Drought modelling based on artificial intelligence and neural network algorithms: a case study in Queensland, Australia
Edited book (chapter)
Chapter Title | Drought modelling based on artificial intelligence and neural network algorithms: a case study in Queensland, Australia |
---|---|
Book Chapter Category | Edited book (chapter) |
ERA Publisher ID | 3337 |
Book Title | Climate change adaptation in Pacific countries:fostering resilience and improving the quality of life |
Authors | Dayal, Kavina (Author), Deo, Ravinesh (Author) and Apan, Armando A. (Author) |
Editors | Filho, Walter Leal |
Edition | Part II |
Page Range | 177-198 |
Series | Climate Change Management |
Chapter Number | 11 |
Number of Pages | 22 |
Year | 2017 |
Publisher | Springer |
ISBN | 9783319500935 |
9783319500942 | |
ISSN | 1610-2010 |
Digital Object Identifier (DOI) | https://doi.org/10.1007/978-3-319-50094-2_11 |
Web Address (URL) | https://link.springer.com/chapter/10.1007/978-3-319-50094-2_11 |
Abstract | The search for better climate change adaptation techniques for addressing environmental and economic issues due to changing climate is of paramount interest in the current era. One of the many ways Pacific Island regions and its people get affected is by dry spells and drought events from extreme climates. A drought is simply a prolonged shortage of water supply in an area. The impact of drought varies both temporally and spatially that can be catastrophic for such regions with lack of resources and facilities to mitigate the drought impacts. Therefore, forecasting drought events using predictive models that have practical implications for understanding drought hydrology and water resources management can allow enough time to take appropriate adaption measures. This study investigates the feasibility of the Artificial Neural Network (ANN) algorithms for prediction of a drought index: Standardized Precipitation-Evapotranspiration Index (SPEI). The purpose of the study was to develop an ANN model to predict the index in two selected regions in Queensland, Australia. The first region, is named as the grassland and the second as the temperate region. The monthly gridded meteorological variables (precipitation, maximum and minimum temperature) that acted as input parameters in ANN model were obtained from Australian Water Availability Project (AWAP) for 1915–2013 period. The potential evapotranspiration (PET), calculated using thornthwaite method, was also an input variable, while SPEI was the predictand for the ANN model. The input data were divided into training (80%), validation (10%) and testing (10%) sets. To determine the optimum ANN model, the Levenberg-Marquardt and Broyden-Fletcher-Goldfarb-Shanno quasi-Newton backpropagation algorithms were used for training the ANN network and the tangent sigmoid, logarithmic sigmoid and linear activation algorithms were used for hidden transfer and output functions. The best architecture of input-hidden neuron-output neurons was 4-28-1 and 4-27-1 for grassland and temperate region, respectively. For evaluation and selection of the optimum ANN model, the statistical metrics: Coefficient of Determination (R 2 ), Willmott’s Index of Agreement (d), Nash-Sutcliffe Coefficient of Efficiency (E), Root Mean Squared Error (RMSE) and Mean Absolute Error (MAE) were employed. The R 2 , d, E, RMSE and MAE for optimum ANN models were 0.9839, 0.9909, 0.9838, 0.1338, 0.0882 and 0.9886, 0.9935, 0.9874, 0.1198, 0.0814 for grassland and temperate region, respectively. When prediction errors were analysed, a value of 0.0025 to 0.8224 was obtained for the grassland region, and a value of 0.0113 to 0.6667 was obtained for the temperate region, indicating that the ANN model exhibit a good skill in predicting the monthly SPEI. Based on the evaluation and statistical analysis of the predicted SPEI and its errors in the test period, we conclude that the ANN model can be used as a useful data-driven tool for forecasting drought events. Broadly, the ANN model can be applied for prediction of other climate related variables, and therefore can play a vital role in the development of climate change adaptation and mitigation plans in developed and developing nations, and most importantly, in the Pacific Island Nations where drought events have a detrimental impact on economic development. |
Keywords | Artificial Neural Network; climate modelling; data-driven model; drought prediction in Australia; Standardized Precipitation-Evapotranspiration Index |
ANZSRC Field of Research 2020 | 370108. Meteorology |
469999. Other information and computing sciences not elsewhere classified | |
460207. Modelling and simulation | |
370202. Climatology | |
410404. Environmental management | |
Public Notes | Files associated with this item cannot be displayed due to copyright restrictions. |
Byline Affiliations | School of Agricultural, Computational and Environmental Sciences |
School of Civil Engineering and Surveying | |
Event | 2016 IEEE Region 10 International Conference: Technologies for Smart Nation (TENCON 2016) |
IEEE Tencon (IEEE Region 10 Conference) | |
Journal Title | 2016 IEEE Region 10 International Conference Proceedings (TENCON 2016) |
Institution of Origin | University of Southern Queensland |
Event Details | IEEE Tencon (IEEE Region 10 Conference) Tencon IEEE Region 10 Conference Rank C C C C C C C C C C C C C C C C C |
Event Details | 2016 IEEE Region 10 International Conference: Technologies for Smart Nation (TENCON 2016) Event Date 22 to end of 25 Nov 2016 Event Location Singapore |
https://research.usq.edu.au/item/q3y1w/drought-modelling-based-on-artificial-intelligence-and-neural-network-algorithms-a-case-study-in-queensland-australia
1679
total views15
total downloads14
views this month0
downloads this month
Export as
Related outputs
The Development of a Novel Quantum Pre-processing Filter to Improve Image Classification Accuracy of Neural Network Models
Riaz, Farina, Abdulla, Shahab, Suzuki, Hajime, Ganguly, Srinjoy, Deo, Ravinesh C. and Hopkins, Susan. 2025. "The Development of a Novel Quantum Pre-processing Filter to Improve Image Classification Accuracy of Neural Network Models ." Journal of Data Science and Intelligent Systems. https://doi.org/10.47852/bonviewJDSIS52024231Hybrid CNN–GRU model for hourly flood forecasting index: case studies from the Fiji islands
Chand, Ravinesh, Deo, Ravinesh C., Ghimire, Sujan, Nguyen-Huy, Thong and Ali, Mumtaz. 2025. "Hybrid CNN–GRU model for hourly flood forecasting index: case studies from the Fiji islands." Stochastic Environmental Research and Risk Assessment. https://doi.org/10.1007/s00477-025-02964-8Electricity demand uncertainty modeling with Temporal Convolution Neural Network models
Ghimire, Sujan, Deo, Ravinesh C., Casillas-Perez, David, Salcedo-Sanz, Sancho, Acharya, Rajendra and Dinh, Toan. 2025. "Electricity demand uncertainty modeling with Temporal Convolution Neural Network models." Renewable and Sustainable Energy Reviews. 209. https://doi.org/10.1016/j.rser.2024.115097Half-hourly electricity price prediction model with explainable-decomposition hybrid deep learning approach
Ghimire, Sujan, Deo, Ravinesh C., Hopf, Konstantin, Liu, Hangyue, Casillas-Perez, David, Helwig, Andreas, Prasad, Salvin S., Perez-Aracil, Jorge, Barua, Prabal Datta and Salcedo-Sanz, Sancho. 2025. "Half-hourly electricity price prediction model with explainable-decomposition hybrid deep learning approach." Energy and AI. 20. https://doi.org/10.1016/j.egyai.2025.100492Explainable deep learning hybrid modeling framework for total suspended particles concentrations prediction
Ghimire, Sujan, Deo, Ravinesh C., Jiang, Ningbo, Ahmed, A. A. Masrur, Prasad, Salvin S., Casillas-Perez, David, Salcedo-Sanz, Sancho and Yaseen, Zaher Mundher. 2025. "Explainable deep learning hybrid modeling framework for total suspended particles concentrations prediction." Atmospheric Environment. 347. https://doi.org/10.1016/j.atmosenv.2025.121079Enhancing Food Security Through Home Gardening: A Case Study in Phoukhoud District, Lao PDR
Shrestha, Suraj, Maraseni, Tek and Apan, Armando. 2025. "Enhancing Food Security Through Home Gardening: A Case Study in Phoukhoud District, Lao PDR." Agriculture. 15 (7). https://doi.org/10.3390/agriculture15070716Explainable hybrid deep learning framework for enhancing multi-step solar ultraviolet-B radiation predictions
Prasad, Salvin S., Joseph, Lionel P., Ghimire, Sujan, Deo, Ravinesh C., Downs, Nathan J., Acharya, Rajendra and Yaseen, Zaher M.. 2025. "Explainable hybrid deep learning framework for enhancing multi-step solar ultraviolet-B radiation predictions." Atmospheric Environment. 343. https://doi.org/10.1016/j.atmosenv.2024.120951Multi‑step solar ultraviolet index prediction: integrating convolutional neural networks with long short‑term memory for a representative case study in Queensland, Australia
AL-Musaylh, Mohan, Al‑Dafaie, Kadhem, Downs, Nathan, Ghimire, Sujan, Ali, Mumtaz, Yaseen, Zaher Mundher, Igoe, Damien P., Deo, Ravinesh C., Paris, Alfo V. and A. Jebar, Mustapha A.. 2025. "Multi‑step solar ultraviolet index prediction: integrating convolutional neural networks with long short‑term memory for a representative case study in Queensland, Australia." Modeling Earth Systems and Environment. 11. https://doi.org/10.1007/s40808-024-02282-yAssessing the Spatio-temporal Activity Pattern and Habitat Use of Bengal Tiger (Panthera tigris tigris) Across Three Forest Management Regimes in Nepal
Maharjan, Amir, Maraseni, Tek, Allen, Benjamin L. and Apan, Armando. 2025. "Assessing the Spatio-temporal Activity Pattern and Habitat Use of Bengal Tiger (Panthera tigris tigris) Across Three Forest Management Regimes in Nepal." Biodiversity and Conservation. 34 (2), pp. 643-663. https://doi.org/10.1007/s10531-024-02991-xExplainable deeply-fused nets electricity demand prediction model: Factoring climate predictors for accuracy and deeper insights with probabilistic confidence interval and point-based forecasts
Ghimire, Sujan, AL-Musaylh, Mohanad S., Nguyen-Huy, Thong, Deo, Ravinesh C., Acharya, Rajendra, Casillas-Perez, David, Yaseen, Zaher Mundher and Salcedo-sanz, Sancho. 2025. "Explainable deeply-fused nets electricity demand prediction model: Factoring climate predictors for accuracy and deeper insights with probabilistic confidence interval and point-based forecasts." Applied Energy. 378 (Part A). https://doi.org/10.1016/j.apenergy.2024.124763The mitigating effect of street trees, urban flora, and the suburban environment on seasonal peak UV indices: A case study from Brisbane, Australia
Downs, Nathan James, Amar, Abdurazaq, Dearnaley, John, Butler, Harry, Dekeyser, Stijn, Igoe, Damien, Parisi, Alfio V., Raj, Nawin, Deo, Ravinesh and Turner, Joanna. 2025. "The mitigating effect of street trees, urban flora, and the suburban environment on seasonal peak UV indices: A case study from Brisbane, Australia." Photochemistry and Photobiology. 101 (1), pp. 251-266. https://doi.org/10.1111/php.13988The Application of Quantum Pre-processing Filter for Binary Image Classification with Small Samples
Riaz, Farina, Abdulla, Shahab, Suzuki, Hajime, Ganguly, Srinjoy, Deo, Ravinesh C. and Hopkins, Susan. 2024. "The Application of Quantum Pre-processing Filter for Binary Image Classification with Small Samples ." Journal of Data Science and Intelligent Systems. 3 (2), pp. 109-116. https://doi.org/10.47852/bonviewJDSIS42024229A Comprehensive Review of UAV-UGV Collaboration: Advancements and Challenges
Munasinghe, Isuru, Perera, Asanka and Deo, Ravinesh C.. 2024. "A Comprehensive Review of UAV-UGV Collaboration: Advancements and Challenges." Journal of Sensor and Actuator Networks. 13 (6). https://doi.org/10.3390/jsan13060081Retinal Health Screening Using Artificial Intelligence with Digital Fundus Images: A Review of the Last Decade (2012-2023)
Islam, Saad, Deo, Ravinesh C., Barua, Prabal Datta, Soar, Jeffrey, Yu, Ping and Acharya, U. Rajendra. 2024. "Retinal Health Screening Using Artificial Intelligence with Digital Fundus Images: A Review of the Last Decade (2012-2023)." IEEE Access. 12, pp. 176630-176685. https://doi.org/10.1109/ACCESS.2024.3477420Hydropower and Climate Resilience of Nepal Himalaya: A bottom-up Hydrological Approach
Bhattarai, Utsav, Maraseni, Tek, Devkota, Laxmi Prasad and Apan, Armando. 2024. "Hydropower and Climate Resilience of Nepal Himalaya: A bottom-up Hydrological Approach ." Earth Systems and Environment. https://doi.org/10.1007/s41748-024-00529-3Assessing the Theoretical Scope of Environmental Justice in Contemporary Literature and Developing a Pragmatic Monitoring Framework
Pandey, Hari Prasad, Maraseni, Tek Narayan and Apan, Armando. 2024. "Assessing the Theoretical Scope of Environmental Justice in Contemporary Literature and Developing a Pragmatic Monitoring Framework ." Sustainability. 16 (24). https://doi.org/10.3390/su162410799Insights into Ecological Resettlements and Conservation-led Displacements: A Systematic Review
Pandey, Hari Prasad, Maraseni, Tek Narayan and Apan, Armando. 2024. "Insights into Ecological Resettlements and Conservation-led Displacements: A Systematic Review." Environmental Management (New York): an international journal for decision-makers, scientists and environmental auditors. https://doi.org/10.1007/s00267-024-02097-8Enhancing systematic literature review adapting 'double diamond approach'
Pandey, Hari Prasad, Maraseni, Tek Narayan and Apan, Armando A.. 2024. "Enhancing systematic literature review adapting 'double diamond approach' ." Heliyon. 10 (22). https://doi.org/10.1016/j.heliyon.2024.e40581Forecasting River Water Temperature Using Explainable Artificial Intelligence and Hybrid Machine Learning: Case Studies in Menindee Region in Australia
Briceno Medina, Leyde, Joehnk, Klaus, Deo, Ravinesh C., Ali, Mumtaz, Prasad, Salvin S. and Downs, Nathan. 2024. "Forecasting River Water Temperature Using Explainable Artificial Intelligence and Hybrid Machine Learning: Case Studies in Menindee Region in Australia." Water. 16 (24). https://doi.org/10.3390/w16243720Explainable artificial intelligence-machine learning models to estimate overall scores in tertiary preparatory general science course
Ghimire, Sujan, Abdulla, Shahab, Joseph, Lionel P., Prasad, Salvin, Murphy, Angela, Devi, Aruna, Barua, Prabal Datta, Deo, Ravinesh C., Acharya, Rajendra and Yaseen, Zaher Mundher. 2024. "Explainable artificial intelligence-machine learning models to estimate overall scores in tertiary preparatory general science course." Computers and Education: Artificial Intelligence. 7. https://doi.org/10.1016/j.caeai.2024.100331Forecasting Multi-Step Soil Moisture with Three-Phase Hybrid Wavelet-Least Absolute Shrinkage Selection Operator-Long Short-Term Memory Network (moDWT-Lasso-LSTM) Model
Jayasinghe, W. J. M. Lakmini Prarthana, Deo, Ravinesh C., Raj, Nawin, Ghimire, Sujan, Yaseen, Zaher Mundher, Nguyen-Huy, Thong and Ghahramani, Afshin. 2024. "Forecasting Multi-Step Soil Moisture with Three-Phase Hybrid Wavelet-Least Absolute Shrinkage Selection Operator-Long Short-Term Memory Network (moDWT-Lasso-LSTM) Model." Water. 16 (21), p. 3133. https://doi.org/10.3390/w16213133Artificial Intelligence-Empowered Doppler Weather Profile for Low-Earth-Orbit Satellites
Sharma, Ekta, Deo, Ravinesh C., Davey, Christopher P and Carter, Brad D.. 2024. "Artificial Intelligence-Empowered Doppler Weather Profile for Low-Earth-Orbit Satellites." Sensors. 24 (16). https://doi.org/10.3390/s24165271Unlocking the tapestry of conservation: Navigating ecological resettlement policies in Nepal
Pandey, Hari Prasad, Maraseni, Tek Narayan, Apan, Armando and Aryal, Kishor. 2024. "Unlocking the tapestry of conservation: Navigating ecological resettlement policies in Nepal." Science of the Total Environment. 946. https://doi.org/10.1016/j.scitotenv.2024.174335Decoupling REDD+ understanding of local stakeholders on the onset of materializing carbon credits from forests in Nepal
Pandey, Hari Prasad, Maraseni, Tek Narayan, Apan, Armando and Bhusal, Shreejana. 2024. "Decoupling REDD+ understanding of local stakeholders on the onset of materializing carbon credits from forests in Nepal." Forest Ecosystems. 11. https://doi.org/10.1016/j.fecs.2024.100239Achieving SOC Conservation without Land-Use Changes between Agriculture and Forests
Pandey, Hari Prasad, Maraseni, Tek Narayan, Apan, Armando and Bhusal, Shreejana. 2024. "Achieving SOC Conservation without Land-Use Changes between Agriculture and Forests." Sustainability. 16 (16). https://doi.org/10.3390/su16167207Wavelet-fusion image super-resolution model with deep learning for downscaling remotely-sensed, multi-band spectral albedo imagery
Karalasingham, Sagthitharan, Deo, Ravinesh C., Casillas-Perez, David, Raj, Nawin and Salcedo-sanz, Sancho. 2024. "Wavelet-fusion image super-resolution model with deep learning for downscaling remotely-sensed, multi-band spectral albedo imagery." Remote Sensing Applications: Society and Environment. 36. https://doi.org/10.1016/j.rsase.2024.101333Half-hourly electricity price prediction with a hybrid convolution neural network-random vector functional link deep learning approach
Ghimire, Sujan, Deo, Ravinesh C., Casillas-Perez, David, Sharma, Ekta, Salcedo-sanz, Sancho, Barua, Prabal and Acharya, U. Rajendra. 2024. "Half-hourly electricity price prediction with a hybrid convolution neural network-random vector functional link deep learning approach." Applied Energy. 374. https://doi.org/10.1016/j.apenergy.2024.123920Facilitating sustainable energy transition of Nepal: A best-fit model to prioritize influential socio-economic and climate perception factors on household energy behaviour
Bhattarai, Utsav, Maraseni, Tek, Devkota, Laxmi Prasad and Apan, Armando. 2024. "Facilitating sustainable energy transition of Nepal: A best-fit model to prioritize influential socio-economic and climate perception factors on household energy behaviour." Energy for Sustainable Development: the journal of the international energy initiative. 81. https://doi.org/10.1016/j.esd.2024.101505Towards next-generation federated learning: A case study on privacy attacks in artificial intelligence systems
Sharma, Ekta, Deo, Ravinesh C, Davey, Christopher P., Carter, Brad D. and Salcedo-sanz, Sancho. 2024. "Towards next-generation federated learning: A case study on privacy attacks in artificial intelligence systems." 2024 IEEE Conference on Artificial Intelligence (CAI 2024). Singapore 25 - 27 Jun 2024 United States. IEEE (Institute of Electrical and Electronics Engineers). https://doi.org/10.1109/CAI59869.2024.00259Poster: Cloud Computing with AI-empowered Trends in Software-Defined Radios: Challenges and Opportunities
Sharma, Ekta, Deo, Ravinesh C., Davey, Christopher P., Carter, Brad D. and Salcedo-sanz, Sancho. 2024. "Poster: Cloud Computing with AI-empowered Trends in Software-Defined Radios: Challenges and Opportunities." 2024 IEEE 25th International Symposium on a World of Wireless, Mobile and Multimedia Networks (WoWMoM 2024). Perth, Australia 04 - 07 Jun 2024 United States. IEEE (Institute of Electrical and Electronics Engineers). https://doi.org/10.1109/WoWMoM60985.2024.00054Modelling Floodplain Vegetation Response to Climate Change, Using the Soil and Water Assessment Tool (SWAT) Model Simulated LAI, Applying Different GCM’s Future Climate Data and MODIS LAI Data
Muhury, Newton, Apan, Armando and Maraseni, Tek. 2024. "Modelling Floodplain Vegetation Response to Climate Change, Using the Soil and Water Assessment Tool (SWAT) Model Simulated LAI, Applying Different GCM’s Future Climate Data and MODIS LAI Data ." Remote Sensing. 16 (7). https://doi.org/10.3390/rs16071204A systematic literature review: Trends and current state of research on Bengal tiger (Panthera tigris tigris), 2010-2022
Maharjan, Amir, Maraseni, Tek, Allen, Benjamin L. and Apan, Armando. 2024. "A systematic literature review: Trends and current state of research on Bengal tiger (Panthera tigris tigris), 2010-2022." Biodiversity and Conservation. 33 (6-7), pp. 1871-1894. https://doi.org/10.1007/s10531-024-02856-3Evaluating four decades of energy policy evolution for sustainable development of a South Asian country-Nepal: A comprehensive review
