Introductory Engineering Mathematics Students’ Weighted Score Predictions Utilising a Novel Multivariate Adaptive Regression Spline Model
Article
Article Title | Introductory Engineering Mathematics Students’ Weighted Score Predictions Utilising a Novel Multivariate Adaptive Regression Spline Model |
---|---|
ERA Journal ID | 41498 |
Article Category | Article |
Authors | Ahmed, Abul Abrar Masrur (Author), Deo, Ravinesh C. (Author), Ghimire, Sujan (Author), Downs, Nathan J. (Author), Devi, Aruna (Author), Barua, Prabal D. (Author) and Yaseen, Zaher M. (Author) |
Journal Title | Sustainability |
Journal Citation | 14 (17), pp. 1-27 |
Article Number | 11070 |
Number of Pages | 27 |
Year | 2022 |
Publisher | MDPI AG |
Place of Publication | Switzerland |
ISSN | 2071-1050 |
Digital Object Identifier (DOI) | https://doi.org/10.3390/su141711070 |
Web Address (URL) | https://www.mdpi.com/2071-1050/14/17/11070 |
Abstract | Introductory Engineering Mathematics (a skill builder for engineers) involves developing problem-solving attributes throughout the teaching period. Therefore, the prediction of students’ final course grades with continuous assessment marks is a useful toolkit for degree program educators. Predictive models are practical tools used to evaluate the effectiveness of teaching as well as assessing the students’ progression and implementing interventions for the best learning outcomes. This study develops a novel multivariate adaptive regression spline (MARS) model to predict the weighted score WS (i.e., the course grade). To construct the proposed MARS model, Introductory Engineering Mathematics performance data over five years from the University of Southern Queensland, Australia, were used to design predictive models using input predictors of online quizzes, written assignments, and examination scores. About 60% of randomised predictor grade data were applied to train the model (with 25% of the training set used for validation) and 40% to test the model. Based on the cross-correlation of inputs vs. the WS, 12 distinct combinations with single (i.e., M1–M5) and multiple (M6–M12) features were created to assess the influence of each on the WS with results bench-marked via a decision tree regression (DTR), kernel ridge regression (KRR), and a k-nearest neighbour (KNN) model. The influence of each predictor on WS clearly showed that online quizzes provide the least contribution. However, the MARS model improved dramatically by including written assignments and examination scores. The research demonstrates the merits of the proposed MARS model in uncovering relationships among continuous learning variables, which also provides a distinct advantage to educators in developing early intervention and moderating their teaching by predicting the performance of students ahead of final outcome for a course. The findings and future application have significant practical implications in teaching and learning interventions or planning aimed to improve graduate outcomes in undergraduate engineering program cohorts. |
Keywords | educational decision making; multivariate regression spline model; student performance; artificial intelligence in education; engineering mathematics student performance |
ANZSRC Field of Research 2020 | 460207. Modelling and simulation |
461106. Semi- and unsupervised learning | |
390303. Higher education | |
Institution of Origin | University of Southern Queensland |
Byline Affiliations | School of Mathematics, Physics and Computing |
School of Mathematics, Physics and Computing | |
University of the Sunshine Coast | |
School of Business |
https://research.usq.edu.au/item/q7qx4/introductory-engineering-mathematics-students-weighted-score-predictions-utilising-a-novel-multivariate-adaptive-regression-spline-model
Download files
Published Version
sustainability-14-11070-Published Copy.pdf | ||
License: CC BY 4.0 | ||
File access level: Anyone |
185
total views47
total downloads3
views this month0
downloads this month
Export as
Related outputs
Multi‑step solar ultraviolet index prediction: integrating convolutional neural networks with long short‑term memory for a representative case study in Queensland, Australia
AL-Musaylh, Mohan, Al‑Dafaie, Kadhem, Downs, Nathan, Ghimire, Sujan, Ali, Mumtaz, Yaseen, Zaher Mundher, Igoe, Damien P., Deo, Ravinesh C., Paris, Alfo V. and A. Jebar, Mustapha A.. 2025. "Multi‑step solar ultraviolet index prediction: integrating convolutional neural networks with long short‑term memory for a representative case study in Queensland, Australia." Modeling Earth Systems and Environment. 11. https://doi.org/https://link.springer.com/article/10.1007/s40808-024-02282-yArtificial Intelligence-Based Suicide Prevention and Prediction: A Systematic Review (2019-2023)
Atmakuru, Anirudh, Shahini, Alen, Chakraborty, Subrata, Seoni, Silvia, Salvi, Massimo, Hafeez-Baig, Abdul, Rashid, Sadaf, Tan, Ru San, Barua, Prabal Datta, Molinari, Filippo and Acharya, U Rajendra. 2025. "Artificial Intelligence-Based Suicide Prevention and Prediction: A Systematic Review (2019-2023)." Information Fusion. 114. https://doi.org/10.1016/j.inffus.2024.102673Explainable deeply-fused nets electricity demand prediction model: Factoring climate predictors for accuracy and deeper insights with probabilistic confidence interval and point-based forecasts
Ghimire, Sujan, AL-Musaylh, Mohanad S., Nguyen-Huy, Thong, Deo, Ravinesh C., Acharya, Rajendra, Casillas-Perez, David, Yaseen, Zaher Mundher and Salcedo-sanz, Sancho. 2025. "Explainable deeply-fused nets electricity demand prediction model: Factoring climate predictors for accuracy and deeper insights with probabilistic confidence interval and point-based forecasts." Applied Energy. 378 (Part A). https://doi.org/10.1016/j.apenergy.2024.124763Tree shade within urban parks and nature strips: standards and perspectives from measurement campaigns conducted in Queensland
Downs, Nathan, Antrobus, Jodie, Dekeyser, Stijn, Butler, Harry, Downs, Melanie, Hickmott, Darren, Madigan, Chris, Brown, Alexandra, Amar, Abdurazaq, Dearnaley, John, Parisi, Alfio V, Vanos, Jennifer, Raj, Nawin, Harrison, Simone and Igoe, Damien. 2024. "Tree shade within urban parks and nature strips: standards and perspectives from measurement campaigns conducted in Queensland ." 6th International conference UV and Skin Cancer Prevention. Brisbane, Australia 11 - 15 Sep 2024 Australia.A Comprehensive Review of UAV-UGV Collaboration: Advancements and Challenges
Munasinghe, Isuru, Perera, Asanka and Deo, Ravinesh C.. 2024. "A Comprehensive Review of UAV-UGV Collaboration: Advancements and Challenges." Journal of Sensor and Actuator Networks. 13 (6). https://doi.org/10.3390/jsan13060081Directed Lobish-based explainable feature engineering model with TTPat and CWINCA for EEG artifact classification
Tuncer, Turker, Dogan, Sengul, Baygin, Mehmet, Tasci, Irem, Mungen, Bulent, Tasci, Burak, Barua, Prabal Datta and Acharya, U.R.. 2024. "Directed Lobish-based explainable feature engineering model with TTPat and CWINCA for EEG artifact classification." Knowledge-Based Systems. 305. https://doi.org/10.1016/j.knosys.2024.112555Retinal Health Screening Using Artificial Intelligence with Digital Fundus Images: A Review of the Last Decade (2012-2023)
Islam, Saad, Deo, Ravinesh C., Barua, Prabal Datta, Soar, Jeffrey, Yu, Ping and Acharya, U. Rajendra. 2024. "Retinal Health Screening Using Artificial Intelligence with Digital Fundus Images: A Review of the Last Decade (2012-2023)." IEEE Access. 12, pp. 176630-176685. https://doi.org/10.1109/ACCESS.2024.3477420Automated EEG-based language detection using directed quantum pattern technique
Dogan, Sengul, Tuncer, Turker, Barua, Prabal Datta and Acharya, U.R.. 2024. "Automated EEG-based language detection using directed quantum pattern technique." Applied Soft Computing. 167 (Part A). https://doi.org/10.1016/j.asoc.2024.112301Forecasting River Water Temperature Using Explainable Artificial Intelligence and Hybrid Machine Learning: Case Studies in Menindee Region in Australia
Briceno Medina, Leyde, Joehnk, Klaus, Deo, Ravinesh C., Ali, Mumtaz, Prasad, Salvin S. and Downs, Nathan. 2024. "Forecasting River Water Temperature Using Explainable Artificial Intelligence and Hybrid Machine Learning: Case Studies in Menindee Region in Australia." Water. 16 (24). https://doi.org/10.3390/w16243720Automated Detection of Gastrointestinal Diseases Using Resnet50*-Based Explainable Deep Feature Engineering Model with Endoscopy Images
Cambay, Veysel Yusu, Barua, Prabal Dat, Hafeez Baig, Abdul, Dogan, Sengul, Baygin, Mehmet, Tuncer, Turker and Acharya, U. R.. 2024. "Automated Detection of Gastrointestinal Diseases Using Resnet50*-Based Explainable Deep Feature Engineering Model with Endoscopy Images ." Sensors. 24 (23). https://doi.org/10.3390/s24237710Explainable artificial intelligence-machine learning models to estimate overall scores in tertiary preparatory general science course
Ghimire, Sujan, Abdulla, Shahab, Joseph, Lionel P., Prasad, Salvin, Murphy, Angela, Devi, Aruna, Barua, Prabal Datta, Deo, Ravinesh C., Acharya, Rajendra and Yaseen, Zaher Mundher. 2024. "Explainable artificial intelligence-machine learning models to estimate overall scores in tertiary preparatory general science course." Computers and Education: Artificial Intelligence. 7. https://doi.org/10.1016/j.caeai.2024.100331Forecasting Multi-Step Soil Moisture with Three-Phase Hybrid Wavelet-Least Absolute Shrinkage Selection Operator-Long Short-Term Memory Network (moDWT-Lasso-LSTM) Model
Jayasinghe, W. J. M. Lakmini Prarthana, Deo, Ravinesh C., Raj, Nawin, Ghimire, Sujan, Yaseen, Zaher Mundher, Nguyen-Huy, Thong and Ghahramani, Afshin. 2024. "Forecasting Multi-Step Soil Moisture with Three-Phase Hybrid Wavelet-Least Absolute Shrinkage Selection Operator-Long Short-Term Memory Network (moDWT-Lasso-LSTM) Model." Water. 16 (21), p. 3133. https://doi.org/10.3390/w16213133Real-time prediction of the week-ahead flood index using hybrid deep learning algorithms with synoptic climate mode indices
Ahmed, A.A. Masrur, Akther, Shahida, Nguyen-Huy, Thong, Raj, Nawin, Jui (Student), S Janifer and S.Z., Farzana. 2024. "Real-time prediction of the week-ahead flood index using hybrid deep learning algorithms with synoptic climate mode indices." Journal of Hydro-Environment Research. 57, pp. 12-26. https://doi.org/10.1016/j.jher.2024.09.001Ultraviolet radiation thin film dosimetry: A review of properties and applications
Parisi, Alfio V., Downs, Nathan J., Schouten, Peter, Igoe, Damien P., Turner, Joanna, Amar, Abdurazaq, Wainwright, Lisa, Dawes, Adrian, Butler, Harry and Dekeyser, Stijn. 2024. "Ultraviolet radiation thin film dosimetry: A review of properties and applications." Photochemistry and Photobiology. https://doi.org/10.1111/php.14022Lobish: Symbolic Language for Interpreting Electroencephalogram Signals in Language Detection Using Channel-Based Transformation and Pattern
Tuncer, Turker, Dogan, Sengul, Tasci, Irem, Baygin, Mehmet, Barua, Prabal and Acharya, U. Rajendra. 2024. "Lobish: Symbolic Language for Interpreting Electroencephalogram Signals in Language Detection Using Channel-Based Transformation and Pattern." Diagnostics. 14 (17). https://doi.org/10.3390/diagnostics14171987Artificial Intelligence-Empowered Doppler Weather Profile for Low-Earth-Orbit Satellites
Sharma, Ekta, Deo, Ravinesh C., Davey, Christopher P and Carter, Brad D.. 2024. "Artificial Intelligence-Empowered Doppler Weather Profile for Low-Earth-Orbit Satellites." Sensors. 24 (16). https://doi.org/10.3390/s24165271ADHD/CD-NET: automated EEG-based characterization of ADHD and CD using explainable deep neural network technique
Loh, Hui Wen, Ooi, Chui Ping, Oh, Shu Lih, Barua, Prabal Datta, Tan, Yi Ren, Acharya, U. Rajendra and Fung, Daniel Shuen Sheng. 2024. "ADHD/CD-NET: automated EEG-based characterization of ADHD and CD using explainable deep neural network technique." Cognitive Neurodynamics. 18 (4), pp. 1609-1625. https://doi.org/10.1007/s11571-023-10028-2Wavelet-fusion image super-resolution model with deep learning for downscaling remotely-sensed, multi-band spectral albedo imagery
Karalasingham, Sagthitharan, Deo, Ravinesh C., Casillas-Perez, David, Raj, Nawin and Salcedo-sanz, Sancho. 2024. "Wavelet-fusion image super-resolution model with deep learning for downscaling remotely-sensed, multi-band spectral albedo imagery." Remote Sensing Applications: Society and Environment. 36. https://doi.org/10.1016/j.rsase.2024.101333Half-hourly electricity price prediction with a hybrid convolution neural network-random vector functional link deep learning approach
Ghimire, Sujan, Deo, Ravinesh C., Casillas-Perez, David, Sharma, Ekta, Salcedo-sanz, Sancho, Barua, Prabal and Acharya, U. Rajendra. 2024. "Half-hourly electricity price prediction with a hybrid convolution neural network-random vector functional link deep learning approach." Applied Energy. 374. https://doi.org/10.1016/j.apenergy.2024.123920ConcatNeXt: An automated blood cell classification with a new deep convolutional neural network
Erten, Mehmet, Barua, Prabal Datta, Dogan, Sengul, Tuncer, Turker, Tan, Ru‑San and Acharya, U. R.. 2024. "ConcatNeXt: An automated blood cell classification with a new deep convolutional neural network." Multimedia Tools and Applications. https://doi.org/10.1007/s11042-024-19899-xN-BodyPat: Investigation on the dementia and Alzheimer's disorder detection using EEG signals
Barua, Prabal Datta, Tuncer, Turker, Baygin, Mehmet, Dogan, Sengul and Acharya, U. Rajendra. 2024. "N-BodyPat: Investigation on the dementia and Alzheimer's disorder detection using EEG signals." Knowledge-Based Systems. 304. https://doi.org/10.1016/j.knosys.2024.112510Deep learning in radiology for lung cancer diagnostics: A systematic review of classification, segmentation, and predictive modeling techniques
Atmakuru, Anirudh, Chakraborty, Subrata, Faust, Oliver, Salvi, Massimo, Barua, Prabal Datta, Molinari, Filippo, Acharya, U.R. and Homaira, Nusrat. 2024. "Deep learning in radiology for lung cancer diagnostics: A systematic review of classification, segmentation, and predictive modeling techniques." Expert Systems with Applications. 255 (Part B). https://doi.org/10.1016/j.eswa.2024.124665Towards next-generation federated learning: A case study on privacy attacks in artificial intelligence systems
Sharma, Ekta, Deo, Ravinesh C, Davey, Christopher P., Carter, Brad D. and Salcedo-sanz, Sancho. 2024. "Towards next-generation federated learning: A case study on privacy attacks in artificial intelligence systems." 2024 IEEE Conference on Artificial Intelligence (CAI 2024). Singapore 25 - 27 Jun 2024 United States. IEEE (Institute of Electrical and Electronics Engineers). https://doi.org/10.1109/CAI59869.2024.00259Poster: Cloud Computing with AI-empowered Trends in Software-Defined Radios: Challenges and Opportunities
Sharma, Ekta, Deo, Ravinesh C., Davey, Christopher P., Carter, Brad D. and Salcedo-sanz, Sancho. 2024. "Poster: Cloud Computing with AI-empowered Trends in Software-Defined Radios: Challenges and Opportunities." 2024 IEEE 25th International Symposium on a World of Wireless, Mobile and Multimedia Networks (WoWMoM 2024). Perth, Australia 04 - 07 Jun 2024 United States. IEEE (Institute of Electrical and Electronics Engineers). https://doi.org/10.1109/WoWMoM60985.2024.00054Barriers to Access and Participation in Higher Education: The Case for a “Bounded Agency” Perspective
Sherwood, Celeste, Green, Jonathan H., Davis, Charmaine and Devi, Aruna. 2024. "Barriers to Access and Participation in Higher Education: The Case for a “Bounded Agency” Perspective." Padro, Fernando F., Green, Jonathan H. and Bull, David (ed.) Widening Participation in Higher Education. Singapore. Springer. pp. 1-26Prediction of Sea Level Using Double Data Decomposition and Hybrid Deep Learning Model for Northern Territory, Australia
Raj, Nawin, Murali, Jaishukh, Singh-Peterson, Lila and Downs, Nathan. 2024. "Prediction of Sea Level Using Double Data Decomposition and Hybrid Deep Learning Model for Northern Territory, Australia." Mathematics. 12 (15). https://doi.org/10.3390/math12152376Lattice 123 pattern for automated Alzheimer's detection using EEG signal
Dogan, Sengul, Barua, Prabal Datta, Baygin, Mehmet, Tuncer, Turker, Tan, Ru-San, Ciaccio, Edward J., Fujita, Hamido, Devi, Aruna and Acharya, U. Rajendra. 2024. "Lattice 123 pattern for automated Alzheimer's detection using EEG signal." Cognitive Neurodynamics. 18, pp. 2503-2519. https://doi.org/10.1007/s11571-024-10104-1FLP: Factor lattice pattern-based automated detection of Parkinson's disease and specific language impairment using recorded speech
Tuncer, Turker, Dogan, Sengul, Baygin, Mehmet, Barua, Prabal Datta, Palmer, Elizabeth Emma, March, Sonja, Ciaccio, Edward J., Tan, Ru-San and Acharya, U. Rajendra. 2024. "FLP: Factor lattice pattern-based automated detection of Parkinson's disease and specific language impairment using recorded speech." Computers in Biology and Medicine. 173. https://doi.org/10.1016/j.compbiomed.2024.108280A lightweight deep convolutional neural network model for skin cancer image classification
Tuncer, Turker, Barua, Prabal Datta, Tuncer, Ilknur, Dogan, Sengul and Acharya, U. Rajendra. 2024. "A lightweight deep convolutional neural network model for skin cancer image classification." Applied Soft Computing. 162. https://doi.org/10.1016/j.asoc.2024.111794Atmospheric Visibility and Cloud Ceiling Predictions With Hybrid IIS-LSTM Integrated Model: Case Studies for Fiji's Aviation Industry
Raj, Shiveel, Deo, Ravinesh C., Sharma, Ekta, Prasad, Ramendra, Dinh, Toan and Salcedo-sanz, Sancho. 2024. "Atmospheric Visibility and Cloud Ceiling Predictions With Hybrid IIS-LSTM Integrated Model: Case Studies for Fiji's Aviation Industry." IEEE Access. 12, pp. 72530-72543. https://doi.org/10.1109/ACCESS.2024.3401091DSWIN: Automated hunger detection model based on hand-crafted decomposed shifted windows architecture using EEG signals
Kirik, Serkan, Tasci, Irem, Barua, Prabal D., Yildiz, Arif Metehan, Keles, Tugce, Baygin, Mehmet, Tuncer, Ilknur, Dogan, Sengul, Tuncer, Turker, Devi, Aruna, Tan, Ru-San and Acharya, U.R.. 2024. "DSWIN: Automated hunger detection model based on hand-crafted decomposed shifted windows architecture using EEG signals." Knowledge-Based Systems. 300. https://doi.org/10.1016/j.knosys.2024.112150Automatic identification of hypertension and assessment of its secondary effects using artificial intelligence: A systematic review (2013–2023)
Gudigar, Anjan, Kadri, Nahrizul Adib, Raghavendra, U., Samanth, Jyothi, Maithri, M., Inamdar, Mahesh Anil, Prabhu, Mukund A., Hegde, Ajay, Salvi, Massimo, Yeong, Chai Hong, Barua, Prabal Datta, Molinari, Filippo and Acharya, U. Rajendra. 2024. "Automatic identification of hypertension and assessment of its secondary effects using artificial intelligence: A systematic review (2013–2023)." Computers in Biology and Medicine. 172. https://doi.org/10.1016/j.compbiomed.2024.108207Point-based and probabilistic electricity demand prediction with a Neural Facebook Prophet and Kernel Density Estimation model
Ghimire, Sujan, Deo, Ravinesh C., Pourmousavi, S. Ali, Casillas-Perez, David and Salcedo-sanz, Sancho. 2024. "Point-based and probabilistic electricity demand prediction with a Neural Facebook Prophet and Kernel Density Estimation model." Engineering Applications of Artificial Intelligence. 135. https://doi.org/10.1016/j.engappai.2024.108702An accurate hypertension detection model based on a new odd-even pattern using ballistocardiograph signals
Dogan, Sengul, Barua, Prabal Datta, Tuncer, Turker and Acharya, U. Rajendra. 2024. "An accurate hypertension detection model based on a new odd-even pattern using ballistocardiograph signals." Engineering Applications of Artificial Intelligence. 133 (Part D). https://doi.org/10.1016/j.engappai.2024.108306End-to-end learning of adaptive coded modulation schemes for resilient wireless communications
Davey, Christopher P., Shakeel, Ismail, Deo, Ravinesh C., Sharma, Ekta, Salcedo-sanz, Sancho and Soar, Jeffrey. 2024. "End-to-end learning of adaptive coded modulation schemes for resilient wireless communications." Applied Soft Computing. 159. https://doi.org/10.1016/j.asoc.2024.111672Deep Learning Based Over-the-Air Training of Wireless Communication Systems without Feedback
Davey, Christopher P., Shakeel, Ismail, Deo, Ravinesh C. and Salcedo-sanz, Sancho. 2024. "Deep Learning Based Over-the-Air Training of Wireless Communication Systems without Feedback." Sensors. 24 (10). https://doi.org/10.3390/s24102993Automated asthma detection in a 1326-subject cohort using a one-dimensional attractive-and-repulsive center-symmetric local binary pattern technique with cough sounds
Barua, Prabal Datta, Keles, Tugce, Kuluozturk, Mutlu, Kobat, Mehmet Ali, Dogan, Sengul, Baygin, Mehmet, Tuncer, Turker, Tan, Ru-San and Acharya, U. Rajendr. 2024. "Automated asthma detection in a 1326-subject cohort using a one-dimensional attractive-and-repulsive center-symmetric local binary pattern technique with cough sounds." Neural Computing and Applications. 36 (27), pp. 16857-16871. https://doi.org/10.1007/s00521-024-09895-5AttentionPoolMobileNeXt: An automated construction damage detection model based on a new convolutional neural network and deep feature engineering models
Aydin, Mehmet, Barua, Prabal Datta, Chadalavada, Sreenivasulu, Dogan, Sengul, Tuncer, Turker, Chakraborty, Subrata and Acharya, Rajendra U.. 2024. "AttentionPoolMobileNeXt: An automated construction damage detection model based on a new convolutional neural network and deep feature engineering models." Multimedia Tools and Applications. https://doi.org/10.1007/s11042-024-19163-2Automated reading level classification model based on improved orbital pattern
Abed, Rusul Qasim, Dikmen, Melih, Aydemir, Emrah, Barua, Prabal Datta, Dogan, Sengul, Tuncer, Turker, Palmer, Elizabeth Emma, Ciaccio, Edward J. and Acharya, U. Rajendra. 2024. "Automated reading level classification model based on improved orbital pattern." Multimedia Tools and Applications. 83 (17), pp. 52819-52840. https://doi.org/10.1007/s11042-023-17535-8A playground shade index for standardising ultraviolet protection assessments of open, mixed and protected outdoor recreational spaces
Downs, Nathan, Butler, Harry, Dexter, Benjamin, Raj, Nawin, Downs, Melanie, Turner, Joanna, Dekeyser, Stijn, Deo, Ravinesh, Vanos, Jennifer, Igoe, Damien and Parisi, Alfio V. 2024. "A playground shade index for standardising ultraviolet protection assessments of open, mixed and protected outdoor recreational spaces." 6th International conference UV and Skin Cancer Prevention. Brisbane, Australia 11 - 15 Sep 2024 Australia.The mitigating effect of street trees, urban flora, and the suburban environment on seasonal peak UV indices: A case study from Brisbane, Australia
Downs, Nathan James, Amar, Abdurazaq, Dearnaley, John, Butler, Harry, Dekeyser, Stijn, Igoe, Damien, Parisi, Alfio V., Raj, Nawin, Deo, Ravinesh and Turner, Joanna. 2024. "The mitigating effect of street trees, urban flora, and the suburban environment on seasonal peak UV indices: A case study from Brisbane, Australia." Photochemistry and Photobiology. https://doi.org/10.1111/php.13988UV albedo and reflectance: is it possible to predict erythemal reflectance from solar visible reflectance with respect to the type of material?
