Edward Elgar Publishing.A comparative analysis of global stakeholders’ perceptions of the governance quality of the clean development mechanism (CDM) and reducing emissions from deforestation and forest degradation (REDD+)
Maraseni, Tek Narayan and Cadman, Tim. 2015. "A comparative analysis of global stakeholders’ perceptions of the governance quality of the clean development mechanism (CDM) and reducing emissions from deforestation and forest degradation (REDD+)." International Journal of Environmental Studies. 72 (2), pp. 288-304. https://doi.org/10.1080/00207233.2014.993569The financial implications of converting farmland to state-supported environmental plantings in the Darling Downs region, Queensland
Maraseni, Tek Narayan and Cockfield, Geoff. 2015. "The financial implications of converting farmland to state-supported environmental plantings in the Darling Downs region, Queensland." Agricultural Systems. 135, pp. 57-65. https://doi.org/10.1016/j.agsy.2014.12.004Vulnerability assessment and interdependency analysis of critical infrastructures for climate adaptation and flood mitigation
Espada Jr., Rodolfo, Apan, Armando and McDougall, Kevin. 2015. "Vulnerability assessment and interdependency analysis of critical infrastructures for climate adaptation and flood mitigation." International Journal of Disaster Resilience in the Built Environment. 6 (3), pp. 313-346. https://doi.org/10.1108/IJDRBE-02-2014-0019Adapting to climate variability: the views of peasant farmers in Nepal
Chalise, Sudarshan, Maraseni, Tek Narayan and Maroulis, Jerry. 2015. "Adapting to climate variability: the views of peasant farmers in Nepal." International Journal of Global Warming. 7 (3), pp. 380-394. https://doi.org/10.1504/IJGW.2015.069369Assessing the roles of community forestry in climate change mitigation and adaptation: a case study from Nepal
Pandey, Shiva Shankar, Cockfield, Geoff and Maraseni, Tek Narayan. 2016. "Assessing the roles of community forestry in climate change mitigation and adaptation: a case study from Nepal." Forest Ecology and Management. 360, pp. 400-407. https://doi.org/10.1016/j.foreco.2015.09.040A comparison of household carbon emission patterns of urban and rural China over the 17 year period (1995–2011)
Qu, Jiansheng, Maraseni, Tek, Liu, Lina, Zhang, Zhiqiang and Yusaf, Talal. 2015. "A comparison of household carbon emission patterns of urban and rural China over the 17 year period (1995–2011)." Energies. 8 (9), pp. 10537-10557. https://doi.org/10.3390/en80910537A comparison of trends and magnitudes of household carbon emissions between China, Canada and UK
Maraseni, Tek Narayan, Qu, Jiansheng and Zeng, Jingjing. 2015. "A comparison of trends and magnitudes of household carbon emissions between China, Canada and UK." Environmental Development. 15, pp. 103-119. https://doi.org/10.1016/j.envdev.2015.04.001Nonlinear Optimisation Using Production Functions to Estimate Economic Benefit of Conjunctive Water Use for Multicrop Production
An-Vo, D.-A., Mushtaq, S., Nguyen-Ky, T., Bundschuh, J., Tran-Cong, T., Maraseni, T. N. and Reardon-Smith, K.. 2015. "Nonlinear Optimisation Using Production Functions to Estimate Economic Benefit of Conjunctive Water Use for Multicrop Production." Water Resources Management. 29 (7), pp. 2153-2170. https://doi.org/10.1007/s11269-015-0933-yVirtual discussions to support climate risk decision making on farms
Reardon-Smith, Kate, Mushtaq, Shahbaz, Farley, Helen S., Cliffe, Neil, Stone, Roger C., Ostini, Jenny, Doyle, Joanne, Martin, Neil, Loch, Adam, Maraseni, Tek, Marcussen, Torben and Lindesay, Janette. 2015. "Virtual discussions to support climate risk decision making on farms." Journal of Economic and Social Policy. 17 (2).Effect of summer livestock grazing on plant species richness and composition in the Himalayan rangelands
Aryal, Suman, Cockfield, Geoff and Maraseni, Tek. 2015. "Effect of summer livestock grazing on plant species richness and composition in the Himalayan rangelands." The Rangeland Journal. 37 (3), pp. 309-321. https://doi.org/10.1071/RJ14088Developing forest-sector and REDD+ governance: a multi-stage, multi-level and multi-stakeholder approach in Nepal