Bhattarai, Utsav, Maraseni, Tek, Devkota, Laxmi P. and Apan, Armando. 2024. "Evaluating four decades of energy policy evolution for sustainable development of a South Asian country-Nepal: A comprehensive review." Sustainable Development. 32 (6), pp. 6703-6731. https://doi.org/10.1002/sd.3053Unravelling the impact of climate change on honey bees: An ensemble modelling approach to predict shifts in habitat suitability in Queensland, Australia
Tennakoon, Sarasie, Apan, Armando and Maraseni, Tek. 2024. "Unravelling the impact of climate change on honey bees: An ensemble modelling approach to predict shifts in habitat suitability in Queensland, Australia." Ecology and Evolution. 14 (4). https://doi.org/10.1002/ece3.11300Atmospheric Visibility and Cloud Ceiling Predictions With Hybrid IIS-LSTM Integrated Model: Case Studies for Fiji's Aviation Industry
Raj, Shiveel, Deo, Ravinesh C., Sharma, Ekta, Prasad, Ramendra, Dinh, Toan and Salcedo-sanz, Sancho. 2024. "Atmospheric Visibility and Cloud Ceiling Predictions With Hybrid IIS-LSTM Integrated Model: Case Studies for Fiji's Aviation Industry." IEEE Access. 12, pp. 72530-72543. https://doi.org/10.1109/ACCESS.2024.3401091Point-based and probabilistic electricity demand prediction with a Neural Facebook Prophet and Kernel Density Estimation model
Ghimire, Sujan, Deo, Ravinesh C., Pourmousavi, S. Ali, Casillas-Perez, David and Salcedo-sanz, Sancho. 2024. "Point-based and probabilistic electricity demand prediction with a Neural Facebook Prophet and Kernel Density Estimation model." Engineering Applications of Artificial Intelligence. 135. https://doi.org/10.1016/j.engappai.2024.108702End-to-end learning of adaptive coded modulation schemes for resilient wireless communications
Davey, Christopher P., Shakeel, Ismail, Deo, Ravinesh C., Sharma, Ekta, Salcedo-sanz, Sancho and Soar, Jeffrey. 2024. "End-to-end learning of adaptive coded modulation schemes for resilient wireless communications." Applied Soft Computing. 159. https://doi.org/10.1016/j.asoc.2024.111672Deep Learning Based Over-the-Air Training of Wireless Communication Systems without Feedback
Davey, Christopher P., Shakeel, Ismail, Deo, Ravinesh C. and Salcedo-sanz, Sancho. 2024. "Deep Learning Based Over-the-Air Training of Wireless Communication Systems without Feedback." Sensors. 24 (10). https://doi.org/10.3390/s24102993Enviro-economic and feasibility analysis of industrial hemp value chain: A systematic literature review
Budhathoki, Rajan, Maraseni, Tek and Apan, Armando. 2024. "Enviro-economic and feasibility analysis of industrial hemp value chain: A systematic literature review." GCB Bioenergy. 16 (6). https://doi.org/10.1111/gcbb.13141A composite indicator-based method to assess the energy security of Nepal and prospects of cross-border electricity sharing in South Asia
Bhattarai, Utsav, Maraseni, Tek, Devkota, Laxmi and Apan, Armando. 2024. "A composite indicator-based method to assess the energy security of Nepal and prospects of cross-border electricity sharing in South Asia." Environmental Development. 51. https://doi.org/10.1016/j.envdev.2024.101002A playground shade index for standardising ultraviolet protection assessments of open, mixed and protected outdoor recreational spaces
Downs, Nathan, Butler, Harry, Dexter, Benjamin, Raj, Nawin, Downs, Melanie, Turner, Joanna, Dekeyser, Stijn, Deo, Ravinesh, Vanos, Jennifer, Igoe, Damien and Parisi, Alfio V. 2024. "A playground shade index for standardising ultraviolet protection assessments of open, mixed and protected outdoor recreational spaces." 6th International conference UV and Skin Cancer Prevention. Brisbane, Australia 11 - 15 Sep 2024 Australia.Identifying human elephant conflict hotspots through satellite remote sensing and GIS to support conflict mitigation
Gunawansa, Thakshila D., Perera, Kithsiri, Apan, Armando and Hettiarachchi, Nandita K.. 2024. "Identifying human elephant conflict hotspots through satellite remote sensing and GIS to support conflict mitigation ." Remote Sensing Applications: Society and Environment. 35. https://doi.org/10.1016/j.rsase.2024.101261Multi-Step-Ahead Wind Speed Forecast System: Hybrid Multivariate Decomposition and Feature Selection-Based Gated Additive Tree Ensemble Model
Joseph, Lionel P., Deo, Ravinesh C., Casillas-Perez, David, Prasad, Ramendra, Raj, Nawin and Salcedo-sanz, Sancho. 2024. "Multi-Step-Ahead Wind Speed Forecast System: Hybrid Multivariate Decomposition and Feature Selection-Based Gated Additive Tree Ensemble Model." IEEE Access. 12, pp. 58750-58777. https://doi.org/10.1109/ACCESS.2024.3392899Uncovering the Hidden Carbon Treasures of the Philippines’ Towering Mountains: A Synergistic Exploration Using Satellite Imagery and Machine Learning
Altarez, Richard Dein D., Apan, Armando and Maraseni, Tek. 2024. "Uncovering the Hidden Carbon Treasures of the Philippines’ Towering Mountains: A Synergistic Exploration Using Satellite Imagery and Machine Learning." PFG - Journal of Photogrammetry, Remote Sensing and Geoinformation Science. 92 (1), pp. 55-73. https://doi.org/10.1007/s41064-023-00264-wDeep Learning-Assisted Sensitive 3C-SiC Sensor for Long-Term Monitoring of Physical Respiration
Tran, Thi Lap, Nguyen, Duy Van, Nguyen, Hung, Nguyen, Thi Phuoc Van, Song, Pingan, Deo, Ravinesh C, Moloney, Clint, Dao, Viet Dung, Nguyen, Nam-Trung and Dinh, Toan. 2024. "Deep Learning-Assisted Sensitive 3C-SiC Sensor for Long-Term Monitoring of Physical Respiration." Advanced Sensor Research. 3 (8). https://doi.org/10.1002/adsr.202300159Copula-Probabilistic Flood Risk Analysis with an Hourly Flood Monitoring Index
Chand, Ravinesh, Nguyen-Huy, Thong, Deo, Ravinesh C., Ghimire, Sujan, Ali, Mumtaz and Ghahramani, Afshin. 2024. "Copula-Probabilistic Flood Risk Analysis with an Hourly Flood Monitoring Index." Water. 16 (11). https://doi.org/10.3390/w16111560Short-term wind speed forecasting using an optimized three-phase convolutional neural network fused with bidirectional long short-term memory network model
Joseph, Lionel P., Deo, Ravinesh C., Casillas-Perez, David, Prasad, Ramendra, Raj, Nawin and Salcedo-sanz, Sancho. 2024. "Short-term wind speed forecasting using an optimized three-phase convolutional neural network fused with bidirectional long short-term memory network model." Applied Energy. 359. https://doi.org/10.1016/j.apenergy.2024.122624Probabilistic-based electricity demand forecasting with hybrid convolutional neural network-extreme learning machine model
Ghimire, Sujan, Deo, Ravinesh C., Casillas-Perez, David, Salcedo-sanz, Sancho, Pourmousavi, S. Ali and Acharya, U. Rajendra. 2024. "Probabilistic-based electricity demand forecasting with hybrid convolutional neural network-extreme learning machine model." Engineering Applications of Artificial Intelligence. 132. https://doi.org/10.1016/j.engappai.2024.107918Electricity demand error corrections with attention bi-directional neural networks
Ghimire, Sujan, Deo, Ravinesh C., Casillas-Perez, David and Salcedo-sanz, Sancho. 2024. "Electricity demand error corrections with attention bi-directional neural networks." Energy. 291. https://doi.org/10.1016/j.energy.2023.129938Micromachined Mechanical Resonant Sensors: From Materials, Structural Designs to Applications
Dinh, Toan, Rais-Zadeh, Mina, Nguyen, Thanh, Phan, Hoang-Phuong, Song, Pingan, Deo, Ravinesh, Dao, Dzung, Nguyen, Nam-Trung and Bell, John. 2024. "Micromachined Mechanical Resonant Sensors: From Materials, Structural Designs to Applications." Advanced Materials Technologies. 9 (2). https://doi.org/10.1002/admt.202300913Machine learning for expediting next-generation of fire-retardant polymer composites
Jafari, Pooya, Zhang, Ruoran, Huo, Siqi, Wang, Qingsheng, Yong, Jianming, Hong, Min, Deo, Ravinesh, Wang, Hao and Song, Pingan. 2024. "Machine learning for expediting next-generation of fire-retardant polymer composites." Composites Communications. 45. https://doi.org/10.1016/j.coco.2023.101806Very short-term solar ultraviolet-A radiation forecasting system with cloud cover images and a Bayesian optimized interpretable artificial intelligence model
Prasad, Salvin Sanjesh, Deo, Ravinesh Chand, Downs, Nathan James, Casillas-Perez, David, Salcedo-sanz, Sancho and Parisi, Alfio Venerando. 2024. "Very short-term solar ultraviolet-A radiation forecasting system with cloud cover images and a Bayesian optimized interpretable artificial intelligence model ." Expert Systems with Applications. 236. https://doi.org/10.1016/j.eswa.2023.121273Short-term wave power forecasting with hybrid multivariate variational mode decomposition model integrated with cascaded feedforward neural networks
Ali, Mumtaz, Prasad, Ramendra, Jamei, Mehdi, Malik, Anurag, Xiang, Yong, Abdulla, Shahab, Deo, Ravinesh C., Farooque, Aitazaz A. and Labban, Abdulhaleem H.. 2024. "Short-term wave power forecasting with hybrid multivariate variational mode decomposition model integrated with cascaded feedforward neural networks." Renewable Energy. 221. https://doi.org/10.1016/j.renene.2023.119773Two-step deep learning framework with error compensation technique for short-term, half-hourly electricity price forecasting
Ghimire, Sujan, Deo, Ravinesh C., Casillas-Perez, David and Salcedo-sanz, Sancho. 2024. "Two-step deep learning framework with error compensation technique for short-term, half-hourly electricity price forecasting." Applied Energy. 353 (Part A). https://doi.org/10.1016/j.apenergy.2023.122059Hybrid Deep Learning Model for Wave Height Prediction in Australia's Wave Energy Region
Ahmed, Abul Abrar Masrur, Jui, S Janifer Jabin, AL-Musaylh, Mohanad S., Raj, Nawin, Saha, Reepa, Deo, Ravinesh C and Saha, Sanjoy Kumar. 2024. "Hybrid Deep Learning Model for Wave Height Prediction in Australia's Wave Energy Region." Applied Soft Computing. 150. https://doi.org/10.1016/j.asoc.2023.111003Modelling future spatial distribution of peanut crops in Australia under climate change scenarios
Haerani, Haerani, Apan, Armando, Nguyen-Huy, Thong and Basnet, Badri. 2024. "Modelling future spatial distribution of peanut crops in Australia under climate change scenarios." Geo-spatial Information Science. 27 (5), pp. 1585-1604. https://doi.org/10.1080/10095020.2022.2155255Author Correction: Explainable AI approach with original vegetation data classifies spatio‑temporal nitrogen in flows from ungauged catchments to the Great Barrier Reef
O’Sullivan, Cherie M., Deo, Ravinesh C. and Ghahramani, Afshin. 2023. "Author Correction: Explainable AI approach with original vegetation data classifies spatio‑temporal nitrogen in flows from ungauged catchments to the Great Barrier Reef." Scientific Reports. 13 (1). https://doi.org/10.1038/s41598-023-48938-0Channel-Agnostic Training of Transmitter and Receiver for Wireless Communications
Davey, Christopher P., Shakeel, Ismail, Deo, Ravinesh C. and Salcedo-sanz, Sancho. 2023. "Channel-Agnostic Training of Transmitter and Receiver for Wireless Communications ." Sensors. 23 (24). https://doi.org/10.3390/s23249848Deep Image Analysis for Microalgae Identification
Soar, Jeffrey, Lih, Oh Shu, Wen, Loh Hui, Ward, Aleth, Sharma, Ekta, Deo, Ravinesh C., Barua, Prabal Datta, Tan, Ru-San, Rinen, Eliezer and Acharya, Rajendra. 2023. "Deep Image Analysis for Microalgae Identification." Lecture notes in computer science. Switzerland . Springer. pp. 280-292TIMESAT software for estimating crop seasonality parameter
Haerani, Haerani and Apan, Armando. 2023. "TIMESAT software for estimating crop seasonality parameter." 1st Unhas International Conference on Agricultural Technology (UICAT 2021). Makassar, Indonesia 27 - 28 Oct 2021 United States. AIP Publishing. https://doi.org/10.1063/5.0118791Statistical and spatial analysis for soil heavy metals over the Murray-Darling river basin in Australia
Tao, Hai, Al-Hilali, Aqeel Ali, Ahmed, Ali M., Mussa, Zainab Haider, Falah, Mayadah W., Abed, Salwan Ali, Deo, Ravinesh, Jawad, Ali H., Maulud, Khairul Nizam Abdul, Latif, Mohd Talib and Yaseen, Zaher Mundher. 2023. "Statistical and spatial analysis for soil heavy metals over the Murray-Darling river basin in Australia." Chemosphere. 317. https://doi.org/10.1016/j.chemosphere.2023.137914Enhanced joint hybrid deep neural network explainable artificial intelligence model for 1-hr ahead solar ultraviolet index prediction
Prasad, Salvin S., Deo, Ravinesh C., Salcedo-sanz, Sancho, Downs, Nathan J., Casillas-Perez, David and Parisi, Alfio V.. 2023. "Enhanced joint hybrid deep neural network explainable artificial intelligence model for 1-hr ahead solar ultraviolet index prediction." Computer Methods and Programs in Biomedicine. 241. https://doi.org/10.1016/j.cmpb.2023.107737Ampelomyces mycoparasites of powdery mildews–a review
Prahl, Rosa E., Khan, Shahjahan and Deo, Ravinesh C.. 2023. "Ampelomyces mycoparasites of powdery mildews–a review." Canadian Journal of Plant Pathology. 45 (4), pp. 391-404. https://doi.org/10.1080/07060661.2023.2206378A fuzzy-based cascade ensemble model for improving extreme wind speeds prediction
Pelaez-Rodriguez, C., Perez-Aracil, J., Prieto-Godino, L., Ghimire, S., Deo, R. and Salcedo-sanz, S.. 2023. "A fuzzy-based cascade ensemble model for improving extreme wind speeds prediction." Journal of Wind Engineering and Industrial Aerodynamics. 240. https://doi.org/10.1016/j.jweia.2023.105507Explainable AI approach with original vegetation data classifies spatio-temporal nitrogen in flows from ungauged catchments to the Great Barrier Reef
O’Sullivan, Cherie M., Deo, Ravinesh C. and Ghahramani, Afshin. 2023. "Explainable AI approach with original vegetation data classifies spatio-temporal nitrogen in flows from ungauged catchments to the Great Barrier Reef." Scientific Reports. 13 (1). https://doi.org/10.1038/s41598-023-45259-0A Novel Attention-Based Model for Semantic Segmentation of Prostate Glands Using Histopathological Images
Inamdar, Mahesh Anil, Raghavendra, U., Gudigar, Anjan, Bhandary, Sarvesh, Salvi, Massimo, Deo, Ravinesh C., Barua, Prabal Datta, Ciaccio, Edward J., Molinari, Filippo and Acharya, RU. Rajendra. 2023. "A Novel Attention-Based Model for Semantic Segmentation of Prostate Glands Using Histopathological Images." IEEE Access. 11, pp. 108982-108994. https://doi.org/10.1109/ACCESS.2023.3321273Efficient daily electricity demand prediction with hybrid deep-learning multi-algorithm approach
Ghimire, Sujan, Deo, Ravinesh C., Casillas-Perez, David and Salcedo-sanz, Sancho. 2023. "Efficient daily electricity demand prediction with hybrid deep-learning multi-algorithm approach." Energy Conversion and Management. 297. https://doi.org/10.1016/j.enconman.2023.117707Application of machine learning to assess people's perception of household energy in the developing world: A case of Nepal
Bhattarai, Utsav, Maraseni, Tek, Devkota, Laxmi Prasad and Apan, Armando. 2023. "Application of machine learning to assess people's perception of household energy in the developing world: A case of Nepal." Energy and AI. 14. https://doi.org/10.1016/j.egyai.2023.100303Understanding production possibility frontiers and utility values of ecosystem services in the Himalayas: An analysis of the supply-demand divide
Aryal, Kishor, Maraseni, Tek and Apan, Armando. 2023. "Understanding production possibility frontiers and utility values of ecosystem services in the Himalayas: An analysis of the supply-demand divide." Journal of Cleaner Production. 423. https://doi.org/10.1016/j.jclepro.2023.138725Automated detection of airflow obstructive diseases: A systematic review of the last decade (2013-2022)
Xu, Shuting, Deo, Ravinesh C, Soar, Jeffrey, Barua, Prabal Datta, Faust, Oliver, Homaira, Nusrat, Jaffe, Adam, Kabir, Arm Luthful and Acharya, U. Rajendra. 2023. "Automated detection of airflow obstructive diseases: A systematic review of the last decade (2013-2022)." Computer Methods and Programs in Biomedicine. 241. https://doi.org/10.1016/j.cmpb.2023.107746Brain tumor detection and screening using artificial intelligence techniques: Current trends and future perspectives
Raghavendra, U., Gudigar, Anjan, Paul, Aritra, Goutham, T.S., Inamdar, Mahesh Anil, Hegde, Ajay, Dev, Aruna, Ooi, Chui Ping, Deo, Ravinesh C., Barua, Prabal Datta, Molinari, Filippo, Ciaccio, Edward J. and Acharya, U. Rajendra. 2023. "Brain tumor detection and screening using artificial intelligence techniques: Current trends and future perspectives." Computers in Biology and Medicine. 163. https://doi.org/10.1016/j.compbiomed.2023.107063Application of Entropy for Automated Detection of Neurological Disorders With Electroencephalogram Signals: A Review of the Last Decade (2012-2022)
Jui, S. Janifer Jabin, Deo, Ravinesh C. Deo, Barua, Prabal Datta, Devi, Aruna, Soar, Jeffrey and Acharya, U. Rajendra. 2023. "Application of Entropy for Automated Detection of Neurological Disorders With Electroencephalogram Signals: A Review of the Last Decade (2012-2022)." IEEE Access. 11, pp. 71905-71924. https://doi.org/10.1109/ACCESS.2023.3294473Integrated Multi-Head Self-Attention Transformer model for electricity demand prediction incorporating local climate variables
Ghimire, Sujan, Nguyen-Huy, Thong, AL-Musaylh, Mohanad S., Deo, Ravinesh C., Casillas-Perez, David and Salcedo-sanz, Sancho. 2023. "Integrated Multi-Head Self-Attention Transformer model for electricity demand prediction incorporating local climate variables." Energy and AI. 14. https://doi.org/10.1016/j.egyai.2023.100302Greenery change and its impact on human-elephant conflict in Sri Lanka: a model-based assessment using Sentinel-2 imagery
Gunawansa, Thakshila D., Perera, Kithsiri, Apan, Armando and Hettiarachchi, Nandita. 2023. "Greenery change and its impact on human-elephant conflict in Sri Lanka: a model-based assessment using Sentinel-2 imagery." International Journal of Remote Sensing. 44 (16), pp. 5121-5146. https://doi.org/10.1080/01431161.2023.2244644Decoding the impacts of space and time on honey bees: GIS based fuzzy AHP and fuzzy overlay to assess land suitability for apiary sites in Queensland, Australia
Mudiyanselage, Sarasie, Apan, Armando, Maraseni, Tek and Altarez, Richard Dein D.. 2023. "Decoding the impacts of space and time on honey bees: GIS based fuzzy AHP and fuzzy overlay to assess land suitability for apiary sites in Queensland, Australia." Applied Geography. 155. https://doi.org/10.1016/j.apgeog.2023.102951Rationalizing donations and subsidies: Energy ecosystem development for sustainable renewable energy transition in Nepal
Bhattarai, Utsav, Maraseni, Tek, Apan, Armando and Devkota, Laxmi Prasad. 2023. "Rationalizing donations and subsidies: Energy ecosystem development for sustainable renewable energy transition in Nepal." Energy Policy. 177. https://doi.org/10.1016/j.enpol.2023.113570Preference, perceived change, and professed relationship among ecosystem services in the Himalayas
Aryal, Kishor, Maraseni, Tek and Apan, Armando. 2023. "Preference, perceived change, and professed relationship among ecosystem services in the Himalayas." Journal of Environmental Management. 344. https://doi.org/10.1016/j.jenvman.2023.118522Examining policy− institution− program (PIP) responses against the drivers of ecosystem dynamics. A chronological review (1960–2020) from Nepal
Aryal, Kishor, Maraseni, Tek and Apan, Armando. 2023. "Examining policy− institution− program (PIP) responses against the drivers of ecosystem dynamics. A chronological review (1960–2020) from Nepal." Land Use Policy: the international journal covering all aspects of land use. 132, p. 106789. https://doi.org/10.1016/j.landusepol.2023.106789Spatial dynamics of biophysical trade-offs and synergies among ecosystem services in the Himalayas
Aryal, Kishor, Maraseni, Tek and Apan, Armando. 2023. "Spatial dynamics of biophysical trade-offs and synergies among ecosystem services in the Himalayas." Ecosystem Services: science, policy and pratice. 59. https://doi.org/10.1016/j.ecoser.2022.101503Comparing global and local land cover maps for ecosystem management in the Himalayas
Aryal, Kishor, Apan, Armando and Maraseni, Tek. 2023. "Comparing global and local land cover maps for ecosystem management in the Himalayas." Remote Sensing Applications: Society and Environment. 30. https://doi.org/10.1016/j.rsase.2023.100952The human-elephant conflict in Sri Lanka: history and present status
Gunawansa, Thakshila, Perera, Kithsiri, Apan, Armando and Hettiarachchi, Nandita K.. 2023. "The human-elephant conflict in Sri Lanka: history and present status." Biodiversity and Conservation. 32 (10), pp. 3025-3052. https://doi.org/10.1007/s10531-023-02650-7The Great 2011 Thailand flood disaster revisited: Could it have been mitigated by different dam operations based on better weather forecasts?