Turner, Joanna, Parisi, Alfio V, Amar, Abdurazaq and Downs, Nathan. 2024. "UV albedo and reflectance: is it possible to predict erythemal reflectance from solar visible reflectance with respect to the type of material?" 6th International conference UV and Skin Cancer Prevention. Brisbane, Australia 11 - 15 Sep 2024 Australia.A legacy of more than two decades of UV radiation measurement studies at the University of Southern Queensland
Amar, Abdurazaq, Parisi, Alfio, Downs, Nathan, Turner, Joanna, Igoe, Damien, Butler, Harry and Dekeyser, Stijn. 2024. "A legacy of more than two decades of UV radiation measurement studies at the University of Southern Queensland." 6th International conference UV and Skin Cancer Prevention. Brisbane, Australia 11 - 15 Sep 2024 Australia.Multi-Step-Ahead Wind Speed Forecast System: Hybrid Multivariate Decomposition and Feature Selection-Based Gated Additive Tree Ensemble Model
Joseph, Lionel P., Deo, Ravinesh C., Casillas-Perez, David, Prasad, Ramendra, Raj, Nawin and Salcedo-sanz, Sancho. 2024. "Multi-Step-Ahead Wind Speed Forecast System: Hybrid Multivariate Decomposition and Feature Selection-Based Gated Additive Tree Ensemble Model." IEEE Access. 12, pp. 58750-58777. https://doi.org/10.1109/ACCESS.2024.3392899Deep Learning-Assisted Sensitive 3C-SiC Sensor for Long-Term Monitoring of Physical Respiration
Tran, Thi Lap, Nguyen, Duy Van, Nguyen, Hung, Nguyen, Thi Phuoc Van, Song, Pingan, Deo, Ravinesh C, Moloney, Clint, Dao, Viet Dung, Nguyen, Nam-Trung and Dinh, Toan. 2024. "Deep Learning-Assisted Sensitive 3C-SiC Sensor for Long-Term Monitoring of Physical Respiration." Advanced Sensor Research. 3 (8). https://doi.org/10.1002/adsr.202300159Copula-Probabilistic Flood Risk Analysis with an Hourly Flood Monitoring Index
Chand, Ravinesh, Nguyen-Huy, Thong, Deo, Ravinesh C., Ghimire, Sujan, Ali, Mumtaz and Ghahramani, Afshin. 2024. "Copula-Probabilistic Flood Risk Analysis with an Hourly Flood Monitoring Index." Water. 16 (11). https://doi.org/10.3390/w16111560Electronic sun journal as an evaluation tool for measuring sunflecks while walking through urban forests
Igoe, Damien P., Parisi, Alfio V, Amar, Abdurazaq, Downs, Nathan J. and Butler, Harry. 2024. "Electronic sun journal as an evaluation tool for measuring sunflecks while walking through urban forests." Instrumentation Science and Technology. https://doi.org/10.1080/10739149.2024.2350009Black-white hole pattern: an investigation on the automated chronic neuropathic pain detection using EEG signals
Tasci, Irem, Baygin, Mehmet, Barua, Prabal Datta, Hafeez-Baig, Abdul, Dogan, Sengul, Tuncer, Turker, Tan, Ru-San and Acharya, U. Rajendra. 2024. "Black-white hole pattern: an investigation on the automated chronic neuropathic pain detection using EEG signals." Cognitive Neurodynamics. 18 (5), pp. 2193-2210. https://doi.org/10.1007/s11571-024-10078-0Application of spatial uncertainty predictor in CNN-BiLSTM model using coronary artery disease ECG signals
Seoni, Silvia, Molinari, Filippo, Acharya, U. Rajendra, Oh, Oh Shu, Barua, Prabal Datta, García, Salvador and Salvi, Massimo. 2024. "Application of spatial uncertainty predictor in CNN-BiLSTM model using coronary artery disease ECG signals." Information Sciences. 665. https://doi.org/10.1016/j.ins.2024.120383Multi-modality approaches for medical support systems: A systematic review of the last decade
Salvi, Massimo, Loh, Hui Wen, Seoni, Silvia, Barua, Prabal Datta, García, Salvador, Molinari, Filippo and Acharya, U. Rajendra. 2024. "Multi-modality approaches for medical support systems: A systematic review of the last decade." Information Fusion. 103. https://doi.org/10.1016/j.inffus.2023.102134Artificial intelligence for atrial fibrillation detection, prediction, and treatment: A systematic review of the last decade (2013–2023)
Salvi, Massimo, Acharya, Madhav R., Seoni, Silvia, Faust, Oliver, Tan, Ru-San, Barua, Prabal Datta, García, Salvador, Molinari, Filippo and Acharya, U. Rajendra Acharya. 2024. "Artificial intelligence for atrial fibrillation detection, prediction, and treatment: A systematic review of the last decade (2013–2023)." WIREs Data Mining and Knowledge Discovery. 14 (3). https://doi.org/10.1002/widm.1530An automated earthquake classification model based on a new butterfly pattern using seismic signals
Ozkaya, Suat Gokhan, Baygin, Mehmet, Barua, Prabal Datta, Tuncer, Turker, Dogan, Sengul, Chakraborty, Subrata and Acharya, U. Rajendra. 2024. "An automated earthquake classification model based on a new butterfly pattern using seismic signals." Expert Systems with Applications. 238 (Part D). https://doi.org/10.1016/j.eswa.2023.122079GCLP: An automated asthma detection model based on global chaotic logistic pattern using cough sounds
Kilic, Mehmet, Barua, Prabal Datta, Keles, Tugce, Yildiz, Arif Metehan, Tuncer, Ilknur, Dogan, Sengul, Baygin, Mehmet, Tuncer, Turker, Kuluozturk, Mutlu, Tan, Ru-San and Acharya, U. Rajendra. 2024. "GCLP: An automated asthma detection model based on global chaotic logistic pattern using cough sounds." Engineering Applications of Artificial Intelligence. 127 (Part A). https://doi.org/10.1016/j.engappai.2023.107184Short-term wind speed forecasting using an optimized three-phase convolutional neural network fused with bidirectional long short-term memory network model
Joseph, Lionel P., Deo, Ravinesh C., Casillas-Perez, David, Prasad, Ramendra, Raj, Nawin and Salcedo-sanz, Sancho. 2024. "Short-term wind speed forecasting using an optimized three-phase convolutional neural network fused with bidirectional long short-term memory network model." Applied Energy. 359. https://doi.org/10.1016/j.apenergy.2024.122624Probabilistic-based electricity demand forecasting with hybrid convolutional neural network-extreme learning machine model
Ghimire, Sujan, Deo, Ravinesh C., Casillas-Perez, David, Salcedo-sanz, Sancho, Pourmousavi, S. Ali and Acharya, U. Rajendra. 2024. "Probabilistic-based electricity demand forecasting with hybrid convolutional neural network-extreme learning machine model." Engineering Applications of Artificial Intelligence. 132. https://doi.org/10.1016/j.engappai.2024.107918Electricity demand error corrections with attention bi-directional neural networks
Ghimire, Sujan, Deo, Ravinesh C., Casillas-Perez, David and Salcedo-sanz, Sancho. 2024. "Electricity demand error corrections with attention bi-directional neural networks." Energy. 291. https://doi.org/10.1016/j.energy.2023.129938Deep learning techniques in PET/CT imaging: A comprehensive review from sinogram to image space
Fallahpoor, Maryam, Chakraborty, Subrata, Pradhan, Biswajeet, Faust, Oliver, Barua, Prabal Datta, Chegeni, Hossein and Acharya, Rajendra. 2024. "Deep learning techniques in PET/CT imaging: A comprehensive review from sinogram to image space." Computer Methods and Programs in Biomedicine. 243. https://doi.org/10.1016/j.cmpb.2023.107880Novel tiny textural motif pattern-based RNA virus protein sequence classification model
Erten, Mehmet, Aydemir, Emrah, Barua, Prabal Datta, Baygin, Mehmet, Dogan, Sengul, Tuncer, Turker, Tan, Ru-San, Hafeez-Baig, Abdul and Acharya, U. Rajendra. 2024. "Novel tiny textural motif pattern-based RNA virus protein sequence classification model." Expert Systems with Applications. 242. https://doi.org/10.1016/j.eswa.2023.122781Micromachined Mechanical Resonant Sensors: From Materials, Structural Designs to Applications
Dinh, Toan, Rais-Zadeh, Mina, Nguyen, Thanh, Phan, Hoang-Phuong, Song, Pingan, Deo, Ravinesh, Dao, Dzung, Nguyen, Nam-Trung and Bell, John. 2024. "Micromachined Mechanical Resonant Sensors: From Materials, Structural Designs to Applications." Advanced Materials Technologies. 9 (2). https://doi.org/10.1002/admt.202300913MNPDenseNet: Automated Monkeypox Detection Using Multiple Nested Patch Division and Pretrained DenseNet201
Demir, Fahrettin Burak, Baygin, Mehmet, Tuncer, Ilknur, Barua, Prabal Datta, Dogan, Sengul, Tuncer, Turker, Ooi, Chui Ping, Ciaccio, Edward J. and Acharya, U. Rajendra. 2024. "MNPDenseNet: Automated Monkeypox Detection Using Multiple Nested Patch Division and Pretrained DenseNet201." Multimedia Tools and Applications. 83 (30), pp. 75061-75083. https://doi.org/10.1007/s11042-024-18416-4Correction to: Automated facial expression recognition using exemplar hybrid deep feature generation technique
Baygin, Mehmet, Tuncer, Ilknur, Dogan, Sengul, Barua, Prabal Datta, Tuncer, Turker, Cheong, Kang Hao and Acharya, U. Rajendra. 2024. "Correction to: Automated facial expression recognition using exemplar hybrid deep feature generation technique ." Soft Computing. https://doi.org/10.1007/s00500-024-09647-6Automated anxiety detection using probabilistic binary pattern with ECG signals
Baygin, Mehmet, Barua, Prabal Datta, Dogan, Sengul, Tuncer, Turker, Hong, Tan Jen, March, Sonja, Tan, Ru-San, Molinari, Filippo and Acharya, U. Rajendra. 2024. "Automated anxiety detection using probabilistic binary pattern with ECG signals." Computer Methods and Programs in Biomedicine. 247. https://doi.org/10.1016/j.cmpb.2024.108076MixSleepNet: A Multi-Type Convolution Combined Sleep Stage Classification Model
Ji, Xiaopeng, Li, Yan, Wen, Peng, Barua, Prabal and Acharya, U Rajendra. 2024. "MixSleepNet: A Multi-Type Convolution Combined Sleep Stage Classification Model ." Computer Methods and Programs in Biomedicine. 244. https://doi.org/10.1016/j.cmpb.2023.107992Machine learning for expediting next-generation of fire-retardant polymer composites
Jafari, Pooya, Zhang, Ruoran, Huo, Siqi, Wang, Qingsheng, Yong, Jianming, Hong, Min, Deo, Ravinesh, Wang, Hao and Song, Pingan. 2024. "Machine learning for expediting next-generation of fire-retardant polymer composites." Composites Communications. 45. https://doi.org/10.1016/j.coco.2023.101806Very short-term solar ultraviolet-A radiation forecasting system with cloud cover images and a Bayesian optimized interpretable artificial intelligence model
Prasad, Salvin Sanjesh, Deo, Ravinesh Chand, Downs, Nathan James, Casillas-Perez, David, Salcedo-sanz, Sancho and Parisi, Alfio Venerando. 2024. "Very short-term solar ultraviolet-A radiation forecasting system with cloud cover images and a Bayesian optimized interpretable artificial intelligence model ." Expert Systems with Applications. 236. https://doi.org/10.1016/j.eswa.2023.121273Short-term wave power forecasting with hybrid multivariate variational mode decomposition model integrated with cascaded feedforward neural networks
Ali, Mumtaz, Prasad, Ramendra, Jamei, Mehdi, Malik, Anurag, Xiang, Yong, Abdulla, Shahab, Deo, Ravinesh C., Farooque, Aitazaz A. and Labban, Abdulhaleem H.. 2024. "Short-term wave power forecasting with hybrid multivariate variational mode decomposition model integrated with cascaded feedforward neural networks." Renewable Energy. 221. https://doi.org/10.1016/j.renene.2023.119773Hybridized artificial intelligence models with nature-inspired algorithms for river flow modeling: A comprehensive review, assessment, and possible future research directions
Tao, Hai, Abba, Sani I., Al-Areeq, Ahmed M., Tangang, Fredolin, Samantaray, Sandeep, Sahoo, Abinash, Siqueira, Hugo Valadares, Maroufpoor, Saman, Demir, Vahdettin, Bokde, Neeraj Dhanraj, Goliatt, Leonardo, Jamei, Mehdi, Ahmadianfar, Iman, Bhagat, Suraj Kumar, Halder, Bijay, Guo, Tianli, Helman, Daniel S., Ali, Mumtaz, Sattar, Sabaa, ..., Yaseen, Zaher Mundher. 2024. "Hybridized artificial intelligence models with nature-inspired algorithms for river flow modeling: A comprehensive review, assessment, and possible future research directions ." Engineering Applications of Artificial Intelligence. 129. https://doi.org/10.1016/j.engappai.2023.107559Two-step deep learning framework with error compensation technique for short-term, half-hourly electricity price forecasting
Ghimire, Sujan, Deo, Ravinesh C., Casillas-Perez, David and Salcedo-sanz, Sancho. 2024. "Two-step deep learning framework with error compensation technique for short-term, half-hourly electricity price forecasting." Applied Energy. 353 (Part A). https://doi.org/10.1016/j.apenergy.2023.122059Automated schizophrenia detection model using blood sample scattergram images and local binary pattern
Tasci, Burak, Tasci, Gulay, Ayyildiz, Hakan, Kamath, Aditya P., Barua, Prabal Datta, Tuncer, Turker, Dogan, Sengul, Ciaccio, Edward J., Chakraborty, Subrata . and Acharya, U. Rajendra. 2024. "Automated schizophrenia detection model using blood sample scattergram images and local binary pattern." Multimedia Tools and Applications. 83 (14), p. 42735–42763. https://doi.org/10.1007/s11042-023-16676-0Teachers' Educational Experiences and Preparedness in Teaching Students with Autism
Barua, Prabal Datta, Devi, Aruna, Palmer, Elizabeth Emma and Ganguly, Rahul. 2024. "Teachers' Educational Experiences and Preparedness in Teaching Students with Autism." The Asia-Pacific Education Researcher. 33 (1), pp. 71-81. https://doi.org/10.1007/s40299-022-00709-7An advanced deep learning predictive model for air quality index forecasting with remote satellite-derived hydro-climatological variables
Ahmed, Abul Abrar Masrur, Jui, S. Janifer Jabin, Sharma, Ekta, Ahmed, Mohammad Hafez, Raj, Nawin and Bose, Aditi. 2024. "An advanced deep learning predictive model for air quality index forecasting with remote satellite-derived hydro-climatological variables." Science of the Total Environment. 906. https://doi.org/10.1016/j.scitotenv.2023.167234Hybrid Deep Learning Model for Wave Height Prediction in Australia's Wave Energy Region
Ahmed, Abul Abrar Masrur, Jui, S Janifer Jabin, AL-Musaylh, Mohanad S., Raj, Nawin, Saha, Reepa, Deo, Ravinesh C and Saha, Sanjoy Kumar. 2024. "Hybrid Deep Learning Model for Wave Height Prediction in Australia's Wave Energy Region." Applied Soft Computing. 150. https://doi.org/10.1016/j.asoc.2023.111003Automated stenosis classification on invasive coronary angiography using modified dual cross pattern with iterative feature selection
Kobat, Mehmet Ali, Barua, Prabal Datta, Tuncer, Turker, Dogan, Sengul, Kivrak, Tarik, Akin, Yusuf, Bairy, G. Muralidhar, Tan, Ru‑San and Acharya, U. Rajendra. 2024. "Automated stenosis classification on invasive coronary angiography using modified dual cross pattern with iterative feature selection." Multimedia Tools and Applications. 83 (12), pp. 35957-35977. https://doi.org/10.1007/s11042-023-16697-9Novel automated detection of sports activities using shadow videos
Barua, Prabal Datta, Tuncer, Turker, Dogan, Sengul, Ooi, Chui Ping and Acharya, Rajendra U.. 2024. "Novel automated detection of sports activities using shadow videos." Multimedia Tools and Applications. 83 (15), pp. 44933-44954. https://doi.org/10.1007/s11042-023-17407-1ExDarkLBP: a hybrid deep feature generation-based genetic malformation detection using facial images
Barua, Prabal Datta, Kirik, Serkan, Dogan, Sengul, Koc, Canan, Ozkaynak, Fatih, Baygin, Mehmet, Tuncer, Turker, Tan, Ru-San and Acharya, U. Rajendra. 2024. "ExDarkLBP: a hybrid deep feature generation-based genetic malformation detection using facial images." Multimedia Tools and Applications. 83 (13), pp. 39823-39840. https://doi.org/10.1007/s11042-023-17057-3Transfer-transfer model with MSNet: An automated accurate multiple sclerosis and myelitis detection system
Tatli, Sinan, Macin, Gulay, Tasci, Irem, Barua, Prabal Datta, Baygin, Mehmet, Tuncer, Turker, Dogan, Sengul, Ciaccio, Edward J. and Acharya, U. Rajendra. 2024. "Transfer-transfer model with MSNet: An automated accurate multiple sclerosis and myelitis detection system." Expert Systems with Applications. 236. https://doi.org/10.1016/j.eswa.2023.121314Automated characterization and detection of fibromyalgia using slow wave sleep EEG signals with glucose pattern and D’hondt pooling technique
Aksalli, Isil Karabey, Baygin, Nursena, Hagiwara, Yuki, Paul, Jose Kunnel, Iype, Thomas, Barua, Prabal Datta, Koh, Joel E. W., Baygin, Mehmet, Dogan, Sengul, Tuncer, Turker and Acharya, U. Rajendra. 2024. "Automated characterization and detection of fibromyalgia using slow wave sleep EEG signals with glucose pattern and D’hondt pooling technique." Cognitive Neurodynamics. 18 (1), pp. 283-404. https://doi.org/10.1007/s11571-023-10005-9Monthly sodium adsorption ratio forecasting in rivers using a dual interpretable glass-box complementary intelligent system: Hybridization of ensemble TVF-EMD-VMD, Boruta-SHAP, and eXplainable GPR
Jamei, Mehdi, Ali, Mumtaz, Karbasi, Masoud, Karimi, Bakhtiar, Jahannemaei, Neshat, Farooque, Aitazaz Ahsan and Yaseen, Zaher Mundher. 2024. "Monthly sodium adsorption ratio forecasting in rivers using a dual interpretable glass-box complementary intelligent system: Hybridization of ensemble TVF-EMD-VMD, Boruta-SHAP, and eXplainable GPR." Expert Systems with Applications. 237 (Part B). https://doi.org/10.1016/j.eswa.2023.121512Automated accurate emotion classification using Clefia pattern-based features with EEG signals
Dogan, Abdullah, Barua, Prabal Datta, Baygin, Mehmet, Tuncer, Turker, Dogan, Sengul, Yaman, Orhan, Dogru, Ali Hikmet and Acharya, Rajendra U.. 2024. "Automated accurate emotion classification using Clefia pattern-based features with EEG signals." International Journal of Healthcare Management. 17 (1), pp. 32-45. https://doi.org/10.1080/20479700.2022.2141694Artificial intelligence assisted tools for the detection of anxiety and depression leading to suicidal ideation in adolescents: a review
Barua, Prabal Datta, Jahmunah, Jahmunah, Oh, Oh Shu, Palmer, Elizabeth Emma, Yamakawa, Toshitaka, Kobayashi, Makiko and Acharya, Udyavara Rajendra. 2024. "Artificial intelligence assisted tools for the detection of anxiety and depression leading to suicidal ideation in adolescents: a review." Cognitive Neurodynamics. 18 (1), pp. 1-22. https://doi.org/10.1007/s11571-022-09904-0Evaluation and Prediction of Groundwater Quality for Irrigation Using an Integrated Water Quality Indices, Machine Learning Models and GIS Approaches: A Representative Case Study
Ibrahim, Hekmat, Yaseen, Zaher Mundher, Scholz, Miklas, Ali, Mumtaz, Gad, Mohamed, Elsayed, Salah, Khadr, Mosaad, Hussein, Hend, Ibrahim, Hazem H., Eid, Mohamed Hamdy, Kovács, Attila, Péter, Szűcs and Khalifa, Moataz M.. 2023. "Evaluation and Prediction of Groundwater Quality for Irrigation Using an Integrated Water Quality Indices, Machine Learning Models and GIS Approaches: A Representative Case Study." Water. 15 (4), pp. 1-26. https://doi.org/https://doi.org/10.3390/w15040694A high dimensional features-based cascaded forward neural network coupled with MVMD and Boruta-GBDT for multi-step ahead forecasting of surface soil moisture