Lopez-Casero, Federico, Cadman, Tim and Maraseni, Tek. 2014. "Developing forest-sector and REDD+ governance: a multi-stage, multi-level and multi-stakeholder approach in Nepal." Policy in Focus.Prioritising carbon sequestration areas in southern Queensland using time series MODIS net primary productivity (NPP) imagery
Apan, A., Suarez Cadavid, L. A., Richardson, L. and Maraseni, T.. 2014. "Prioritising carbon sequestration areas in southern Queensland using time series MODIS net primary productivity (NPP) imagery." Dadhwal, V. K., Seshasai, M. V. R., Hakeem, A., Diwakar, P. G. and Raju, P. L. N. (ed.) Technical Commission 8th Mid-Term Symposium (ISPRS 2014). Hyderabad, India 09 - 12 Dec 2014 Goettingen, Germany. https://doi.org/10.5194/isprsarchives-XL-8-549-2014Virtual world technologies to enhance climate risk management on Australian sugar cane farms
Reardon-Smith, Kate, Mushtaq, Shahbaz, Stone, Roger, Cliffe, Neil, Farley, Helen, Ostini, Jenny, Doyle, Joanne, Martin, Neil, Maraseni, Tek, Marcussen, Torben, Loch, Adam and Lindesay, Janette. 2014. "Virtual world technologies to enhance climate risk management on Australian sugar cane farms." Climate Adaptation (NCCARF 2014): Future Challenges. Gold Coast, Australia 30 Sep - 02 Oct 2014 Gold Coast, Australia.Integrated assessment of water-energy-GHG emissions tradeoffs in an irrigated lucerne production system in eastern Australia
Mushtaq, S., Maraseni, T. N., Reardon-Smith, K., Bundschuh, J. and Jackson, T.. 2015. "Integrated assessment of water-energy-GHG emissions tradeoffs in an irrigated lucerne production system in eastern Australia." Journal of Cleaner Production. 103, pp. 491-498. https://doi.org/10.1016/j.jclepro.2014.05.037Spectral discrimination of bulloak (Allocasuarina luehmannii) and associated woodland for habitat mapping of the endangered bulloak jewel butterfly (Hypochrysops piceata) in southern Queensland
Zainol Abdullah, Wan Nor Zanariah, Apan, Armando A., Maraseni, Tek N. and Le Brocque, Andrew F.. 2014. "Spectral discrimination of bulloak (Allocasuarina luehmannii) and associated woodland for habitat mapping of the endangered bulloak jewel butterfly (Hypochrysops piceata) in southern Queensland." Journal of Applied Remote Sensing. 8 (1), pp. 083561-1. https://doi.org/10.1117/1.JRS.8.083561Spatial modelling of natural disaster risk reduction policies with Markov decision processes
Espada Jr., Rodolfo, Apan, Armando and McDougall, Kevin. 2014. "Spatial modelling of natural disaster risk reduction policies with Markov decision processes." Applied Geography. 53, pp. 284-298. https://doi.org/10.1016/j.apgeog.2014.06.021Re-evaluating the rationale for irrigation technology adoption through an integrated trade-off analysis: case study of a cotton farming system in Australia
Maraseni, T. N., Mushtaq, S. and Reardon-Smith, K.. 2014. "Re-evaluating the rationale for irrigation technology adoption through an integrated trade-off analysis: case study of a cotton farming system in Australia." Journal of Water and Climate Change. 5 (3), pp. 328-340. https://doi.org/10.2166/wcc.2014.046Carbon stock dynamics in different vegetation dominated community forests under REDD+: a case from Nepal
Pandey, Shiva Shankar, Maraseni, Tek Narayan and Cockfield, Geoff. 2014. "Carbon stock dynamics in different vegetation dominated community forests under REDD+: a case from Nepal." Forest Ecology and Management. 327, pp. 40-47. https://doi.org/10.1016/j.foreco.2014.04.028Tree species diversity in community managed and national park forests in the mid-hills of central Nepal
Pandey, Shiva Shankar, Maraseni, Tek Narayan, Cockfield, Geoff and Gerhard, Karin. 2014. "Tree species diversity in community managed and national park forests in the mid-hills of central Nepal." Journal of Sustainable Forestry. 33 (8), pp. 796-813. https://doi.org/10.1080/10549811.2014.925811An assessment of the impacts of the REDD+ pilot project on community forests user groups (CFUGs) and their community forests in Nepal