Loc, Ho Huu, Emadzadeh, Adel, Park, Edward, Nontikansak, Piyanuch and Deo, Ravinesh C.. 2023. "The Great 2011 Thailand flood disaster revisited: Could it have been mitigated by different dam operations based on better weather forecasts? " Environmental Research. 216 (Part 2). https://doi.org/10.1016/j.envres.2022.114493Convolutional Neural Network-Based Deep Learning Approach for Automatic Flood Mapping Using NovaSAR-1 and Sentinel-1 Data
Apan, Armando, Andrew, Ogbaje, Paudyal, Dev Raj and Perera, Kithsiri. 2023. "Convolutional Neural Network-Based Deep Learning Approach for Automatic Flood Mapping Using NovaSAR-1 and Sentinel-1 Data." ISPRS International Journal of Geo-Information. 12 (194), pp. 1-26. https://doi.org/10.3390/ijgi12050194Comparison of machine learning methods emulating process driven crop models
Johnston, David B., Pembleton, Keith G., Huth, Neil I. and Deo, Ravinesh C.. 2023. "Comparison of machine learning methods emulating process driven crop models ." Environmental Modelling and Software. 162. https://doi.org/10.1016/j.envsoft.2023.105634A novel approach based on integration of convolutional neural networks and echo state network for daily electricity demand prediction
Ghimire, Sujan, Nguyen-Huy, Thong, AL-Musaylh, Mohanad S., Deo, Ravinesh, Casillas-Perez, David and Salcedo-sanz, Sancho. 2023. "A novel approach based on integration of convolutional neural networks and echo state network for daily electricity demand prediction." Energy. 275, p. 127430. https://doi.org/10.1016/j.energy.2023.127430Accurate Image Multi-Class Classification Neural Network Model with Quantum Entanglement Approach
Riaz, Farina, Abdulla, Shahab, Suzuki, Hajime, Ganguly, Srinjoy, Deo, Ravinesh C. and Hopkins, Susan. 2023. "Accurate Image Multi-Class Classification Neural Network Model with Quantum Entanglement Approach." Sensors. 23 (5), pp. 1-11. https://doi.org/10.3390/s23052753Near real-time wind speed forecast model with bidirectional LSTM networks
Joseph, Lionel P., Deo, Ravinesh C., Prasad, Ramendra, Salcedo-Sanz, Sancho, Raj, Nawin and Soar, Jeffrey. 2023. "Near real-time wind speed forecast model with bidirectional LSTM networks." Renewable Energy. 204, pp. 39-58. https://doi.org/10.1016/j.renene.2022.12.123Downscaling Surface Albedo to Higher Spatial Resolutions With an Image Super-Resolution Approach and PROBA-V Satellite Images
Deo, Ravinesh C., Karalasingham, Sagthitharan, Casillas-Perez, David, Raj, Narwin and Salcedo-sanz, Sancho. 2023. "Downscaling Surface Albedo to Higher Spatial Resolutions With an Image Super-Resolution Approach and PROBA-V Satellite Images." IEEE Access. 11, pp. 5558-5577. https://doi.org/10.1109/ACCESS.2023.3236253A call for ‘management authorship’ in community forestry
Aryal, Kishor, Maraseni, Tek and Apan, Armando. 2023. "A call for ‘management authorship’ in community forestry." Environmental Science and Policy. 139, pp. 204-208. https://doi.org/10.1016/j.envsci.2022.10.023Transforming agroforestry in contested landscapes: A win-win solution to trade-offs in ecosystem services in Nepal
Aryal, Kishor, Maraseni, Tek and Apan, Armando. 2023. "Transforming agroforestry in contested landscapes: A win-win solution to trade-offs in ecosystem services in Nepal." Science of the Total Environment. 857 (Part 1). https://doi.org/10.1016/j.scitotenv.2022.159301Developing a novel hybrid method based on dispersion entropy and adaptive boosting algorithm for human activity recognition
Diykh, Mohammed, Abdulla, Shahab, Deo, Ravinesh C, Siuly, Siuly and Ali, Mumtaz. 2023. "Developing a novel hybrid method based on dispersion entropy and adaptive boosting algorithm for human activity recognition." Computer Methods and Programs in Biomedicine. 229. https://doi.org/10.1016/j.cmpb.2022.107305Cloud cover bias correction in numerical weather models for solar energy monitoring and forecasting systems with kernel ridge regression
Deo, Ravinesh C., Ahmed, A.A. Masrur, Casillas-Perez, David, Pourmousavi, S. Ali, Segal, Gary, Yu, Yanshan and Salcedo-sanz, Sancho. 2023. "Cloud cover bias correction in numerical weather models for solar energy monitoring and forecasting systems with kernel ridge regression." Renewable Energy. 203, pp. 113-130. https://doi.org/10.1016/j.renene.2022.12.048Deep learning U-Net classification of Sentinel-1 and 2 fusions effectively demarcates tropical montane forest's deforestation
Altarez, Richard Dein D., Apan, Armando and Maraseni, Tek. 2023. "Deep learning U-Net classification of Sentinel-1 and 2 fusions effectively demarcates tropical montane forest's deforestation." Remote Sensing Applications: Society and Environment. 29. https://doi.org/10.1016/j.rsase.2022.100887Deep Multi-Stage Reference Evapotranspiration Forecasting Model: Multivariate Empirical Mode Decomposition Integrated With the Boruta-Random Forest Algorithm
Jayasinghe, W. J. M. Lakmini Prarthana, Deo, Ravinesh C., Ghahramani, Afshin, Ghimire, Sujan and Raj, Nawin. 2021. "Deep Multi-Stage Reference Evapotranspiration Forecasting Model: Multivariate Empirical Mode Decomposition Integrated With the Boruta-Random Forest Algorithm." IEEE Access. 9, pp. 166695-166708. https://doi.org/10.1109/ACCESS.2021.3135362Designing Deep-based Learning Flood Forecast Model with ConvLSTM Hybrid Algorithm
Moishin, Mohammed, Deo, Ravinesh C., Prasad, Ramendra, Raj, Nawin and Abdulla, Shahab. 2021. "Designing Deep-based Learning Flood Forecast Model with ConvLSTM Hybrid Algorithm." IEEE Access. 9, pp. 50982-50993. https://doi.org/10.1109/ACCESS.2021.3065939Pattern recognition describing spatio-temporal drivers of catchment classification for water quality
O'Sullivan, Cherie M., Ghahramani, Afshin, Deo, Ravinesh C. and Pembleton, Keith G.. 2023. "Pattern recognition describing spatio-temporal drivers of catchment classification for water quality." Science of the Total Environment. 861, pp. 1-42. https://doi.org/10.1016/j.scitotenv.2022.160240The Playground Shade Index: A New Design Metric for Measuring Shade and Seasonal Ultraviolet Protection Characteristics of Parks and Playgrounds
Downs, Nathan, Raj, Nawin, Vanos, Jennifer, Parisi, Alfio, Butler, Harry, Deo, Ravinesh, Igoe, Damien, Dexter, Benjamin, Beckman-Downs, Melanie, Turner, Joanna and Dekeyser, Stijn. 2023. "The Playground Shade Index: A New Design Metric for Measuring Shade and Seasonal Ultraviolet Protection Characteristics of Parks and Playgrounds." Photochemistry and Photobiology. 99 (4), pp. 1193-1207. https://doi.org/10.1111/php.13745Using Sequence-to-Sequence Models for Carrier Frequency Offset Estimation of Short Messages and Chaotic Maps
Davey, Christopher P., Shakeel, Ismail, Deo, Ravinesh C., Salcedo-sanz, Sancho and Soar, Jeffrey. 2022. "Using Sequence-to-Sequence Models for Carrier Frequency Offset Estimation of Short Messages and Chaotic Maps." IEEE Access. 10, pp. 119814 - 119825. https://doi.org/10.1109/ACCESS.2022.3221762Modelling and Real-time Optimisation of Air Quality Predictions for Australia through Artificial Intelligence Algorithm
Sharma, Ekta, Deo, Ravinesh C., Prasad, Ramendra and Parisi, Alfio V.. 2019. "Modelling and Real-time Optimisation of Air Quality Predictions for Australia through Artificial Intelligence Algorithm." AMSI Optimise 2019. Perth, Australia 17 - 21 Jun 2019 Perth, Australia.Hybrid Convolutional Neural Network-Multilayer Perceptron Model for Solar Radiation Prediction
Ghimire, Sujan, Nguyen-Huy, Thong, Prasad, Ramendra, Deo, Ravinesh C., Casillas-Perez, David, Salcedo-sanz, Sancho and Bhandari, Binayak. 2023. "Hybrid Convolutional Neural Network-Multilayer Perceptron Model for Solar Radiation Prediction." Cognitive Computation. 15 (2), pp. 645-671. https://doi.org/10.1007/s12559-022-10070-yStudent Performance Predictions for Advanced Engineering Mathematics Course With New Multivariate Copula Models
Nguyen-Huy, Thong, Deo, Ravinesh C., Khan, Shahjahan, Devi, Aruna, Adeyinka, Adewuyi Ayodele, Apan, Armando A. and Yaseen, Zaher Mundher. 2022. "Student Performance Predictions for Advanced Engineering Mathematics Course With New Multivariate Copula Models." IEEE Access. 10, pp. 45112 -45136. https://doi.org/10.1109/ACCESS.2022.3168322Assay of renewable energy transition: A systematic literature review
Bhattarai, Utsav, Maraseni, Tek and Apan, Armando. 2022. "Assay of renewable energy transition: A systematic literature review." Science of the Total Environment. 833, pp. 1-18. https://doi.org/10.1016/j.scitotenv.2022.155159Rapid assessment of mine rehabilitation areas with airborne LiDAR and deep learning: bauxite strip mining in Queensland, Australia
Murray, Xavier, Apan, Armando, Deo, Ravinesh and Maraseni, Tek. 2022. "Rapid assessment of mine rehabilitation areas with airborne LiDAR and deep learning: bauxite strip mining in Queensland, Australia." Geocarto International. 37 (26), pp. 11223-11252. https://doi.org/10.1080/10106049.2022.2048902Spaceborne satellite remote sensing of tropical montane forests: a review of applications and future trends
Altarez, Richard Dein D., Apan, Armando and Maraseni, Tek. 2022. "Spaceborne satellite remote sensing of tropical montane forests: a review of applications and future trends." Geocarto International. 37 (26), pp. 11900-11928. https://doi.org/10.1080/10106049.2022.2060330Multi-strategy Slime Mould Algorithm for hydropower multi-reservoir systems optimization
Ahmadianfar, Iman, Noori, Ramzia Majeed, Togun, Hussein, Falah, Mayadah W., Homod, Raad Z., Fu, Minglei, Halder, Bijay, Deo, Ravinesh and Yaseen, Zaher Mundher. 2022. "Multi-strategy Slime Mould Algorithm for hydropower multi-reservoir systems optimization." Knowledge-Based Systems. 250, pp. 1-18. https://doi.org/10.1016/j.knosys.2022.109048Suspended sediment load modeling using advanced hybrid rotation forest based elastic network approach
Khosravi, Khabat, Golkarian, Ali, Melesse, Assefa M. and Deo, Ravinesh C.. 2022. "Suspended sediment load modeling using advanced hybrid rotation forest based elastic network approach." Journal of Hydrology. 610, pp. 1-14. https://doi.org/10.1016/j.jhydrol.2022.127963Delineating the Crop-Land Dynamic due to Extreme Environment Using Landsat Datasets: A Case Study
Halder, Bijay, Bandyopadhyay, Jatisankar, Afan, Haitham Abdulmohsin, Naser, Maryam H., Abed, Salwan Ali, Khedher, Khaled Mohamed, Falih, Khaldoon T., Deo, Ravinesh, Scholz, Miklas and Yaseen, Zaher Mundher. 2022. "Delineating the Crop-Land Dynamic due to Extreme Environment Using Landsat Datasets: A Case Study." Agronomy. 12 (6), pp. 1-23. https://doi.org/10.3390/agronomy12061268Coupled online sequential extreme learning machine model with ant colony optimization algorithm for wheat yield prediction
Ali, Mumtaz, Deo, Ravinesh C., Xiang, Yong, Prasad, Ramendra, Li, Jianxin, Farooque, Aitazaz and Yaseen, Zaher Mundher. 2022. "Coupled online sequential extreme learning machine model with ant colony optimization algorithm for wheat yield prediction." Scientific Reports. 12 (1), pp. 1-23. https://doi.org/10.1038/s41598-022-09482-5Forecasting solar photosynthetic photon flux density under cloud cover effects: novel predictive model using convolutional neural network integrated with long short-term memory network
Deo, Ravinesh C., Grant, Richard H., Webb, Ann, Ghimire, Sujan, Igoe, Damien P., Downs, Nathan J., Al-Musaylh, Mohanad S., Parisi, Alfio V. and Soar, Jeffrey. 2022. "Forecasting solar photosynthetic photon flux density under cloud cover effects: novel predictive model using convolutional neural network integrated with long short-term memory network." Stochastic Environmental Research and Risk Assessment. 36, p. 3183–3220. https://doi.org/10.1007/s00477-022-02188-0Improved Complete Ensemble Empirical Mode Decomposition with Adaptive Noise Deep Residual model for short-term multi-step solar radiation prediction
Ghimire, Sujan, Deo, Ravinesh C, Casillas-Perez, David and Salcedo-sanz, Sancho. 2022. "Improved Complete Ensemble Empirical Mode Decomposition with Adaptive Noise Deep Residual model for short-term multi-step solar radiation prediction." Renewable Energy. 190, pp. 408-424. https://doi.org/10.1016/j.renene.2022.03.120Efficient daily solar radiation prediction with deep learning 4-phase convolutional neural network, dual stage stacked regression and support vector machine CNN-REGST hybrid model
Ghimire, Sujan, Nguyen-Huy, Thong, Deo, Ravinesh C., Casillas-Perez, David and Salcedo-sanz, Sancho. 2022. "Efficient daily solar radiation prediction with deep learning 4-phase convolutional neural network, dual stage stacked regression and support vector machine CNN-REGST hybrid model." Sustainable Materials and Technologies. 32, pp. 1-24. https://doi.org/10.1016/j.susmat.2022.e00429Hybrid deep CNN-SVR algorithm for solar radiation prediction problems in Queensland, Australia
Ghimire, Sujan, Bhandari, Binayak, Casillas-Perez, David, Deo, Ravinesh C. and Salcedo-sanz, Sancho. 2022. "Hybrid deep CNN-SVR algorithm for solar radiation prediction problems in Queensland, Australia." Engineering Applications of Artificial Intelligence. 112, pp. 1-26. https://doi.org/10.1016/j.engappai.2022.104860Boosting solar radiation predictions with global climate models, observational predictors and hybrid deep-machine learning algorithms
Ghimire, Sujan, Deo, Ravinesh C., Casillas-Perez, David and Salcedo-sanz, Sancho. 2022. "Boosting solar radiation predictions with global climate models, observational predictors and hybrid deep-machine learning algorithms." Applied Energy. 316, pp. 1-25. https://doi.org/10.1016/j.apenergy.2022.119063Machine learning regression and classification methods for fog events prediction
Castillo-Boton, C., Casillas-Perez, D., Casanova-Mateo, C., Ghimire, S., Cerro-Prada, E., Gutierrez, P. A., Deo, R. C. and Salcedo-sanz, S.. 2022. "Machine learning regression and classification methods for fog events prediction." Atmospheric Research. 272, pp. 1-23. https://doi.org/10.1016/j.atmosres.2022.106157Quantum Artificial Intelligence Predictions Enhancement by Improving Signal Processing
Riaz, Farina, Abdulla, Shahab, Ni, Wei, Radfar, Mohsen, Deo, Ravinesh and Hopkins, Susan. 2022. "Quantum Artificial Intelligence Predictions Enhancement by Improving Signal Processing." Quantum Australia Conference 2022. Online 23 - 25 Feb 2022 Toowoomba, Australia. https://doi.org/10.13140/RG.2.2.34754.66245Detection of calcium, magnesium, and chlorophyll variations of wheat genotypes on sodic soils using hyperspectral red edge parameters
Choudhury, Malini Roy, Christopher, Jack, Das, Sumanta, Apan, Armando, Menzies, Neal W., Chapman, Scott, Mellor, Vincent and Dang, Yash P.. 2022. "Detection of calcium, magnesium, and chlorophyll variations of wheat genotypes on sodic soils using hyperspectral red edge parameters." Environmental Technology and Innovation. 27, pp. 1-14. https://doi.org/10.1016/j.eti.2022.102469Deep learning CNN-LSTM-MLP hybrid fusion model for feature optimizations and daily solar radiation prediction
Ghimire, Sujan, Deo, Ravinesh C., Casillas-Perez, David, Salcedo-sanz, Sancho, Sharma, Ekta and Ali, Mumtaz. 2022. "Deep learning CNN-LSTM-MLP hybrid fusion model for feature optimizations and daily solar radiation prediction." Measurement. 202, pp. 1-22. https://doi.org/10.1016/j.measurement.2022.111759Basin management inspiration from impacts of alternating dry and wet conditions on water production and carbon uptake in Murray-Darling Basin
Lu, Zhixiang, Feng, Qi, Wei, Yongping, Zhao, Yan, Deo, Ravinesh C., Xie, Jiali, Zhou, Sha, Zhu, Meng and Xu, Min. 2022. "Basin management inspiration from impacts of alternating dry and wet conditions on water production and carbon uptake in Murray-Darling Basin." Science of the Total Environment. 851 (Part 2), pp. 1-8. https://doi.org/10.1016/j.scitotenv.2022.158359Introductory Engineering Mathematics Students’ Weighted Score Predictions Utilising a Novel Multivariate Adaptive Regression Spline Model
Ahmed, Abul Abrar Masrur, Deo, Ravinesh C., Ghimire, Sujan, Downs, Nathan J., Devi, Aruna, Barua, Prabal D. and Yaseen, Zaher M.. 2022. "Introductory Engineering Mathematics Students’ Weighted Score Predictions Utilising a Novel Multivariate Adaptive Regression Spline Model." Sustainability. 14 (17), pp. 1-27. https://doi.org/10.3390/su141711070Kernel Ridge Regression Hybrid Method for Wheat Yield Prediction with Satellite-Derived Predictors
Ahmed, A. A. Masrur, Sharma, Ekta, Jui, S. Janifer Jabin, Deo, Ravinesh C., Nguyen-Huy, Thong and Ali, Mumtaz. 2022. "Kernel Ridge Regression Hybrid Method for Wheat Yield Prediction with Satellite-Derived Predictors." Remote Sensing. 14 (5), pp. 1-24. https://doi.org/10.3390/rs14051136Cloud Affected Solar UV Predictions with Three-Phase Wavelet Hybrid Convolutional Long Short-Term Memory Network Multi-Step Forecast System
Prasad, Salvin S., Deo, Ravinesh C., Downs, Nathan, Igoe, Damien, Parisi, Alfio V. and Soar, Jeffrey. 2022. "Cloud Affected Solar UV Predictions with Three-Phase Wavelet Hybrid Convolutional Long Short-Term Memory Network Multi-Step Forecast System." IEEE Access. 10, pp. 24704-24720. https://doi.org/10.1109/ACCESS.2022.3153475Development and evaluation of hybrid deep learning long short-term memory network model for pan evaporation estimation trained with satellite and ground-based data
Jayasinghe, W. J. M. Lakmini Prarthana, Deo, Ravinesh C., Ghahramani, Afshin, Ghimire, Sujan and Raj, Nawin. 2022. "Development and evaluation of hybrid deep learning long short-term memory network model for pan evaporation estimation trained with satellite and ground-based data." Journal of Hydrology. 607, pp. 1-19. https://doi.org/10.1016/j.jhydrol.2022.127534Integrative artificial intelligence models for Australian coastal sediment lead prediction: An investigation of in-situ measurements and meteorological parameters effects
Bhagat, Suraj Kumar, Tiyasha, Tiyasha, Kumar, Adarsh, Malik, Tabarak, Jawad, Ali H., Khedher, Khaled Mohamed, Deo, Ravinesh C. and Yaseen, Zaher Mundher. 2022. "Integrative artificial intelligence models for Australian coastal sediment lead prediction: An investigation of in-situ measurements and meteorological parameters effects." Journal of Environmental Management. 309, pp. 1-16. https://doi.org/10.1016/j.jenvman.2022.114711An Eigenvalues-Based Covariance Matrix Bootstrap Model Integrated With Support Vector Machines for Multichannel EEG Signals Analysis
Al-Hadeethi, Hanan, Abdulla, Shahab, Diykh, Mohammed, Deo, Ravinesh C. and Green, Jonathan H.. 2022. "An Eigenvalues-Based Covariance Matrix Bootstrap Model Integrated With Support Vector Machines for Multichannel EEG Signals Analysis." Frontiers in Neuroinformatics. 15, pp. 1-15. https://doi.org/10.3389/fninf.2021.808339Stacked LSTM Sequence-to-Sequence Autoencoder with Feature Selection for Daily Solar Radiation Prediction: A Review and New Modeling Results
Ghimire, Sujan, Deo, Ravinesh C., Wang, Hua, Al-Musaylh, Mohanad S., Casillas-Perez, David and Salcedo-sanz, Sancho. 2022. "Stacked LSTM Sequence-to-Sequence Autoencoder with Feature Selection for Daily Solar Radiation Prediction: A Review and New Modeling Results." Energies. 15 (3), pp. 1-39. https://doi.org/10.3390/en15031061Texture analysis based graph approach for automatic detection of neonatal seizure from multi-channel EEG signals
Diykh, Mohammed, Miften, Firas Sabar, Abdulla, Shahab, Deo, Ravinesh C., Siuly, Siuly, Green, Jonathan H. and Oudah, Atheer Y.. 2022. "Texture analysis based graph approach for automatic detection of neonatal seizure from multi-channel EEG signals." Measurement. 190 (110731), pp. 1-13. https://doi.org/10.1016/j.measurement.2022.110731Identifying and prioritising climate change adaptation actions for greater one-horned rhinoceros (Rhinoceros unicornis) conservation in Nepal
Pant, Ganesh, Maraseni, Tek, Apan, Armando and Allen, Benjamin L.. 2022. "Identifying and prioritising climate change adaptation actions for greater one-horned rhinoceros (Rhinoceros unicornis) conservation in Nepal." PEERJ. 10. https://doi.org/10.7717/peerj.12795Classification of catchments for nitrogen using Artificial Neural Network Pattern Recognition and spatial data
O'Sullivan, Cherie M., Ghahramani, Afshin, Deo, Ravinesh C., Pembleton, Keith, Khan, Urooj and Tuteja, Narendra. 2022. "Classification of catchments for nitrogen using Artificial Neural Network Pattern Recognition and spatial data." Science of the Total Environment. 809, pp. 1-15. https://doi.org/10.1016/j.scitotenv.2021.151139How much do we know about trade-offs in ecosystem services? A systematic review of empirical research observations
Aryal, Kishor, Maraseni, Tek N. and Apan, Armando. 2022. "How much do we know about trade-offs in ecosystem services? A systematic review of empirical research observations." Science of the Total Environment. 806, pp. 1-13. https://doi.org/10.1016/j.scitotenv.2021.151229Domino effect of climate change over two millennia in ancient China’s Hexi Corridor
Feng, Qi, Yan, Linshan, Deo, Ravinesh C., AghaKouchak, Amir, Adamowski, Jan F., Stone, Roger, Yin, Zhenliang, Liu, Wei, Si, Jianhua, Wen, Xiaohu, Zhu, Meng and Cao, Shixiong. 2019. "Domino effect of climate change over two millennia in ancient China’s Hexi Corridor." Nature Sustainability. 2, pp. 957-961. https://doi.org/10.1038/s41893-019-0397-9Evaluation of drought tolerance of wheat genotypes in rain-fed sodic soil environments using high-resolution UAV remote sensing techniques
Das, Sumanta, Christopher, Jack, Choudhury, Malini Roy, Apan, Armando, Chapman, Scott, Menzies, Neal W. and Dang, Yash P.. 2022. "Evaluation of drought tolerance of wheat genotypes in rain-fed sodic soil environments using high-resolution UAV remote sensing techniques." Biosystems Engineering. 217, pp. 68-82. https://doi.org/10.1016/j.biosystemseng.2022.03.004Bat algorithm for dam–reservoir operation
Ethteram, Mohammad, Mousavi, Sayed-Farhad, Karami, Hojat, Farzin, Saeed, Deo, Ravinesh, Othman, Faridah Binti, Chau, Kwok-Wing, Sarkamaryan, Saeed, Singh, Vijay P. and El-Shafie, Ahmed. 2018. "Bat algorithm for dam–reservoir operation." Environmental Earth Sciences. 77 (13), pp. 1-15. https://doi.org/10.1007/s12665-018-7662-5Characteristics of ecosystem water use efficiency in a desert riparian forest