Jamei, Mehdi, Ali, Mumtaz, Karbasi, Masoud, Sharma, Ekta, Jamei, Mozhdeh, Chu, Xuefeng and Yaseen, Zaher Mundher. 2023. "A high dimensional features-based cascaded forward neural network coupled with MVMD and Boruta-GBDT for multi-step ahead forecasting of surface soil moisture." Engineering Applications of Artificial Intelligence. 120. https://doi.org/10.1016/j.engappai.2023.105895Channel-Agnostic Training of Transmitter and Receiver for Wireless Communications
Davey, Christopher P., Shakeel, Ismail, Deo, Ravinesh C. and Salcedo-sanz, Sancho. 2023. "Channel-Agnostic Training of Transmitter and Receiver for Wireless Communications ." Sensors. 23 (24). https://doi.org/10.3390/s23249848Optimizing the Seasonal Shade and Ultraviolet Protection of a Suburban Playground by Utilization of the Playground Shade Index
Downs, Nathan, Butler, Harry, Parisi, Alfio, Beckman-Downs, Melanie and Igoe, Damien. 2023. "Optimizing the Seasonal Shade and Ultraviolet Protection of a Suburban Playground by Utilization of the Playground Shade Index." Photochemistry and Photobiology. 99 (6), pp. 1483-1492. https://doi.org/10.1111/php.13792Deep Image Analysis for Microalgae Identification
Soar, Jeffrey, Lih, Oh Shu, Wen, Loh Hui, Ward, Aleth, Sharma, Ekta, Deo, Ravinesh C., Barua, Prabal Datta, Tan, Ru-San, Rinen, Eliezer and Acharya, Rajendra. 2023. "Deep Image Analysis for Microalgae Identification." Lecture notes in computer science. Switzerland . Springer. pp. 280-292Development of an enhanced bidirectional recurrent neural network combined with time-varying filter-based empirical mode decomposition to forecast weekly reference evapotranspiration
Karbasi, Masoud, Jamei, Mehdi, Ali, Mumtaz, Malik, Anurag, Chu, Xuefeng, Farooque, Aitazaz Ahsan and Yaseen, Zaher Mundher. 2023. "Development of an enhanced bidirectional recurrent neural network combined with time-varying filter-based empirical mode decomposition to forecast weekly reference evapotranspiration." Agricultural Water Management. 290. https://doi.org/10.1016/j.agwat.2023.108604A Power Efficient Solution to Determine Red Blood Cell Deformation Type Using Binarized DenseNet
Reza, Md Tanzim, Dipto, Shakib Mahmud, Parvez, Mohammad Zavid, Barua, Prabal Datta and Chakraborty, Subrata. 2023. "A Power Efficient Solution to Determine Red Blood Cell Deformation Type Using Binarized DenseNet." 2023 International Conference on Advances in Computing Research (ACR’23). Orlando, United States 08 - 10 May 2023 Switzerland. https://doi.org/10.1007/978-3-031-33743-7_21CNN-Based Handwriting Analysis for the Prediction of Autism Spectrum Disorder
Nawer, Nafisa, Parvez, Mohammad Zavid, Hossain, Muhammad Iqbal, Barua, Prabal Datta, Rahim, Mia and Chakraborty, Subrata. 2023. "CNN-Based Handwriting Analysis for the Prediction of Autism Spectrum Disorder." Second International Conference on Innovations in Computing Research (ICR’23). Madrid, Spain 04 - 06 Sep 2023 Switzerland . https://doi.org/10.1007/978-3-031-35308-6_14A Comparison of LSTM and GRU for Bengali Speech-to-Text Transformation
Sathi, Nusrat, Shahid, Zakia, Chowdhury, Fahim, Ahmed, Sajjad, Parvez, Mohammad Zavid, Barua, Prabal Datta and Chakraborty, Subrata. 2023. "A Comparison of LSTM and GRU for Bengali Speech-to-Text Transformation." 2023 International Conference on Advances in Computing Research (ACR’23). Orlando, United States 08 - 10 May 2023 Switzerland. https://doi.org/10.1007/978-3-031-33743-7_18An XAI Integrated Identification System of White Blood Cell Type Using Variants of Vision Transformer
Dipto, Shakib Mahmud, Reza, Md Tanzim, Rahman, Md Nowroz Junaed, Parvez, Mohammad Zavid, Barua, Prabal Datta and Chakraborty, Subrata. 2023. "An XAI Integrated Identification System of White Blood Cell Type Using Variants of Vision Transformer." Second International Conference on Innovations in Computing Research (ICR’23). Madrid, Spain 04 - 06 Sep 2023 Spain. https://doi.org/10.1007/978-3-031-35308-6_26A pH-Sensitive Surface of Chitosan/Sepiolite Clay/Algae Biocomposite for the Removal of Malachite Green and Remazol Brilliant Blue R Dyes: Optimization and Adsorption Mechanism Study
Zain, Zahirah Mohd, Abdulhameed, Ahmed Saud, Jawad, Ali H., ALOthman, Zeid A. and Yaseen, Zaher Mundher. 2023. "A pH-Sensitive Surface of Chitosan/Sepiolite Clay/Algae Biocomposite for the Removal of Malachite Green and Remazol Brilliant Blue R Dyes: Optimization and Adsorption Mechanism Study." Journal of Polymers and the Environment. 31 (2), pp. 501-518. https://doi.org/10.1007/s10924-022-02614-yFF-BTP Model for Novel Sound-Based Community Emotion Detection
Yildiz, Arif Metehan, Tanabe, Masayuki, Kobayashi, Makiko, Tuncer, Ilknur, Barua, Prabal Datta, Dogan, Sengul, Tuncer, Turker, Tan, Ru-San and Acharya, U. Rajendra. 2023. "FF-BTP Model for Novel Sound-Based Community Emotion Detection." IEEE Access. 11, pp. 108705-108715. https://doi.org/10.1109/ACCESS.2023.3318751Statistical and spatial analysis for soil heavy metals over the Murray-Darling river basin in Australia
Tao, Hai, Al-Hilali, Aqeel Ali, Ahmed, Ali M., Mussa, Zainab Haider, Falah, Mayadah W., Abed, Salwan Ali, Deo, Ravinesh, Jawad, Ali H., Maulud, Khairul Nizam Abdul, Latif, Mohd Talib and Yaseen, Zaher Mundher. 2023. "Statistical and spatial analysis for soil heavy metals over the Murray-Darling river basin in Australia." Chemosphere. 317. https://doi.org/10.1016/j.chemosphere.2023.137914Application of uncertainty quantification to artificial intelligence in healthcare: A review of last decade (2013–2023)
Seoni, Silvia, Jahmunah, Vicnesh, Salvi, Massimo, Barua, Prabal Datta, Molinari, Filippo and Acharya, U. Rajendra. 2023. "Application of uncertainty quantification to artificial intelligence in healthcare: A review of last decade (2013–2023)." Computers in Biology and Medicine. 165. https://doi.org/10.1016/j.compbiomed.2023.107441Enhanced joint hybrid deep neural network explainable artificial intelligence model for 1-hr ahead solar ultraviolet index prediction
Prasad, Salvin S., Deo, Ravinesh C., Salcedo-sanz, Sancho, Downs, Nathan J., Casillas-Perez, David and Parisi, Alfio V.. 2023. "Enhanced joint hybrid deep neural network explainable artificial intelligence model for 1-hr ahead solar ultraviolet index prediction." Computer Methods and Programs in Biomedicine. 241. https://doi.org/10.1016/j.cmpb.2023.107737Ampelomyces mycoparasites of powdery mildews–a review
Prahl, Rosa E., Khan, Shahjahan and Deo, Ravinesh C.. 2023. "Ampelomyces mycoparasites of powdery mildews–a review." Canadian Journal of Plant Pathology. 45 (4), pp. 391-404. https://doi.org/10.1080/07060661.2023.2206378A fuzzy-based cascade ensemble model for improving extreme wind speeds prediction
Pelaez-Rodriguez, C., Perez-Aracil, J., Prieto-Godino, L., Ghimire, S., Deo, R. and Salcedo-sanz, S.. 2023. "A fuzzy-based cascade ensemble model for improving extreme wind speeds prediction." Journal of Wind Engineering and Industrial Aerodynamics. 240. https://doi.org/10.1016/j.jweia.2023.105507Explainable AI approach with original vegetation data classifies spatio-temporal nitrogen in flows from ungauged catchments to the Great Barrier Reef
O’Sullivan, Cherie M., Deo, Ravinesh C. and Ghahramani, Afshin. 2023. "Explainable AI approach with original vegetation data classifies spatio-temporal nitrogen in flows from ungauged catchments to the Great Barrier Reef." Scientific Reports. 13 (1). https://doi.org/10.1038/s41598-023-45259-0A Novel Attention-Based Model for Semantic Segmentation of Prostate Glands Using Histopathological Images
Inamdar, Mahesh Anil, Raghavendra, U., Gudigar, Anjan, Bhandary, Sarvesh, Salvi, Massimo, Deo, Ravinesh C., Barua, Prabal Datta, Ciaccio, Edward J., Molinari, Filippo and Acharya, RU. Rajendra. 2023. "A Novel Attention-Based Model for Semantic Segmentation of Prostate Glands Using Histopathological Images." IEEE Access. 11, pp. 108982-108994. https://doi.org/10.1109/ACCESS.2023.3321273Natural Language Processing in Electronic Health Records in relation to healthcare decision-making: A systematic review
Hossain, Elias, Rana, Rajib, Higgins, Niall, Soar, Jeffrey, Barua, Prabal Datta, Pisani, Anthony R. and Turner, Kathryn. 2023. "Natural Language Processing in Electronic Health Records in relation to healthcare decision-making: A systematic review." Computers in Biology and Medicine. 155. https://doi.org/10.1016/j.compbiomed.2023.106649Efficient daily electricity demand prediction with hybrid deep-learning multi-algorithm approach
Ghimire, Sujan, Deo, Ravinesh C., Casillas-Perez, David and Salcedo-sanz, Sancho. 2023. "Efficient daily electricity demand prediction with hybrid deep-learning multi-algorithm approach." Energy Conversion and Management. 297. https://doi.org/10.1016/j.enconman.2023.117707Innovative Fibromyalgia Detection Approach Based on Quantum-Inspired 3LBP Feature Extractor Using ECG Signal
Barua, Prabal Datta, Kobayashi, Makiko, Tanabe, Masayuki, Baygin, Mehmet, Paul, Jose Kunnel, Iype, Thomas, Dogan, Sengul, Tuncer, Turker, Tan, Ru-San and Acharya, U. Rajendra. 2023. "Innovative Fibromyalgia Detection Approach Based on Quantum-Inspired 3LBP Feature Extractor Using ECG Signal." IEEE Access. 11, pp. 101359-101372. https://doi.org/10.1109/ACCESS.2023.3315149The development of dissolved oxygen forecast model using hybrid machine learning algorithm with hydro-meteorological variables
Ahmed, Abul Abrar Masrur, Jui, S. Janifer Jabin, Chowdhury, Mohammad Aktarul Islam, Ahmed, Oli and Sutradhar, Ambica. 2023. "The development of dissolved oxygen forecast model using hybrid machine learning algorithm with hydro-meteorological variables." Environmental Science and Pollution Research. 30 (3), pp. 7851-7873. https://doi.org/10.1007/s11356-022-22601-zA novel tree pattern-based violence detection model using audio signals
Yildiz, Arif Metehan, Barua, Prabal D., Dogan, Sengul, Baygin, Mehmet, Tuncer, Turker, Ooi, Chui Ping, Fujita, Hamido and Acharya, U. Rajendra. 2023. "A novel tree pattern-based violence detection model using audio signals." Expert Systems with Applications. 224. https://doi.org/10.1016/j.eswa.2023.120031Automated detection of airflow obstructive diseases: A systematic review of the last decade (2013-2022)
Xu, Shuting, Deo, Ravinesh C, Soar, Jeffrey, Barua, Prabal Datta, Faust, Oliver, Homaira, Nusrat, Jaffe, Adam, Kabir, Arm Luthful and Acharya, U. Rajendra. 2023. "Automated detection of airflow obstructive diseases: A systematic review of the last decade (2013-2022)." Computer Methods and Programs in Biomedicine. 241. https://doi.org/10.1016/j.cmpb.2023.107746Explainable attention ResNet18-based model for asthma detection using stethoscope lung sounds
Topaloglu, Ihsan, Barua, Prabal Datta, Yildiz, Arif Metehan, Keles, Tugce, Dogan, Sengul, Baygin, Mehmet, Gul, Huseyin Fatih, Tuncer, Turker, Tan, Ru-San and Acharya, U. Rajendra. 2023. "Explainable attention ResNet18-based model for asthma detection using stethoscope lung sounds." Engineering Applications of Artificial Intelligence. 126. https://doi.org/10.1016/j.engappai.2023.106887Epilepsy detection in 121 patient populations using hypercube pattern from EEG signals
Tasci, Irem, Tasci, Burak, Barua, Prabal D., Dogan, Sengul, Tuncer, Turker, Emmanuel, Elizabeth Emma, Fujita, Hamido and Acharya, U. Rajendra. 2023. "Epilepsy detection in 121 patient populations using hypercube pattern from EEG signals." Information Fusion. 96, pp. 252-268. https://doi.org/10.1016/j.inffus.2023.03.022Automated accurate detection of depression using twin Pascal's triangles lattice pattern with EEG Signals
Tasci, Gulay, Loh, Hui Wen, Barua, Prabal Datta, Baygin, Mehmet, Tasci, Burak, Dogan, Sengul, Tuncer, Turker, Palmer, Elizabeth Emma, Tan, Ru-San and Acharya, U. Rajendra. 2023. "Automated accurate detection of depression using twin Pascal's triangles lattice pattern with EEG Signals." Knowledge-Based Systems. 260. https://doi.org/10.1016/j.knosys.2022.110190QLBP: Dynamic patterns-based feature extraction functions for automatic detection of mental health and cognitive conditions using EEG signals
Tasci, Gulay, Gun, Mehmet Veysel, Keles, Tugce, Tasci, Burak Tasci, Barua, Prabal Datta, Tasci, Irem, Dogan, Sengul, Baygin, Mehmet, Palmer, Elizabeth Emma, Tuncer, Turker, Ooi, Chui Ping and Acharya, U. Rajendra. 2023. "QLBP: Dynamic patterns-based feature extraction functions for automatic detection of mental health and cognitive conditions using EEG signals." Chaos, Solitons and Fractals. 172. https://doi.org/10.1016/j.chaos.2023.113472Automated Adrenal Gland Disease Classes Using Patch-Based Center Symmetric Local Binary Pattern Technique with CT Images
Sut, Suat Kamil, Koc, Mustafa, Zorlu, Gokhan, Serhatlioglu, Ihsan, Barua, Prabal Datta, Dogan, Sengul, Baygin, Mehmet, Tuncer, Turker, Tan, Ru‑San and Acharya, U. Rajendra. 2023. "Automated Adrenal Gland Disease Classes Using Patch-Based Center Symmetric Local Binary Pattern Technique with CT Images." Journal of Digital Imaging. 36 (3), pp. 879-892. https://doi.org/10.1007/s10278-022-00759-9NRC-Net: Automated noise robust cardio net for detecting valvular cardiac diseases using optimum transformation method with heart sound signals
Shuvo, Samiul Based, Alam, Syed Samiul, Ayman, Syeda Umme, Chakma, Arbil, Barua, Prabal Datta and Acharya, U. Rajendra. 2023. "NRC-Net: Automated noise robust cardio net for detecting valvular cardiac diseases using optimum transformation method with heart sound signals." Biomedical Signal Processing and Control. 86 (Part C). https://doi.org/10.1016/j.bspc.2023.105272PrismPatNet: Novel prism pattern network for accurate fault classification using engine sound signals
Sahin, Sakir Engin, Gulhan, Gokhan, Barua, Prabal Datta, Tuncer, Turker, Dogan, Sengul, Faust, Oliver and Acharya, U. Rajendra. 2023. "PrismPatNet: Novel prism pattern network for accurate fault classification using engine sound signals." Expert Systems: the journal of knowledge engineering. 40 (8). https://doi.org/10.1111/exsy.13312Brain tumor detection and screening using artificial intelligence techniques: Current trends and future perspectives
Raghavendra, U., Gudigar, Anjan, Paul, Aritra, Goutham, T.S., Inamdar, Mahesh Anil, Hegde, Ajay, Dev, Aruna, Ooi, Chui Ping, Deo, Ravinesh C., Barua, Prabal Datta, Molinari, Filippo, Ciaccio, Edward J. and Acharya, U. Rajendra. 2023. "Brain tumor detection and screening using artificial intelligence techniques: Current trends and future perspectives." Computers in Biology and Medicine. 163. https://doi.org/10.1016/j.compbiomed.2023.107063PatchResNet: Multiple Patch Division–Based Deep Feature Fusion Framework for Brain Tumor Classification Using MRI Images
Muezzinoglu, Taha, Baygin, Nursena, Tuncer, Ilknur, Barua, Prabal Datta, Baygin, Mehmet, Dogan, Sengul, Tuncer, Turker, Palmer, Elizabeth E., Cheong, Kang Hao and Acharya, U. Rajendra. 2023. "PatchResNet: Multiple Patch Division–Based Deep Feature Fusion Framework for Brain Tumor Classification Using MRI Images." Journal of Digital Imaging. 36 (3), pp. 973-987. https://doi.org/10.1007/s10278-023-00789-xDeep neural network technique for automated detection of ADHD and CD using ECG signal
Loh, Hui Wen, Ooi, Chui Pin, Oh, Shu Lih, Barua, Prabal Datta, Tan, Yi Ren, Molinari, Filippo, March, Sonja, Acharya, U. Rajendra and Fung, Daniel Shuen Sheng. 2023. "Deep neural network technique for automated detection of ADHD and CD using ECG signal." Computer Methods and Programs in Biomedicine. 241. https://doi.org/10.1016/j.cmpb.2023.107775EpilepsyNet: Novel automated detection of epilepsy using transformer model with EEG signals from 121 patient population
Oh, Shu Lih, Jahmunah, V., Emmanuel, Elizabeth Emma, Barua, Prabal D., Dogan, Sengul, Tuncer, Turker, García, Salvador, Molinari, Filippo and Acharya, U. Rajendra. 2023. "EpilepsyNet: Novel automated detection of epilepsy using transformer model with EEG signals from 121 patient population." Computers in Biology and Medicine. 164. https://doi.org/10.1016/j.compbiomed.2023.107312FGPat18: Feynman graph pattern-based language detection model using EEG signals
Kirik, Serkan, Dogan, Sengul, Baygin, Mehmet, Barua, Prabal Datta, Demir, Caner Feyzi, Keles, Tugce, Yildiz, Arif Metehan, Baygin, Nursena, Tuncer, Ilknur, Tuncer, Turker, Tan, Ru-San and Acharya, U. Rajendra. 2023. "FGPat18: Feynman graph pattern-based language detection model using EEG signals." Biomedical Signal Processing and Control. 85. https://doi.org/10.1016/j.bspc.2023.104927Application of data fusion for automated detection of children with developmental and mental disorders: A systematic review of the last decade
Khare, Smith K., March, Sonja, Barua, Prabal Datta, Gadre, Vikram M. and Acharya, U. Rajendra. 2023. "Application of data fusion for automated detection of children with developmental and mental disorders: A systematic review of the last decade." Information Fusion. 99. https://doi.org/10.1016/j.inffus.2023.101898PFP-HOG: Pyramid and Fixed-Size Patch-Based HOG Technique for Automated Brain Abnormality Classification with MRI
Kaplan, Ela, Chan, Wai Yee, Altinsoy, Hasan Baki, Baygin, Mehmet, Barua, Prabal Datta, Chakraborty, Subrata, Dogan, Sengul, Tuncer, Turker and Acharya, U. Rajendra. 2023. "PFP-HOG: Pyramid and Fixed-Size Patch-Based HOG Technique for Automated Brain Abnormality Classification with MRI." Journal of Digital Imaging. 23, p. 2441–2460. https://doi.org/10.1007/s10278-023-00889-8ExHiF: Alzheimer's disease detection using exemplar histogram-based features with CT and MR images
Kaplan, Ela, Baygin, Mehmet, Barua, Prabal D., Dogan, Sengul, Tuncer, Turker, Altunisik, Erman, Palmer, Elizabeth Emma and Acharya, U. Rajendra. 2023. "ExHiF: Alzheimer's disease detection using exemplar histogram-based features with CT and MR images." Medical Engineering and Physics. 115. https://doi.org/10.1016/j.medengphy.2023.103971Application of Entropy for Automated Detection of Neurological Disorders With Electroencephalogram Signals: A Review of the Last Decade (2012-2022)
Jui, S. Janifer Jabin, Deo, Ravinesh C. Deo, Barua, Prabal Datta, Devi, Aruna, Soar, Jeffrey and Acharya, U. Rajendra. 2023. "Application of Entropy for Automated Detection of Neurological Disorders With Electroencephalogram Signals: A Review of the Last Decade (2012-2022)." IEEE Access. 11, pp. 71905-71924. https://doi.org/10.1109/ACCESS.2023.3294473Endoscopy, video capsule endoscopy, and biopsy for automated celiac disease detection: A review