Maraseni, T. N., Neupane, P. R., Lopez-Casero, F. and Cadman, T.. 2014. "An assessment of the impacts of the REDD+ pilot project on community forests user groups (CFUGs) and their community forests in Nepal." Journal of Environmental Management. 136, pp. 37-46. https://doi.org/10.1016/j.jenvman.2014.01.011The impact of varying statutory arrangements on spatial data sharing and access in regional NRM bodies
Paudyal, D. R., McDougall, K. and Apan, A.. 2014. "The impact of varying statutory arrangements on spatial data sharing and access in regional NRM bodies." ISPRS Annals of the Photogrammetry, Remote Sensing and Spatial Information Sciences. II (8), pp. 193-197. https://doi.org/10.5194/isprsannals-II-8-193-2014An assessment of willingness to pay to avoid climate change induced flood
Devkota, Rohini Prasad, Maraseni, Tek Narayan and Cockfield, Geoff. 2014. "An assessment of willingness to pay to avoid climate change induced flood." Journal of Water and Climate Change. 5 (4), pp. 569-577. https://doi.org/10.2166/wcc.2014.134The development of virtual world tools to enhance learning and real world decision making in the Australian sugar farming industry
Reardon-Smith, Kathryn, Farley, Helen, Cliffe, Neil, Mushtaq, Shahbaz, Stone, Roger, Doyle, Joanne, Martin, Neil, Ostini, Jenny, Maraseni, Tek, Marcussen, Torben, Loch, Adam and Lindesay, Janette. 2014. "The development of virtual world tools to enhance learning and real world decision making in the Australian sugar farming industry." International Journal of Advanced Corporate Learning. 7 (3), pp. 17-23. https://doi.org/10.3991/ijac.v7i3.4002Climate change and indigenous people: perceptions of transhumant herders and implications to the transhumance system in the Himalayas
Aryal, Suman, Maraseni, Tek N. and Cockfield, Geoff. 2014. "Climate change and indigenous people: perceptions of transhumant herders and implications to the transhumance system in the Himalayas." Geology and Geosciences. 3 (4), pp. 1-5. https://doi.org/10.4172/2329-6755.1000162Can vegetation types work as an indicator of soil organic carbon? An insight from native vegetations in Nepal
Maraseni, T. N. and Pandey, S. S.. 2014. "Can vegetation types work as an indicator of soil organic carbon? An insight from native vegetations in Nepal." Ecological Indicators. 46, pp. 315-322. https://doi.org/10.1016/j.ecolind.2014.06.038Vulnerability of Himalayan transhumant communities to climate change
Aryal, Suman, Cockfield, Geoff and Maraseni, Tek Narayan. 2014. "Vulnerability of Himalayan transhumant communities to climate change." Climatic Change: an interdisciplinary, international journal devoted to the description, causes and implications of climatic change. 125 (2), pp. 193-208. https://doi.org/10.1007/s10584-014-1157-5Addressing the participation gap in institutional investment: an assessment framework and preliminary results
Cadman, Tim and Maraseni, Tek. 2014. "Addressing the participation gap in institutional investment: an assessment framework and preliminary results." Hawley, James P., Hoepner, Andreas G. F., Johnson, Keith L., Sandberg, Joakim and Waitzer, Edward W. (ed.) Cambridge handbook of institutional investment and fiduciary duty. Cambridge, United Kingdom. Cambridge University Press. pp. 452-465Using a virtual world to enable real world decision making
Reardon-Smith, Kate, Farley, Helen, Stone, Roger, Mushtaq, Shahbaz, Cliffe, Neil, Doyle, Joanne, Martin, Neil, Maraseni, Tek, Marcussen, Torben, Lindesay, Janette, Loch, Adam and Kealley, Matt. 2013. "Using a virtual world to enable real world decision making." Lamb, David (ed.) 1st Digital Rural Futures Conference: Smart Farms - Smart Regions (DRF 2013). Armidale, Australia 26 - 28 Jun 2013 Armidale, Australia.Effects of spatial resolution on measurement of landscape function using the landscape leakiness calculator
Dunwoody, Ernest, Apan, Armando and Liu, Xiaoye. 2013. "Effects of spatial resolution on measurement of landscape function using the landscape leakiness calculator." Surveying and Spatial Sciences Conference (SSSC 2013): Collect, Connect, Capitalise. Canberra, Australia 17 - 19 Apr 2013 Canberra, Australia.Developing spatial information sharing strategies across natural resource management communities