Ma, Xiaohong, Feng, Qi, Su, Yonghong, Yu, Tengfei and Deo, Ravinesh C.. 2018. "Characteristics of ecosystem water use efficiency in a desert riparian forest." Environmental Earth Sciences. 77 (358). https://doi.org/10.1007/s12665-018-7518-zThe influence of climatic inputs on stream-flow pattern forecasting: case study of Upper Senegal River
Diop, Lamine, Bodian, Ansoumana, Djaman, Koffi, Yaseen, Zaher Mundher, Deo, Ravinesh C., El-Shafie, Ahmed and Brown, Larry C.. 2018. "The influence of climatic inputs on stream-flow pattern forecasting: case study of Upper Senegal River." Environmental Earth Sciences. 77 (5). https://doi.org/10.1007/s1266Uncertainty assessment of the multilayer perceptron (MLP) neural network model with implementation of the novel hybrid MLP-FFA method for prediction of biochemical oxygen demand and dissolved oxygen: a case study of Langat River
Raheli, Bahare, Aalami, Mohammad Taghi, El-Shafie, Ahmed, Ghorbani, Mohammad Ali and Deo, Ravinesh C.. 2017. "Uncertainty assessment of the multilayer perceptron (MLP) neural network model with implementation of the novel hybrid MLP-FFA method for prediction of biochemical oxygen demand and dissolved oxygen: a case study of Langat River." Environmental Earth Sciences. 76 (14). https://doi.org/10.1007/s12665-017-6842-zNovel hybrid deep learning model for satellite based PM10 forecasting in the most polluted Australian hotspots
Sharma, Ekta, Deo, Ravinesh C., Soar, Jeffrey, Prasad, Ramendra, Parisi, Alfio V. and Raj, Nawin. 2022. "Novel hybrid deep learning model for satellite based PM10 forecasting in the most polluted Australian hotspots." Atmospheric Environment. 279, pp. 1-13. https://doi.org/10.1016/j.atmosenv.2022.119111New double decomposition deep learning methods for river water level forecasting
Ahmed, A. A. Masrur, Deo, Ravinesh C., Ghahramani, Afshin, Feng, Qi, Raj, Nawin, Yin, Zhenliang and Yang, Linshan. 2022. "New double decomposition deep learning methods for river water level forecasting." Science of the Total Environment. 831, pp. 1-21. https://doi.org/10.1016/j.scitotenv.2022.154722Predicted declines in suitable habitat for greater one-horned rhinoceros (Rhinoceros unicornis) under future climate and land use change scenarios
Pant, Ganesh, Maraseni, Tek, Apan, Armando and Allen, Benjamin L.. 2021. "Predicted declines in suitable habitat for greater one-horned rhinoceros (Rhinoceros unicornis) under future climate and land use change scenarios." Ecology and Evolution. 11 (24), pp. 18288-18304. https://doi.org/10.1002/ece3.8421Application of Sentinel-2 Satellite Data to Map Forest Cover in Southeast Sri Lanka through the Random Forest Classifier
Gunawansa, Thakshila, Perera, Kithsiri, Apan, Armando and Hettiarachchi, Nandita. 2022. "Application of Sentinel-2 Satellite Data to Map Forest Cover in Southeast Sri Lanka through the Random Forest Classifier." Journal of Advances in Engineering and Technology. I (I), pp. 049-1 to 049-10.A hierarchical classification/regression algorithm for improving extreme wind speed events prediction
Pelaez-Rodriguez, C., Perez-Aracil, J., Fister, D, Prieto-Godino, L., Deo, R.C. and Salcedo-sanz, S.. 2022. "A hierarchical classification/regression algorithm for improving extreme wind speed events prediction." Renewable Energy. 201 (Part 2), pp. 157-178. https://doi.org/10.1016/j.renene.2022.11.042Modelling Floodplain Vegetation Response to Groundwater Variability Using the ArcSWAT Hydrological Model, MODIS NDVI Data, and Machine Learning
Muhury, Newton, Apan, Armando A., Maraseni, Tek N. and Ayele, Gebiaw T.. 2022. "Modelling Floodplain Vegetation Response to Groundwater Variability Using the ArcSWAT Hydrological Model, MODIS NDVI Data, and Machine Learning." Land. 11 (12), pp. 1-23. https://doi.org/10.3390/land11122154Mapping Prominent Cash Crops Employing ALOS PALSAR-2 and Selected Machine Learners
Panuju, Dyah R., Haerani, H., Apan, Armando, Griffin, Amy L., Paull, David J. and Trisasongko, Bambang Hendro. 2022. "Mapping Prominent Cash Crops Employing ALOS PALSAR-2 and Selected Machine Learners." Kumar, Arvind, Kumar, Pavan, Singh, S. S., Trisasongko, Bambang Hendro and Rani, Meenu (ed.) Agriculture, Livestock Production and Aquaculture: Advances for Smallholder Farming Systems. Cham, Switzerland. Springer. pp. 131-146Wind speed forecasting in Nepal using self-organizing map-based online sequential extreme learning machine
Sharma, Neelesh and Deo, Ravinesh. 2021. "Wind speed forecasting in Nepal using self-organizing map-based online sequential extreme learning machine." Deo, Ravinesh, Samui, Pijush and Roy, Sanjiban Sekhar (ed.) Predictive Modelling for Energy Management and Power Systems Engineering. Netherlands. Elsevier. pp. 437-484Support vector machine model for multistep wind speed forecasting
Prasad, Shobna Mohini Mala, Nguyen-Huy, Thong and Deo, Ravinesh. 2021. "Support vector machine model for multistep wind speed forecasting." Deo, Ravinesh, Samui, Pijush and Roy, Sanjiban Sekhar (ed.) Predictive modelling for energy management and power systems engineering. Netherlands. Elsevier. pp. 335-389Development of data-driven models for wind speed forecasting in Australia
Neupane, Ananta, Raj, Nawin, Deo, Ravinesh and Ali, Mumtaz. 2021. "Development of data-driven models for wind speed forecasting in Australia." Deo, R., Samui, Pijush and Roy, Sanjiban Sekhar (ed.) Predictive modelling for energy management and power systems engineering. Netherlands. Elsevier. pp. 143-190Improving Biomass and Grain Yield Prediction of Wheat Genotypes on Sodic Soil Using Integrated High-Resolution Multispectral, Hyperspectral, 3D Point Cloud, and Machine Learning Techniques
Choudhury, Malini Roy, Das, Sumanta, Christopher, Jack, Apan, Armando, Chapman, Scott, Menzies, Neal W. and Dang, Yash P.. 2021. "Improving Biomass and Grain Yield Prediction of Wheat Genotypes on Sodic Soil Using Integrated High-Resolution Multispectral, Hyperspectral, 3D Point Cloud, and Machine Learning Techniques." Remote Sensing. 13 (17), pp. 1-27. https://doi.org/10.3390/rs13173482Application of deep learning models for automated identification of Parkinson’s disease: a review (2011–2021)
Loh, Hui Wen, Hong, Wanrong, Ooi, Chui Ping, Chakraborty, Subrata, Barua, Prabal Datta, Deo, Ravinesh C., Soar, Jeffrey, Palmer, Elizabeth E. and Acharya, U. Rajendra. 2021. "Application of deep learning models for automated identification of Parkinson’s disease: a review (2011–2021)." Sensors. 21, pp. 1-27. https://doi.org/10.3390/s21217034Artificial intelligence models for suspended river sediment prediction: state-of-the art, modeling framework appraisal, and proposed future research directions
Tao, Hai, Al-Khafaji, Zainab S., Qi, Chongchong, Zounemat-Kermani, Mohammad, Kisi, Ozgur, Tiyasha, Tiyasha, Chau, Kwok-Wing, Nourani, Vahid, Melesse, Assefa M., Elhakeem, Mohamed, Farooque, Aitazaz Ahsan, Nejadhashemi, A. Pouyan, Khedher, Khaled Mohamed, Alawi, Omer A., Deo, Ravinesh C., Shahid, Shamsuddin, Singh, Vijay P. and Yaseen, Zaher Mundher. 2021. "Artificial intelligence models for suspended river sediment prediction: state-of-the art, modeling framework appraisal, and proposed future research directions." Engineering Applications of Computational Fluid Mechanics. 15 (1), pp. 1585-1612. https://doi.org/10.1080/19942060.2021.1984992UAV-thermal imaging: A technological breakthrough for monitoring and quantifying crop abiotic stress to help sustain productivity on sodic soils – A case review on wheat
Das, Sumanta, Chapman, Scott, Christopher, Jack, Choudhury, Malini Roy, Menzies, Neal W., Apan, Armando and Dang, Yash P.. 2021. "UAV-thermal imaging: A technological breakthrough for monitoring and quantifying crop abiotic stress to help sustain productivity on sodic soils – A case review on wheat." Remote Sensing Applications: Society and Environment. 23, pp. 1-13. https://doi.org/10.1016/j.rsase.2021.100583Improving estimation of in-season crop water use and health of wheat genotypes on sodic soils using spatial interpolation techniques and multi-component metrics
Choudhury, Malini Roy, Mellor, Vincent, Das, Sumanta, Christopher, Jack, Apan, Armando, Menzies, Neal W., Chapman, Scott and Dang, Yash P.. 2021. "Improving estimation of in-season crop water use and health of wheat genotypes on sodic soils using spatial interpolation techniques and multi-component metrics." Agricultural Water Management. 255. https://doi.org/10.1016/j.agwat.2021.107007Evaluation of water status of wheat genotypes to aid prediction of yield on sodic soils using UAV-thermal imaging and machine learning
Das, Sumanta, Christopher, Jack, Apan, Armando, Choudhury, Malini Roy, Chapman, Scott, Menzies, Neal W. and Dang, Yash P.. 2021. "Evaluation of water status of wheat genotypes to aid prediction of yield on sodic soils using UAV-thermal imaging and machine learning." Agricultural and Forest Meteorology. 307. https://doi.org/10.1016/j.agrformet.2021.108477UAV-thermal imaging and agglomerative hierarchical clustering techniques to evaluate and rank physiological performance of wheat genotypes on sodic soil
Das, Sumanta, Christopher, Jack, Apan, Armando, Choudhury, Malini Roy, Chapman, Scott, Menzies, Neal W. and Dang, Yash P.. 2021. "UAV-thermal imaging and agglomerative hierarchical clustering techniques to evaluate and rank physiological performance of wheat genotypes on sodic soil." ISPRS Journal of Photogrammetry and Remote Sensing. 173, pp. 221-237. https://doi.org/10.1016/j.isprsjprs.2021.01.014Advanced extreme learning machines vs. deep learning models for peak wave energy period forecasting: A case study in Queensland, Australia
Ali, Mumtaz, Prasad, Ramendra, Xiang, Yong, Sankaran, Adarsh, Deo, Ravinesh C., Xiao, Fuyuan and Zhu, Shuyu. 2021. "Advanced extreme learning machines vs. deep learning models for peak wave energy period forecasting: A case study in Queensland, Australia." Renewable Energy. 177, pp. 1033-1044. https://doi.org/10.1016/j.renene.2021.06.052Novel short-term solar radiation hybrid model: long short-term memory network integrated with robust local mean decomposition
Huynh, Anh Ngoc-Lan, Deo, Ravinesh C., Ali, Mumtaz, Abdulla, Shahab and Raj, Nawin. 2021. "Novel short-term solar radiation hybrid model: long short-term memory network integrated with robust local mean decomposition." Applied Energy. 298, pp. 1-19. https://doi.org/10.1016/j.apenergy.2021.117193Evaluating management strategies for sustainable crop production under changing climate conditions: a system dynamics approach
Pham, Yen Hoang, Reardon-Smith, Kathryn and Deo, Ravinesh C.. 2021. "Evaluating management strategies for sustainable crop production under changing climate conditions: a system dynamics approach." Journal of Environmental Management. 292. https://doi.org/10.1016/j.jenvman.2021.112790An interplay of soil salinization and groundwater degradation threatening coexistence of oasis-desert ecosystems
Yin, Xinwei, Feng, Qi, Li, Yan, Deo, Ravinesh C., Liu, Wei, Zhu, Meng, Zheng, Xinjun and Liu, Ran. 2022. "An interplay of soil salinization and groundwater degradation threatening coexistence of oasis-desert ecosystems." Science of the Total Environment. 806 (2), pp. 1-20. https://doi.org/10.1016/j.scitotenv.2021.150599Hybrid deep learning method for a week-ahead evapotranspiration forecasting
Ahmed, A. A. Masrur, Deo, Ravinesh C., Feng, Qi, Ghahramani, Afshin, Raj, Nawin, Yin, Zhenliang and Yang, Linshan. 2022. "Hybrid deep learning method for a week-ahead evapotranspiration forecasting." Stochastic Environmental Research and Risk Assessment. 36 (3), pp. 831-849. https://doi.org/10.1007/s00477-021-02078-xStreamflow prediction using an integrated methodology based on convolutional neural network and long short‑term memory networks
Ghimire, Sujan, Yaseen, Zaher Mundher, Farooque, Aitazaz A., Deo, Ravinesh C., Zhang, Ji and Tao, Xiaohui. 2021. "Streamflow prediction using an integrated methodology based on convolutional neural network and long short‑term memory networks." Scientific Reports. 11, pp. 1-26. https://doi.org/10.1038/s41598-021-96751-4The role of internal transcribed spacer 2 secondary structures in classifying mycoparasitic Ampelomyces
Prahl, Rosa E., Khan, Shahjahan and Deo, Ravinesh C.. 2021. "The role of internal transcribed spacer 2 secondary structures in classifying mycoparasitic Ampelomyces." PLoS One. 16 (6), pp. 1-28. https://doi.org/10.1371/journal.pone.0253772Deep Learning Forecasts of Soil Moisture: Convolutional Neural Network and Gated Recurrent Unit Models Coupled with Satellite-Derived MODIS, Observations and Synoptic-Scale Climate Index Data
Ahmed, A. A. Masrur, Deo, Ravinesh C., Raj, Nawin, Ghahramani, Afshin, Feng, Qi, Yin, Zhenliang and Yang, Linshan. 2021. "Deep Learning Forecasts of Soil Moisture: Convolutional Neural Network and Gated Recurrent Unit Models Coupled with Satellite-Derived MODIS, Observations and Synoptic-Scale Climate Index Data." Remote Sensing. 13 (4), pp. 1-30. https://doi.org/10.3390/rs13040554LSTM integrated with Boruta-random forest optimiser for soil moisture estimation under RCP4.5 and RCP8.5 global warming scenarios
Ahmed, A. A. Masrur, Deo, Ravinesh C., Ghahramani, Afshin, Raj, Nawin, Feng, Qi, Yin, Zhenliang and Yang, Linshan. 2021. "LSTM integrated with Boruta-random forest optimiser for soil moisture estimation under RCP4.5 and RCP8.5 global warming scenarios." Stochastic Environmental Research and Risk Assessment. 35, pp. 1851-1881. https://doi.org/10.1007/s00477-021-01969-3A new framework for classification of multi-category hand grasps using EMG signals
Miften, Firas Sabar, Diykh, Mohammed, Abdulla, Shahab, Siuly, Siuly, Green, Jonathan H. and Deo, Ravinesh C.. 2021. "A new framework for classification of multi-category hand grasps using EMG signals." Artificial Intelligence in Medicine. 112, pp. 1-14. https://doi.org/10.1016/j.artmed.2020.102005Deep learning hybrid model with Boruta-Random forest optimiser algorithm for streamflow forecasting with climate mode indices, rainfall, and periodicity
Ahmed, A. A. Masrur, Deo, Ravinesh C., Feng, Qi, Ghahramani, Afshin, Raj, Nawin, Yin, Zhenliang and Yang, Linshan. 2021. "Deep learning hybrid model with Boruta-Random forest optimiser algorithm for streamflow forecasting with climate mode indices, rainfall, and periodicity." Journal of Hydrology. 599, pp. 1-23. https://doi.org/10.1016/j.jhydrol.2021.126350Modeling soil temperature using air temperature features in diverse climatic conditions with complementary machine learning models
Bayatvarkeshi, Maryam, Bhagat, Suraj Kumar, Mohammadi, Kourosh, Kisi, Ozgur, Farahani, M., Hasani, A., Deo, Ravinesh and Yaseen, Zaher Mundher. 2021. "Modeling soil temperature using air temperature features in diverse climatic conditions with complementary machine learning models." Computers and Electronics in Agriculture. 185. https://doi.org/10.1016/j.compag.2021.106158Mapping rice area and yield in northeastern asia by incorporating a crop model with dense vegetation index profiles from a geostationary satellite
Yeom, Jong-Min, Jeong, Seungtaek, Deo, Ravinesh C. and Ko, Jonghan. 2021. "Mapping rice area and yield in northeastern asia by incorporating a crop model with dense vegetation index profiles from a geostationary satellite." GIScience and Remote Sensing. https://doi.org/10.1080/15481603.2020.1853352Deep Air Quality Forecasts: Suspended Particulate Matter Modeling With Convolutional Neural and Long Short-Term Memory Networks
Sharma, Ekta, Deo, Ravinesh C., Prasad, Ramendra, Parisi, Alfio and Raj, Nawin. 2020. "Deep Air Quality Forecasts: Suspended Particulate Matter Modeling With Convolutional Neural and Long Short-Term Memory Networks." IEEE Access. 8, pp. 209503-209516. https://doi.org/10.1109/ACCESS.2020.3039002MARS model for prediction of short- and long-term global solar radiation
Balalla, Dilki T., Nguyen-Huy, Thong and Deo, Ravinesh. 2021. "MARS model for prediction of short- and long-term global solar radiation." Deo, Ravinesh, Roy, Sanjiban Sekhar and Samui, Pijush (ed.) Predictive Modelling for Energy Management and Power Systems Engineering. United Kingdom. Elsevier. pp. 391-436Developing reservoir evaporation predictive model for successful dam management
Allawi, Mohammed Falah, Ahmed, Mohammed Lateef, Aidan, Ibraheem Abdallah, Deo, Ravinesh C. and El-Shafie, Ahmed. 2021. "Developing reservoir evaporation predictive model for successful dam management." Stochastic Environmental Research and Risk Assessment. 35 (2), pp. 499-514. https://doi.org/10.1007/s00477-020-01918-6Application of effective drought index for quantification of meteorological drought events: a case study in Australia
Deo, Ravinesh C., Byun, Hi-Ryong, Adamowski, Jan F. and Begum, Khaleda. 2017. "Application of effective drought index for quantification of meteorological drought events: a case study in Australia." Theoretical and Applied Climatology. 128 (1-2), pp. 359-379. https://doi.org/10.1007/s00704-015-1706-5Feedback modelling of the impacts of drought: A case study in coffee production systems in Viet Nam
Pham, Yen, Reardon-Smith, Kathryn, Mushtaq, Shahbaz and Deo, Ravinesh C.. 2020. "Feedback modelling of the impacts of drought: A case study in coffee production systems in Viet Nam." Climate Risk Management. 30, pp. 1-17. https://doi.org/10.1016/j.crm.2020.100255Forecasting long-term precipitation for water resource management: a new multi-step data-intelligent modelling approach
Ali, Mumtaz, Deo, Ravinesh C., Xiang, Yong, Li, Ya and Yaseen, Zaher Mundher. 2020. "Forecasting long-term precipitation for water resource management: a new multi-step data-intelligent modelling approach." Hydrological Sciences Journal. 65 (16), pp. 2693-2708. https://doi.org/10.1080/02626667.2020.1808219Characterizing net primary productivity of forests and non-forest areas in the Philippines using satellite imagery
Apan, Armando and Maraseni, Tek. 2012. "Characterizing net primary productivity of forests and non-forest areas in the Philippines using satellite imagery." 1st National Symposium and Workshop on Environmental Science (IESM 2012): Environmental Science in a Changing Environment. Manila, Philippines 07 - 08 May 2012 Manila, Philippines.Climate change vulnerability of Asia’s most iconic megaherbivore: greater one-horned rhinoceros (Rhinoceros unicornis)
Pant, Ganesh, Maraseni, Tek, Apan, Armando and Allen, Benjamin L.. 2020. "Climate change vulnerability of Asia’s most iconic megaherbivore: greater one-horned rhinoceros (Rhinoceros unicornis)." Global Ecology and Conservation. 23, pp. 1-14. https://doi.org/10.1016/j.gecco.2020.e01180Development of Flood Monitoring Index for daily flood risk evaluation: case studies in Fiji
Moishin, Mohammed, Deo, Ravinesh C., Prasad, Ramendra, Raj, Nawin and Abdulla, Shahab. 2021. "Development of Flood Monitoring Index for daily flood risk evaluation: case studies in Fiji." Stochastic Environmental Research and Risk Assessment. 35 (7), pp. 1387-1402. https://doi.org/10.1007/s00477-020-01899-6The modeling of human facial pain intensity based on Temporal Convolutional Networks trained with video frames in HSV color space
Bargshady, Ghazal, Zhou, Xujuan, Deo, Ravinesh C., Soar, Jeffrey, Whittaker, Frank and Wang, Hua. 2020. "The modeling of human facial pain intensity based on Temporal Convolutional Networks trained with video frames in HSV color space." Applied Soft Computing. 97 (Part A), pp. 1-14. https://doi.org/10.1016/j.asoc.2020.106805The climate-induced alteration of future geographic distribution of aflatoxin in peanut crops and its adaptation options
Haerani, Haerani, Apan, Armando and Basnet, Badri. 2020. "The climate-induced alteration of future geographic distribution of aflatoxin in peanut crops and its adaptation options." Mitigation and Adaptation Strategies for Global Change. 25 (6), pp. 1149-1175. https://doi.org/10.1007/s11027-020-09927-0Using a Mixed Method Research to Develop Spatial Information Sharing Strategies Across Natural Resource Management Communities
Paudyal, Dev Raj, McDougall, Kevin and Apan, Armando. 2019. "Using a Mixed Method Research to Develop Spatial Information Sharing Strategies Across Natural Resource Management Communities." Inaugural Australasia & Pacific Regional Mixed Methods International Research Association Conference 2019: Expanding Conceptual and Methodological Boundaries (MMIRA 2019). Wellington, New Zealand 04 - 06 Dec 2019 Indianapolis, United States.Modeling wheat yield with data-intelligent algorithms: artificial neural network versus genetic programming and minimax probability machine regression
Ali, Mumtaz and Deo, Ravinesh C.. 2020. "Modeling wheat yield with data-intelligent algorithms: artificial neural network versus genetic programming and minimax probability machine regression." Samui, Pijush, Bui, Dieu Tien, Chakraborty, Subrata and Deo, Ravinesh C. (ed.) Handbook of probabilistic models. Oxford, United Kingdom. Elsevier. pp. 37-87MARS model for prediction of short- and long-term global solar radiation
Balalla, Dilki T., Nguyen-Huy, Thong and Deo, Ravinesh. 2021. "MARS model for prediction of short- and long-term global solar radiation." Deo, Ravinesh, Samui, Pijush and Roy, Sanjiban Sekhar (ed.) Predictive modelling for energy management and power systems engineering. Amsterdam, Netherlands. Elsevier. pp. 391-436Short-term electrical energy demand prediction under heat island effects using emotional neural network integrated with genetic algorithm
Karalasingham, Sagthitharan, Deo, Ravinesh and Prasad, Ramendra. 2021. "Short-term electrical energy demand prediction under heat island effects using emotional neural network integrated with genetic algorithm." Deo, Ravinesh, Samui, Pijush and Roy, Sanjiban Sekhar (ed.) Predictive modelling for energy management and power systems engineering. Amsterdam, Netherlands. Elsevier. pp. 271-298Hybrid multilayer perceptron-firefly optimizer algorithm for modelling photosynthetic active solar radiation for biofuel energy exploration