Jahmunah, V., Koh, Joel En Wei, Sudarshan, Vidya K., Raghavendra, U., Gudigar, Anjan, Oh, Shu Lih, Loh, Hui Wen, Faust, Oliver, Barua, Prabal Datta, Ciaccio, Edward J. and Acharya, U. Rajendra. 2023. "Endoscopy, video capsule endoscopy, and biopsy for automated celiac disease detection: A review." Biocybernetics and Biomedical Engineering. 43 (1), pp. 82-108. https://doi.org/10.1016/j.bbe.2022.12.002Development of Debiasing Technique for Lung Nodule Chest X-ray Datasets to Generalize Deep Learning Models
Horry, Michael J., Chakraborty, Subrata, Pradhan, Biswajeet, Paul, Manoranjan, Zhu, Jing, Loh, Hui Wen, Barua, Prabal Datta and Acharya, U. Rajendra. 2023. "Development of Debiasing Technique for Lung Nodule Chest X-ray Datasets to Generalize Deep Learning Models." Sensors. 23 (14). https://doi.org/10.3390/s23146585Most complicated lock pattern-based seismological signal framework for automated earthquake detection
Gokhan, Suat Gokhan, Baygin, Nursena, Barua, Prabal D., Singh, Arvind R., Bajaj, Mohit, Baygin, Mehmet, Dogan, Sengul, Tuncer, Turker, Tan, Ru-San and Acharya, U. Rajendra. 2023. "Most complicated lock pattern-based seismological signal framework for automated earthquake detection." International Journal of Applied Earth Observation and Geoinformation. 118. https://doi.org/10.1016/j.jag.2023.103297Automated Urine Cell Image Classification Model Using Chaotic Mixer Deep Feature Extraction
Erten, Mehmet, Tuncer, Ilknur, Barua, Prabal D., Yildirim, Kubra, Dogan, Sengul, Tuncer, Turker, Tan, Ru-San, Fujita, Hamido and Acharya, U. Rajendra. 2023. "Automated Urine Cell Image Classification Model Using Chaotic Mixer Deep Feature Extraction." Journal of Digital Imaging. 36 (4), pp. 1675-1686. https://doi.org/10.1007/s10278-023-00827-8Swin-LBP: a competitive feature engineering model for urine sediment classification
Erten, Mehmet, Barua, Praba Datta, Tuncer, Ilknur, Dogan, Sengul, Baygin, Mehmet, Tuncer, Turker, Tan, Ru-San and Acharya, U. Rajendra. 2023. "Swin-LBP: a competitive feature engineering model for urine sediment classification." Neural Computing and Applications. 35 (29), pp. 21621-21632. https://doi.org/10.1007/s00521-023-08919-wHybrid-Patch-Alex: A new patch division and deep feature extraction-based image classification model to detect COVID-19, heart failure, and other lung conditions using medical images
Erdem, Kenan, Kobat, Mehmet Ali, Bilen, Mehmet Nail, Balik, Yunus, Alkan, Sevim, Cavlak, Feyzanur, Poyraz, Ahmet Kursad, Barua, Prabal Datta, Tuncer, Ilknur, Dogan, Sengul, Baygin, Mehmet, Erten, Mehmet, Tuncer, Turker, Tan, Ru-San and Acharya, U. Rajendra. 2023. "Hybrid-Patch-Alex: A new patch division and deep feature extraction-based image classification model to detect COVID-19, heart failure, and other lung conditions using medical images." International Journal of Imaging Systems and Technology. 33 (4), pp. 1144-1159. https://doi.org/10.1002/ima.22914Primate brain pattern-based automated Alzheimer's disease detection model using EEG signals
Dogan, Sengul, Baygin, Mehmet, Tasci, Burak, Loh, Hui Wen, Barua, Prabal D., Tuncer, Turker, Tan, Ru-San and Acharya, U. Rajendra. 2023. "Primate brain pattern-based automated Alzheimer's disease detection model using EEG signals." Cognitive Neurodynamics. 17 (3), pp. 647-659. https://doi.org/10.1007/s11571-022-09859-2Automated mental arithmetic performance detection using quantum pattern- and triangle pooling techniques with EEG signals
Baygin, Nursena, Aydemir, Emrah, Barua, Prabal D., Baygin, Mehmet, Dogan, Sengul, Tuncer, Turker, Tan, Ru-San and Acharya, Rajendra. 2023. "Automated mental arithmetic performance detection using quantum pattern- and triangle pooling techniques with EEG signals." Expert Systems with Applications. 227. https://doi.org/10.1016/j.eswa.2023.120306Automated facial expression recognition using exemplar hybrid deep feature generation technique
Baygin, Mehmet, Tuncer, Ilknur, Dogan, Sengul, Barua, Prabal Datta, Tuncer, Turker, Cheong, Kang Hao and Acharya, U. Rajendra. 2023. "Automated facial expression recognition using exemplar hybrid deep feature generation technique." Soft Computing. 27 (13), pp. 8721-8737. https://doi.org/10.1007/s00500-023-08230-9CCPNet136: automated detection of schizophrenia using carbon chain pattern and iterative TQWT technique with EEG signals
Baygin, Mehmet, Barua, Prabal Datta, Chakraborty, Subrata, Tuncer, Ilknur, Dogan, Sengul, Palmer, Elizabeth, Tuncer, Turker, Kamath, Aditya, Ciaccio, Edward J and Acharya, U Rajendra. 2023. "CCPNet136: automated detection of schizophrenia using carbon chain pattern and iterative TQWT technique with EEG signals ." Physiological Measurement. 44 (3). https://doi.org/10.1088/1361-6579/acb03cNFSDense201: microstructure image classification based on non-fixed size patch division with pre-trained DenseNet201 layers
Barua, Prabal Datta, Dogan, Sengul, Kavuran, Gurkan, Tuncer, Turker, Tan, Ru-San and Acharya, U. Rajendra. 2023. "NFSDense201: microstructure image classification based on non-fixed size patch division with pre-trained DenseNet201 layers." Neural Computing and Applications. 35 (30), pp. 22253-22263. https://doi.org/10.1007/s00521-023-08825-1Multilevel hybrid accurate handcrafted model for myocardial infarction classification using ECG signals
Barua, Prabal Datta, Aydemir, Emrah, Dogan, Sengul, Kobat, Mehmet Ali, Demir, Fahrettin Burak, Baygin, Mehmet, Tuncer, Turker, Oh, Shu Lih, Tan, Ru-San and Acharya, U. Rajendra. 2023. "Multilevel hybrid accurate handcrafted model for myocardial infarction classification using ECG signals." International Journal of Machine Learning and Cybernetics. 14 (5), pp. 1651-1668. https://doi.org/10.1007/s13042-022-01718-0Novel favipiravir pattern-based learning model for automated detection of specific language impairment disorder using vowels
Barua, Prabal Datta, Aydemir, Emrah, Dogan, Sengul, Erten, Mehmet, Kaysi, Feyzi, Tuncer, Turker, Fujita, Hamido, Palmer, Elizabeth and Acharya, U Rajendra. 2023. "Novel favipiravir pattern-based learning model for automated detection of specific language impairment disorder using vowels." Neural Computing and Applications. 35 (8), pp. 6065-6077. https://doi.org/10.1007/s00521-022-07999-4LSGP-USFNet: Automated Attention Deficit Hyperactivity Disorder Detection Using Locations of Sophie Germain’s Primes on Ulam’s Spiral-Based Features with Electroencephalogram Signals
Atila, Orhan, Deniz, Erkan, Ari, Ali, Sengur, Abdulkadir, Chakraborty, Subrata, Barua, Prabal Datta and Acharya, U. Rajendra. 2023. "LSGP-USFNet: Automated Attention Deficit Hyperactivity Disorder Detection Using Locations of Sophie Germain’s Primes on Ulam’s Spiral-Based Features with Electroencephalogram Signals." Sensors. 23 (16). https://doi.org/10.3390/s23167032Explainable automated anuran sound classification using improved one-dimensional local binary pattern and Tunable Q Wavelet Transform techniques
Akbal, Erhan, Barua, Prabal Datta, Dogan, Sengul, Tuncer, Turker and Acharya, U. Rajendra. 2023. "Explainable automated anuran sound classification using improved one-dimensional local binary pattern and Tunable Q Wavelet Transform techniques." Expert Systems with Applications. 225. https://doi.org/10.1016/j.eswa.2023.120089Integrated Multi-Head Self-Attention Transformer model for electricity demand prediction incorporating local climate variables
Ghimire, Sujan, Nguyen-Huy, Thong, AL-Musaylh, Mohanad S., Deo, Ravinesh C., Casillas-Perez, David and Salcedo-sanz, Sancho. 2023. "Integrated Multi-Head Self-Attention Transformer model for electricity demand prediction incorporating local climate variables." Energy and AI. 14. https://doi.org/10.1016/j.egyai.2023.100302Multivariate data decomposition based deep learning approach to forecast one-day ahead significant wave height for ocean energy generation
Zheng, Zihao, Ali, Mumtaz, Jamei, Mehdi, Xiang, Yong, Abdulla, Shahab, Yaseen, Zaher Mundher and Farooque, Aitazaz A.. 2023. "Multivariate data decomposition based deep learning approach to forecast one-day ahead significant wave height for ocean energy generation." Renewable and Sustainable Energy Reviews. 185. https://doi.org/10.1016/j.rser.2023.113645Application of local configuration pattern for automated detection of schizophrenia with electroencephalogram signals
WeiKoh, Joel En, Rajinikanth, Venkatesan, Vicnesh, Jahmunah, Pham, The-Hanh, Oh, Shu Lih, Yeong, Chai Hong, Sankaranarayanan, Meena, Kamath, Aditya, Bairy, Giliyar Muralidhar, Barua, Prabal Datta and Cheong, Kang Hao. 2024. "Application of local configuration pattern for automated detection of schizophrenia with electroencephalogram signals." Expert Systems: the journal of knowledge engineering. 41 (5). https://doi.org/10.1111/exsy.12957Modeling and optimization of the adsorptive removal of crystal violet dye by durian (Durio zibethinus) seeds powder: insight into kinetic, isotherm, thermodynamic, and adsorption mechanism
Jani, Nur Aimi, Haddad, Larbi, Abdulhameed, Ahmed Saud, Jawad, Ali H., ALOthman, Zeid A. and Yaseen, Zaher Mundher. 2024. "Modeling and optimization of the adsorptive removal of crystal violet dye by durian (Durio zibethinus) seeds powder: insight into kinetic, isotherm, thermodynamic, and adsorption mechanism." Biomass Conversion and Biorefinery. 14 (11), pp. 12441-12454. https://doi.org/10.1007/s13399-022-03319-xA Hand-Modeled Feature Extraction-Based Learning Network to Detect Grasps Using sEMG Signal
Baygin, Mehmet, Barua, Prabal Datta, Dogan, Sengul, Tuncer, Turker, Key, Sefa, Acharya, U. Rajendra and Cheong, Kang Hao. 2022. "A Hand-Modeled Feature Extraction-Based Learning Network to Detect Grasps Using sEMG Signal." Sensors. 22 (5). https://doi.org/10.3390/s22052007Mental performance classification using fused multilevel feature generation with EEG signals
Aydemir, Emrah, Baygin, Mehmet, Dogan, Sengul, Tuncer, Turker, Barua, Prabal Datta, Chakraborty, Subrata, Faust, Oliver, Arunkumar, N., Kaysi, Feyzi and Acharya, U. Rajendra. 2023. "Mental performance classification using fused multilevel feature generation with EEG signals." International Journal of Healthcare Management. 16 (4), pp. 574-587. https://doi.org/10.1080/20479700.2022.2130645Novel multiple pooling and local phase quantization stable feature extraction techniques for automated classification of brain infarcts
Dogan, Sengul, Barua, Prabal Datta, Baygin, Mehmet, Chakraborty, Subrata, Ciaccio, Edward J., Tuncer, Turker, Kadir, Khairul Azmi Abd, Shah, Mohammad Nazri Md, Azman, Raja Rizal, Lee, Chin Chew, Ng, Kwan Hoong and Acharya, U. Rajendra. 2022. "Novel multiple pooling and local phase quantization stable feature extraction techniques for automated classification of brain infarcts." Biocybernetics and Biomedical Engineering. 42 (3), pp. 815-828. https://doi.org/10.1016/j.bbe.2022.06.004Role of Four-Chamber Heart Ultrasound Images in Automatic Assessment of Fetal Heart: A Systematic Understanding
Gudigar, Anjan, U., Raghavendra, Samanth, Jyothi, Vasudeva, Akhila, A. J., Ashwal, Nayak, Krishnananda, Tan, Ru-San, Ciaccio, Edward J., Ooi, Chui Ping, Barua, Prabal Datta, Molinari, Filippo and Acharya, U. Rajendra. 2022. "Role of Four-Chamber Heart Ultrasound Images in Automatic Assessment of Fetal Heart: A Systematic Understanding." Informatics. 9 (2). https://doi.org/10.3390/informatics9020034Heart rate variability for medical decision support systems: A review
Faust, Oliver, Hong, Wanrong, Loh, Hui Wen, Xu, Shuting, Tan, Ru-San, Chakraborty, Subrata, Barua, Prabal Datta, Molinari, Filippo and Acharya, U. Rajendra. 2022. "Heart rate variability for medical decision support systems: A review." Computers in Biology and Medicine. 145. https://doi.org/10.1016/j.compbiomed.2022.105407Hamlet-Pattern-Based Automated COVID-19 and Influenza Detection Model Using Protein Sequences
Erten, Mehmet, Acharya, Madhav R., Kamath, Aditya P., Sampathila, Niranjana, Bairy, G. Muralidhar, Aydemir, Emrah, Barua, Prabal Datta, Baygin, Mehmet, Tuncer, Ilknur, Dogan, Sengul and Tuncer, Turker. 2022. "Hamlet-Pattern-Based Automated COVID-19 and Influenza Detection Model Using Protein Sequences." Diagnostics. 12 (12). https://doi.org/10.3390/diagnostics12123181Exemplar Darknet19 feature generation technique for automated kidney stone detection with coronal CT images
Baygin, Mehmet, Yaman, Orhan, Barua, Prabal Datta, Dogan, Sengul, Tuncer, Turker and Acharya, U. Rajendra. 2022. "Exemplar Darknet19 feature generation technique for automated kidney stone detection with coronal CT images." Artificial Intelligence in Medicine. 127. https://doi.org/10.1016/j.artmed.2022.102274DesPatNet25: Data encryption standard cipher model for accurate automated construction site monitoring with sound signals
Akbal, Erhan, Barua, Prabal Datta, Dogan, Sengul, Tuncer, Turker and Acharya, U. Rajendra. 2022. "DesPatNet25: Data encryption standard cipher model for accurate automated construction site monitoring with sound signals." Expert Systems with Applications. 193, pp. 1-10. https://doi.org/10.1016/j.eswa.2021.116447Automated Diabetic Retinopathy Detection Using Horizontal and Vertical Patch Division-Based Pre-Trained DenseNET with Digital Fundus Images
Kobat, Sabiha Gungor, Baygin, Nursena, Yusufoglu, Elif, Baygin, Mehmet, Barua, Prabal Datta, Dogan, Sengul, Yaman, Orhan, Celiker, Ulku, Yildirim, Hakan, Tan, Ru-San, Tuncer, Turker, Islam, Nazrul and Acharya, U. Rajendra. 2022. "Automated Diabetic Retinopathy Detection Using Horizontal and Vertical Patch Division-Based Pre-Trained DenseNET with Digital Fundus Images." Diagnostics. 12 (8). https://doi.org/10.3390/diagnostics12081975Automated detection of ADHD: Current trends and future perspective
Loh, Hui Wen, Ooi, Chui Ping, Barua, Prabal Datta, Palmer, Elizabeth E., Molinari, Filippo and Acharya, U. Rajendra. 2022. "Automated detection of ADHD: Current trends and future perspective." Computers in Biology and Medicine. 146. https://doi.org/10.1016/j.compbiomed.2022.105525An accurate valvular heart disorders detection model based on a new dual symmetric tree pattern using stethoscope sounds
Barua, Prabal Datta, Karasu, Mehdi, Kobat, Mehmet Ali, Balik, Yunus, Kivrak, Tarik, Baygin, Mehmet, Dogan, Sengul, Demir, Fahrettin Burak, Tuncer, Turker, Tan, Ru-San and Acharya, U. Rajendra. 2022. "An accurate valvular heart disorders detection model based on a new dual symmetric tree pattern using stethoscope sounds." Computers in Biology and Medicine. 146, pp. 1-10. https://doi.org/10.1016/j.compbiomed.2022.105599A new lateral geniculate nucleus pattern-based environmental sound classification using a new large sound dataset
Tasci, Burak, Acharya, Madhav R., Barua, Prabal Datta, Yildiz, Arif Metehan, Gun, Mehmet Veysel, Keles, Tugce, Dogan, Sengul and Tuncer, Turker. 2022. "A new lateral geniculate nucleus pattern-based environmental sound classification using a new large sound dataset." Applied Acoustics. 196. https://doi.org/10.1016/j.apacoust.2022.108897CGP17Pat: Automated Schizophrenia Detection Based on a Cyclic Group of Prime Order Patterns Using EEG Signals
Aydemir, Emrah, Dogan, Sengul, Baygin, Mehmet, Ooi, Chui Ping, Barua, Prabal Datta, Tuncer, Turker and Acharya, U. Rajendra. 2022. "CGP17Pat: Automated Schizophrenia Detection Based on a Cyclic Group of Prime Order Patterns Using EEG Signals." Healthcare. 10 (4), pp. 1-18. https://doi.org/10.3390/healthcare10040643Application of photoplethysmography signals for healthcare systems: An in-depth review
Loh, Hui Wen, Xu, Shuting, Faust, Oliver, Ooi, Chui Ping, Barua, Prabal Datta, Chakraborty, Subrata, Tan, Ru-San, Molinari, Filippo and Acharya, U. Rajendra. 2022. "Application of photoplethysmography signals for healthcare systems: An in-depth review." Computer Methods and Programs in Biomedicine. 216, pp. 1-14. https://doi.org/10.1016/j.cmpb.2022.106677EPSPatNet86: eight-pointed star pattern learning network for detection ADHD disorder using EEG signals
Tanko, Dahiru, Barua, Prabal Datta, Dogan, Sengul, Tuncer, Turker, Palmer, Elizabeth, Ciaccio, Edward J. and Acharya, U. Rajendra. 2022. "EPSPatNet86: eight-pointed star pattern learning network for detection ADHD disorder using EEG signals." Physiological Measurement. 43 (3), pp. 1-13. https://doi.org/10.1088/1361-6579/ac59dcDesigning a decomposition-based multi-phase pre-processing strategy coupled with EDBi-LSTM deep learning approach for sediment load forecasting
Jamei, Mehdi, Ali, Mumtaz, Malik, Anurag, Rai, Priya, Karbasi, Masoud, Farooque, Aitazaz A. and Yaseen, Zaher Mundher. 2023. "Designing a decomposition-based multi-phase pre-processing strategy coupled with EDBi-LSTM deep learning approach for sediment load forecasting." Ecological Indicators. 153. https://doi.org/10.1016/j.ecolind.2023.110478An accurate automated speaker counting architecture based on James Webb Pattern
Barua, Prabal Datta, Yildiz, Arif Metehan, Canpolat, Nida, Keles, Tugce, Dogan, Sengul, Baygin, Mehmet, Tuncer, Ilknur, Tuncer, Turker, Tan, Ru-San, Fujita, Hamido and Acharya, U. Rajendra. 2023. "An accurate automated speaker counting architecture based on James Webb Pattern ." Engineering Applications of Artificial Intelligence. 119. https://doi.org/10.1016/j.engappai.2023.105821Automated analysis of small intestinal lamina propria to distinguish normal, Celiac Disease, and Non-Celiac Duodenitis biopsy images
Faust, Oliver, De Michele, Simona, Koh, Joel E. W., Jahmunah, V., Lih, Oh Shu, Kamath, Aditya P., Barua, Prabal Datta, Ciaccio, Edward J., Lewis, Suzanne K., Green, Peter H., Bhagat, Govind and Acharya, U. Rajendra. 2023. "Automated analysis of small intestinal lamina propria to distinguish normal, Celiac Disease, and Non-Celiac Duodenitis biopsy images." Computer Methods and Programs in Biomedicine. 230. https://doi.org/10.1016/j.cmpb.2022.107320Swin-textural: A novel textural features-based image classification model for COVID-19 detection on chest computed tomography
Tuncer, Ilknur, Barua, Prabal Datta, Dogan, Sengul, Baygin, Mehmet, Tuncer, Turker, Tan, Ru-San, Yeong, Chai Hong and Acharya, U. Rajendra. 2023. "Swin-textural: A novel textural features-based image classification model for COVID-19 detection on chest computed tomography." Informatics in Medicine Unlocked. 36. https://doi.org/10.1016/j.imu.2022.101158Role of Artificial Intelligence in COVID-19 Detection
Gudigar, Anjan, Raghavendra, U., Nayak, Sneha, Ooi, Chui Ping, Chan, Wai Yee, Gangavarapu, Mokshagna Rohit, Dharmik, Chinmay, Samanth, Jyothi, Kadri, Nahrizul Adib, Hasikin, Khairunnisa, Barua, Prabal Datta, Chakraborty, Subrata, Ciaccio, Edward J. and Acharya, U. Rajendra. 2021. "Role of Artificial Intelligence in COVID-19 Detection." Sensors. 21 (23). https://doi.org/10.3390/s21238045A new one-dimensional testosterone pattern-based EEG sentence classification method