Paudyal, Dev Raj, McDougall, Kevin and Apan, Armando. 2013. "Developing spatial information sharing strategies across natural resource management communities." Onsrud, Harlan and Rajabifard, Abbas (ed.) Spatial Enablement in Support of Economic Development and Poverty Reduction: Research, Development and Education Perspectives. Needham, MA, USA. GSDI Association Press. pp. 141-168Using spatial modelling to develop flood risk and climate adaptation capacity metrics for vulnerability assessments of urban community and critical water supply infrastructure
Espada Jr., Rodolfo, Apan, Armando and McDougall, Kevin. 2013. "Using spatial modelling to develop flood risk and climate adaptation capacity metrics for vulnerability assessments of urban community and critical water supply infrastructure." Featherstone, Jeffrey (ed.) 49th World Congress of the International Society of City and Regional Planners (ISOCARP 2013): Frontiers of Planning: Evolving and Declining Models of City Planning Practice. Brisbane, Australia 01 - 04 Oct 2013 Netherlands.Dynamics of carbon and biodiversity under REDD+ regime: a case from Nepal
Pandey, Shiva Shankar, Cockfield, Geoff and Maraseni, Tek Narayan. 2014. "Dynamics of carbon and biodiversity under REDD+ regime: a case from Nepal." Environmental Science and Policy. 38, pp. 272-281. https://doi.org/10.1016/j.envsci.2014.01.005Adaptation and resilience in two flood-prone Queensland communities
King, David, Apan, Armando, Keogh, Diane and Thomas, Melanie. 2013. "Adaptation and resilience in two flood-prone Queensland communities." Boulter, Sarah, Palutikof, Jean, Karoly, David John and Guitart, Daniella (ed.) Natural disasters and adaptation to climate change. New York, NY. United States. Cambridge University Press. pp. 95-106Evaluating the Clean Development Mechanism
Maraseni, Tek Narayan. 2013. "Evaluating the Clean Development Mechanism ." Cadman, Timothy (ed.) Climate Change and Global Policy Regimes:Towards Institutional Legitimacy. United Kingdom. Palgrave Macmillan. pp. 96-110Using spatial modelling to develop flood risk and climate adaptation capacity metrics for assessing urban community and critical electricity infrastructure vulnerability
Espada Jr., R., Apan, A. and McDougall, K.. 2013. "Using spatial modelling to develop flood risk and climate adaptation capacity metrics for assessing urban community and critical electricity infrastructure vulnerability." Piantadosi, J., Anderssen, R. S. and Boland, J. (ed.) 20th International Congress on Modelling and Simulation (MODSIM2013). Adelaide, Australia 01 - 06 Dec 2013 Australia. Modelling and Simulation Society of Australia and New Zealand . https://doi.org/10.36334/modsim.2013.L1.espadaPayments to landholders for managing water, land and ecosystems (WLE) in coastal agricultural catchments for protecting the Great Barrier Reef
Maraseni, Tek N. and Hanjra, Munir A.. 2014. "Payments to landholders for managing water, land and ecosystems (WLE) in coastal agricultural catchments for protecting the Great Barrier Reef." Mohammed, Essam Yassin (ed.) Economic incentives for marine and coastal conservation: prospects, challenges and policy implications. Abingdon, Oxon. United Kingdom. Routledge. pp. 190-209Major drivers of deforestation and forest degradation in developing countries and REDD+
Pandey, Shiva S., Cockfield, Geoff and Maraseni, Tek Narayan. 2013. "Major drivers of deforestation and forest degradation in developing countries and REDD+." International Journal of Forest Usufructs Management. 14 (1), pp. 99-107.Quality-of-governance standards for carbon emissions trading: developing REDD+ governance through a multi-stage, multi-level and multi-stakeholder approach
Lopez-Casero, Federico, Cadman, Tim and Maraseni, Tek. 2013. Quality-of-governance standards for carbon emissions trading: developing REDD+ governance through a multi-stage, multi-level and multi-stakeholder approach. Hayama, Japan. Institute for Global Environmental Strategies (IGES).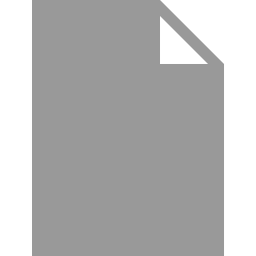