Goundar, Harshna, Yaseen, Zaher Mundher and Deo, Ravinesh. 2021. "Hybrid multilayer perceptron-firefly optimizer algorithm for modelling photosynthetic active solar radiation for biofuel energy exploration." Deo, Ravinesh, Samui, Pijush and Roy, Sanjiban Sekhar (ed.) Predictive modelling for energy management and power systems engineering. Amsterdam, Netherlands. Elsevier. pp. 191-232Design and performance of two decomposition paradigms in forecasting daily solar radiation with evolutionary polynomial regression: wavelet transform versus ensemble empirical mode decomposition
Rezaie-Balf, Mohammad, Kim, Sungwon, Ghaemi, Alireza and Deo, Ravinesh. 2021. "Design and performance of two decomposition paradigms in forecasting daily solar radiation with evolutionary polynomial regression: wavelet transform versus ensemble empirical mode decomposition." Deo, Ravinesh, Samui, Pijush and Roy, Sanjiban Sekhar (ed.) Predictive modelling for energy management and power systems engineering. Amsterdam, Netherlands. Elsevier. pp. 115-142Experimental Study on the Rainfall-Runoff Responses of Typical Urban Surfaces and Two Green Infrastructures Using Scale-Based Models
Liu, Wen, Feng, Qi, Deo, Ravinesh C., Yao, Lei and Wei, Wei. 2020. "Experimental Study on the Rainfall-Runoff Responses of Typical Urban Surfaces and Two Green Infrastructures Using Scale-Based Models ." Environmental Management (New York): an international journal for decision-makers, scientists and environmental auditors. 66 (4), pp. 683-693. https://doi.org/10.1007/s00267-020-01339-9Ensemble neural network approach detecting pain intensity from facial expressions
Bargshady, Ghazal, Zhou, Xujuan, Deo, Ravinesh C., Soar, Jeffrey, Whittaker, Frank and Wang, Hua. 2020. "Ensemble neural network approach detecting pain intensity from facial expressions." Artificial Intelligence in Medicine. 109, pp. 1-12. https://doi.org/10.1016/j.artmed.2020.101954Daily flood forecasts with intelligent data analytic models: multivariate empirical mode decomposition-based modeling methods
Prasad, Ramendra, Charan, Dhrishna, Joseph, Lionel, Nguyen-Huy, Thong, Deo, Ravinesh C. and Singh, Sanjay. 2021. "Daily flood forecasts with intelligent data analytic models: multivariate empirical mode decomposition-based modeling methods." Deo, Ravinesh C., Samui, Pijush, Kisi, Ozgur and Yaseen, Zaher Mundher (ed.) Intelligent data analytics for decision-support systems in hazard mitigation: theory and practice of hazard mitigation. Singapore. Springer. pp. 359-381Artificial neural networks for prediction of Steadman Heat Index
Chand, Bhuwan, Nguyen-Huy, Thong and Deo, Ravinesh C.. 2021. "Artificial neural networks for prediction of Steadman Heat Index." Deo, Ravinesh C., Samui, Pijush, Kisi, Ozgur and Yaseen, Zaher Mundher (ed.) Intelligent data analytics for decision-support systems in hazard mitigation: theory and practice of hazard mitigation. Singapore. Springer. pp. 293-357Spatial prediction of landslide susceptibility using random forest algorithm
Rahmati, Omid, Kornejady, Aiding and Deo, Ravinesh C.. 2021. "Spatial prediction of landslide susceptibility using random forest algorithm." Deo, Ravinesh C., Samui, Pijush, Kisi, Ozgur and Yaseen, Zaher Mundher (ed.) Intelligent data analytics for decision-support systems in hazard mitigation: theory and practice of hazard mitigation. Singapore. Springer. pp. 281-292Intelligent data analytics for time series, trend analysis and drought indices comparison
Dayal, Kavina S., Deo, Ravinesh C. and Apan, Armando A.. 2021. "Intelligent data analytics for time series, trend analysis and drought indices comparison." Deo, Ravinesh C., Samui, Pijush, Kisi, Ozgur and Yaseen, Zaher Mundher (ed.) Intelligent data analytics for decision-support systems in hazard mitigation: theory and practice of hazard mitigation. Singapore. Springer. pp. 151-169Modulation of tropical cyclone genesis by Madden–Julian Oscillation in the Southern Hemisphere
Dayal, Kavina S., Wang, Bin and Deo, Ravinesh C.. 2021. "Modulation of tropical cyclone genesis by Madden–Julian Oscillation in the Southern Hemisphere." Deo, Ravinesh C., Samui, Pijush, Kisi, Ozgur and Yaseen, Zaher Mundher (ed.) Intelligent data analytics for decision-support systems in hazard mitigation: theory and practice of hazard mitigation. Singapore. Springer. pp. 127-150Bayesian Markov Chain Monte Carlo-based copulas: factoring the role of large-scale climate indices in monthly flood prediction
Nguyen-Huy, Thong, Deo, Ravinesh C., Yaseen, Zaher Mundher, Mushtaq, Shahbaz and Prasad, Ramendra. 2021. "Bayesian Markov Chain Monte Carlo-based copulas: factoring the role of large-scale climate indices in monthly flood prediction." Deo, Ravinesh C., Samui, Pijush, Kisi, Ozgur and Yaseen, Zaher Mundher (ed.) Intelligent data analytics for decision-support systems in hazard mitigation: theory and practice of hazard mitigation. Singapore. Springer. pp. 29-47Intelligent data analytics for decision-support systems in hazard mitigation: theory and practice of hazard mitigation
Deo, Ravinesh C., Samui, Pijush, Kisi, Ozgur and Yaseen, Zaher Mundher. 2021. Intelligent data analytics for decision-support systems in hazard mitigation: theory and practice of hazard mitigation. Singapore. Springer.Near real-time significant wave height forecasting with hybridized multiple linear regression algorithms
Ali, Mumtaz, Prasad, Ramendra, Xiang, Yong and Deo, Ravinesh C.. 2020. "Near real-time significant wave height forecasting with hybridized multiple linear regression algorithms." Renewable and Sustainable Energy Reviews. 132. https://doi.org/10.1016/j.rser.2020.110003Electrical Energy Demand Forecasting Model Development and Evaluation with Maximum Overlap Discrete Wavelet Transform-Online Sequential Extreme Learning Machines Algorithms
Al-Musaylh, Mohanad S., Deo, Ravinesh C. and Li, Yan. 2020. "Electrical Energy Demand Forecasting Model Development and Evaluation with Maximum Overlap Discrete Wavelet Transform-Online Sequential Extreme Learning Machines Algorithms." Energies. 13 (9). https://doi.org/10.3390/en13092307Monitoring rice growth status in the Mekong Delta, Vietnam using multitemporal Sentinel-1 data
Phung, Hoang-Phi, Lam-Dao, Nguyen, Nguyen-Huy, Thong, Le-Toan, Thuy and Apan, Armando A.. 2020. "Monitoring rice growth status in the Mekong Delta, Vietnam using multitemporal Sentinel-1 data." Journal of Applied Remote Sensing. 14 (1), pp. 014518-1-014518-23. https://doi.org/10.1117/1.JRS.14.014518Near real-time global solar radiation forecasting at multiple time-step horizons using the long short-term memory network
Huynh, Anh Ngoc‐Lan, Deo, Ravinesh C., An-Vo, Duc-Anh, Ali, Mumtaz, Raj, Nawin and Abdulla, Shahab. 2020. "Near real-time global solar radiation forecasting at multiple time-step horizons using the long short-term memory network." Energies. 13 (14). https://doi.org/10.3390/en13143517Adaptive boost LS-SVM classification approach for time-series signal classification in epileptic seizure diagnosis applications
Al-Hadeethi, Hanan, Abdulla, Shahab, Diykh, Mohammed, Deo, Ravinesh C. and Green, Jonathan H.. 2020. "Adaptive boost LS-SVM classification approach for time-series signal classification in epileptic seizure diagnosis applications." Expert Systems with Applications. 161. https://doi.org/10.1016/j.eswa.2020.113676Random forest predictive model development with uncertainty analysis capability for the estimation of evapotranspiration in an arid oasis region
Wu, Min, Feng, Qi, Wen, Xiaohu, Deo, Ravinesh C., Yin, Zhenliang, Yang, Linshan and Sheng, Danrui. 2020. "Random forest predictive model development with uncertainty analysis capability for the estimation of evapotranspiration in an arid oasis region." Hydrology Research: an international journal. 51 (4), pp. 648-665. https://doi.org/10.2166/nh.2020.012Stormwater runoff and pollution retention performances of permeable pavements and the effects of structural factors
Liu, Wen, Feng, Qi, Chen, Weiping and Deo, Ravinesh C.. 2020. "Stormwater runoff and pollution retention performances of permeable pavements and the effects of structural factors." Environmental Science and Pollution Research. 27 (24), pp. 30831-30843. https://doi.org/10.1007/s11356-020-09220-2Spatial mapping of short-term solar radiation prediction incorporating geostationary satellite images coupled with deep convolutional LSTM networks for South Korea
Yeom, Jong-Min, Deo, Ravinesh C., Adamowski, Jan F., Park, Seonyoung and Lee, Chang-Suk. 2020. "Spatial mapping of short-term solar radiation prediction incorporating geostationary satellite images coupled with deep convolutional LSTM networks for South Korea." Environmental Research Letters. 15 (9), pp. 1-10. https://doi.org/10.1088/1748-9326/ab9467Integrative stochastic model standardization with genetic algorithm for rainfall pattern forecasting in tropical and semi-arid environments
Salih, Sinan Q., Sharafati, Ahmad, Ebtehaj, Isa, Sanikhani, Hadi, Siddique, Ridwan, Deo, Ravinesh C., Bonakdari, Hossein, Shahid, Shamsuddin and Yaseen, Zaher Mundher. 2020. "Integrative stochastic model standardization with genetic algorithm for rainfall pattern forecasting in tropical and semi-arid environments." Hydrological Sciences Journal. 65 (7), pp. 1145-1157. https://doi.org/10.1080/02626667.2020.1734813Hybridized neural fuzzy ensembles for dust source modeling and prediction
Rahmati, Omid, Panahi, Mahdi, Ghiasi, Seid Saeid, Deo, Ravinesh C., Tiefenbacher, John P., Pradhan, Biswajeet, Jahani, Ali, Goshtasb, Hamid, Kornejady, Aiding, Shahabi, Himan, Shirzadi, Ataollah, Khosravi, Hassan, Moghaddam, Davoud Davoudi, Mohtashamian, Maryamsadat and Bui, Dieu Tien. 2020. "Hybridized neural fuzzy ensembles for dust source modeling and prediction." Atmospheric Environment. 224, pp. 1-11. https://doi.org/10.1016/j.atmosenv.2020.117320Trends and current state of research on greater one-horned rhinoceros (Rhinoceros unicornis): a systematic review of the literature over a period of 33 years (1985–2018)
Pant, Ganesh, Maraseni, Tek, Apan, Armando and Allen, Benjamin L.. 2020. "Trends and current state of research on greater one-horned rhinoceros (Rhinoceros unicornis): a systematic review of the literature over a period of 33 years (1985–2018)." Science of the Total Environment. 710, pp. 1-14. https://doi.org/10.1016/j.scitotenv.2019.136349Global solar radiation estimation and climatic variability analysis using extreme learning machine based predictive model
Tao, Hai, Sharafati, Ahmad, Mohammed, Achite, Salih, Sinan Q., Deo, Ravinesh C., Al-Ansari, Nadhir and Yaseen, Zaher Mundher. 2020. "Global solar radiation estimation and climatic variability analysis using extreme learning machine based predictive model." IEEE Access. 8, pp. 12026-12042. https://doi.org/10.1109/ACCESS.2020.2965303Development and evaluation of the cascade correlation neural network and the random forest models for river stage and river flow prediction in Australia
Ghorbani, Mohammad Ali, Deo, Ravinesh C., Kim, Sungwon, Kashani, Mahasa Hasanpour, Karimi, Vahid and Izadkhah, Maryam. 2020. "Development and evaluation of the cascade correlation neural network and the random forest models for river stage and river flow prediction in Australia." Soft Computing. 24, pp. 12079-12090. https://doi.org/10.1007/s00500-019-04648-2Causality of climate, food production and conflict over the last two millennia in the Hexi Corridor, China
Yang, Linshan, Feng, Qi, Adamowski, Jan F., Deo, Ravinesh C., Yin, Zhenlaing, Wen, Xiaohu, Tang, Xia and Wu, Min. 2020. "Causality of climate, food production and conflict over the last two millennia in the Hexi Corridor, China." Science of the Total Environment. 713, pp. 1-11. https://doi.org/10.1016/j.scitotenv.2020.136587Mixing characteristics of a film-exciting flapping jet
Wu, M., Xu, M., Mi, J. and Deo, R. C.. 2020. "Mixing characteristics of a film-exciting flapping jet." International Journal of Heat and Fluid Flow. 82, pp. 1-9. https://doi.org/10.1016/j.ijheatfluidflow.2019.108532Enhanced deep learning algorithm development to detect pain intensity from facial expression images
Bargshady, Ghazal, Zhou, Xujuan, Deo, Ravinesh C., Soar, Jeffery, Whittaker, Frank and Wang, Hua. 2020. "Enhanced deep learning algorithm development to detect pain intensity from facial expression images." Expert Systems with Applications. 149, pp. 1-10. https://doi.org/10.1016/j.eswa.2020.113305A general extensible learning approach for multi-disease recommendations in a telehealth environment
Lafta, Raid, Zhang, Ji, Tao, Xiaohui, Zhu, Xiaodong, Li, Hongzhou, Chang, Liang and Deo, Ravinesh. 2020. "A general extensible learning approach for multi-disease recommendations in a telehealth environment ." Pattern Recognition Letters. 132, pp. 106-114. https://doi.org/10.1016/j.patrec.2018.11.006A hybrid air quality early-warning framework: an hourly forecasting model with online sequential extreme learning machines and empirical mode decomposition algorithms
Sharma, Ekta, Deo, Ravinesh C., Prasad, Ramendra and Parisi, Alfio V.. 2020. "A hybrid air quality early-warning framework: an hourly forecasting model with online sequential extreme learning machines and empirical mode decomposition algorithms." Science of the Total Environment. 709, pp. 1-23. https://doi.org/10.1016/j.scitotenv.2019.135934Machine learning approaches for spatial modeling of agricultural droughts in the south-east region of Queensland Australia
Rahmati, Omid, Falah, Fatemeh, Dayal, Kavina Shaanu, Deo, Ravinesh C., Mohammadi, Farnoush, Biggs, Trent, Moghaddam, Davoud Davoudi, Naghibi, Seyed Amir and Bui, Dieu Tien. 2020. "Machine learning approaches for spatial modeling of agricultural droughts in the south-east region of Queensland Australia." Science of the Total Environment. 699 (134230). https://doi.org/10.1016/j.scitotenv.2019.134230Projected spatial patterns in precipitation and air temperature for China's northwest region derived from high‐resolution regional climate models
Yin, Zhenliang, Feng, Qi, Yang, Linshan, Deo, Ravinesh C., Adamowski, Jan F., Wen, Xiaohu, Jia, Bing and Si, Jianhua. 2020. "Projected spatial patterns in precipitation and air temperature for China's northwest region derived from high‐resolution regional climate models." International Journal of Climatology. 40 (8), pp. 3922-3941. https://doi.org/10.1002/joc.6435An assessment of governance quality for community-based forest management systems in Asia: Prioritisation of governance indicators at various scales
Maraseni, Tek Narayan, Bhattarai, Nabin, Karky, Bhaskar Singh, Cadman, Timothy, Timalsina, Niroj, Bhandari, Trishna Singh, Apan, Armando, Ma, Hwan Ok, Rawat, R. S., Verma, Nemit, San, Su Mon, Oo, Thaung Naing, Dorji, Kinley, Dhungana, Sindhu and Poudel, Mohan. 2019. "An assessment of governance quality for community-based forest management systems in Asia: Prioritisation of governance indicators at various scales." Land Use Policy: the international journal covering all aspects of land use. 81, pp. 750-671. https://doi.org/10.1016/j.landusepol.2018.11.044Exploring solar and wind energy resources in North Korea with COMS MI geostationary satellite data coupled with numerical weather prediction reanalysis variables
Yeom, Jong-Min, Deo, Ravinesh, Adamowski, Jan F., Chae, Taebyeong, Kim, Dong-Su, Han, Kyung-Soo and Kim, Do-Yong. 2020. "Exploring solar and wind energy resources in North Korea with COMS MI geostationary satellite data coupled with numerical weather prediction reanalysis variables." Renewable and Sustainable Energy Reviews. 119, pp. 1-14. https://doi.org/10.1016/j.rser.2019.109570Capability and robustness of novel hybridized models used for drought hazard modeling in southeast Queensland, Australia
Rahmati, Omid, Panahi, Mahdi, Kalantari, Zahra, Soltani, Elinaz, Falah, Fatemeh, Dayal, Kavina S., Mohammadi, Farnoush, Deo, Ravinesh C., Tiefenbacher, John and Bui, Dieu Tien. 2020. "Capability and robustness of novel hybridized models used for drought hazard modeling in southeast Queensland, Australia." Science of the Total Environment. 718, pp. 1-17. https://doi.org/10.1016/j.scitotenv.2019.134656Regional hydrology heterogeneity and the response to climate and land surface changes in arid alpine basin, northwest China
Yang, Linshan, Feng, Qi, Yin, Zhenliang, Deo, Ravinesh C., Wen, Xiaohu, Si, Jianhua and Liu, Wen. 2020. "Regional hydrology heterogeneity and the response to climate and land surface changes in arid alpine basin, northwest China." Catena. 187. https://doi.org/10.1016/j.catena.2019.104345An ensemble tree-based machine learning model for predicting the uniaxial compressive strength of travertine rocks
Barzegar, Rahim, Sattarpour, Masoud, Deo, Ravinesh, Fijani, Elham and Adamowski, Jan. 2020. "An ensemble tree-based machine learning model for predicting the uniaxial compressive strength of travertine rocks." Neural Computing and Applications. 32 (13), pp. 9065-9080. https://doi.org/10.1007/s00521-019-04418-zModern artificial intelligence model development for undergraduate student performance prediction: an investigation on engineering mathematics courses
Deo, Ravinesh C., Yaseen, Zaher Mundher, Al-Ansari, Nadhir, Nguyen-Huy, Thong, Langlands, Trevor and Galligan, Linda. 2020. "Modern artificial intelligence model development for undergraduate student performance prediction: an investigation on engineering mathematics courses." IEEE Access. 8, pp. 136697-136724. https://doi.org/10.1109/ACCESS.2020.3010938Correcting satellite precipitation data and assimilating satellite-derived soil moisture data to generate ensemble hydrological forecasts within the HBV rainfall-runoff model
Ciupak, Maurycy, Ozga-Zielinski, Bogdan, Adamowski, Jan, Deo, Ravinesh C and Kochanek, Krzysztof. 2019. "Correcting satellite precipitation data and assimilating satellite-derived soil moisture data to generate ensemble hydrological forecasts within the HBV rainfall-runoff model." Water: an open access journal. 11 (10), pp. 1-31. https://doi.org/10.3390/w11102138Partitioning groundwater recharge sources in multiple aquifers system within a desert oasis environment: Implications for water resources management in endorheic basins
Guo, Xiaoyan, Feng, Qi, Si, Jianhua, Xi, Haiyang, Zhao, Yan and Deo, Ravinesh C.. 2019. "Partitioning groundwater recharge sources in multiple aquifers system within a desert oasis environment: Implications for water resources management in endorheic basins." Journal of Hydrology. 579, pp. 1-11. https://doi.org/10.1016/j.jhydrol.2019.124212Probabilistic seasonal rainfall forecasts using semiparametric d-vine copula-based quantile regression
Nguyen-Huy, Thong, Deo, Ravinesh C., Mushtaq, Shahbaz and Khan, Shahjahan. 2020. "Probabilistic seasonal rainfall forecasts using semiparametric d-vine copula-based quantile regression." Samui, Pijush, Bui, Dieu Tien, Chakraborty, Subrata and Deo, Ravinesh C. (ed.) Handbook of probabilistic models. Oxford, United Kingdom. Elsevier. pp. 203-227Monthly rainfall forecasting with Markov Chain Monte Carlo simulations integrated with statistical bivariate copulas
Ali, Mumtaz, Deo, Ravinesh C., Downs, Nathan J. and Maraseni, Tek. 2020. "Monthly rainfall forecasting with Markov Chain Monte Carlo simulations integrated with statistical bivariate copulas." Samui, Pijush, Bui, Dieu Tien, Chakraborty, Subrata and Deo, Ravinesh C. (ed.) Handbook of probabilistic models. Oxford, United Kingdom. Elsevier. pp. 89-105Development of copula statistical drought prediction model using the Standardized Precipitation-Evapotranspiration Index
Dayal, Kavina S., Deo, Ravinesh C. and Apan, Armando A.. 2020. "Development of copula statistical drought prediction model using the Standardized Precipitation-Evapotranspiration Index." Samui, Pijush, Bui, Dieu Tien, Chakraborty, Subrata and Deo, Ravinesh C. (ed.) Handbook of probabilistic models. Oxford, United Kingdom. Elsevier. pp. 141-178Soil organic carbon in semiarid alpine regions: the spatial distribution, stock estimation, and environmental controls
Zhu, Meng, Feng, Qi, Zhang, Mengxu, Liu, Wei, Deo, Ravinesh C., Zhang, Chengqi and Yang, Linshan. 2019. "Soil organic carbon in semiarid alpine regions: the spatial distribution, stock estimation, and environmental controls." Journal of Soils and Sediments: protection, risk assessment and remediation. 19 (10), pp. 3427-3441. https://doi.org/10.1007/s11368-019-02295-6Controlling factors of plant community composition with respect to the slope aspect gradient in the Qilian Mountains
Qin, Yanyan, Adamowski, Jan F., Deo, Ravinesh C., Hu, Zeyong, Cao, Jianjun, Zhu, Meng and Feng, Qi. 2019. "Controlling factors of plant community composition with respect to the slope aspect gradient in the Qilian Mountains." Ecosphere. 10 (9), pp. 1-13. https://doi.org/10.1002/ecs2.2851Grassland degradation on the Qinghai-Tibetan Plateau: reevaluation of causative factors
Cao, Jianjun, Adamowski, Jan F., Deo, Ravinesh C., Xu, Xueyun, Gong, Yifan and Feng, Qi. 2019. "Grassland degradation on the Qinghai-Tibetan Plateau: reevaluation of causative factors." Rangeland Ecology and Management. 72 (6), pp. 988-995. https://doi.org/10.1016/j.rama.2019.06.001Fractal dimension undirected correlation graph-based support vector machine model for identification of focal and non-focal electroencephalography signals
Diykh, Mohammed, Abdulla, Shahab, Saleh, Khalid and Deo, Ravinesh C.. 2019. "Fractal dimension undirected correlation graph-based support vector machine model for identification of focal and non-focal electroencephalography signals." Biomedical Signal Processing and Control. 54, pp. 1-10. https://doi.org/10.1016/j.bspc.2019.101611The impacts of substrate and vegetation on stormwater runoff quality from extensive green roofs
Liu, Wen, Wei, Wei, Chen, Weiping, Deo, Ravinesh C., Si, Jianhua, Xi, Haiyang, Li, Baofeng and Feng, Qi. 2019. "The impacts of substrate and vegetation on stormwater runoff quality from extensive green roofs." Journal of Hydrology. 576, pp. 575-582. https://doi.org/10.1016/j.jhydrol.2019.06.061Application of multivariate recursive nesting bias correction, multiscale wavelet entropy and AI-based models to improve future precipitation projection in upstream of the Heihe River, Northwest China