Keles, Tugce, Yildiz, Arif Metehan, Barua, Prabal Datta, Dogan, Sengul, Baygin, Mehmet, Tuncer, Turker, Demir, Caner Feyzi, Ciaccio, Edward J. and Acharya, U. Rajendra. 2023. "A new one-dimensional testosterone pattern-based EEG sentence classification method." Engineering Applications of Artificial Intelligence. 119. https://doi.org/10.1016/j.engappai.2022.105722Automated accurate fire detection system using ensemble pretrained residual network
Dogan, Sengul, Barua, Prabal Datta, Kutlu, Huseyin, Baygin, Mehmet, Fujita, Hamido, Tuncer, Turker and Acharya, U. Rajendra. 2022. "Automated accurate fire detection system using ensemble pretrained residual network." Expert Systems with Applications. 203. https://doi.org/10.1016/j.eswa.2022.117407Automated BI-RADS classification of lesions using pyramid triple deep feature generator technique on breast ultrasound images
Kaplan, Ela, Chan, Wai Yee, Dogan, Sengul, Barua, Prabal D., Bulut, Haci Taner, Tuncer, Turker, Cizik, Mert, Tan, Ru-San and Acharya, U Rajendra. 2022. "Automated BI-RADS classification of lesions using pyramid triple deep feature generator technique on breast ultrasound images." Medical Engineering and Physics. 108. https://doi.org/10.1016/j.medengphy.2022.103895L-Tetrolet Pattern-Based Sleep Stage Classification Model Using Balanced EEG Datasets
Barua, Prabal Datta, Tuncer, Ilknur, Aydemir, Emrah, Faust, Oliver, Chakraborty, Subrata, Subbhuraam, Vinithasree, Tuncer, Turker, Dogan, Sengul and Acharya, U. Rajendra. 2022. "L-Tetrolet Pattern-Based Sleep Stage Classification Model Using Balanced EEG Datasets." Diagnostics. 12 (10). https://doi.org/10.3390/diagnostics12102510A review of automated sleep disorder detection
Xu, Shuting, Faust, Oliver, Seoni, Silvia, Chakraborty, Subrata, Barua, Prabal Datta, Loh, Hui Wen, Elphick, Heather, Molinari, Filippo and Acharya, U. Rajendra. 2022. "A review of automated sleep disorder detection." Computers in Biology and Medicine. 150. https://doi.org/10.1016/j.compbiomed.2022.106100Application of explainable artificial intelligence for healthcare: A systematic review of the last decade (2011–2022)
Loh, Hui Wen, Ooi, Chui Ping, Seoni, Silvia, Barua, Prabal Datta, Molinari, Filippo and Acharya, U. Rajendra. 2022. "Application of explainable artificial intelligence for healthcare: A systematic review of the last decade (2011–2022)." Computer Methods and Programs in Biomedicine. 226. https://doi.org/10.1016/j.cmpb.2022.107161Development of novel automated language classification model using pyramid pattern technique with speech signals
Akbal, Erhan, Barua, Prabal Datta, Tuncer, Turker, Dogan, Sengul and Acharya, U. Rajendra. 2022. "Development of novel automated language classification model using pyramid pattern technique with speech signals." Neural Computing and Applications. 34 (23), pp. 21319-21333. https://doi.org/10.1007/s00521-022-07613-7Automated detection of pain levels using deep feature extraction from shutter blinds‑based dynamic‑sized horizontal patches with facial images
Barua, Prabal Datta, Baygin, Nursena, Dogan, Sengul, Baygin, Mehmet, Arunkumar, N., Fujita, Hamido, Tuncer, Turker, Tan, Ru‑San, Palmer, Elizabeth, Azizan, Muhammad Mokhzaini Bin, Kadri, Nahrizul Adib and Acharya, U. Rajendra. 2022. "Automated detection of pain levels using deep feature extraction from shutter blinds‑based dynamic‑sized horizontal patches with facial images." Scientific Reports. 12 (1). https://doi.org/10.1038/s41598-022-21380-4Automated EEG sentence classification using novel dynamic-sized binary pattern and multilevel discrete wavelet transform techniques with TSEEG database
Barua, Prabal Datta, Keles, Tugce, Dogan, Sengul, Baygin, Mehmet, Tuncer, Turker, Demir, Caner Feyzi, Fujita, Hamido, Tan, Ru-San, Ooi, Chui Ping and Acharya, U. Rajendra. 2023. "Automated EEG sentence classification using novel dynamic-sized binary pattern and multilevel discrete wavelet transform techniques with TSEEG database." Biomedical Signal Processing and Control. 79 (Part 1). https://doi.org/10.1016/j.bspc.2022.104055Development of high-resolution gridded data for water availability identification through GRACE data downscaling: Development of machine learning models
Tao, Hai, Al-Sulttani, Ahmed H., Salih, Sinan Q., Mohammed, Mustafa K.A., Khan, Mohammad Amir, Beyaztas, Beste Hamiye, Ali, Mumtaz, Elsayed, Salah, Shahid, Shamsuddin and Yaseen, Zaher Mundher. 2023. "Development of high-resolution gridded data for water availability identification through GRACE data downscaling: Development of machine learning models." Atmospheric Research. 291. https://doi.org/10.1016/j.atmosres.2023.106815The Great 2011 Thailand flood disaster revisited: Could it have been mitigated by different dam operations based on better weather forecasts?
Loc, Ho Huu, Emadzadeh, Adel, Park, Edward, Nontikansak, Piyanuch and Deo, Ravinesh C.. 2023. "The Great 2011 Thailand flood disaster revisited: Could it have been mitigated by different dam operations based on better weather forecasts? " Environmental Research. 216 (Part 2). https://doi.org/10.1016/j.envres.2022.114493Comparison of machine learning methods emulating process driven crop models
Johnston, David B., Pembleton, Keith G., Huth, Neil I. and Deo, Ravinesh C.. 2023. "Comparison of machine learning methods emulating process driven crop models ." Environmental Modelling and Software. 162. https://doi.org/10.1016/j.envsoft.2023.105634Multi-step ahead hourly forecasting of air quality indices in Australia: Application of an optimal time-varying decomposition-based ensemble deep learning algorithm
Jamei, Mehdi, Ali, Mumtaz, Jun, Changhyun, Bateni, Sayed M., Karbasi, Masoud, Farooque, Aitazaz A. and Yaseen, Zaher Mundher. 2023. "Multi-step ahead hourly forecasting of air quality indices in Australia: Application of an optimal time-varying decomposition-based ensemble deep learning algorithm." Atmospheric Pollution Research. 14 (6). https://doi.org/10.1016/j.apr.2023.101752Machine Learning Algorithms for High-Resolution Prediction of Spatiotemporal Distribution of Air Pollution from Meteorological and Soil Parameters
Tao, Hai, Jawad, Ali H., Shather, A.H., Al-Khafaji, Zainab Al, Rashid, Tarik A., Ali, Mumtaz, Al-Ansari, Nadhir Al, Marhoon, Haydar Abdulameer, Shahid, Shamsuddin and Yaseen, Zaher Mundher. 2023. "Machine Learning Algorithms for High-Resolution Prediction of Spatiotemporal Distribution of Air Pollution from Meteorological and Soil Parameters." Environment International. 175. https://doi.org/10.1016/j.envint.2023.107931A novel approach based on integration of convolutional neural networks and echo state network for daily electricity demand prediction
Ghimire, Sujan, Nguyen-Huy, Thong, AL-Musaylh, Mohanad S., Deo, Ravinesh, Casillas-Perez, David and Salcedo-sanz, Sancho. 2023. "A novel approach based on integration of convolutional neural networks and echo state network for daily electricity demand prediction." Energy. 275, p. 127430. https://doi.org/10.1016/j.energy.2023.127430Surface water electrical conductivity and bicarbonate ion determination using a smart hybridization of optimal Boruta package with Elman recurrent neural network
Jamei, Mehdi, Ali, Mumtaz, Karimi, Bakhtiar, Karbasi, Masoud, Farooque, Aitataz Ahsan and Yaseen, Zaher Mundher. 2023. "Surface water electrical conductivity and bicarbonate ion determination using a smart hybridization of optimal Boruta package with Elman recurrent neural network." Process Safety and Environmental Protection. 174, pp. 115-134. https://doi.org/10.1016/j.psep.2023.03.062Accurate Image Multi-Class Classification Neural Network Model with Quantum Entanglement Approach
Riaz, Farina, Abdulla, Shahab, Suzuki, Hajime, Ganguly, Srinjoy, Deo, Ravinesh C. and Hopkins, Susan. 2023. "Accurate Image Multi-Class Classification Neural Network Model with Quantum Entanglement Approach." Sensors. 23 (5), pp. 1-11. https://doi.org/10.3390/s23052753Near real-time wind speed forecast model with bidirectional LSTM networks
Joseph, Lionel P., Deo, Ravinesh C., Prasad, Ramendra, Salcedo-Sanz, Sancho, Raj, Nawin and Soar, Jeffrey. 2023. "Near real-time wind speed forecast model with bidirectional LSTM networks." Renewable Energy. 204, pp. 39-58. https://doi.org/10.1016/j.renene.2022.12.123A novel global solar exposure forecasting model based on air temperature: Designing a new multi-processing ensemble deep learning paradigm
Jamei, Mehdi, Karbasi, Masoud, Ali, Mumtaz, Malik, Anurag, Chu, Xuefeng and Yaseen, Zaher Mundher. 2023. "A novel global solar exposure forecasting model based on air temperature: Designing a new multi-processing ensemble deep learning paradigm." Expert Systems with Applications. 222. https://doi.org/https://doi.org/10.1016/j.eswa.2023.119811Downscaling Surface Albedo to Higher Spatial Resolutions With an Image Super-Resolution Approach and PROBA-V Satellite Images
Deo, Ravinesh C., Karalasingham, Sagthitharan, Casillas-Perez, David, Raj, Narwin and Salcedo-sanz, Sancho. 2023. "Downscaling Surface Albedo to Higher Spatial Resolutions With an Image Super-Resolution Approach and PROBA-V Satellite Images." IEEE Access. 11, pp. 5558-5577. https://doi.org/10.1109/ACCESS.2023.3236253Design data decomposition-based reference evapotranspiration forecasting model: A soft feature filter based deep learning driven approach
Zheng, Zihao, Ali, Mumtaz, Jamei, Mehdi, Xiang, Yong, Karbasi, Masoud, Yaseen, Zaher Mundher and Farooque, Aitazaz Ahsan. 2023. "Design data decomposition-based reference evapotranspiration forecasting model: A soft feature filter based deep learning driven approach." Engineering Applications of Artificial Intelligence. 121. https://doi.org/10.1016/j.engappai.2023.105984Ensemble robust local mean decomposition integrated with random forest for short-term significant wave height forecasting
Ali, Mumtaz, Prasad, Ramendra, Xiang, Yong, Jamei, Mehdi and Yaseen, Zaher Mundher. 2023. "Ensemble robust local mean decomposition integrated with random forest for short-term significant wave height forecasting." Renewable Energy. 205, pp. 731-746. https://doi.org/10.1016/j.renene.2023.01.108Developing a novel hybrid method based on dispersion entropy and adaptive boosting algorithm for human activity recognition
Diykh, Mohammed, Abdulla, Shahab, Deo, Ravinesh C, Siuly, Siuly and Ali, Mumtaz. 2023. "Developing a novel hybrid method based on dispersion entropy and adaptive boosting algorithm for human activity recognition." Computer Methods and Programs in Biomedicine. 229. https://doi.org/10.1016/j.cmpb.2022.107305Cloud cover bias correction in numerical weather models for solar energy monitoring and forecasting systems with kernel ridge regression
Deo, Ravinesh C., Ahmed, A.A. Masrur, Casillas-Perez, David, Pourmousavi, S. Ali, Segal, Gary, Yu, Yanshan and Salcedo-sanz, Sancho. 2023. "Cloud cover bias correction in numerical weather models for solar energy monitoring and forecasting systems with kernel ridge regression." Renewable Energy. 203, pp. 113-130. https://doi.org/10.1016/j.renene.2022.12.048Development of a TVF-EMD-based multi-decomposition technique integrated with Encoder-Decoder-Bidirectional-LSTM for monthly rainfall forecasting
Jamei, Mehdi, Ali, Mumtaz, Malik, Anurag, Karbasi, Masoud, Rai, Priya and Yaseen, Zaher Mundher. 2023. "Development of a TVF-EMD-based multi-decomposition technique integrated with Encoder-Decoder-Bidirectional-LSTM for monthly rainfall forecasting." Journal of Hydrology. 617 (Part C). https://doi.org/10.1016/j.jhydrol.2023.129105Serial Cross-Sectional Observations of Sun-Protective Behaviors at an Annual Outdoor Motorsport Event in Tropical Queensland, Australia
Dexter, Ben, Smith, Annika, King, Rachel, Downs, Nathan J., Nikles, Catherine Jane, Parisi, Alfio V., Ho, Yik-Hong and Harrison, Simone Lee. 2023. "Serial Cross-Sectional Observations of Sun-Protective Behaviors at an Annual Outdoor Motorsport Event in Tropical Queensland, Australia ." Photochemistry and Photobiology. 99 (5), pp. 1352-1356. https://doi.org/10.1111/php.13773Deep Multi-Stage Reference Evapotranspiration Forecasting Model: Multivariate Empirical Mode Decomposition Integrated With the Boruta-Random Forest Algorithm
Jayasinghe, W. J. M. Lakmini Prarthana, Deo, Ravinesh C., Ghahramani, Afshin, Ghimire, Sujan and Raj, Nawin. 2021. "Deep Multi-Stage Reference Evapotranspiration Forecasting Model: Multivariate Empirical Mode Decomposition Integrated With the Boruta-Random Forest Algorithm." IEEE Access. 9, pp. 166695-166708. https://doi.org/10.1109/ACCESS.2021.3135362Academic Success
Bartlett, Cristy, Cawthray, Tyler, Clark, Linda, Conway, Sam, Derrington, Kate, Devi, Aruna, Frederiks, Anita, Gunton, Lyndelle, Hargreaves, Wendy, Howarth, Debi, Irvine, Sarah, Jeffers, Marjorie, Lovric, Kristen, McGregor, Rowena, Peters, Emma, Pickstone, Leigh, Retallick, Bianca, Rose, Yvonne, Sahay, Akshay, ..., Tweedale, Robyn. Hargreaves, Wendy and Andersen, Nikki (ed.) 2021. Academic Success. Australia. University of Southern Queensland.Satellite monitoring of environmental solar ultraviolet A (UVA) exposure and irradiance: a review of OMI and GOME-2
Parisi, Alfio V., Igoe, Damien, Downs, Nathan J., Turner, Joanna, Amar, Abdurazaq and A Jebar, Mustapha A.. 2021. "Satellite monitoring of environmental solar ultraviolet A (UVA) exposure and irradiance: a review of OMI and GOME-2." Remote Sensing. 13 (4), pp. 1-19. https://doi.org/10.3390/rs13040752Designing Deep-based Learning Flood Forecast Model with ConvLSTM Hybrid Algorithm
Moishin, Mohammed, Deo, Ravinesh C., Prasad, Ramendra, Raj, Nawin and Abdulla, Shahab. 2021. "Designing Deep-based Learning Flood Forecast Model with ConvLSTM Hybrid Algorithm." IEEE Access. 9, pp. 50982-50993. https://doi.org/10.1109/ACCESS.2021.3065939Pattern recognition describing spatio-temporal drivers of catchment classification for water quality
O'Sullivan, Cherie M., Ghahramani, Afshin, Deo, Ravinesh C. and Pembleton, Keith G.. 2023. "Pattern recognition describing spatio-temporal drivers of catchment classification for water quality." Science of the Total Environment. 861, pp. 1-42. https://doi.org/10.1016/j.scitotenv.2022.160240The Playground Shade Index: A New Design Metric for Measuring Shade and Seasonal Ultraviolet Protection Characteristics of Parks and Playgrounds
Downs, Nathan, Raj, Nawin, Vanos, Jennifer, Parisi, Alfio, Butler, Harry, Deo, Ravinesh, Igoe, Damien, Dexter, Benjamin, Beckman-Downs, Melanie, Turner, Joanna and Dekeyser, Stijn. 2023. "The Playground Shade Index: A New Design Metric for Measuring Shade and Seasonal Ultraviolet Protection Characteristics of Parks and Playgrounds." Photochemistry and Photobiology. 99 (4), pp. 1193-1207. https://doi.org/10.1111/php.13745Queensland Guidelines for the provision of tree shade on suburban nature-strips
Downs, Nathan. 2022. Queensland Guidelines for the provision of tree shade on suburban nature-strips. Toowoomba, Australia. University of Southern Queensland.Using Sequence-to-Sequence Models for Carrier Frequency Offset Estimation of Short Messages and Chaotic Maps
Davey, Christopher P., Shakeel, Ismail, Deo, Ravinesh C., Salcedo-sanz, Sancho and Soar, Jeffrey. 2022. "Using Sequence-to-Sequence Models for Carrier Frequency Offset Estimation of Short Messages and Chaotic Maps." IEEE Access. 10, pp. 119814 - 119825. https://doi.org/10.1109/ACCESS.2022.3221762Developing a novel hybrid Auto Encoder Decoder Bidirectional Gated Recurrent Unit model enhanced with empirical wavelet transform and Boruta-Catboost to forecast significant wave height
Karbasi, Masoud, Jamei, Mehdi, Ali, Mumtaz, Abdulla, Shahab, Chu, Xuefeng and Yaseen, Zaher Mundher. 2022. "Developing a novel hybrid Auto Encoder Decoder Bidirectional Gated Recurrent Unit model enhanced with empirical wavelet transform and Boruta-Catboost to forecast significant wave height." Journal of Cleaner Production. 379 (Part 2). https://doi.org/10.1016/j.jclepro.2022.134820Modelling and Real-time Optimisation of Air Quality Predictions for Australia through Artificial Intelligence Algorithm
Sharma, Ekta, Deo, Ravinesh C., Prasad, Ramendra and Parisi, Alfio V.. 2019. "Modelling and Real-time Optimisation of Air Quality Predictions for Australia through Artificial Intelligence Algorithm." AMSI Optimise 2019. Perth, Australia 17 - 21 Jun 2019 Perth, Australia.Hybrid Convolutional Neural Network-Multilayer Perceptron Model for Solar Radiation Prediction
Ghimire, Sujan, Nguyen-Huy, Thong, Prasad, Ramendra, Deo, Ravinesh C., Casillas-Perez, David, Salcedo-sanz, Sancho and Bhandari, Binayak. 2023. "Hybrid Convolutional Neural Network-Multilayer Perceptron Model for Solar Radiation Prediction." Cognitive Computation. 15 (2), pp. 645-671. https://doi.org/10.1007/s12559-022-10070-yStudent Performance Predictions for Advanced Engineering Mathematics Course With New Multivariate Copula Models
Nguyen-Huy, Thong, Deo, Ravinesh C., Khan, Shahjahan, Devi, Aruna, Adeyinka, Adewuyi Ayodele, Apan, Armando A. and Yaseen, Zaher Mundher. 2022. "Student Performance Predictions for Advanced Engineering Mathematics Course With New Multivariate Copula Models." IEEE Access. 10, pp. 45112 -45136. https://doi.org/10.1109/ACCESS.2022.3168322Rapid assessment of mine rehabilitation areas with airborne LiDAR and deep learning: bauxite strip mining in Queensland, Australia
Murray, Xavier, Apan, Armando, Deo, Ravinesh and Maraseni, Tek. 2022. "Rapid assessment of mine rehabilitation areas with airborne LiDAR and deep learning: bauxite strip mining in Queensland, Australia." Geocarto International. 37 (26), pp. 11223-11252. https://doi.org/10.1080/10106049.2022.2048902Multi-strategy Slime Mould Algorithm for hydropower multi-reservoir systems optimization
Ahmadianfar, Iman, Noori, Ramzia Majeed, Togun, Hussein, Falah, Mayadah W., Homod, Raad Z., Fu, Minglei, Halder, Bijay, Deo, Ravinesh and Yaseen, Zaher Mundher. 2022. "Multi-strategy Slime Mould Algorithm for hydropower multi-reservoir systems optimization." Knowledge-Based Systems. 250, pp. 1-18. https://doi.org/10.1016/j.knosys.2022.109048Suspended sediment load modeling using advanced hybrid rotation forest based elastic network approach
Khosravi, Khabat, Golkarian, Ali, Melesse, Assefa M. and Deo, Ravinesh C.. 2022. "Suspended sediment load modeling using advanced hybrid rotation forest based elastic network approach." Journal of Hydrology. 610, pp. 1-14. https://doi.org/10.1016/j.jhydrol.2022.127963Delineating the Crop-Land Dynamic due to Extreme Environment Using Landsat Datasets: A Case Study