Yang, Linshan, Feng, Qi, Yin, Zhenliang, Wen, Xiaohu, Deo, Ravinesh C., Si, Jianhua and Li, Changbin. 2019. "Application of multivariate recursive nesting bias correction, multiscale wavelet entropy and AI-based models to improve future precipitation projection in upstream of the Heihe River, Northwest China." Theoretical and Applied Climatology. 137 (1-2), pp. 323-339. https://doi.org/10.1007/s00704-018-2598-yWavelet-based 3-phase hybrid SVR model trained with satellite-derived predictors, particle swarm optimization and maximum overlap discrete wavelet transform for solar radiation prediction
Ghimire, Sujan, Deo, Ravinesh C., Raj, Nawin and Mi, Jianchun. 2019. "Wavelet-based 3-phase hybrid SVR model trained with satellite-derived predictors, particle swarm optimization and maximum overlap discrete wavelet transform for solar radiation prediction." Renewable and Sustainable Energy Reviews. 113, pp. 1-19. https://doi.org/10.1016/j.rser.2019.109247Deep solar radiation forecasting with convolutional neural network and long short-term memory network algorithms
Ghimire, Sujan, Deo, Ravinesh C., Raj, Nawin and Mi, Jianchun. 2019. "Deep solar radiation forecasting with convolutional neural network and long short-term memory network algorithms." Applied Energy. 253, pp. 1-20. https://doi.org/10.1016/j.apenergy.2019.113541Sleep EEG signal analysis based on correlation graph similarity coupled with an ensemble extreme machine learning algorithm
Abdulla, Shahab, Diykh, Mohammed, Lafta, Raid Luaibi, Saleh, Khalid and Deo, Ravinesh C.. 2019. "Sleep EEG signal analysis based on correlation graph similarity coupled with an ensemble extreme machine learning algorithm." Expert Systems with Applications. 138, pp. 1-15. https://doi.org/10.1016/j.eswa.2019.07.007Short-term electricity demand forecasting using machine learning methods enriched with ground-based climate and ECMWF Reanalysis atmospheric predictors in southeast Queensland, Australia
Al-Musaylh, Mohanad S., Deo, Ravinesh C., Adamowski, Jan F. and Li, Yan. 2019. "Short-term electricity demand forecasting using machine learning methods enriched with ground-based climate and ECMWF Reanalysis atmospheric predictors in southeast Queensland, Australia." Renewable and Sustainable Energy Reviews. 113. https://doi.org/10.1016/j.rser.2019.109293Development of statistical and geospatial-based framework for drought-risk assessment
Dayal, Kavina Shaanu. 2018. Development of statistical and geospatial-based framework for drought-risk assessment. PhD Thesis Doctor of Philosophy. University of Southern Queensland. https://doi.org/10.26192/9gt4-nt78Designing a new data intelligence model for global solar radiation prediction: application of multivariate modeling scheme
Tao, Hai, Ebtehaj, Isa, Bonakdari, Hossein, Heddam, Salim, Voyant, Cyril, Al-Ansari, Nadhir, Deo, Ravinesh and Yaseen, Zaheer Mundher. 2019. "Designing a new data intelligence model for global solar radiation prediction: application of multivariate modeling scheme." Energies. 12 (7), pp. 1-24. https://doi.org/10.3390/en12071365Incorporating synoptic-scale climate signals for streamflow modelling over the Mediterranean region using machine learning models
Kisi, Ozgur, Choubin, Bahram, Deo, Ravinesh C. and Yaseen, Zaheer Mundher. 2019. "Incorporating synoptic-scale climate signals for streamflow modelling over the Mediterranean region using machine learning models." Hydrological Sciences Journal. 64 (10), pp. 1240-1252. https://doi.org/10.1080/02626667.2019.1632460Improving SPI-derived drought forecasts incorporating synoptic-scale climate indices in multi-phase multivariate empirical mode decomposition model hybridized with simulated annealing and kernel ridge regression algorithms
Ali, Mumtaz, Deo, Ravinesh C., Maraseni, Tek and Downs, Nathan J.. 2019. "Improving SPI-derived drought forecasts incorporating synoptic-scale climate indices in multi-phase multivariate empirical mode decomposition model hybridized with simulated annealing and kernel ridge regression algorithms." Journal of Hydrology. 576, pp. 164-184. https://doi.org/10.1016/j.jhydrol.2019.06.032Deep Learning Neural Networks Trained with MODIS Satellite-Derived Predictors for Long-Term Global Solar Radiation Prediction
Ghimire, Sujan, Deo, Ravinesh C., Raj, Nawin and Mi, Jianchun. 2019. "Deep Learning Neural Networks Trained with MODIS Satellite-Derived Predictors for Long-Term Global Solar Radiation Prediction." Energies. 12 (12), pp. 1-42. https://doi.org/10.3390/en12122407Situations, challenges and strategies of urban water management in Beijing under rapid urbanization effect
Liu, Wen, Chen, Weiping, Feng, Qi and Deo, Ravinesh C.. 2019. "Situations, challenges and strategies of urban water management in Beijing under rapid urbanization effect." Water Science and Technology: Water Supply. 19 (1), pp. 115-127. https://doi.org/10.2166/ws.2018.057Suitable exclosure duration for the restoration of degraded alpine grasslands on the Qinghai-Tibetan Plateau
Cao, Jianjun, Li, Guangdong, Adamowski, Jan F., Holden, Nicolas M., Deo, Ravinesh C., Hu, Zeyong, Zhu, Guofeng, Xu, Xueyun and Feng, Qi. 2019. "Suitable exclosure duration for the restoration of degraded alpine grasslands on the Qinghai-Tibetan Plateau." Land Use Policy: the international journal covering all aspects of land use. 86, pp. 261-267. https://doi.org/10.1016/j.landusepol.2019.05.008Environmental and economic impacts and trade-offs from simultaneous management of soil constraints, nitrogen and water
Kodur, Shreevatsa, Shrestha, Uttam Babu, Maraseni, Tek Narayan and Deo, Ravinesh C.. 2019. "Environmental and economic impacts and trade-offs from simultaneous management of soil constraints, nitrogen and water." Journal of Cleaner Production. 222, pp. 960-970. https://doi.org/10.1016/j.jclepro.2019.03.079Land subsidence modelling using tree-based machine learning algorithms
Rahmati, Omid, Falah, Fatemeh, Naghibi, Seyed Amir, Biggs, Trent, Soltani, Milad, Deo, Ravinesh C., Cerda, Artemi, Mohammadi, Farnoush and Bui, Dieu Tien. 2019. "Land subsidence modelling using tree-based machine learning algorithms." Science of the Total Environment. 672, pp. 239-252. https://doi.org/10.1016/j.scitotenv.2019.03.496Weekly soil moisture forecasting with multivariate sequential, ensemble empirical mode decomposition and Boruta-random forest hybridizer algorithm approach
Prasad, Ramendra, Deo, Ravinesh C., Li, Yan and Maraseni, Tek. 2019. "Weekly soil moisture forecasting with multivariate sequential, ensemble empirical mode decomposition and Boruta-random forest hybridizer algorithm approach." Catena. 177, pp. 149-166. https://doi.org/10.1016/j.catena.2019.02.012Copula statistical models for analyzing stochastic dependencies of systemic drought risk and potential adaptation strategies
Nguyen-Huy, Thong, Deo, Ravinesh C., Mushtaq, Shahbaz, Kath, Jarrod and Khan, Shahjahan. 2019. "Copula statistical models for analyzing stochastic dependencies of systemic drought risk and potential adaptation strategies." Stochastic Environmental Research and Risk Assessment. 33 (3), pp. 779-799. https://doi.org/10.1007/s00477-019-01662-6Effects of stand age on carbon storage in dragon spruce forest ecosystems in the upper reaches of the Bailongjiang River basin, China
Cao, Jianjun, Gong, Yifan, Adamowski, Jan F., Deo, Ravinesh C., Zhu, Guofeng, Dong, Xiaogang, Zhang, Xiaofang, Liu, Haibo and Xin, Cunlin. 2019. "Effects of stand age on carbon storage in dragon spruce forest ecosystems in the upper reaches of the Bailongjiang River basin, China." Scientific Reports. 9 (1), pp. 1-11. https://doi.org/10.1038/s41598-019-39626-zThe role of topography in shaping the spatial patterns of soil organic carbon
Zhu, Meng, Feng, Qi, Qin, Yanyan, Cao, Jiajun, Zhang, Mengxu, Liu, Wei, Deo, Ravinesh C., Zhang, Chengqi, Li, Ruolin and Li, Baofeng. 2019. "The role of topography in shaping the spatial patterns of soil organic carbon." Catena. 176, pp. 296-305. https://doi.org/10.1016/j.catena.2019.01.029PMT: new analytical framework for automated evaluation of geo-environmental modelling approaches
Rahmati, Omid, Kornejady, Aiding, Samadi, Mahmood, Deo, Ravinesh C., Conoscenti, Christian, Lombardo, Luigi, Dayal, Kavina, Taghizadeh-Mehrjardi, Ruhollah, Pourghasemi, Hamid Reza, Kumar, Sandeep and Bui, Dieu Tien. 2019. "PMT: new analytical framework for automated evaluation of geo-environmental modelling approaches." Science of the Total Environment. 664, pp. 296-311. https://doi.org/10.1016/j.scitotenv.2019.02.017Universally deployable extreme learning machines integrated with remotely sensed MODIS satellite predictors over Australia to forecast global solar radiation: a new approach
Deo, Ravinesh C., Sahin, Mehmet, Adamowski, Jan F. and Mi, Jianchun. 2019. "Universally deployable extreme learning machines integrated with remotely sensed MODIS satellite predictors over Australia to forecast global solar radiation: a new approach." Renewable and Sustainable Energy Reviews. 104, pp. 235-261. https://doi.org/10.1016/j.rser.2019.01.009Two-phase extreme learning machines integrated with the complete ensemble empirical mode decomposition with adaptive noise algorithm for multi-scale runoff prediction problems
Wen, Xiaohu, Feng, Qi, Deo, Ravinesh C., Wu, Min, Yin, Zhenliang, Yang, Linshan and Singh, Vijay P.. 2019. "Two-phase extreme learning machines integrated with the complete ensemble empirical mode decomposition with adaptive noise algorithm for multi-scale runoff prediction problems." Journal of Hydrology. 570, pp. 167-184. https://doi.org/10.1016/j.jhydrol.2018.12.060Remotely sensed agricultural grassland productivity responses to land use and hydro-climatic drivers under extreme drought and rainfall
Kath, Jarrod, Le Brocque, Andrew, Reardon-Smith, Kathryn and Apan, Armando. 2019. "Remotely sensed agricultural grassland productivity responses to land use and hydro-climatic drivers under extreme drought and rainfall." Agricultural and Forest Meteorology. 268, pp. 11-22. https://doi.org/10.1016/j.agrformet.2019.01.007New approach for sediment yield forecasting with a two-phase feed forward neuron network-particle swarm optimization model integrated with the gravitational search algorithm
Meshram, Sarita Gajbhiye, Ghorbani, M. A., Deo, Ravinesh C., Kashani, Mahsa Hasanpour, Meshram, Chandrashekhar and Karimi, Vahid. 2019. "New approach for sediment yield forecasting with a two-phase feed forward neuron network-particle swarm optimization model integrated with the gravitational search algorithm." Water Resources Management. 33 (7), pp. 2335-2356. https://doi.org/10.1007/s11269-019-02265-0Long-term modelling of wind speeds using six different heuristic artificial intelligence approaches
Maroufpoor, Saman, Sanikhani, Hadi, Kisi, Ozgur, Deo, Ravinesh C. and Yaseen, Zaher Mundher. 2019. "Long-term modelling of wind speeds using six different heuristic artificial intelligence approaches." International Journal of Climatology. 39 (8), pp. 3543-3557. https://doi.org/10.1002/joc.6037Development and evaluation of hybrid artificial neural network architectures for modeling spatio-temporal groundwater fluctuations in a complex aquifer system
Roshni, Thendiyath, Jha, Madan K., Deo, Ravinesh C. and Vandana, A.. 2019. "Development and evaluation of hybrid artificial neural network architectures for modeling spatio-temporal groundwater fluctuations in a complex aquifer system." Water Resources Management. 33 (7), pp. 2381-2397. https://doi.org/10.1007/s11269-019-02253-4An enhanced extreme learning machine model for river flow forecasting: state-of-the-art, practical applications in water resource engineering area and future research direction
Yaseen, Zaher Mundher, Sulaiman, Sadeq Oleiwi, Deo, Ravinesh C. and Chau, Kwok-Wing. 2019. "An enhanced extreme learning machine model for river flow forecasting: state-of-the-art, practical applications in water resource engineering area and future research direction." Journal of Hydrology. 569, pp. 387-408. https://doi.org/10.1016/j.jhydrol.2018.11.069The influence of structural factors on stormwater runoff retention of extensive green roofs: new evidence from scale-based models and real experiments
Liu, Wen, Feng, Qi, Chen, Weiping, Wei, Wei and Deo, Ravinesh C.. 2019. "The influence of structural factors on stormwater runoff retention of extensive green roofs: new evidence from scale-based models and real experiments." Journal of Hydrology. 569, pp. 230-238. https://doi.org/10.1016/j.jhydrol.2018.11.066Direct and indirect impacts of ionic components of saline water on irrigated soil chemical and microbial processes
Chen, Lijuan, Li, Changsheng, Feng, Qi, Wei, Yongping, Zhao, Yan, Zhu, Meng and Deo, Ravinesh C.. 2019. "Direct and indirect impacts of ionic components of saline water on irrigated soil chemical and microbial processes." Catena. 172, pp. 581-589. https://doi.org/10.1016/j.catena.2018.09.030Global solar radiation prediction by ANN integrated with European Centre for medium range weather forecast fields in solar rich cities of Queensland Australia
Ghimire, Sujan, Deo, Ravinesh C., Downs, Nathan J. and Raj, Nawin. 2019. "Global solar radiation prediction by ANN integrated with European Centre for medium range weather forecast fields in solar rich cities of Queensland Australia." Journal of Cleaner Production. 216, pp. 288-310. https://doi.org/10.1016/j.jclepro.2019.01.158Future projection with an extreme-learning machine and support vector regression of reference evapotranspiration in a mountainous inland watershed in north-west China
Yin, Zhenliang, Feng, Qi, Yang, Linshan, Deo, Ravinesh C., Wen, Xiaohu, Si, Jianhua and Xiao, Shengchun. 2017. "Future projection with an extreme-learning machine and support vector regression of reference evapotranspiration in a mountainous inland watershed in north-west China." Water: an open access journal. 9 (11), pp. 880-902. https://doi.org/10.3390/w9110880The spatial and temporal contribution of glacier runoff to watershed discharge in the Yarkant River Basin, Northwest China
Yin, Zhenliang, Feng, Qi, Liu, Shiyin, Zhou, Songbing, Li, Jing, Yang, Linshan and Deo, Ravinesh C.. 2017. "The spatial and temporal contribution of glacier runoff to watershed discharge in the Yarkant River Basin, Northwest China." Water: an open access journal. 9 (3), pp. 159-178. https://doi.org/10.3390/w9030159Tree biomass quantity, carbon stock and canopy correlates in mangrove forest and land uses that replaced mangroves in Honda Bay, Philippines
Castillo, Jose Alan A., Apan, Armando A., Maraseni, Tek Narayan and Salmo, Severino G., III. 2018. "Tree biomass quantity, carbon stock and canopy correlates in mangrove forest and land uses that replaced mangroves in Honda Bay, Philippines." Regional Studies in Marine Science. 24, pp. 174-183. https://doi.org/10.1016/j.rsma.2018.08.006Particle swarm optimized–support vector regression hybrid model for daily horizon electricity demand forecasting using climate dataset
Al-Musaylh, Mohanad S., Deo, Ravinesh C. and Li, Yan. 2018. "Particle swarm optimized–support vector regression hybrid model for daily horizon electricity demand forecasting using climate dataset." Huang, Q. and Kolhe, M. (ed.) 3rd International Conference on Power and Renewable Energy (ICPRE 2018). Berlin, Germany 21 - 24 Sep 2018 Germany. https://doi.org/10.1051/e3sconf/20186408001Effects of topography on soil organic carbon stocks in grasslands of a semiarid alpine region, northwestern China
Zhu, Meng, Feng, Qi, Zhang, Mengxu, Liu, Wei, Qin, Yanyan, Deo, Ravinesh C. and Zhang, Chengqi. 2019. "Effects of topography on soil organic carbon stocks in grasslands of a semiarid alpine region, northwestern China." Journal of Soils and Sediments: protection, risk assessment and remediation. 19 (4), pp. 1640-1650. https://doi.org/10.1007/s11368-018-2203-0A joint deep neural network model for pain recognition from face
Bargshady, Ghazal, Soar, Jeffrey, Zhou, Xujuan, Deo, Ravinesh, Whittaker, Frank and Wang, Hua. 2019. "A joint deep neural network model for pain recognition from face." 4th IEEE International Conference on Computer and Communication Systems (ICCCS 2019). Singapore 23 - 25 Feb 2019 Singapore. pp. 52-56 https://doi.org/10.1109/CCOMS.2019.8821779Effects of Afforestation on Soil Bulk Density and pH in the Loess Plateau, China
Zhang, Xiaofang, Adamowski, Jan F., Deo, Ravinesh C., Xu, Xueyun, Zhu, Guofeng and Cao, Jianjun. 2018. "Effects of Afforestation on Soil Bulk Density and pH in the Loess Plateau, China." Water: an open access journal. 10 (12), pp. 1-15. https://doi.org/10.3390/w10121710Assessment of soil salinisation in the Ejina Oasis located in the lower reaches of Heihe River, Northwestern China
Zhao, Yu, Feng, Qi, Lu, Aigang and Deo, Ravinesh C.. 2019. "Assessment of soil salinisation in the Ejina Oasis located in the lower reaches of Heihe River, Northwestern China." Chemistry and Ecology. 35 (4), pp. 330-343. https://doi.org/10.1080/02757540.2018.1554060Design and implementation of a hybrid MLP-GSA model with multilayer perceptron-gravitational search algorithm for monthly lake water level forecasting
Ghorbani, Mohammad Ali, Deo, Ravinesh C., Karimi, Vahid, Kashani, Mahsa H. and Ghorbani, Shahryar. 2018. "Design and implementation of a hybrid MLP-GSA model with multilayer perceptron-gravitational search algorithm for monthly lake water level forecasting." Stochastic Environmental Research and Risk Assessment. 33 (1), pp. 125-147. https://doi.org/10.1007/s00477-018-1630-1Monitoring paddy productivity in North Korea employing geostationary satellite images integrated with GRAMI-rice model
Yeom, Jong-min, Jeong, Seungtaek, Jeong, Gwanyong, Ng, Chi Tim, Deo, Ravinesh C. and Ko, Jonghan. 2018. "Monitoring paddy productivity in North Korea employing geostationary satellite images integrated with GRAMI-rice model." Scientific Reports. 8, pp. 1-15. https://doi.org/10.1038/s41598-018-34550-0Artificial intelligence approach for the prediction of Robusta coffee yield using soil fertility properties
Kouadio, Louis, Deo, Ravinesh C., Byrareddy, Vivekananda, Adamowski, Jan F., Mushtaq, Shahbaz and Nguyen, Van Phuong. 2018. "Artificial intelligence approach for the prediction of Robusta coffee yield using soil fertility properties." Computers and Electronics in Agriculture. 155, pp. 324-338. https://doi.org/10.1016/j.compag.2018.10.014Artificial intelligence-based fast and efficient hybrid approach for spatial modelling of soil electrical conductivity
Ghorbani, Mohammad Ali, Deo, Ravinesh C., Kashani, Mahsa H., Shahabi, Mahmoud and Ghorbani, Shahryar. 2019. "Artificial intelligence-based fast and efficient hybrid approach for spatial modelling of soil electrical conductivity." Soil and Tillage Research. 186, pp. 152-164. https://doi.org/10.1016/j.still.2018.09.012Quantifying flood events in Bangladesh with a daily-step flood monitoring index based on the concept of daily effective precipitation
Deo, Ravinesh C., Adamowski, Jan F., Begum, Khaleda, Salcedo-sanz, Sancho, Kim, Do-Woo, Dayal, Kavina S. and Byun, Hi-Ryong. 2019. "Quantifying flood events in Bangladesh with a daily-step flood monitoring index based on the concept of daily effective precipitation." Theoretical and Applied Climatology. 137, pp. 1201-1215. https://doi.org/10.1007/s00704-018-2657-4Mapping of peanut crops in Queensland, Australia using time-series PROBA-V 100-m normalized difference vegetation index imagery
Haerani, Haerani, Apan, Armando and Basnet, Badri. 2018. "Mapping of peanut crops in Queensland, Australia using time-series PROBA-V 100-m normalized difference vegetation index imagery." Journal of Applied Remote Sensing. 12 (3), pp. 1-22. https://doi.org/10.1117/1.JRS.12.036005Shear strength prediction of steel fiber reinforced concrete beam using hybrid intelligence models: a new approach
Yaseen, Zaheer Munder, Tran, Minh Tung, Kim, Sungwon, Bakhshpoori, Taha and Deo, Ravinesh C.. 2018. "Shear strength prediction of steel fiber reinforced concrete beam using hybrid intelligence models: a new approach." Engineering Structures. 177, pp. 244-255. https://doi.org/10.1016/j.engstruct.2018.09.074Adaptive Neuro-Fuzzy Inference System integrated with solar zenith angle for forecasting sub-tropical photosynthetically active radiation
Deo, Ravinesh C., Downs, Nathan J., Adamowski, Jan F. and Parisi, Alfio V.. 2018. "Adaptive Neuro-Fuzzy Inference System integrated with solar zenith angle for forecasting sub-tropical photosynthetically active radiation." Food and Energy Security. 8 (1). https://doi.org/10.1002/fes3.151Cotton yield prediction with Markov Chain Monte Carlo-based simulation model integrated with genetic programing algorithm: a new hybrid copula-driven approach
Ali, Mumtaz, Deo, Ravinesh C., Downs, Nathan J. and Maraseni, Tek. 2018. "Cotton yield prediction with Markov Chain Monte Carlo-based simulation model integrated with genetic programing algorithm: a new hybrid copula-driven approach." Agricultural and Forest Meteorology. 263, pp. 428-448. https://doi.org/10.1016/j.agrformet.2018.09.002Weekly heat wave death prediction model using zero-inflated regression approach
Kim, Do-Woo, Deo, Ravinesh C., Park, Sang-Jin, Lee, Jong-Seol and Lee, Woo-Seop. 2019. "Weekly heat wave death prediction model using zero-inflated regression approach." Theoretical and Applied Climatology. 137 (1-2), pp. 823-838. https://doi.org/10.1007/s00704-018-2636-9A comparative study of temperature and precipitation‑based aridity indices and their trends in Mongolia
Nyamtseren, Mandakh, Feng, Qi and Deo, Ravinesh C.. 2018. "A comparative study of temperature and precipitation‑based aridity indices and their trends in Mongolia." International Journal of Environmental Research. 12, pp. 887-899. https://doi.org/10.1007/s41742-018-0143-6Can individual land ownership reduce grassland degradation and favor socioeconomic sustainability on the Qinghai-Tibetan Plateau?
Cao, J. J., Holden, N. M., Adamowski, J. F., Deo, R. C., Xu, X. Y. and Feng, Q.. 2018. "Can individual land ownership reduce grassland degradation and favor socioeconomic sustainability on the Qinghai-Tibetan Plateau?" Environmental Science and Policy. 89, pp. 192-197. https://doi.org/10.1016/j.envsci.2018.08.003Copula-based agricultural conditional value-at-risk modelling for geographical diversifications in wheat farming portfolio management
Nguyen-Huy, Thong, Deo, Ravinesh C., Mushtaq, Shahbaz, Kath, Jarrod and Khan, Shahjahan. 2018. "Copula-based agricultural conditional value-at-risk modelling for geographical diversifications in wheat farming portfolio management." Weather and Climate Extremes. 21, pp. 76-89. https://doi.org/10.1016/j.wace.2018.07.002Design and evaluation of SVR, MARS and M5Tree models for 1, 2 and 3-day lead time forecasting of river flow data in a semiarid mountainous catchment
Yin, Zhenliang, Feng, Qi, Wen, Xiaohu, Deo, Ravinesh C., Yang, Linshan, Si, Jianhua and He, Zhibin. 2018. "Design and evaluation of SVR, MARS and M5Tree models for 1, 2 and 3-day lead time forecasting of river flow data in a semiarid mountainous catchment." Stochastic Environmental Research and Risk Assessment. 32 (9), pp. 2457-2476. https://doi.org/10.1007/s00477-018-1585-2Mapping hypothermia death vulnerability in Korea
Park, Sang-Jin, Kim, Do-Woo, Deo, Ravinesh C. and Lee, Jong-Seol. 2018. "Mapping hypothermia death vulnerability in Korea." International Journal of Disaster Risk Reduction. 31, pp. 668-678. https://doi.org/10.1016/j.ijdrr.2018.06.016Multi-stage committee based extreme learning machine model incorporating the influence of climate parameters and seasonality on drought forecasting
Ali, Mumtaz, Deo, Ravinesh C., Downs, Nathan J. and Maraseni, Tek. 2018. "Multi-stage committee based extreme learning machine model incorporating the influence of climate parameters and seasonality on drought forecasting." Computers and Electronics in Agriculture. 152, pp. 149-165. https://doi.org/10.1016/j.compag.2018.07.013A real-time hourly water index for flood risk monitoring: pilot studies in Brisbane, Australia, and Dobong Observatory, South Korea
Deo, Ravinesh C., Byun, Hi-Ryong, Kim, Ga-Byn and Adamowski, Jan F.. 2018. "A real-time hourly water index for flood risk monitoring: pilot studies in Brisbane, Australia, and Dobong Observatory, South Korea." Environmental Monitoring and Assessment. 190 (450). https://doi.org/10.1007/s10661-018-6806-0Multi-stage hybridized online sequential extreme learning machine integrated with Markov Chain Monte Carlo copula-Bat algorithm for rainfall forecasting
Ali, Mumtaz, Deo, Ravinesh C., Downs, Nathan J. and Maraseni, Tek. 2018. "Multi-stage hybridized online sequential extreme learning machine integrated with Markov Chain Monte Carlo copula-Bat algorithm for rainfall forecasting." Atmospheric Research. 213, pp. 450-464. https://doi.org/10.1016/j.atmosres.2018.07.005Influence of stand type and stand age on soil carbon storage in China’s arid and semi-arid regions
Cao, Jianjun, Zhang, Xiaofang, Deo, Ravinesh, Gong, Yifan and Feng, Qi. 2018. "Influence of stand type and stand age on soil carbon storage in China’s arid and semi-arid regions." Land Use Policy: the international journal covering all aspects of land use. 78, pp. 258-265. https://doi.org/10.1016/j.landusepol.2018.07.002Design and implementation of a hybrid model based on two-layer decomposition method coupled with extreme learning machines to support real-time environmental monitoring of water quality parameters
Fijani, Elham, Barzegar, Rahim, Deo, Ravinesh, Tziritis, Evangelos and Konstantinos, Skordas. 2019. "Design and implementation of a hybrid model based on two-layer decomposition method coupled with extreme learning machines to support real-time environmental monitoring of water quality parameters." Science of the Total Environment. 648, pp. 839-853. https://doi.org/10.1016/j.scitotenv.2018.08.221Optimization of windspeed prediction using an artificial neural network compared with a genetic programming model
Deo, Ravinesh C., Ghimire, Sujan, Downs, Nathan J. and Raj, Nawin. 2018. "Optimization of windspeed prediction using an artificial neural network compared with a genetic programming model." Kim, Dookie, Roy, Sanjiban Sekhar, Lansivaara, Tim, Deo, Ravinesh C. and Samui, Pijush (ed.) Handbook of research on predictive modeling and optimization methods in science and engineering. Hershey, United States. IGI Global. pp. 328-359Hybrid data intelligent models and applications for water level prediction
Yaseen, Zaher Mundher, Deo, Ravinesh C., Ebtehaj, Isa and Bonakdari, Hossein. 2018. "Hybrid data intelligent models and applications for water level prediction." Kim, Dookie, Roy, Sanjiban Sekhar, Länsivaara, Tim, Deo, Ravinesh C. and Samui, Pijush (ed.) Handbook of research on predictive modeling and optimization methods in science and engineering. Hershey, United States. IGI Global. pp. 121-139Selection of representative feature training sets with self-organized maps for optimized time series modeling and prediction: application to forecasting daily drought conditions with ARIMA and neural network models
McCarthy, Elizabeth, Deo, Ravinesh C., Li, Yan and Maraseni, Tek. 2018. "Selection of representative feature training sets with self-organized maps for optimized time series modeling and prediction: application to forecasting daily drought conditions with ARIMA and neural network models." Kim, Dookie, Roy, Sanjiban Sekhar, Lansivaara, Tim, Deo, Ravinesh C. and Samui, Pijush (ed.) Handbook of research on predictive modeling and optimization methods in science and engineering. Hershey, United States. IGI Global. pp. 446-464Survey of different data-intelligent modeling strategies for forecasting air temperature using geographic information as model predictors
Sanikhani, Hadi, Deo, Ravinesh C., Samui, Pijush, Kisi, Ozgur, Mert, Chian, Mirabbasi, Rasoul, Gavili, Siavash and Yaseen, Zaher Mundher. 2018. "Survey of different data-intelligent modeling strategies for forecasting air temperature using geographic information as model predictors." Computers and Electronics in Agriculture. 152, pp. 242-260. https://doi.org/10.1016/j.compag.2018.07.008Soil moisture forecasting by a hybrid machine learning technique: ELM integrated with ensemble empirical mode decomposition
Prasad, Ramendra, Deo, Ravinesh C., Li, Yan and Maraseni, Tek. 2018. "Soil moisture forecasting by a hybrid machine learning technique: ELM integrated with ensemble empirical mode decomposition." Geoderma. 330, pp. 136-161. https://doi.org/10.1016/j.geoderma.2018.05.035Modeling the joint influence of multiple synoptic-scale, climate mode indices on Australian wheat yield using a vine copula-based approach
Nguyen-Huy, Thong, Deo, Ravinesh C., Mushtaq, Shahbaz, An-Vo, Duc-Anh and Khan, Shahjahan. 2018. "Modeling the joint influence of multiple synoptic-scale, climate mode indices on Australian wheat yield using a vine copula-based approach." European Journal of Agronomy. 98, pp. 65-81. https://doi.org/10.1016/j.eja.2018.05.006Non-tuned data intelligent model for soil temperature estimation: a new approach
Sanikhani, Hadi, Deo, Ravinesh C., Yaseen, Zaher Mundheer, Eray, Okan and Kisi, Ozgur. 2018. "Non-tuned data intelligent model for soil temperature estimation: a new approach." Geoderma. 330, pp. 52-64. https://doi.org/10.1016/j.geoderma.2018.05.030Drought prediction with standardized precipitation and evapotranspiration index and support vector regression models
Deo, Ravinesh C., Salcedo-sanz, Sancho, Carro-Calvo, Leopoldo and Saavedra-Moreno, Beatriz. 2018. "Drought prediction with standardized precipitation and evapotranspiration index and support vector regression models." Samui, Pijush, Kim, Dookie and Ghosh, Chandan (ed.) Integrating disaster science and management. Amsterdam, Netherlands. Elsevier. pp. 151-174Drivers of grassland resistance and recovery to long term drought
Le Brocque, Andrew F, Kath, Jarrod, Reardon-Smith, Kate and Apan, Armando. 2017. "Drivers of grassland resistance and recovery to long term drought." 6th Joint Conference of the Ecological Society of Australia and the New Zealand Ecological Society (EcoTAS 2017). Pokolbin, Australia 26 Nov - 01 Dec 2017Input selection and data-driven model performance optimization to predict the Standardized Precipitation and Evaporation Index in a drought-prone region
Mouatadid, Soukayna, Raj, Nawin, Deo, Ravinesh C. and Adamowski, Jan F.. 2018. "Input selection and data-driven model performance optimization to predict the Standardized Precipitation and Evaporation Index in a drought-prone region." Atmospheric Research. 212, pp. 130-149. https://doi.org/10.1016/j.atmosres.2018.05.012Re-imagining standard timescales in forecasting precipitation events for Queensland’s grazing enterprises
McCarthy, E., Deo, R. C., Li, Y. and Maraseni, T.. 2017. "Re-imagining standard timescales in forecasting precipitation events for Queensland’s grazing enterprises." Syme, G., Hatton MacDonald, D., Fulton, B. and Piantadosi, J. (ed.) 22nd International Congress on Modelling and Simulation (MODSIM2017). Hobart, Australia 03 - 08 Dec 2017 Australia. Modelling and Simulation Society of Australia and New Zealand . https://doi.org/10.36334/modsim.2017.L1.mccarthySelf-adaptive differential evolutionary extreme learning machines for long-term solar radiation prediction with remotely-sensed MODIS satellite and Reanalysis atmospheric products in solar-rich cities
Ghimire, Sujan, Deo, Ravinesh C., Downs, Nathan J. and Raj, Nawin. 2018. "Self-adaptive differential evolutionary extreme learning machines for long-term solar radiation prediction with remotely-sensed MODIS satellite and Reanalysis atmospheric products in solar-rich cities." Remote Sensing of Environment: an interdisciplinary journal. 212, pp. 176-198. https://doi.org/10.1016/j.rse.2018.05.003An ensemble-ANFIS based uncertainty assessment model for forecasting multi-scalar standardized precipitation index
Ali, Mumtaz, Deo, Ravinesh C., Downs, Nathan J. and Maraseni, Tek. 2018. "An ensemble-ANFIS based uncertainty assessment model for forecasting multi-scalar standardized precipitation index." Atmospheric Research. 207, pp. 155-180. https://doi.org/10.1016/j.atmosres.2018.02.024Wavelet analysis–artificial neural network conjunction models for multi-scale monthly groundwater level predicting in an arid inland river basin, northwestern China
Wen, Xiaohu, Feng, Qi, Deo, Ravinesh C., Wu, Min and Si, Jianhua. 2017. "Wavelet analysis–artificial neural network conjunction models for multi-scale monthly groundwater level predicting in an arid inland river basin, northwestern China." Hydrology Research: an international journal. 48 (6), pp. 1710-1729. https://doi.org/10.2166/nh.2016.396Ensemble committee-based data intelligent approach for generating soil moisture forecasts with multivariate hydro-meteorological predictors
Prasad, Ramendra, Deo, Ravinesh C., Li, Yan and Maraseni, Tek. 2018. "Ensemble committee-based data intelligent approach for generating soil moisture forecasts with multivariate hydro-meteorological predictors." Soil and Tillage Research. 181, pp. 63-81. https://doi.org/10.1016/j.still.2018.03.021Comparison of social-ecological resilience between two grassland management patterns driven by grassland land contract policy in the Maqu, Qinghai-Tibetan Plateau
Cao, Jianjun, Li, Mengtian, Deo, Ravinesh C., Adamowski, Jan F, Cerda, Artemi, Feng, Qi, Liu, Minxia, Zhang, Jian, Zhu, Guofeng, Zhang, Xuebin, Xu, Xueyun, Yang, Shurong and Gong, Yifan. 2018. "Comparison of social-ecological resilience between two grassland management patterns driven by grassland land contract policy in the Maqu, Qinghai-Tibetan Plateau." Land Use Policy: the international journal covering all aspects of land use. 74, pp. 88-96. https://doi.org/10.1016/j.landusepol.2017.07.027Spatio-temporal drought risk mapping approach and its application in the drought-prone region of south-east Queensland, Australia
Dayal, Kavina S., Deo, Ravinesh C. and Apan, Armando A.. 2018. "Spatio-temporal drought risk mapping approach and its application in the drought-prone region of south-east Queensland, Australia." Natural Hazards. 93 (2), pp. 823-847. https://doi.org/10.1007/s11069-018-3326-8A new approach to predict daily pH in rivers based on the 'a trous' redundant wavelet transform algorithm
Rajaee, Taher, Ravansalar, Masoud, Adamowski, Jan F. and Deo, Ravinesh C.. 2018. "A new approach to predict daily pH in rivers based on the 'a trous' redundant wavelet transform algorithm." Water, Air and Soil Pollution: an international journal of environmental pollution. 229 (3). https://doi.org/10.1007/s11270-018-3715-3Computational intelligence approaches for energy load forecasting in smart energy management grids: state of the art, future challenges, and research directions and Research Directions
Fallah, Seyedeh Narjes, Deo, Ravinesh Chand, Shojafar, Mohammad, Conti, Mauro and Shamshirband, Shahaboddin. 2018. "Computational intelligence approaches for energy load forecasting in smart energy management grids: state of the art, future challenges, and research directions and Research Directions." Energies. 11 (3). https://doi.org/10.3390/en11030596Two-phase particle swarm optimized-support vector regression hybrid model integrated with improved empirical mode decomposition with adaptive noise for multiple-horizon electricity demand forecasting
Al-Musaylh, Mohanad S., Deo, Ravinesh C., Li, Yan and Adamowski, Jan F.. 2018. "Two-phase particle swarm optimized-support vector regression hybrid model integrated with improved empirical mode decomposition with adaptive noise for multiple-horizon electricity demand forecasting." Applied Energy. 217, pp. 422-439. https://doi.org/10.1016/j.apenergy.2018.02.140Application of the hybrid artificial neural network coupled with rolling mechanism and grey model algorithms for streamflow forecasting over multiple time horizons
Yaseen, Zaher Mundher, Fu, Minglei, Wang, Chen, Mohtar, Wan Hanna Melini Wan, Deo, Ravinesh C. and El-Shafie, Ahmed. 2018. "Application of the hybrid artificial neural network coupled with rolling mechanism and grey model algorithms for streamflow forecasting over multiple time horizons." Water Resources Management. 32 (5), pp. 1883-1899. https://doi.org/10.1007/s11269-018-1909-5Multi-household grazing management pattern maintains better soil fertility
Cao, Jianjun, Xu, Xueyun, Deo, Ravinesh C., Holden, Nicholas M., Adamowski, Jan F., Gong, Yifan, Feng, Qi, Yang, Shurong, Li, Mengtian, Zhou, Junju, Zhang, Jian and Liu, Minxia. 2018. "Multi-household grazing management pattern maintains better soil fertility." Agronomy for Sustainable Development: sciences des productions vegetales et de l'environnement. 38 (1). https://doi.org/10.1007/s13593-017-0482-2Short-term electricity demand forecasting with MARS, SVR and ARIMA models using aggregated demand data in Queensland, Australia
Al-Musaylh, Mohanad S., Deo, Ravinesh C., Adamowski, Jan F. and Li, Yan. 2018. "Short-term electricity demand forecasting with MARS, SVR and ARIMA models using aggregated demand data in Queensland, Australia." Advanced Engineering Informatics: the science of supporting knowledge-intensive activities. 35 (C), pp. 1-16. https://doi.org/10.1016/j.aei.2017.11.002Mapping groundwater contamination risk of multiple aquifers using multi-model ensemble of machine learning algorithms
Barzegar, Rahim, Moghaddam, Asghar Asghari, Deo, Ravinesh, Fijani, Elham and Tziritis, Evangelos. 2018. "Mapping groundwater contamination risk of multiple aquifers using multi-model ensemble of machine learning algorithms." Science of the Total Environment. 621, pp. 697-712. https://doi.org/10.1016/j.scitotenv.2017.11.185Investigating drought duration-severity-intensity characteristics using the Standardized Precipitation-Evapotranspiration Index: case studies in drought-prone Southeast Queensland
Dayal, Kavina S., Deo, Ravinesh C. and Apan, Armando A.. 2018. "Investigating drought duration-severity-intensity characteristics using the Standardized Precipitation-Evapotranspiration Index: case studies in drought-prone Southeast Queensland." Journal of Hydrologic Engineering. 23 (1), p. 05017029. https://doi.org/10.1061/(ASCE)HE.1943-5584.0001593An efficient neuro-evolutionary hybrid modelling mechanism for the estimation of daily global solar radiation in the Sunshine State of Australia
Salcedo-sanz, Sancho, Deo, Ravinesh C., Cornejo-Bueno, Laura, Camacho-Gomez, Carlos and Ghimire, Sujan. 2018. "An efficient neuro-evolutionary hybrid modelling mechanism for the estimation of daily global solar radiation in the Sunshine State of Australia." Applied Energy. 209, pp. 79-94. https://doi.org/10.1016/j.apenergy.2017.10.076Multi-layer perceptron hybrid model integrated with the firefly optimizer algorithm for windspeed prediction of target site using a limited set of neighboring reference station data
Deo, Ravinesh C., Ghorbani, Mohammad Ali, Samadianfard, Saeed, Maraseni, Tek, Bilgili, Mehmet and Biazar, Mustafa. 2018. "Multi-layer perceptron hybrid model integrated with the firefly optimizer algorithm for windspeed prediction of target site using a limited set of neighboring reference station data." Renewable Energy. 116 (Part A), pp. 309-323. https://doi.org/10.1016/j.renene.2017.09.078Identifying separate impacts of climate and land use/cover change on hydrological processes in upper stream of Heihe River, northwest China
Yang, Linshan, Feng, Qi, Yin, Zhenliang, Wen, Xiaohu, Si, Jianhua, Li, Changbin and Deo, Ravinesh C.. 2017. "Identifying separate impacts of climate and land use/cover change on hydrological processes in upper stream of Heihe River, northwest China." Hydrological Processes. 31 (5), pp. 1100-1112. https://doi.org/10.1002/hyp.11098Reservoir inflow forecasting with a modified coactive neuro-fuzzy inference system: a case study for a semi-arid region
Allawi, Mohammed Falah, Jaafar, Othman, Hamzah, Firdaus Mohamad, Mohd, Nuruol Syuhadaa, Deo, Ravinesh C. and El-Shafie, Ahmed. 2018. "Reservoir inflow forecasting with a modified coactive neuro-fuzzy inference system: a case study for a semi-arid region." Theoretical and Applied Climatology. 134 (1-2), pp. 545-563. https://doi.org/10.1007/s00704-017-2292-5Implementation of a hybrid MLP-FFA model for water level prediction of Lake Egirdir, Turkey
Ghorbani, Mohammad Ali, Deo, Ravinesh C., Karimi, Vahid, Yaseen, Zaher Mundher and Terz, Ozlem. 2018. "Implementation of a hybrid MLP-FFA model for water level prediction of Lake Egirdir, Turkey." Stochastic Environmental Research and Risk Assessment. 32 (6), pp. 1683-1697. https://doi.org/10.1007/s00477-017-1474-0Pan evaporation prediction using a hybrid multilayer perceptron-firefly algorithm (MLP-FFA) model: case study in North Iran
Ghorbani, M. A., Deo, Ravinesh C., Yaseen, Zaher Mundher, Kashani, Mahsa H. and Mohammadi, Babak. 2018. "Pan evaporation prediction using a hybrid multilayer perceptron-firefly algorithm (MLP-FFA) model: case study in North Iran." Theoretical and Applied Climatology. 133 (3-4), pp. 1119-1131. https://doi.org/10.1007/s00704-017-2244-0Impact of grassland contract policy on soil organic carbon losses from alpine grassland on the Qinghai–Tibetan Plateau
Cao, J., Gong, Y., Yeh, E. T., Holden, N. M., Adamowski, J. F., Deo, R. C., Liu, M., Zhou, J., Zhang, J., Zhang, S., Sheng, D., Yang, S., Xu, X., Li, M. and Feng, Q.. 2017. "Impact of grassland contract policy on soil organic carbon losses from alpine grassland on the Qinghai–Tibetan Plateau." Soil Use and Management. 33 (4), pp. 633-671. https://doi.org/10.1111/sum.12387Effects of ecological water transport on photosynthesis and chlorophyll fluorescence of Populus euphratica
Zhao, Chun Yan, Si, Jian Hua, Feng, Qi, Yu, Teng Fei, Deo, Ravinesh C. and Luo, Huan. 2018. "Effects of ecological water transport on photosynthesis and chlorophyll fluorescence of Populus euphratica." Water Science and Technology: Water Supply. 18 (5), pp. 1747-1756. https://doi.org/10.2166/ws.2017.236Trend analysis of Water Poverty Index for assessment of water stress and water management polices: a case study in the Hexi Corridor, China
Huang, Shan, Feng, Qi, Lu, Zhixiang, Wen, Xiaohu and Deo, Ravinesh C.. 2017. "Trend analysis of Water Poverty Index for assessment of water stress and water management polices: a case study in the Hexi Corridor, China." Sustainability. 9 (5), pp. 756-772. https://doi.org/10.3390/su9050756Forecasting evaporative loss by least-square support-vector regression and evaluation with genetic programming, Gaussian process, and minimax probability machine regression: case study of Brisbane City
Deo, Ravinesh C. and Samui, Pijush. 2017. "Forecasting evaporative loss by least-square support-vector regression and evaluation with genetic programming, Gaussian process, and minimax probability machine regression: case study of Brisbane City." Journal of Hydrologic Engineering. 22 (6), pp. 1-15. https://doi.org/10.1061/(ASCE)HE.1943-5584.0001506Statistical evaluation of rainfall time series in concurrence with agriculture and water resources of Ken River basin, Central India (1901–2010)
Meshram, Sarita Gajbhiye, Singh, Sudhir Kumar, Meshram, Chandrashekhar, Deo, Ravinesh C. and Ambade, Balram. 2018. "Statistical evaluation of rainfall time series in concurrence with agriculture and water resources of Ken River basin, Central India (1901–2010)." Theoretical and Applied Climatology. 134, pp. 1231-1243. https://doi.org/10.1007/s00704-017-2335-yAn international comparison of rice consumption behaviours and greenhouse gas emissions from rice production
Maraseni, Tek Narayan, Deo, Ravinesh C., Qu, Jiansheng, Gentle, Popular and Neupane, Prem Raj. 2018. "An international comparison of rice consumption behaviours and greenhouse gas emissions from rice production." Journal of Cleaner Production. 172, pp. 2288-2300. https://doi.org/10.1016/j.jclepro.2017.11.182Physiological response to salinity stress and tolerance mechanics of Populus euphratica
Zhao, Chun Yan, Si, Jian Hua, Feng, Qi, Deo, Ravinesh C., Yu, Teng Fei and Li, Pei Du. 2017. "Physiological response to salinity stress and tolerance mechanics of Populus euphratica." Environmental Monitoring and Assessment. 189 (11), pp. 533-543. https://doi.org/10.1007/s10661-017-6257-zCarbon dioxide fluxes and their environmental controls in a riparian forest within the hyper-arid region of Northwest China
Ma, Xiaohong, Feng, Qi, Yu, Tengfei, Su, Yonghong and Deo, Ravinesh C.. 2017. "Carbon dioxide fluxes and their environmental controls in a riparian forest within the hyper-arid region of Northwest China." Forests. 8 (10), pp. 1-17. https://doi.org/10.3390/f8100379Comparative study of hybrid-wavelet artificial intelligence models for monthly groundwater depth forecasting in extreme arid regions, Northwest China
Yu, Haijiao, Wen, Xiaohu, Feng, Qi, Deo, Ravinesh C., Si, Jianhua and Wu, Min. 2018. "Comparative study of hybrid-wavelet artificial intelligence models for monthly groundwater depth forecasting in extreme arid regions, Northwest China." Water Resources Management. 32 (1), pp. 301-323. https://doi.org/10.1007/s11269-017-1811-6Predicting compressive strength of lightweight foamed concrete using extreme learning machine model
Yaseen, Zaher Mundher, Deo, Ravinesh C., Hilal, Ameer, Abd, Abbas M., Bueno, Laura Cornejo, Salcedo-sanz, Sancho and Nehdi, Moncef L.. 2018. "Predicting compressive strength of lightweight foamed concrete using extreme learning machine model." Advances in Engineering Software. 115, pp. 112-125. https://doi.org/10.1016/j.advengsoft.2017.09.004Novel approach for streamflow forecasting using a hybrid ANFIS-FFA model
Yaseen, Zaher Mundher, Ebtehaj, Isa, Bonakdari, Hossein, Deo, Ravinesh C., Mehr, Ali Danandeh, Mohtar, Wan Hanna Melini Wan, Diop, Lamine, El-Shafie, Ahmed and Singh, Vijay P.. 2017. "Novel approach for streamflow forecasting using a hybrid ANFIS-FFA model." Journal of Hydrology. 554, pp. 263-276. https://doi.org/10.1016/j.jhydrol.2017.09.007Rainfall Pattern Forecasting Using Novel Hybrid Intelligent Model Based ANFIS-FFA
Yaseen, Zaher Mundher, Ghareb, Mazen Ismaeel, Ebtehaj, Isa, Bonakdari, Hossein, Siddique, Ridwan, Heddam, Sali, Yusif, Ali A. and Deo, Ravinesh. 2018. "Rainfall Pattern Forecasting Using Novel Hybrid Intelligent Model Based ANFIS-FFA." Water Resources Management. 32 (1), pp. 105-122. https://doi.org/10.1007/s11269-017-1797-0Synthetic retrieval of hourly net ecosystem exchange using the neural network model with combined MI and GOCI geostationary sensor datasets and ground-based measurements
Yeom, Jong-Min, Deo, Ravinesh, Chun, Junghwa, Hong, Jinkyu, Kim, Dong-Su, Han, Kyung-Soo and Cho, Jaeil. 2017. "Synthetic retrieval of hourly net ecosystem exchange using the neural network model with combined MI and GOCI geostationary sensor datasets and ground-based measurements." International Journal of Remote Sensing. 38 (23), pp. 7441-7456. https://doi.org/10.1080/01431161.2017.1375573Separation of the Climatic and Land Cover Impacts on the Flow Regime Changes in Two Watersheds of Northeastern Tibetan Plateau