Halder, Bijay, Bandyopadhyay, Jatisankar, Afan, Haitham Abdulmohsin, Naser, Maryam H., Abed, Salwan Ali, Khedher, Khaled Mohamed, Falih, Khaldoon T., Deo, Ravinesh, Scholz, Miklas and Yaseen, Zaher Mundher. 2022. "Delineating the Crop-Land Dynamic due to Extreme Environment Using Landsat Datasets: A Case Study." Agronomy. 12 (6), pp. 1-23. https://doi.org/10.3390/agronomy12061268Coupled online sequential extreme learning machine model with ant colony optimization algorithm for wheat yield prediction
Ali, Mumtaz, Deo, Ravinesh C., Xiang, Yong, Prasad, Ramendra, Li, Jianxin, Farooque, Aitazaz and Yaseen, Zaher Mundher. 2022. "Coupled online sequential extreme learning machine model with ant colony optimization algorithm for wheat yield prediction." Scientific Reports. 12 (1), pp. 1-23. https://doi.org/10.1038/s41598-022-09482-5Forecasting solar photosynthetic photon flux density under cloud cover effects: novel predictive model using convolutional neural network integrated with long short-term memory network
Deo, Ravinesh C., Grant, Richard H., Webb, Ann, Ghimire, Sujan, Igoe, Damien P., Downs, Nathan J., Al-Musaylh, Mohanad S., Parisi, Alfio V. and Soar, Jeffrey. 2022. "Forecasting solar photosynthetic photon flux density under cloud cover effects: novel predictive model using convolutional neural network integrated with long short-term memory network." Stochastic Environmental Research and Risk Assessment. 36, p. 3183–3220. https://doi.org/10.1007/s00477-022-02188-0Improved Complete Ensemble Empirical Mode Decomposition with Adaptive Noise Deep Residual model for short-term multi-step solar radiation prediction
Ghimire, Sujan, Deo, Ravinesh C, Casillas-Perez, David and Salcedo-sanz, Sancho. 2022. "Improved Complete Ensemble Empirical Mode Decomposition with Adaptive Noise Deep Residual model for short-term multi-step solar radiation prediction." Renewable Energy. 190, pp. 408-424. https://doi.org/10.1016/j.renene.2022.03.120Efficient daily solar radiation prediction with deep learning 4-phase convolutional neural network, dual stage stacked regression and support vector machine CNN-REGST hybrid model
Ghimire, Sujan, Nguyen-Huy, Thong, Deo, Ravinesh C., Casillas-Perez, David and Salcedo-sanz, Sancho. 2022. "Efficient daily solar radiation prediction with deep learning 4-phase convolutional neural network, dual stage stacked regression and support vector machine CNN-REGST hybrid model." Sustainable Materials and Technologies. 32, pp. 1-24. https://doi.org/10.1016/j.susmat.2022.e00429Hybrid deep CNN-SVR algorithm for solar radiation prediction problems in Queensland, Australia
Ghimire, Sujan, Bhandari, Binayak, Casillas-Perez, David, Deo, Ravinesh C. and Salcedo-sanz, Sancho. 2022. "Hybrid deep CNN-SVR algorithm for solar radiation prediction problems in Queensland, Australia." Engineering Applications of Artificial Intelligence. 112, pp. 1-26. https://doi.org/10.1016/j.engappai.2022.104860Boosting solar radiation predictions with global climate models, observational predictors and hybrid deep-machine learning algorithms
Ghimire, Sujan, Deo, Ravinesh C., Casillas-Perez, David and Salcedo-sanz, Sancho. 2022. "Boosting solar radiation predictions with global climate models, observational predictors and hybrid deep-machine learning algorithms." Applied Energy. 316, pp. 1-25. https://doi.org/10.1016/j.apenergy.2022.119063Machine learning regression and classification methods for fog events prediction
Castillo-Boton, C., Casillas-Perez, D., Casanova-Mateo, C., Ghimire, S., Cerro-Prada, E., Gutierrez, P. A., Deo, R. C. and Salcedo-sanz, S.. 2022. "Machine learning regression and classification methods for fog events prediction." Atmospheric Research. 272, pp. 1-23. https://doi.org/10.1016/j.atmosres.2022.106157Quantum Artificial Intelligence Predictions Enhancement by Improving Signal Processing
Riaz, Farina, Abdulla, Shahab, Ni, Wei, Radfar, Mohsen, Deo, Ravinesh and Hopkins, Susan. 2022. "Quantum Artificial Intelligence Predictions Enhancement by Improving Signal Processing." Quantum Australia Conference 2022. Online 23 - 25 Feb 2022 Toowoomba, Australia. https://doi.org/10.13140/RG.2.2.34754.66245Novel nested patch-based feature extraction model for automated Parkinson’s Disease symptom classification using MRI images
Kaplan, Ela, Altunisik, Erman, Firat, Yasemin Ekmekyapar, Barua, Prabal Datta, Dogan, Sengul, Baygin, Mehmet, Demir, Fahrettin Burak, Tuncer, Turker, Palmer, Elizabeth, Tan, Ru-San, Yu, Ping, Soar, Jeffrey, Fujita, Hamido and Acharya, U. Rajendra. 2022. "Novel nested patch-based feature extraction model for automated Parkinson’s Disease symptom classification using MRI images." Computer Methods and Programs in Biomedicine. 224, pp. 1-11. https://doi.org/10.1016/j.cmpb.2022.107030Forecasting Daily Flood Water Level Using Hybrid Advanced Machine Learning Based Time‑Varying Filtered Empirical Mode Decomposition Approach
Jamei, Mehdi, Ali, Mumtaz, Malik, Anurag, Prasad, Ramendra, Abdulla, Shahab and Yaseen, Zaher Mundher. 2022. "Forecasting Daily Flood Water Level Using Hybrid Advanced Machine Learning Based Time‑Varying Filtered Empirical Mode Decomposition Approach." Water Resources Management. 36 (12), p. 4637–4676. https://doi.org/10.1007/s11269-022-03270-6Deep learning CNN-LSTM-MLP hybrid fusion model for feature optimizations and daily solar radiation prediction
Ghimire, Sujan, Deo, Ravinesh C., Casillas-Perez, David, Salcedo-sanz, Sancho, Sharma, Ekta and Ali, Mumtaz. 2022. "Deep learning CNN-LSTM-MLP hybrid fusion model for feature optimizations and daily solar radiation prediction." Measurement. 202, pp. 1-22. https://doi.org/10.1016/j.measurement.2022.111759Basin management inspiration from impacts of alternating dry and wet conditions on water production and carbon uptake in Murray-Darling Basin
Lu, Zhixiang, Feng, Qi, Wei, Yongping, Zhao, Yan, Deo, Ravinesh C., Xie, Jiali, Zhou, Sha, Zhu, Meng and Xu, Min. 2022. "Basin management inspiration from impacts of alternating dry and wet conditions on water production and carbon uptake in Murray-Darling Basin." Science of the Total Environment. 851 (Part 2), pp. 1-8. https://doi.org/10.1016/j.scitotenv.2022.158359Kernel Ridge Regression Hybrid Method for Wheat Yield Prediction with Satellite-Derived Predictors
Ahmed, A. A. Masrur, Sharma, Ekta, Jui, S. Janifer Jabin, Deo, Ravinesh C., Nguyen-Huy, Thong and Ali, Mumtaz. 2022. "Kernel Ridge Regression Hybrid Method for Wheat Yield Prediction with Satellite-Derived Predictors." Remote Sensing. 14 (5), pp. 1-24. https://doi.org/10.3390/rs14051136Cloud Affected Solar UV Predictions with Three-Phase Wavelet Hybrid Convolutional Long Short-Term Memory Network Multi-Step Forecast System
Prasad, Salvin S., Deo, Ravinesh C., Downs, Nathan, Igoe, Damien, Parisi, Alfio V. and Soar, Jeffrey. 2022. "Cloud Affected Solar UV Predictions with Three-Phase Wavelet Hybrid Convolutional Long Short-Term Memory Network Multi-Step Forecast System." IEEE Access. 10, pp. 24704-24720. https://doi.org/10.1109/ACCESS.2022.3153475Development and evaluation of hybrid deep learning long short-term memory network model for pan evaporation estimation trained with satellite and ground-based data
Jayasinghe, W. J. M. Lakmini Prarthana, Deo, Ravinesh C., Ghahramani, Afshin, Ghimire, Sujan and Raj, Nawin. 2022. "Development and evaluation of hybrid deep learning long short-term memory network model for pan evaporation estimation trained with satellite and ground-based data." Journal of Hydrology. 607, pp. 1-19. https://doi.org/10.1016/j.jhydrol.2022.127534Integrative artificial intelligence models for Australian coastal sediment lead prediction: An investigation of in-situ measurements and meteorological parameters effects
Bhagat, Suraj Kumar, Tiyasha, Tiyasha, Kumar, Adarsh, Malik, Tabarak, Jawad, Ali H., Khedher, Khaled Mohamed, Deo, Ravinesh C. and Yaseen, Zaher Mundher. 2022. "Integrative artificial intelligence models for Australian coastal sediment lead prediction: An investigation of in-situ measurements and meteorological parameters effects." Journal of Environmental Management. 309, pp. 1-16. https://doi.org/10.1016/j.jenvman.2022.114711Assessment and Prediction of Sea Level Trend in the South Pacific Region
Raj, Nawin, Gharineiat, Zahra, Ahmed, Abul Abrar Masrur and Stepanyants, Yury. 2022. "Assessment and Prediction of Sea Level Trend in the South Pacific Region." Remote Sensing. 14 (4), pp. 1-25. https://doi.org/10.3390/rs14040986An Eigenvalues-Based Covariance Matrix Bootstrap Model Integrated With Support Vector Machines for Multichannel EEG Signals Analysis
Al-Hadeethi, Hanan, Abdulla, Shahab, Diykh, Mohammed, Deo, Ravinesh C. and Green, Jonathan H.. 2022. "An Eigenvalues-Based Covariance Matrix Bootstrap Model Integrated With Support Vector Machines for Multichannel EEG Signals Analysis." Frontiers in Neuroinformatics. 15, pp. 1-15. https://doi.org/10.3389/fninf.2021.808339Stacked LSTM Sequence-to-Sequence Autoencoder with Feature Selection for Daily Solar Radiation Prediction: A Review and New Modeling Results
Ghimire, Sujan, Deo, Ravinesh C., Wang, Hua, Al-Musaylh, Mohanad S., Casillas-Perez, David and Salcedo-sanz, Sancho. 2022. "Stacked LSTM Sequence-to-Sequence Autoencoder with Feature Selection for Daily Solar Radiation Prediction: A Review and New Modeling Results." Energies. 15 (3), pp. 1-39. https://doi.org/10.3390/en15031061Texture analysis based graph approach for automatic detection of neonatal seizure from multi-channel EEG signals
Diykh, Mohammed, Miften, Firas Sabar, Abdulla, Shahab, Deo, Ravinesh C., Siuly, Siuly, Green, Jonathan H. and Oudah, Atheer Y.. 2022. "Texture analysis based graph approach for automatic detection of neonatal seizure from multi-channel EEG signals." Measurement. 190 (110731), pp. 1-13. https://doi.org/10.1016/j.measurement.2022.110731Air quality monitoring based on chemical and meteorological drivers: Application of a novel data filtering-based hybridized deep learning model
Jamei, Mehdi, Ali, Mumtaz, Malik, Anurag, Karbasi, Masoud, Sharma, Ekta and Yaseen, Zaher Mundher. 2022. "Air quality monitoring based on chemical and meteorological drivers: Application of a novel data filtering-based hybridized deep learning model." Journal of Cleaner Production. 374, pp. 1-17. https://doi.org/10.1016/j.jclepro.2022.134011Classification of catchments for nitrogen using Artificial Neural Network Pattern Recognition and spatial data
O'Sullivan, Cherie M., Ghahramani, Afshin, Deo, Ravinesh C., Pembleton, Keith, Khan, Urooj and Tuteja, Narendra. 2022. "Classification of catchments for nitrogen using Artificial Neural Network Pattern Recognition and spatial data." Science of the Total Environment. 809, pp. 1-15. https://doi.org/10.1016/j.scitotenv.2021.151139The Learning Centre: Supporting student success
Kek, Megan, Padro, Fernando, Kimmins, Lindy, Frederiks, Anita, Ayriss, Peter, Emmerson, Mark, Thangavelu, Anbarasu, Dickson, Bronwen, McCarthy, Elizabeth, Devi, Aruna, Eacersall, Douglas and Atwell, Brenda. 2016. "The Learning Centre: Supporting student success." Learning and Teaching Celebration. Toowoomba, Australia 2016 Toowoomba, Australia.Meet-Up Program: Peer learning for success
Kimmins, Lindy, Eacersall, Douglas, Devi, Aruna, Kek, Megan, Frederiks, Anita, Emmerson, Mark, Thangavelu, Eddie, Dickson, Bronwen and McCarthy, Elizabeth. 2016. "Meet-Up Program: Peer learning for success." Learning and Teaching Celebration. Toowoomba, Australia 2016 Toowoomba, Australia.Domino effect of climate change over two millennia in ancient China’s Hexi Corridor
Feng, Qi, Yan, Linshan, Deo, Ravinesh C., AghaKouchak, Amir, Adamowski, Jan F., Stone, Roger, Yin, Zhenliang, Liu, Wei, Si, Jianhua, Wen, Xiaohu, Zhu, Meng and Cao, Shixiong. 2019. "Domino effect of climate change over two millennia in ancient China’s Hexi Corridor." Nature Sustainability. 2, pp. 957-961. https://doi.org/10.1038/s41893-019-0397-9Bat algorithm for dam–reservoir operation
Ethteram, Mohammad, Mousavi, Sayed-Farhad, Karami, Hojat, Farzin, Saeed, Deo, Ravinesh, Othman, Faridah Binti, Chau, Kwok-Wing, Sarkamaryan, Saeed, Singh, Vijay P. and El-Shafie, Ahmed. 2018. "Bat algorithm for dam–reservoir operation." Environmental Earth Sciences. 77 (13), pp. 1-15. https://doi.org/10.1007/s12665-018-7662-5Characteristics of ecosystem water use efficiency in a desert riparian forest
Ma, Xiaohong, Feng, Qi, Su, Yonghong, Yu, Tengfei and Deo, Ravinesh C.. 2018. "Characteristics of ecosystem water use efficiency in a desert riparian forest." Environmental Earth Sciences. 77 (358). https://doi.org/10.1007/s12665-018-7518-zThe influence of climatic inputs on stream-flow pattern forecasting: case study of Upper Senegal River
Diop, Lamine, Bodian, Ansoumana, Djaman, Koffi, Yaseen, Zaher Mundher, Deo, Ravinesh C., El-Shafie, Ahmed and Brown, Larry C.. 2018. "The influence of climatic inputs on stream-flow pattern forecasting: case study of Upper Senegal River." Environmental Earth Sciences. 77 (5). https://doi.org/10.1007/s1266Uncertainty assessment of the multilayer perceptron (MLP) neural network model with implementation of the novel hybrid MLP-FFA method for prediction of biochemical oxygen demand and dissolved oxygen: a case study of Langat River
Raheli, Bahare, Aalami, Mohammad Taghi, El-Shafie, Ahmed, Ghorbani, Mohammad Ali and Deo, Ravinesh C.. 2017. "Uncertainty assessment of the multilayer perceptron (MLP) neural network model with implementation of the novel hybrid MLP-FFA method for prediction of biochemical oxygen demand and dissolved oxygen: a case study of Langat River." Environmental Earth Sciences. 76 (14). https://doi.org/10.1007/s12665-017-6842-zNovel hybrid deep learning model for satellite based PM10 forecasting in the most polluted Australian hotspots
Sharma, Ekta, Deo, Ravinesh C., Soar, Jeffrey, Prasad, Ramendra, Parisi, Alfio V. and Raj, Nawin. 2022. "Novel hybrid deep learning model for satellite based PM10 forecasting in the most polluted Australian hotspots." Atmospheric Environment. 279, pp. 1-13. https://doi.org/10.1016/j.atmosenv.2022.119111New double decomposition deep learning methods for river water level forecasting
Ahmed, A. A. Masrur, Deo, Ravinesh C., Ghahramani, Afshin, Feng, Qi, Raj, Nawin, Yin, Zhenliang and Yang, Linshan. 2022. "New double decomposition deep learning methods for river water level forecasting." Science of the Total Environment. 831, pp. 1-21. https://doi.org/10.1016/j.scitotenv.2022.154722A Case Study of UV Exposure Risk in Sydney during the 2019/2020 New South Wales Bushfires
Igoe, Damien P., Parisi, Alfio V., Downs, Nathan J. and Butler, Harry. 2022. "A Case Study of UV Exposure Risk in Sydney during the 2019/2020 New South Wales Bushfires." Photochemistry and Photobiology. 98 (5), pp. 1236-1244. https://doi.org/10.1111/php.13603Spatiotemporal Hybrid Random Forest Model for Tea Yield Prediction Using Satellite-Derived Variables
Jui, S. Janifer Jabin, Ahmed, A. A. Masrur, Bose, Aditi, Raj, Nawin, Sharma, Ekta, Soar, Jeffrey and Chowdhury, Md Wasique Islam. 2022. "Spatiotemporal Hybrid Random Forest Model for Tea Yield Prediction Using Satellite-Derived Variables." Remote Sensing. 14 (3), pp. 1-18. https://doi.org/10.3390/rs14030805Application of CycleGAN and transfer learning techniques for automated detection of COVID-19 using X-ray images
Bargshady, Ghazal, Zhou, Xujuan, Barua, Prabal Datta, Gururajan, Raj, Li, Yuefeng and Acharya, U. Rajendra. 2022. "Application of CycleGAN and transfer learning techniques for automated detection of COVID-19 using X-ray images." Pattern Recognition Letters. 153, pp. 67-74. https://doi.org/10.1016/j.patrec.2021.11.020Efficient Heat Transfer Augmentation in Channels with Semicircle Ribs and Hybrid Al2O3-Cu/Water Nanofluids
Togun, Hussein, Homod, Raad Z., Yaseen, Zaher Mundher, Abed, Azher M., Dhabab, Jameel M., Ibrahem, Raed Khalid, Dhahbi, Sami, Rashidi, Mohammad Mehdi, Ahmadi, Goodarz, Yaïci, Wahiba and Mahdi, Jasim M.. 2022. "Efficient Heat Transfer Augmentation in Channels with Semicircle Ribs and Hybrid Al2O3-Cu/Water Nanofluids." Nanomaterials. 12 (15). https://doi.org/10.3390/nano12152720Improved Fitness-Dependent Optimizer for Solving Economic Load Dispatch Problem
Tahir, Barzan Hussein, Rashid, Tarik A., Rauf, Hafiz Tayyab, Bacanin, Nebojsa, Chhabra, Amit, Vimal, S. and Yaseen, Zaher Mundher. 2022. "Improved Fitness-Dependent Optimizer for Solving Economic Load Dispatch Problem." Computational Intelligence and Neuroscience. 2022. https://doi.org/10.1155/2022/7055910Natural Time Series Parameters Forecasting: Validation of the Pattern-Sequence-Based Forecasting (PSF) Algorithm; A New Python Package
Shende, Mayur Kishor, Salih, Sinan Q., Bokde, Neeraj Dhanraj, Scholz, Miklas, Oudah, Atheer Y. and Yaseen, Zaher Mundher. 2022. "Natural Time Series Parameters Forecasting: Validation of the Pattern-Sequence-Based Forecasting (PSF) Algorithm; A New Python Package." Applied Sciences. 12 (12). https://doi.org/10.3390/app12126194Integration of Multiple Models with Hybrid Artificial Neural Network-Genetic Algorithm for Soil Cation-Exchange Capacity Prediction
Shahabi, Mahmood, Ghorbani, Mohammad Ali, Naganna, Sujay Raghavendra, Kim, Sungwon, Hadi, Sinan Jasim, Inyurt, Samed, Farooque, Aitazaz Ahsan and Yaseen, Zaher Mundher. 2022. "Integration of Multiple Models with Hybrid Artificial Neural Network-Genetic Algorithm for Soil Cation-Exchange Capacity Prediction." Complexity. 2022. https://doi.org/10.1155/2022/3123475Coupled data pre-processing approach with data intelligence models for monthly precipitation forecasting
Nikpour, M. R., Abdollahi, S., Sanikhani, H., Raeisi, J. and Yaseen, Z. M.. 2022. "Coupled data pre-processing approach with data intelligence models for monthly precipitation forecasting." International Journal of Environmental Science and Technology. 19 (12), pp. 11919-11934. https://doi.org/10.1007/s13762-022-04395-2Automated emotion recognition: Current trends and future perspectives
Maithri, M., Raghavendra, U., Gudigar, Anjan, Samanth, Jyothi, Barua, Prabal Datta, Murugappan, Murugappan, Chakole, Yashas and Acharya, U. Rajendra. 2022. "Automated emotion recognition: Current trends and future perspectives." Computer Methods and Programs in Biomedicine. 215. https://doi.org/10.1016/j.cmpb.2022.106646DKPNet41: Directed knight pattern network-based cough sound classification model for automatic disease diagnosis