Yang, Linshan, Feng, Qi, Yin, Zhenliang, Deo, Ravinesh C., Wen, Xiaohu, Si, Jianhua and Li, Changbin. 2017. "Separation of the Climatic and Land Cover Impacts on the Flow Regime Changes in Two Watersheds of Northeastern Tibetan Plateau." Advances in Meteorology. 2017, pp. 1-15. https://doi.org/10.1155/2017/6310401Changes in climatic elements in the Pan-Hexi region during 1960–2014 and responses to global climatic changes
Wei, Liu, Feng, Qi and Deo, Ravinesh C.. 2018. "Changes in climatic elements in the Pan-Hexi region during 1960–2014 and responses to global climatic changes." Theoretical and Applied Climatology. 133 (1-2), pp. 405-420. https://doi.org/10.1007/s00704-017-2194-6Mapping heatwave vulnerability in Korea
Kim, Do-Woo, Deo, Ravinesh C., Lee, Jong-Seol and Yeom, Jong-Min. 2017. "Mapping heatwave vulnerability in Korea." Natural Hazards. 89 (1), pp. 35-55. https://doi.org/10.1007/s11069-017-2951-yCopula-statistical precipitation forecasting model in Australia’s agro-ecological zones
Nguyen-Huy, Thong, Deo, Ravinesh C., An-Vo, Duc-Anh, Mushtaq, Shahbaz and Khan, Shahjahan. 2017. "Copula-statistical precipitation forecasting model in Australia’s agro-ecological zones." Agricultural Water Management. 191, pp. 153-172. https://doi.org/10.1016/j.agwat.2017.06.010Input selection and performance optimization of ANN-based streamflow forecasts in the drought-prone Murray Darling Basin region using IIS and MODWT algorithm
Prasad, Ramendra, Deo, Ravinesh C., Li, Yan and Maraseni, Tek. 2017. "Input selection and performance optimization of ANN-based streamflow forecasts in the drought-prone Murray Darling Basin region using IIS and MODWT algorithm." Atmospheric Research. 197, pp. 42-63. https://doi.org/10.1016/j.atmosres.2017.06.014Soil C quantities of mangrove forests, their competing land uses, and their spatial distribution in the coast of Honda Bay, Philippines
Castillo, Jose Alan A., Apan, Armando A., Maraseni, Tek Narayan and Salmo, Severino G., III. 2017. "Soil C quantities of mangrove forests, their competing land uses, and their spatial distribution in the coast of Honda Bay, Philippines." Geoderma. 293, pp. 82-90. https://doi.org/10.1016/j.geoderma.2017.01.025Very short-term reactive forecasting of the solar ultraviolet index using an extreme learning machine integrated with the solar zenith angle
Deo, Ravinesh C., Downs, Nathan, Parisi, Alfio V., Adamowski, Jan F. and Quilty, John M.. 2017. "Very short-term reactive forecasting of the solar ultraviolet index using an extreme learning machine integrated with the solar zenith angle." Environmental Research. 155, pp. 141-166. https://doi.org/10.1016/j.envres.2017.01.035The rate, extent and spatial predictors of forest loss (2000-2012) in the terrestrial protected areas of the Philippines
Apan, Armando, Suarez, Luz Angelica, Maraseni, Tek and Castillo, Jose Alan. 2017. "The rate, extent and spatial predictors of forest loss (2000-2012) in the terrestrial protected areas of the Philippines." Applied Geography. 81, pp. 32-42. https://doi.org/10.1016/j.apgeog.2017.02.007Association between plant species diversity and edaphic factors in the lower reaches of the Heihe River, northwestern China
Zhao, Yu, Feng, Qi, Xi, Haiyang, Li, Huiya, Yang, Huaide and Deo, Ravinesh C.. 2017. "Association between plant species diversity and edaphic factors in the lower reaches of the Heihe River, northwestern China." Chemistry and Ecology. 33 (3), pp. 181-195. https://doi.org/10.1080/02757540.2017.1287904Forecasting long-term global solar radiation with an ANN algorithm coupled with satellite-derived (MODIS) land surface temperature (LST) for regional locations in Queensland
Deo, Ravinesh C. and Sahin, Mehmet. 2017. "Forecasting long-term global solar radiation with an ANN algorithm coupled with satellite-derived (MODIS) land surface temperature (LST) for regional locations in Queensland." Renewable and Sustainable Energy Reviews. 72, pp. 828-848. https://doi.org/10.1016/j.rser.2017.01.114Computational intelligence approach for modeling hydrogen production: a review
Faizollahzadeh, Sina, Najafi, Bahman, Shamshirband, Shahaboddin, Bidgoli, Behrouz Minaei, Deo, Ravinesh Chand and Chau, Kwok-wing. 2018. "Computational intelligence approach for modeling hydrogen production: a review." Engineering Applications of Computational Fluid Mechanics. 12 (1), pp. 438-458. https://doi.org/10.1080/19942060.2018.1452296Savanna burning methodology for fire management and emissions reduction: a critical review of influencing factors
Maraseni, Tek Narayan, Reardon‑Smith, Kathryn, Griffiths, Greg and Apan, Armando. 2016. "Savanna burning methodology for fire management and emissions reduction: a critical review of influencing factors." Carbon Balance and Management. 11, pp. 1-11. https://doi.org/10.1186/s13021-016-0067-4Stream-flow forecasting using extreme learning machines: a case study in a semi-arid region in Iraq
Yaseen, Zaher Mundher, Jaafar, Othman, Deo, Ravinesh C., Kisi, Ozgur, Adamowski, Jan, Quilty, John and El-Shafie, Ahmed. 2016. "Stream-flow forecasting using extreme learning machines: a case study in a semi-arid region in Iraq." Journal of Hydrology. 542, pp. 603-614. https://doi.org/10.1016/j.jhydrol.2016.09.035Quantitative definition and spatiotemporal distribution of little water season (LIWAS) in Korea
Kim, Su-Jeong, Byun, Hi-Ryong and Deo, Ravinesh C.. 2016. "Quantitative definition and spatiotemporal distribution of little water season (LIWAS) in Korea." Asia-Pacific Journal of Atmospheric Sciences. 52 (4), pp. 379-393. https://doi.org/10.1007/s13143-016-0012-1Soil greenhouse gas fluxes in tropical mangrove forests and in land uses on deforested mangrove lands
Castillo, Jose Alan A., Apan, Armando A., Maraseni, Tek N. and Salmo, Severino G., III. 2017. "Soil greenhouse gas fluxes in tropical mangrove forests and in land uses on deforested mangrove lands." Catena. 159, pp. 60-69. https://doi.org/10.1016/j.catena.2017.08.005Estimation and mapping of above-ground biomass of mangrove forests and their replacement land uses in the Philippines using Sentinel imagery
Castillo, Jose Alan A., Apan, Armando A., Maraseni, Tek N. and Salmo, Severino G., III. 2017. "Estimation and mapping of above-ground biomass of mangrove forests and their replacement land uses in the Philippines using Sentinel imagery." ISPRS Journal of Photogrammetry and Remote Sensing. 134, pp. 70-85. https://doi.org/10.1016/j.isprsjprs.2017.10.016Hyperspectral sensing to detect the impact of herbicide drift on cotton growth and yield
Suarez, L. A., Apan, Armando and Werth, Jeff. 2016. "Hyperspectral sensing to detect the impact of herbicide drift on cotton growth and yield." ISPRS Journal of Photogrammetry and Remote Sensing. 120, pp. 65-76. https://doi.org/10.1016/j.isprsjprs.2016.08.004An extreme learning machine model for the simulation of monthly mean streamflow water level in eastern Queensland
Deo, Ravinesh C. and Sahin, Mehmet. 2016. "An extreme learning machine model for the simulation of monthly mean streamflow water level in eastern Queensland." Environmental Monitoring and Assessment. 188 (90). https://doi.org/10.1007/s10661-016-5094-9Groundwater decline and climatic variability: Implications for groundwater linked agro-ecosystems
Le Brocque, Andrew F., Kath, Jarrod, Reardon-Smith, Kate and Apan, Armando. 2016. "Groundwater decline and climatic variability: Implications for groundwater linked agro-ecosystems." 2016 Conference of the Ecological Society of Australia (ESA 2016). Fremantle, Australia 29 Nov - 02 Dec 2016 Australia.Forecasting effective drought index using a wavelet extreme learning machine (W-ELM) model
Deo, Ravinesh C., Tiwari, Mukesh K., Adamowski, Jan F. and Quilty, John M.. 2017. "Forecasting effective drought index using a wavelet extreme learning machine (W-ELM) model." Stochastic Environmental Research and Risk Assessment. 31 (5), pp. 1211-1240. https://doi.org/10.1007/s00477-016-1265-zVulnerability assessment of urban community and critical infrastructures for integrated flood risk management and climate adaptation strategies
Espada, Rudolf, Apan, Armando and McDougall, Kevin. 2017. "Vulnerability assessment of urban community and critical infrastructures for integrated flood risk management and climate adaptation strategies." International Journal of Disaster Resilience in the Built Environment. 8 (4), pp. 375-411. https://doi.org/10.1108/IJDRBE-03-2015-0010Drought forecasting in eastern Australia using multivariate adaptive regression spline, least square support vector machine and M5Tree model
Deo, Ravinesh C., Kisi, Ogzur and Singh, Vijay P.. 2017. "Drought forecasting in eastern Australia using multivariate adaptive regression spline, least square support vector machine and M5Tree model." Atmospheric Research. 184, pp. 149-175. https://doi.org/10.1016/j.atmosres.2016.10.004A wavelet-coupled support vector machine model for forecasting global incident solar radiation using limited meteorological dataset
Deo, Ravinesh C., Wen, Xiaohu and Feng, Qi. 2016. "A wavelet-coupled support vector machine model for forecasting global incident solar radiation using limited meteorological dataset." Applied Energy. 168, pp. 568-593. https://doi.org/10.1016/j.apenergy.2016.01.130Application of hybrid artificial neural network algorithm for the prediction of Standardized Precipitation Index
Dayal, Kavina S., Deo, Ravinesh C. and Apan, Armando A.. 2016. "Application of hybrid artificial neural network algorithm for the prediction of Standardized Precipitation Index." 2016 IEEE Region 10 International Conference: Technologies for Smart Nation (TENCON 2016). Singapore 22 - 25 Nov 2016 Singapore. https://doi.org/10.1109/TENCON.2016.7848588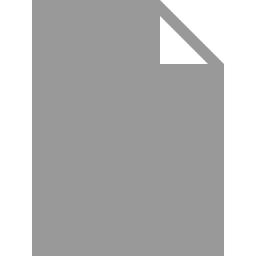
Application of Modis data to assess the latest forest cover changes of Sri Lanka
Perera, Kithsiri, Herath, Srikantha, Apan, Armando and Tateishi, Ryutaro. 2013. "Application of Modis data to assess the latest forest cover changes of Sri Lanka." Ogleby, Cliff (ed.) 22nd Annual Photogrammetry, Remote Sensing and Spatial Information Science Congress (ISPRS 2012): Imaging a Sustainable Future. Melbourne, Australia 25 Aug - 01 Sep 2012 Australia. Copernicus GmbH. https://doi.org/10.5194/isprsannals-I-7-165-2012Detection of phenoxy herbicide dosage in cotton crops through the analysis of hyperspectral data
Suarez, L. A., Apan, Armando and Werth, J.. 2017. "Detection of phenoxy herbicide dosage in cotton crops through the analysis of hyperspectral data." International Journal of Remote Sensing. 38 (23), pp. 6528-6553. https://doi.org/10.1080/01431161.2017.1362128Delineation of management zones using multiple crop yield data
Basnet, Badri, Kelly, Rob, Jensen, Troy, Strong, Wayne, Apan, Armando and Butler, David. 2003. "Delineation of management zones using multiple crop yield data." 16th Triennial Congress of the International Soil Tillage Research Organisation (ISTRO 2003). Brisbane, Australia 13 - 18 Jul 2003 Brisbane, Australia.Correlating high resolution aerial imagery with wheat yield and protein content
Apan, A., Kelly, R., Jensen, T., Strong, W. and Butler, D.. 2004. "Correlating high resolution aerial imagery with wheat yield and protein content." Smith, Richard and Dawbin, Ken (ed.) 12th Australasian Remote Sensing and Photogrammetry Conference (ARSPC 2004): To Measure is to Manage. Fremantle, Australia 18 - 22 Oct 2004 Adelaide, Australia.Spectral discrimination and classification of sugarcane varieties using EO-1 hyperion hyperspectral imagery
Apan, Armando, Held, Alex, Phinn, Stuart and Markley, John. 2004. "Spectral discrimination and classification of sugarcane varieties using EO-1 hyperion hyperspectral imagery." 25th Asian Conference on Remote Sensing (ACRS 2004). Chiang Mai, Thailand 22 - 26 Nov 2004 China.Application of aerial imagery to analyse on-farm trials
Basnet, B., Kelly, R., Jensen, T., Kane, T., Apan, A. and Butler, D.. 2002. "Application of aerial imagery to analyse on-farm trials." 11th Australasian Remote Sensing and Photogrammetry Conference (ARSPC2002): Images to Information. Brisbane, Australia 02 - 06 Sep 2002 Australia.Capturing of remotely sensed images to advance the use of yield maps and other spatial information to improve crop management and forecasting
Jensen, T., Apan, A., Young, F. and Zeller, L.. 2002. "Capturing of remotely sensed images to advance the use of yield maps and other spatial information to improve crop management and forecasting." 11th Australasian Remote Sensing and Photogrammetry Conference (ARSPC2002): Images to Information. Brisbane, Australia 02 - 06 Sep 2002 Adelaide, Australia.Spectral discrimination and separability analysis of agricultural crops and soil attributes using ASTER imagery
Apan, Armando, Kelly, Rob, Jensen, Troy, Butler, David, Strong, Wayne and Basnet, Badri. 2002. "Spectral discrimination and separability analysis of agricultural crops and soil attributes using ASTER imagery." 11th Australasian Remote Sensing and Photogrammetry Conference (ARSPC2002): Images to Information. Brisbane, Australia 02 - 06 Sep 2002 Adelaide, Australia.Quantifying landscape fragmentation in the Lockyer Valley Catchment, Queensland: 1973 – 1997
Apan, Armando A., Raine, Steven R. and Paterson, Mark S.. 2000. "Quantifying landscape fragmentation in the Lockyer Valley Catchment, Queensland: 1973 – 1997." AURISA 2000 Conference (AURISA 2000). Coolum, Australia 20 - 24 Nov 2000Image analysis techniques for assessing landscape structural change: a case study of the Lockyer Valley Catchment, Queensland
Apan, Armando A., Raine, Steven R. and Paterson, Mark S.. 2000. "Image analysis techniques for assessing landscape structural change: a case study of the Lockyer Valley Catchment, Queensland." 10th Australasian Remote Sensing and Photogrammetry Conference (ARSPC 2000). Adelaide, Australia 21 - 25 Aug 2000Prediction of SPEI using MLR and ANN: a case study for Wilsons Promontory Station in Victoria
Mouatadid, Soukayna, Deo, Ravinesh C. and Adamowski, Jan F.. 2015. "Prediction of SPEI using MLR and ANN: a case study for Wilsons Promontory Station in Victoria." Guerrero, Juan E. (ed.) 2015 IEEE 14th International Conference on Machine Learning and Applications. Miami, United States of America 09 - 11 Dec 2015 United States. https://doi.org/10.1109/ICMLA.2015.87Statistical downscaling of climate change scenarios of rainfall and temperature over Indira Sagar Canal Command area in Madhya Pradesh, India
Shukla, Rituraj, Deo, Ravinesh and Khare, Deepak. 2015. "Statistical downscaling of climate change scenarios of rainfall and temperature over Indira Sagar Canal Command area in Madhya Pradesh, India." Guerrero, Juan E. (ed.) 2015 IEEE 14th International Conference on Machine Learning and Applications. Miami, United States of America 09 - 11 Dec 2015 USA. https://doi.org/10.1109/ICMLA.2015.75Monthly prediction of air temperature in Australia and New Zealand with machine learning algorithms
Salcedo-sanz, S., Deo, R. C., Carro-Calvo, L. and Saavedra-Moreno, B.. 2016. "Monthly prediction of air temperature in Australia and New Zealand with machine learning algorithms." Theoretical and Applied Climatology. 125 (1-2), pp. 13-25. https://doi.org/10.1007/s00704-015-1480-4Vulnerability assessment and interdependency analysis of critical infrastructures for climate adaptation and flood mitigation
Espada Jr., Rodolfo, Apan, Armando and McDougall, Kevin. 2015. "Vulnerability assessment and interdependency analysis of critical infrastructures for climate adaptation and flood mitigation." International Journal of Disaster Resilience in the Built Environment. 6 (3), pp. 313-346. https://doi.org/10.1108/IJDRBE-02-2014-0019Projection of heat wave mortality related to climate change in Korea
Kim, Do-Woo, Deo, Ravinesh C., Chung, Jea-Hak and Lee, Jong-Seol. 2016. "Projection of heat wave mortality related to climate change in Korea." Natural Hazards. 80 (1), pp. 623-637. https://doi.org/10.1007/s11069-015-1987-0Estimation of monthly evaporative loss using relevance vector machine, extreme learning machine and multivariate adaptive regression spline models
Deo, Ravinesh C., Samui, Pijush and Kim, Dookie. 2016. "Estimation of monthly evaporative loss using relevance vector machine, extreme learning machine and multivariate adaptive regression spline models." Stochastic Environmental Research and Risk Assessment. 30 (6), pp. 1769-1784. https://doi.org/10.1007/s00477-015-1153-yNumerical modelling of the velocity field of a plane jet flow at moderate jet exit Reynolds numbers
Mossad, Ruth and Deo, Ravinesh C.. 2015. "Numerical modelling of the velocity field of a plane jet flow at moderate jet exit Reynolds numbers." Solnordal, C. B., Liovic, P., Delaney, G. W., Cummins, S. J., Schwarz, M. P. and Witt, P. J. (ed.) 11th International Conference on CFD in the Minerals and Process Industries. Melbourne, Australia 07 - 09 Dec 2015 Melbourne, Australia.On the new concept of the available water climatology and its application
Byun, H. R., Kim, D. W., Choi, K. S., Deo, R. C., Lee, S. M., Park, C. K., Kwon, S. H., Kim, G. B. and Kwon, H. N.. 2014. "On the new concept of the available water climatology and its application." American Geophysical Union, Fall Meeting 2014. San Francisco, United States 15 - 19 Dec 2014 San Francisco, USA.A real-time flood monitoring index based on daily effective precipitation and its application to Brisbane and Lockyer Valley flood events
Deo, Ravinesh C., Byun, Hi-Ryong, Adamowski, Jan F. and Kim, Do-Woo. 2015. "A real-time flood monitoring index based on daily effective precipitation and its application to Brisbane and Lockyer Valley flood events." Water Resources Management. 29 (11), pp. 4075-4093. https://doi.org/10.1007/s11269-015-1046-3Prioritising carbon sequestration areas in southern Queensland using time series MODIS net primary productivity (NPP) imagery
Apan, A., Suarez Cadavid, L. A., Richardson, L. and Maraseni, T.. 2014. "Prioritising carbon sequestration areas in southern Queensland using time series MODIS net primary productivity (NPP) imagery." Dadhwal, V. K., Seshasai, M. V. R., Hakeem, A., Diwakar, P. G. and Raju, P. L. N. (ed.) Technical Commission 8th Mid-Term Symposium (ISPRS 2014). Hyderabad, India 09 - 12 Dec 2014 Goettingen, Germany. https://doi.org/10.5194/isprsarchives-XL-8-549-2014Application of the artificial neural network model for prediction of monthly standardized precipitation and evapotranspiration index using hydrometeorological parameters and climate indices in eastern Australia
Deo, Ravinesh C. and Sahin, Mehmet. 2015. "Application of the artificial neural network model for prediction of monthly standardized precipitation and evapotranspiration index using hydrometeorological parameters and climate indices in eastern Australia." Atmospheric Research. 161-162, pp. 65-81. https://doi.org/10.1016/j.atmosres.2015.03.018Spectral discrimination of bulloak (Allocasuarina luehmannii) and associated woodland for habitat mapping of the endangered bulloak jewel butterfly (Hypochrysops piceata) in southern Queensland
Zainol Abdullah, Wan Nor Zanariah, Apan, Armando A., Maraseni, Tek N. and Le Brocque, Andrew F.. 2014. "Spectral discrimination of bulloak (Allocasuarina luehmannii) and associated woodland for habitat mapping of the endangered bulloak jewel butterfly (Hypochrysops piceata) in southern Queensland." Journal of Applied Remote Sensing. 8 (1), pp. 083561-1. https://doi.org/10.1117/1.JRS.8.083561Spatial modelling of natural disaster risk reduction policies with Markov decision processes
Espada Jr., Rodolfo, Apan, Armando and McDougall, Kevin. 2014. "Spatial modelling of natural disaster risk reduction policies with Markov decision processes." Applied Geography. 53, pp. 284-298. https://doi.org/10.1016/j.apgeog.2014.06.021Diagnosis of flood events in Brisbane (Australia) using a flood index based on daily effective precipitation
Deo, R. C., Byun, H. R., Adamowski, J. F. and Kim, D. W.. 2014. "Diagnosis of flood events in Brisbane (Australia) using a flood index based on daily effective precipitation." International Conference on Analysis and Management of Changing Risks for Natural Hazards (2014). Padua, Italy Padua, Italy.The impact of varying statutory arrangements on spatial data sharing and access in regional NRM bodies
Paudyal, D. R., McDougall, K. and Apan, A.. 2014. "The impact of varying statutory arrangements on spatial data sharing and access in regional NRM bodies." ISPRS Annals of the Photogrammetry, Remote Sensing and Spatial Information Sciences. II (8), pp. 193-197. https://doi.org/10.5194/isprsannals-II-8-193-2014Application of the extreme learning machine algorithm for the prediction of monthly Effective Drought Index in eastern Australia
Deo, Ravinesh C. and Sahin, Mehmet. 2015. "Application of the extreme learning machine algorithm for the prediction of monthly Effective Drought Index in eastern Australia." Atmospheric Research. 153, pp. 512-525. https://doi.org/10.1016/j.atmosres.2014.10.016Drought prediction till 2100 under RCP 8.5 climate change scenarios for Korea
Park, Chang-Kyun, Byun, Hi-Ryong, Deo, Ravinesh and Lee, Bo-Ra. 2015. "Drought prediction till 2100 under RCP 8.5 climate change scenarios for Korea." Journal of Hydrology. 526, pp. 221-230. https://doi.org/10.1016/j.jhydrol.2014.10.043Influence of sidewalls on the centerline small-scale turbulence of a turbulent high-aspect-ratio rectangular jet
Liu, Y., Zhang, J., Deo, R., Mi, J., Nathan, G. J. and Zhu, R.. 2014. "Influence of sidewalls on the centerline small-scale turbulence of a turbulent high-aspect-ratio rectangular jet." Experimental Thermal and Fluid Science. 58, pp. 139-144. https://doi.org/10.1016/j.expthermflusci.2014.06.021Effects of spatial resolution on measurement of landscape function using the landscape leakiness calculator
Dunwoody, Ernest, Apan, Armando and Liu, Xiaoye. 2013. "Effects of spatial resolution on measurement of landscape function using the landscape leakiness calculator." Surveying and Spatial Sciences Conference (SSSC 2013): Collect, Connect, Capitalise. Canberra, Australia 17 - 19 Apr 2013 Canberra, Australia.Developing spatial information sharing strategies across natural resource management communities
Paudyal, Dev Raj, McDougall, Kevin and Apan, Armando. 2013. "Developing spatial information sharing strategies across natural resource management communities." Onsrud, Harlan and Rajabifard, Abbas (ed.) Spatial Enablement in Support of Economic Development and Poverty Reduction: Research, Development and Education Perspectives. Needham, MA, USA. GSDI Association Press. pp. 141-168Using spatial modelling to develop flood risk and climate adaptation capacity metrics for vulnerability assessments of urban community and critical water supply infrastructure
Espada Jr., Rodolfo, Apan, Armando and McDougall, Kevin. 2013. "Using spatial modelling to develop flood risk and climate adaptation capacity metrics for vulnerability assessments of urban community and critical water supply infrastructure." Featherstone, Jeffrey (ed.) 49th World Congress of the International Society of City and Regional Planners (ISOCARP 2013): Frontiers of Planning: Evolving and Declining Models of City Planning Practice. Brisbane, Australia 01 - 04 Oct 2013 Netherlands.Adaptation and resilience in two flood-prone Queensland communities
King, David, Apan, Armando, Keogh, Diane and Thomas, Melanie. 2013. "Adaptation and resilience in two flood-prone Queensland communities." Boulter, Sarah, Palutikof, Jean, Karoly, David John and Guitart, Daniella (ed.) Natural disasters and adaptation to climate change. New York, NY. United States. Cambridge University Press. pp. 95-106Comparative analysis of turbulent plane jets from a sharp-edged orifice, a beveled-edge orifice and a radially contoured nozzle
Deo, Ravinesh C.. 2013. "Comparative analysis of turbulent plane jets from a sharp-edged orifice, a beveled-edge orifice and a radially contoured nozzle." International Journal of Mechanical, Industrial Science and Engineering. 7 (12), pp. 1471-1480.Using spatial modelling to develop flood risk and climate adaptation capacity metrics for assessing urban community and critical electricity infrastructure vulnerability
Espada Jr., R., Apan, A. and McDougall, K.. 2013. "Using spatial modelling to develop flood risk and climate adaptation capacity metrics for assessing urban community and critical electricity infrastructure vulnerability." Piantadosi, J., Anderssen, R. S. and Boland, J. (ed.) 20th International Congress on Modelling and Simulation (MODSIM2013). Adelaide, Australia 01 - 06 Dec 2013 Australia. Modelling and Simulation Society of Australia and New Zealand . https://doi.org/10.36334/modsim.2013.L1.espadaThe role of nozzle-exit conditions on the flow field of a plane jet
Deo, Ravinesh C.. 2013. "The role of nozzle-exit conditions on the flow field of a plane jet." International Journal of Mechanical, Industrial Science and Engineering. 7 (12), pp. 1059-1069.Similarity analysis of the momentum field of a subsonic, plane air jet with varying jet-exit and local Reynolds numbers
Deo, Ravinesh C., Nathan, Graham J. and Mi, Jianchun. 2013. "Similarity analysis of the momentum field of a subsonic, plane air jet with varying jet-exit and local Reynolds numbers." Physics of Fluids. 25 (1). https://doi.org/10.1063/1.4776782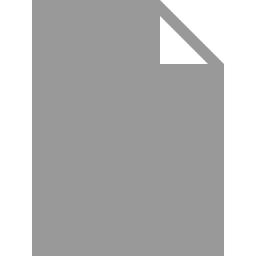