Kuluozturk, Mutlu, Kobat, Mehmet Ali, Barua, Prabal Datta, Dogan, Sengul, Tuncer, Turker, Tan, Ru-San, Ciaccio, Edward J. and Acharya, U Rajendra. 2022. "DKPNet41: Directed knight pattern network-based cough sound classification model for automatic disease diagnosis." Medical Engineering and Physics. 110. https://doi.org/10.1016/j.medengphy.2022.103870ViVGG19: Novel exemplar deep feature extraction-based shoulder rotator cuff tear and biceps tendinosis detection using magnetic resonance images
Key, Sefa, Demir, Sukru, Gurger, Murat, Yilmaz, Erhan, Barua, Prabal Datta, Dogan, Sengul, Tuncer, Turker, Arunkumar, N., Tan, Ru-San and Acharya, U Rajendra. 2022. "ViVGG19: Novel exemplar deep feature extraction-based shoulder rotator cuff tear and biceps tendinosis detection using magnetic resonance images." Medical Engineering and Physics. 110. https://doi.org/10.1016/j.medengphy.2022.103864Reference evapotranspiration prediction using high-order response surface method
Keshtegar, Behrooz, Abdullah, Shafika Sultan, Huang, Yuk Feng, Saggi, Mandeep Kaur, Khedher, Khaled Mohamed and Yaseen, Zaher Mundher. 2022. "Reference evapotranspiration prediction using high-order response surface method." Theoretical and Applied Climatology. 148 (1-2), pp. 849-867. https://doi.org/10.1007/s00704-022-03954-4PFP-LHCINCA: Pyramidal Fixed-Size Patch-Based Feature Extraction and Chi-Square Iterative Neighborhood Component Analysis for Automated Fetal Sex Classification on Ultrasound Images
Kaplan, Ela, Ekinci, Tekin, Kaplan, Selcuk, Barua, Prabal Datta, Dogan, Sengul, Tuncer, Turker, Tan, Ru-San, Arunkumar, N and Acharya, U. Rajendra. 2022. "PFP-LHCINCA: Pyramidal Fixed-Size Patch-Based Feature Extraction and Chi-Square Iterative Neighborhood Component Analysis for Automated Fetal Sex Classification on Ultrasound Images." Contrast Media and Molecular Imaging. 2022. https://doi.org/10.1155/2022/6034971Mesoporous high-surface-area activated carbon from biomass waste via microwave-assisted-H3PO4 activation for methylene blue dye adsorption: An optimized process
Jawad, Ali H., Malek, Nurul Najwa Abd, Khadiran, Tumirah, ALOthman, Zeid A. and Yaseen, Zaher Mundher. 2022. "Mesoporous high-surface-area activated carbon from biomass waste via microwave-assisted-H3PO4 activation for methylene blue dye adsorption: An optimized process." Diamond and Related Materials. 128. https://doi.org/10.1016/j.diamond.2022.109288Long-term multi-step ahead forecasting of root zone soil moisture in different climates: Novel ensemble-based complementary data-intelligent paradigms
Jamei, Mehdi, Karbasi, Masoud, Malik, Anurag, Jamei, Mozhdeh, Jamei, Mozhdeh, Kisi, Ozgur and Yaseen, Zaher Mundher. 2022. "Long-term multi-step ahead forecasting of root zone soil moisture in different climates: Novel ensemble-based complementary data-intelligent paradigms." Agricultural Water Management. 269. https://doi.org/10.1016/j.agwat.2022.107679Earth skin temperature long-term prediction using novel extended Kalman filter integrated with Artificial Intelligence models and information gain feature selection
Jamei, Mehdi, Karbasi, Masoud, Alawi, Omer A., Kamar, Haslinda Mohamed, Khedher, Khaled Mohamed, Abba, S.I. and Yaseen, Zaher Mundher. 2022. "Earth skin temperature long-term prediction using novel extended Kalman filter integrated with Artificial Intelligence models and information gain feature selection." Sustainable Computing: Informatics and Systems . 35. https://doi.org/10.1016/j.suscom.2022.100721Distributed Hydrological Model Based on Machine Learning Algorithm: Assessment of Climate Change Impact on Floods
Iqbal, Zafar, Shahid, Shamsuddin, Ismail, Tarmizi, Sa’adi, Zulfaqar, Farooque, Aitazaz and Yaseen, Zaher Mundher. 2022. "Distributed Hydrological Model Based on Machine Learning Algorithm: Assessment of Climate Change Impact on Floods." Sustainability. 14 (11). https://doi.org/10.3390/su14116620Dynamics analysis of a novel hybrid deep clustering for unsupervised learning by reinforcement of multi-agent to energy saving in intelligent buildings
Homod, Raad Z., Togun, Hussein, Hussein, Ahmed Kadhim, Al-Mousawi, Fadhel Noraldeen, Yaseen, Zaher Mundher, Al-Kouz, Wael, Abd, Haider J., Alawi, Omer A., Goodarzi, Marjan and Hussein, Omar A.. 2022. "Dynamics analysis of a novel hybrid deep clustering for unsupervised learning by reinforcement of multi-agent to energy saving in intelligent buildings." Applied Energy. 313. https://doi.org/10.1016/j.apenergy.2022.118863An innovative clustering technique to generate hybrid modeling of cooling coils for energy analysis: A case study for control performance in HVAC systems
Homod, Raad Z., Togun, Hussein, Ateeq, Adnan A., Al-Mousawi, Fadhel Noraldeen, Yaseen, Zaher Mundher, Al-Kouz, Wael, Hussein, Ahmed Kadhim, Alawi, Omer A., Goodarzi, Marjan and Ahmadi, Goodarz. 2022. "An innovative clustering technique to generate hybrid modeling of cooling coils for energy analysis: A case study for control performance in HVAC systems." Renewable and Sustainable Energy Reviews. 166. https://doi.org/10.1016/j.rser.2022.112676Cyanobacteria blue-green algae prediction enhancement using hybrid machine learning–based gamma test variable selection and empirical wavelet transform
Heddam, Salim, Yaseen, Zaher Mundher, Falah, Mayadah W., Goliatt, Leonardo, Tan, Mou Leong, Sa’adi, Zulfaqar, Ahmadianfar, Iman, Saggi, Mandeep, Bhatia, Amandeep and Samui, Pijush. 2022. "Cyanobacteria blue-green algae prediction enhancement using hybrid machine learning–based gamma test variable selection and empirical wavelet transform." Environmental Science and Pollution Research. 29 (51), pp. 77157-77187. https://doi.org/10.1007/s11356-022-21201-1Temperature and precipitation trend analysis of the Iraq Region under SRES scenarios during the twenty-first century
Hashim, Bassim Mohammed, Maliki, Ali Al, Alraheem, Esam Abd, Al-Janabi, Ahmed Mohammed Sami, Halder, Bijay and Yaseen, Zaher Mundher. 2022. "Temperature and precipitation trend analysis of the Iraq Region under SRES scenarios during the twenty-first century." Theoretical and Applied Climatology. 148 (3-4), pp. 881-898. https://doi.org/10.1007/s00704-022-03976-yDelineation of urban expansion and drought-prone areas using vegetation conditions and other geospatial indices
Halder, Bijay, Tiyasha, Tiyasha, Shahid, Shamsuddin and Yaseen, Zaher Mundher. 2022. "Delineation of urban expansion and drought-prone areas using vegetation conditions and other geospatial indices." Theoretical and Applied Climatology. 149 (3-4), pp. 1277-1295. https://doi.org/10.1007/s00704-022-04108-2Investigating the relationship between land alteration and the urban heat island of Seville city using multi-temporal Landsat data
Halder, Bijay, Karimi, Alireza, Mohammad, Pir, Bandyopadhyay, Jatisankar, Brown, Robert D. and Yaseen, Zaher Mundher. 2022. "Investigating the relationship between land alteration and the urban heat island of Seville city using multi-temporal Landsat data." Theoretical and Applied Climatology. 150 (1-2), pp. 613-635. https://doi.org/10.1007/s00704-022-04180-8The impact of climate change on land degradation along with shoreline migration in Ghoramara Island, India
Halder, Bijay, Ameen, Ameen Mohammed Salih, Bandyopadhyay, Jatisankar, Khedher, Khaled Mohamed and Yaseen, Zaher Mundher. 2022. "The impact of climate change on land degradation along with shoreline migration in Ghoramara Island, India." Physics and Chemistry of the Earth. 126. https://doi.org/10.1016/j.pce.2022.103135Grasshopper Optimization Algorithm with Crossover Operators for Feature Selection and Solving Engineering Problems
Ewees, Ahmed A., Gaheen, Marwa A., Yaseen, Zaher Mundher Yaseen and Ghoniem, Rania M.. 2022. "Grasshopper Optimization Algorithm with Crossover Operators for Feature Selection and Solving Engineering Problems." IEEE Access. 10, pp. 23304-23320. https://doi.org/10.1109/ACCESS.2022.3153038Application of the ANOVA method in the optimization of a thermoelectric cooler-based dehumidification system
Eltaweel, Mahmoud, Heggy, Aya H., Yaseen, Zaher Mundher, Alawi, Omer A., Falah, Mayadah W., Hussein, Omar A., Ahmed, Waqar, Homod, Raad Z. and Abdelrazek, Ali H.. 2022. "Application of the ANOVA method in the optimization of a thermoelectric cooler-based dehumidification system." Energy Reports. 8, pp. 10533-10545. https://doi.org/10.1016/j.egyr.2022.08.193Assessing the Efficiency of Remote Sensing and Machine Learning Algorithms to Quantify Wheat Characteristics in the Nile Delta Region of Egypt
Elmetwalli, Adel H., Mazrou, Yasser S. A., Tyler, Andrew N., Hunter, Peter D., Elsherbiny, Osama, Yaseen, Zaher Mundher and Elsayed, Salah. 2022. "Assessing the Efficiency of Remote Sensing and Machine Learning Algorithms to Quantify Wheat Characteristics in the Nile Delta Region of Egypt." Agriculture. 12 (3). https://doi.org/10.3390/agriculture12030332Households’ perceptions and socio-economic determinants of climate change awareness: Evidence from Selangor Coast Malaysia
Ehsan, Sofia, Begum, Rawshan Ara, Maulud, Khairul Nizam Abdul and Yaseen, Zaher Mundher. 2022. "Households’ perceptions and socio-economic determinants of climate change awareness: Evidence from Selangor Coast Malaysia." Journal of Environmental Management. 316. https://doi.org/10.1016/j.jenvman.2022.115261Artificial Intelligence Enabled Personalised Assistive Tools to Enhance Education of Children with Neurodevelopmental Disorders—A Review
Barua, Prabal Datta, Jahmunah, Jahmunah, Gururajan, Raj, Oh, Shu Lih, Palmer, Elizabeth, Azizan, Muhammad Mokhzaini, Kadri, Nahrizul Adib and Acharya, U. Rajendra. 2022. "Artificial Intelligence Enabled Personalised Assistive Tools to Enhance Education of Children with Neurodevelopmental Disorders—A Review ." International Journal of Environmental Research and Public Health. 19 (3). https://doi.org/10.3390/ijerph19031192Hybrid Deep Feature Generation for Appropriate Face Mask Use Detection
Aydemir, Emrah, Yalcinkaya, Mehmet Ali, Barua, Prabal Datta, Baygin, Mehmet, Faust, Oliver, Dogan, Sengul, Chakraborty, Subrata, Tuncer, Turker and Acharya, Rajendra. 2022. "Hybrid Deep Feature Generation for Appropriate Face Mask Use Detection." International Journal of Environmental Research and Public Health. 19 (4). https://doi.org/10.3390/ijerph19041939Optimization algorithms as training approach with hybrid deep learning methods to develop an ultraviolet index forecasting model
Ahmed, A. A. Masrur, Ahmed, Mohammad Hafez, Saha, Sanjoy Kanti, Ahmed, Oli and Sutradhar, Ambica. 2022. "Optimization algorithms as training approach with hybrid deep learning methods to develop an ultraviolet index forecasting model." Stochastic Environmental Research and Risk Assessment. 36 (10), pp. 3011-3039. https://doi.org/10.1007/s00477-022-02177-3Surface water sodium (Na+) concentration prediction using hybrid weighted exponential regression model with gradient-based optimization
Ahmadianfar, Iman, Shirvani-Hosseini, Seyedehelham, Samadi-Koucheksaraee, Arvin and Yaseen, Zaher Mundher. 2022. "Surface water sodium (Na+) concentration prediction using hybrid weighted exponential regression model with gradient-based optimization." Environmental Science and Pollution Research. 29 (35), pp. 53456-53481. https://doi.org/10.1007/s11356-022-19300-0An improved adaptive neuro fuzzy inference system model using conjoined metaheuristic algorithms for electrical conductivity prediction
Ahmadianfar, Iman, Shirvani-Hosseini, Seyedehelham, He, Jianxun He, Samadi-Koucheksaraee, Arvin and Yaseen, Zaher Mundher. 2022. "An improved adaptive neuro fuzzy inference system model using conjoined metaheuristic algorithms for electrical conductivity prediction." Scientific Reports. 12 (1). https://doi.org/10.1038/s41598-022-08875-wThermal and Hydraulic Performances of Carbon and Metallic Oxides-Based Nanomaterials
Afan, Haitham Abdulmohsin, Aldlemy, Mohammed Suleman, Ahmed, Ali M., Jawad, Ali H., Naser, Maryam H., Homod, Raad Z., Mussa, Zainab Haider, Abdulkadhim, Adnan Hashim, Scholz, Miklas and Yaseen, Zaher Mundher. 2022. "Thermal and Hydraulic Performances of Carbon and Metallic Oxides-Based Nanomaterials." Nanomaterials. 12 (9). https://doi.org/10.3390/nano12091545Different TiO2 Phases (Degussa/Anatase) Modified Cross-Linked Chitosan Composite for the Removal of Reactive Red 4 Dye: Box–Behnken Design
Abdulhameed, Ahmed Saud, Jawad, Ali H., Vigneshwaran, Sivakumar, ALOthman, Zeid A. and Yaseen, Zaher Mundher Yaseen. 2022. "Different TiO2 Phases (Degussa/Anatase) Modified Cross-Linked Chitosan Composite for the Removal of Reactive Red 4 Dye: Box–Behnken Design." Journal of Polymers and the Environment. 30 (12), pp. 5084-5099. https://doi.org/10.1007/s10924-022-02568-1Chitosan/Carbon-Doped TiO2 Composite for Adsorption of Two Anionic Dyes in Solution and Gaseous SO2 Capture: Experimental Modeling and Optimization
Abdulhameed, Ahmed Saud, Jawad, Ali H., Ridwan, Muhammad, Khadiran, Tumirah, Wilson, Lee D. and Yaseen, Zaher Mundher. 2022. "Chitosan/Carbon-Doped TiO2 Composite for Adsorption of Two Anionic Dyes in Solution and Gaseous SO2 Capture: Experimental Modeling and Optimization." Journal of Polymers and the Environment. 30 (11), pp. 4619-4636. https://doi.org/10.1007/s10924-022-02532-zFusion of B-mode and shear wave elastography ultrasound features for automated detection of axillary lymph node metastasis in breast carcinoma
Pham, The-Hanh, Faust, Oliver, Koh, Joel En Wei, Ciaccio, Edward J., Barua, Prabal D., Omar, Norlia, Ng, Wei Lin, Mumin, Nazimah Ab, Rahmat, Kartini and Acharya, U. Rajendra. 2022. "Fusion of B-mode and shear wave elastography ultrasound features for automated detection of axillary lymph node metastasis in breast carcinoma." Expert Systems: the journal of knowledge engineering. 39 (5), pp. 1-19. https://doi.org/10.1111/exsy.12947An Accurate Multiple Sclerosis Detection Model Based on Exemplar Multiple Parameters Local Phase Quantization: ExMPLPQ
Macin, Gulay, Tasci, Burak, Tasci, Irem, Faust, Oliver, Barua, Prabal Datta, Dogan, Sengul, Tuncer, Turker, Tan, Ru-San and Acharya, U. Rajendra. 2022. "An Accurate Multiple Sclerosis Detection Model Based on Exemplar Multiple Parameters Local Phase Quantization: ExMPLPQ." Applied Sciences. 12 (10). https://doi.org/10.3390/app12104920TMP19: A Novel Ternary Motif Pattern-Based ADHD Detection Model Using EEG Signals
Barua, Prabal Datta, Dogan, Sengul, Baygin, Mehmet, Tuncer, Turker, Palmer, Elizabeth Emma, Ciaccio, Edward J. and Acharya, U. Rajendra. 2022. "TMP19: A Novel Ternary Motif Pattern-Based ADHD Detection Model Using EEG Signals." Diagnostics. 12 (10). https://doi.org/10.3390/diagnostics12102544Multi-step daily forecasting of reference evapotranspiration for different climates of India: A modern multivariate complementary technique reinforced with ridge regression feature selection
Malik, Anurag, Jamei, Mehdi, Ali, Mumtaz, Prasad, Ramendra, Karbasi, Masoud and Yaseen, Zaher Mundher. 2022. "Multi-step daily forecasting of reference evapotranspiration for different climates of India: A modern multivariate complementary technique reinforced with ridge regression feature selection." Agricultural Water Management. 272. https://doi.org/https://doi.org/10.1016/j.agwat.2022.107812A hierarchical classification/regression algorithm for improving extreme wind speed events prediction
Pelaez-Rodriguez, C., Perez-Aracil, J., Fister, D, Prieto-Godino, L., Deo, R.C. and Salcedo-sanz, S.. 2022. "A hierarchical classification/regression algorithm for improving extreme wind speed events prediction." Renewable Energy. 201 (Part 2), pp. 157-178. https://doi.org/10.1016/j.renene.2022.11.042Designing a Multi-Stage Expert System for daily ocean wave energy forecasting: A multivariate data decomposition-based approach
Jamei, Mehdi, Ali, Mumtaz, Karbasi, Masoud, Xiang, Yong, Ahmadianfar, Iman and Yaseen, Zaher Mundher. 2022. "Designing a Multi-Stage Expert System for daily ocean wave energy forecasting: A multivariate data decomposition-based approach ." Applied Energy. 326, pp. 1-24. https://doi.org/10.1016/j.apenergy.2022.119925Forecasting weekly reference evapotranspiration using Auto Encoder Decoder Bidirectional LSTM model hybridized with a Boruta-CatBoost input optimizer
Karbasi, Masoud, Jamei, Mehdi, Ali, Mumtaz, Malik, Anurag and Yaseen, Zaher Mundher. 2022. "Forecasting weekly reference evapotranspiration using Auto Encoder Decoder Bidirectional LSTM model hybridized with a Boruta-CatBoost input optimizer." Computers and Electronics in Agriculture. 198, pp. 1-17. https://doi.org/10.1016/j.compag.2022.107121Development of Deep Learning Hybrid Models for Hydrological Predictions
Ahmed, Abul Abrar Masrur. 2022. Development of Deep Learning Hybrid Models for Hydrological Predictions. PhD by Publication Doctor of Philosophy. University of Southern Queensland. https://doi.org/10.26192/q7q5zA set of quality metrics for the evaluation of voice termination services
Chan, Ka C., Zhou, Xujuan, Gururajan, Raj and Barua, Prabal. 2018. "A set of quality metrics for the evaluation of voice termination services." 5th International Conference on Behavioral, Economic, and Socio-Cultural Computing (BESC 2018). Kaohsiung, Taiwan 12 - 14 Nov 2018 Los Alamitos, CA, United States. https://doi.org/10.1109/BESC.2018.00036Wearable ultraviolet radiation sensors for research and personal use
Henning, Alyssa, Downs, Nathan J. and Vanos, Jennifer K.. 2022. "Wearable ultraviolet radiation sensors for research and personal use." International Journal of Biometeorology: the description, causes, and implications of climatic change. 66 (3), pp. 627-640. https://doi.org/10.1007/s00484-021-02216-8Wind speed forecasting in Nepal using self-organizing map-based online sequential extreme learning machine
Sharma, Neelesh and Deo, Ravinesh. 2021. "Wind speed forecasting in Nepal using self-organizing map-based online sequential extreme learning machine." Deo, Ravinesh, Samui, Pijush and Roy, Sanjiban Sekhar (ed.) Predictive Modelling for Energy Management and Power Systems Engineering. Netherlands. Elsevier. pp. 437-484Support vector machine model for multistep wind speed forecasting
Prasad, Shobna Mohini Mala, Nguyen-Huy, Thong and Deo, Ravinesh. 2021. "Support vector machine model for multistep wind speed forecasting." Deo, Ravinesh, Samui, Pijush and Roy, Sanjiban Sekhar (ed.) Predictive modelling for energy management and power systems engineering. Netherlands. Elsevier. pp. 335-389Development of data-driven models for wind speed forecasting in Australia
Neupane, Ananta, Raj, Nawin, Deo, Ravinesh and Ali, Mumtaz. 2021. "Development of data-driven models for wind speed forecasting in Australia." Deo, R., Samui, Pijush and Roy, Sanjiban Sekhar (ed.) Predictive modelling for energy management and power systems engineering. Netherlands. Elsevier. pp. 143-190Sun exposure and physical activity: the valuable role of UV wearables
Henning, Alyssa, Vanos, Jennifer and Downs, Nathan. 2021. "Sun exposure and physical activity: the valuable role of UV wearables." IEEE Technology and Society Magazine. 40 (3), pp. 16-18. https://doi.org/10.1109/MTS.2021.3101927Combatting Procrastination
Devi, Aruna. 2021. "Combatting Procrastination." Bartlett, Cristy, Cawthray, Tyler, Clark, Linda, Conway, Sam, Derrington, Kate, Devi, Aruna, Frederiks, Anita, Gunton, Lyndelle, Hargreaves, Wendy, Howarth, Debi, Irvine, Sarah, Jeffers, Marjorie, Lovric, Kristen, McGregor, Rowena, Peters, Emma, Pickstone, Leigh, Retallick, Bianca, Rose, Yvonne, Sahay, Akshay, Schultz, Susanne, Sachdeva, Karanpal Singh, Thangavelu, Anbarasu and Tweedale, Robyn (ed.) Academic Success. Toowoomba, Australia. University of Southern Queensland.Application of deep learning models for automated identification of Parkinson’s disease: a review (2011–2021)
Loh, Hui Wen, Hong, Wanrong, Ooi, Chui Ping, Chakraborty, Subrata, Barua, Prabal Datta, Deo, Ravinesh C., Soar, Jeffrey, Palmer, Elizabeth E. and Acharya, U. Rajendra. 2021. "Application of deep learning models for automated identification of Parkinson’s disease: a review (2011–2021)." Sensors. 21, pp. 1-27. https://doi.org/10.3390/s21217034Solar ultraviolet radiation, shade profile and heat index survey report: New South Wales playgrounds (2020 - 2021)
Downs, Nathan J.. 2021. Solar ultraviolet radiation, shade profile and heat index survey report: New South Wales playgrounds (2020 - 2021). Unpublished.Artificial intelligence models for suspended river sediment prediction: state-of-the art, modeling framework appraisal, and proposed future research directions
Tao, Hai, Al-Khafaji, Zainab S., Qi, Chongchong, Zounemat-Kermani, Mohammad, Kisi, Ozgur, Tiyasha, Tiyasha, Chau, Kwok-Wing, Nourani, Vahid, Melesse, Assefa M., Elhakeem, Mohamed, Farooque, Aitazaz Ahsan, Nejadhashemi, A. Pouyan, Khedher, Khaled Mohamed, Alawi, Omer A., Deo, Ravinesh C., Shahid, Shamsuddin, Singh, Vijay P. and Yaseen, Zaher Mundher. 2021. "Artificial intelligence models for suspended river sediment prediction: state-of-the art, modeling framework appraisal, and proposed future research directions." Engineering Applications of Computational Fluid Mechanics. 15 (1), pp. 1585-1612. https://doi.org/10.1080/19942060.2021.1984992Advanced extreme learning machines vs. deep learning models for peak wave energy period forecasting: A case study in Queensland, Australia
Ali, Mumtaz, Prasad, Ramendra, Xiang, Yong, Sankaran, Adarsh, Deo, Ravinesh C., Xiao, Fuyuan and Zhu, Shuyu. 2021. "Advanced extreme learning machines vs. deep learning models for peak wave energy period forecasting: A case study in Queensland, Australia." Renewable Energy. 177, pp. 1033-1044. https://doi.org/10.1016/j.renene.2021.06.052Novel short-term solar radiation hybrid model: long short-term memory network integrated with robust local mean decomposition
Huynh, Anh Ngoc-Lan, Deo, Ravinesh C., Ali, Mumtaz, Abdulla, Shahab and Raj, Nawin. 2021. "Novel short-term solar radiation hybrid model: long short-term memory network integrated with robust local mean decomposition." Applied Energy. 298, pp. 1-19. https://doi.org/10.1016/j.apenergy.2021.117193Evaluating management strategies for sustainable crop production under changing climate conditions: a system dynamics approach
Pham, Yen Hoang, Reardon-Smith, Kathryn and Deo, Ravinesh C.. 2021. "Evaluating management strategies for sustainable crop production under changing climate conditions: a system dynamics approach." Journal of Environmental Management. 292. https://doi.org/10.1016/j.jenvman.2021.112790An interplay of soil salinization and groundwater degradation threatening coexistence of oasis-desert ecosystems
Yin, Xinwei, Feng, Qi, Li, Yan, Deo, Ravinesh C., Liu, Wei, Zhu, Meng, Zheng, Xinjun and Liu, Ran. 2022. "An interplay of soil salinization and groundwater degradation threatening coexistence of oasis-desert ecosystems." Science of the Total Environment. 806 (2), pp. 1-20. https://doi.org/10.1016/j.scitotenv.2021.150599Hybrid deep learning method for a week-ahead evapotranspiration forecasting
Ahmed, A. A. Masrur, Deo, Ravinesh C., Feng, Qi, Ghahramani, Afshin, Raj, Nawin, Yin, Zhenliang and Yang, Linshan. 2022. "Hybrid deep learning method for a week-ahead evapotranspiration forecasting." Stochastic Environmental Research and Risk Assessment. 36 (3), pp. 831-849. https://doi.org/10.1007/s00477-021-02078-xStreamflow prediction using an integrated methodology based on convolutional neural network and long short‑term memory networks
Ghimire, Sujan, Yaseen, Zaher Mundher, Farooque, Aitazaz A., Deo, Ravinesh C., Zhang, Ji and Tao, Xiaohui. 2021. "Streamflow prediction using an integrated methodology based on convolutional neural network and long short‑term memory networks." Scientific Reports. 11, pp. 1-26. https://doi.org/10.1038/s41598-021-96751-4The role of internal transcribed spacer 2 secondary structures in classifying mycoparasitic Ampelomyces
Prahl, Rosa E., Khan, Shahjahan and Deo, Ravinesh C.. 2021. "The role of internal transcribed spacer 2 secondary structures in classifying mycoparasitic Ampelomyces." PLoS One. 16 (6), pp. 1-28. https://doi.org/10.1371/journal.pone.0253772Evaluation of the Long-term Cumulative UVA Facial Exposure of Queensland School Teachers derived for an Extended Period from the OMI Satellite Irradiance
A Jebar, Mustapha A., Downs, Nathan J., Parisi, Alfio V. and Turner, Joanna. 2021. "Evaluation of the Long-term Cumulative UVA Facial Exposure of Queensland School Teachers derived for an Extended Period from the OMI Satellite Irradiance." Photochemistry and Photobiology. 97 (1), pp. 192-197. https://doi.org/10.1111/php.13329Deep Learning Forecasts of Soil Moisture: Convolutional Neural Network and Gated Recurrent Unit Models Coupled with Satellite-Derived MODIS, Observations and Synoptic-Scale Climate Index Data
Ahmed, A. A. Masrur, Deo, Ravinesh C., Raj, Nawin, Ghahramani, Afshin, Feng, Qi, Yin, Zhenliang and Yang, Linshan. 2021. "Deep Learning Forecasts of Soil Moisture: Convolutional Neural Network and Gated Recurrent Unit Models Coupled with Satellite-Derived MODIS, Observations and Synoptic-Scale Climate Index Data." Remote Sensing. 13 (4), pp. 1-30. https://doi.org/10.3390/rs13040554LSTM integrated with Boruta-random forest optimiser for soil moisture estimation under RCP4.5 and RCP8.5 global warming scenarios
Ahmed, A. A. Masrur, Deo, Ravinesh C., Ghahramani, Afshin, Raj, Nawin, Feng, Qi, Yin, Zhenliang and Yang, Linshan. 2021. "LSTM integrated with Boruta-random forest optimiser for soil moisture estimation under RCP4.5 and RCP8.5 global warming scenarios." Stochastic Environmental Research and Risk Assessment. 35, pp. 1851-1881. https://doi.org/10.1007/s00477-021-01969-3A new framework for classification of multi-category hand grasps using EMG signals
Miften, Firas Sabar, Diykh, Mohammed, Abdulla, Shahab, Siuly, Siuly, Green, Jonathan H. and Deo, Ravinesh C.. 2021. "A new framework for classification of multi-category hand grasps using EMG signals." Artificial Intelligence in Medicine. 112, pp. 1-14. https://doi.org/10.1016/j.artmed.2020.102005Deep learning hybrid model with Boruta-Random forest optimiser algorithm for streamflow forecasting with climate mode indices, rainfall, and periodicity
Ahmed, A. A. Masrur, Deo, Ravinesh C., Feng, Qi, Ghahramani, Afshin, Raj, Nawin, Yin, Zhenliang and Yang, Linshan. 2021. "Deep learning hybrid model with Boruta-Random forest optimiser algorithm for streamflow forecasting with climate mode indices, rainfall, and periodicity." Journal of Hydrology. 599, pp. 1-23. https://doi.org/10.1016/j.jhydrol.2021.126350Modeling soil temperature using air temperature features in diverse climatic conditions with complementary machine learning models
Bayatvarkeshi, Maryam, Bhagat, Suraj Kumar, Mohammadi, Kourosh, Kisi, Ozgur, Farahani, M., Hasani, A., Deo, Ravinesh and Yaseen, Zaher Mundher. 2021. "Modeling soil temperature using air temperature features in diverse climatic conditions with complementary machine learning models." Computers and Electronics in Agriculture. 185. https://doi.org/10.1016/j.compag.2021.106158SEQ Ultraviolet and Cloud Measurement Data Collection
Downs, Nathan. SEQ Ultraviolet and Cloud Measurement Data Collection. Toowoomba. https://doi.org/10.26192/9209-3504Electronic Sun Journal Versus Self-report Sun Diary: A Comparison of Recording Personal Sunlight Exposure Methods
Dexter, Benjamin R., King, Rachel, Parisi, Alfio V., Harrison, Simone L., Igoe, Damien P. and Downs, Nathan J.. 2021. "Electronic Sun Journal Versus Self-report Sun Diary: A Comparison of Recording Personal Sunlight Exposure Methods." Photochemistry and Photobiology. 97 (3), pp. 641-649. https://doi.org/10.1111/php.13359Mapping rice area and yield in northeastern asia by incorporating a crop model with dense vegetation index profiles from a geostationary satellite
Yeom, Jong-Min, Jeong, Seungtaek, Deo, Ravinesh C. and Ko, Jonghan. 2021. "Mapping rice area and yield in northeastern asia by incorporating a crop model with dense vegetation index profiles from a geostationary satellite." GIScience and Remote Sensing. https://doi.org/10.1080/15481603.2020.1853352Deep Air Quality Forecasts: Suspended Particulate Matter Modeling With Convolutional Neural and Long Short-Term Memory Networks
Sharma, Ekta, Deo, Ravinesh C., Prasad, Ramendra, Parisi, Alfio and Raj, Nawin. 2020. "Deep Air Quality Forecasts: Suspended Particulate Matter Modeling With Convolutional Neural and Long Short-Term Memory Networks." IEEE Access. 8, pp. 209503-209516. https://doi.org/10.1109/ACCESS.2020.3039002A New Deep Convolutional Neural Network Model for Automated Breast Cancer Detection
Zhou, Xujuan, Li, Yuefeng, Gururajan, Raj, Bargshady, Ghazal, Tao, Xiaohui, Venkataraman, Revathi, Barua, Prabal D. and Kondalsamy-Chennakesavan, Srinivas. 2020. "A New Deep Convolutional Neural Network Model for Automated Breast Cancer Detection." 7th International Conference on Behavioural and Social Computing (BESC 2020). Bournemouth, United Kingdom 05 - 07 Nov 2020 United Kingdom. https://doi.org/10.1109/BESC51023.2020.9348322Multi-scale convolutional neural network for accurate corneal segmentation in early detection of fungal keratitis
Mayya, Veena, Kamath S, Sowmya, Kulkarni, Uma, Hazarika, Manali, Barua, Prabal Datta and Acharya, U. Rajendra. 2021. "Multi-scale convolutional neural network for accurate corneal segmentation in early detection of fungal keratitis." Journal of Fungi. 7 (10). https://doi.org/10.3390/jof7100850Gaborpdnet: Gabor transformation and deep neural network for parkinson’s disease detection using eeg signals
Loh, Hui Wen, Ooi, Chui Ping, Palmer, Elizabeth, Barua, Prabal Datta, Dogan, Sengul, Tuncer, Turker, Baygin, Mehmet and Acharya, U. Rajendra. 2021. "Gaborpdnet: Gabor transformation and deep neural network for parkinson’s disease detection using eeg signals." Electronics. 10 (14). https://doi.org/10.3390/electronics10141740Automated covid-19 and heart failure detection using dna pattern technique with cough sounds
Kobat, Mehmet Ali, Kivrak, Tarik, Barua, Prabal Datta, Tuncer, Turker, Dogan, Sengul, Tan, Ru-San, Ciaccio, Edward J. and Acharya, U. Rajendra. 2021. "Automated covid-19 and heart failure detection using dna pattern technique with cough sounds." Diagnostics. 11 (11). https://doi.org/10.3390/diagnostics11111962A review on computer aided diagnosis of acute brain stroke
Inamdar, Mahesh Anil, Raghavendra, Udupi, Gudigar, Anjan, Chakole, Yashas, Hegde, Ajay, Menon, Girish R., Barua, Prabal, Palmer, Elizabeth Emma, Cheong, Kang Hao, Chan, Wai Yee, Ciaccio, Edward J. and Acharya, U. Rajendra. 2021. "A review on computer aided diagnosis of acute brain stroke." Sensors. 21 (24). https://doi.org/10.3390/s21248507Recent trends in artificial intelligence-assisted coronary atherosclerotic plaque characterization
Gudigar, Anjan, Nayak, Sneha, Samanth, Jyothi, Raghavendra, U., Ashwal, A J, Barua, Prabal Datta, Hasan, Md Nazmul, Ciaccio, Edward J., Tan, Ru-San and Acharya, U. Rajendra. 2021. "Recent trends in artificial intelligence-assisted coronary atherosclerotic plaque characterization." International Journal of Environmental Research and Public Health. 18 (19). https://doi.org/10.3390/ijerph181910003PrimePatNet87: Prime pattern and tunable q-factor wavelet transform techniques for automated accurate EEG emotion recognition
Dogan, Abdullah, Akay, Merve, Barua, Prabal Datta, Baygin, Mehmet, Dogan, Sengul, Tuncer, Turker, Dogru, Ali Hikmet and Acharya, U. Rajendra. 2021. "PrimePatNet87: Prime pattern and tunable q-factor wavelet transform techniques for automated accurate EEG emotion recognition." Computers in Biology and Medicine. 138. https://doi.org/10.1016/j.compbiomed.2021.104867Automated accurate schizophrenia detection system using Collatz pattern technique with EEG signals
Baygin, Mehmet, Yaman, Orhan, Tuncer, Turker, Dogan, Sengul, Barua, Prabal Datta and Acharya, U. Rajendra. 2021. "Automated accurate schizophrenia detection system using Collatz pattern technique with EEG signals." Biomedical Signal Processing and Control. 70. https://doi.org/10.1016/j.bspc.2021.102936Automated ASD detection using hybrid deep lightweight features extracted from EEG signals
Baygin, Mehmet, Dogan, Sengul, Tuncer, Turker, Barua, Prabal Datta, Faust, Oliver, Arunkumar, N., Abdulhay, Enas W., Palmer, Elizabeth Emma and Acharya, U. Rajendra. 2021. "Automated ASD detection using hybrid deep lightweight features extracted from EEG signals." Computers in Biology and Medicine. 134. https://doi.org/10.1016/j.compbiomed.2021.104548Automatic covid-19 detection using exemplar hybrid deep features with x-ray images
Barua, Prabal Datta, Muhammad Gowdh, Nadia Fareeda, Rahmat, Kartini, Ramli, Norlisah, Ng, Wei Lin, Chan, Wai Yee, Kuluozturk, Mutlu, Dogan, Sengul, Baygin, Mehmet, Yaman, Orhan, Tuncer, Turker, Wen, Tao, Cheong, Kang Hao and Acharya, U. Rajendra. 2021. "Automatic covid-19 detection using exemplar hybrid deep features with x-ray images." International Journal of Environmental Research and Public Health. 18 (15). https://doi.org/10.3390/ijerph18158052Novel automated PD detection system using aspirin pattern with EEG signals
Barua, Prabal Datta, Dogan, Sengul, Tuncer, Turker, Baygin, Mehmet and Acharya, Rajendra. 2021. "Novel automated PD detection system using aspirin pattern with EEG signals." Computers in Biology and Medicine. 137. https://doi.org/10.1016/j.compbiomed.2021.104841Multilevel deep feature generation framework for automated detection of retinal abnormalities using OCT images
Barua, Prabal Datta, Chan, Wai Yee Chan, Dogan, Sengul, Baygin, Mehmet, Tuncer, Turker, Ciaccio, Edward J., Islam, Nazrul, Cheong, Kang Hao, Shahid, Zakia Sultana and Acharya, Rajendra. 2021. "Multilevel deep feature generation framework for automated detection of retinal abnormalities using OCT images." Entropy: international and interdisciplinary journal of entropy and information studies. 23 (12). https://doi.org/10.3390/e23121651Variational mode decomposition based random forest model for solar radiation forecasting: New emerging machine learning technology
Ali, Mumtaz, Prasad, Ramendra, Xiang, Yong, Khan, Mohsin, Farooque, Aitazaz Ahsan, Zong, Tianrui and Yaseen, Zaher Mundher. 2021. "Variational mode decomposition based random forest model for solar radiation forecasting: New emerging machine learning technology." Energy Reports. 7, pp. 6700-6717. https://doi.org/10.1016/j.egyr.2021.09.113Forecasting standardized precipitation index using data intelligence models: regional investigation of Bangladesh
Yaseen, Zaher Mundher, Ali, Mumtaz, Sharafati, Ahmad, Al-Ansari, Nadhir and Shahid, Shamsuddin. 2021. "Forecasting standardized precipitation index using data intelligence models: regional investigation of Bangladesh." Scientific Reports. 11 (1), pp. 1-25. https://doi.org/10.1038/s41598-021-82977-9MARS model for prediction of short- and long-term global solar radiation
Balalla, Dilki T., Nguyen-Huy, Thong and Deo, Ravinesh. 2021. "MARS model for prediction of short- and long-term global solar radiation." Deo, Ravinesh, Roy, Sanjiban Sekhar and Samui, Pijush (ed.) Predictive Modelling for Energy Management and Power Systems Engineering. United Kingdom. Elsevier. pp. 391-436Developing reservoir evaporation predictive model for successful dam management
Allawi, Mohammed Falah, Ahmed, Mohammed Lateef, Aidan, Ibraheem Abdallah, Deo, Ravinesh C. and El-Shafie, Ahmed. 2021. "Developing reservoir evaporation predictive model for successful dam management." Stochastic Environmental Research and Risk Assessment. 35 (2), pp. 499-514. https://doi.org/10.1007/s00477-020-01918-6Application of effective drought index for quantification of meteorological drought events: a case study in Australia
Deo, Ravinesh C., Byun, Hi-Ryong, Adamowski, Jan F. and Begum, Khaleda. 2017. "Application of effective drought index for quantification of meteorological drought events: a case study in Australia." Theoretical and Applied Climatology. 128 (1-2), pp. 359-379. https://doi.org/10.1007/s00704-015-1706-5Feedback modelling of the impacts of drought: A case study in coffee production systems in Viet Nam
Pham, Yen, Reardon-Smith, Kathryn, Mushtaq, Shahbaz and Deo, Ravinesh C.. 2020. "Feedback modelling of the impacts of drought: A case study in coffee production systems in Viet Nam." Climate Risk Management. 30, pp. 1-17. https://doi.org/10.1016/j.crm.2020.100255Forecasting long-term precipitation for water resource management: a new multi-step data-intelligent modelling approach
Ali, Mumtaz, Deo, Ravinesh C., Xiang, Yong, Li, Ya and Yaseen, Zaher Mundher. 2020. "Forecasting long-term precipitation for water resource management: a new multi-step data-intelligent modelling approach." Hydrological Sciences Journal. 65 (16), pp. 2693-2708. https://doi.org/10.1080/02626667.2020.1808219Keratinocyte skin cancer risks for working school teachers: scenarios and implications of the timing of scheduled duty periods in Queensland, Australia
Dexter, B. R., King, R., Parisi, A. V., Harrison, S. L., Konovalov, D. A. and Downs, N. J.. 2020. "Keratinocyte skin cancer risks for working school teachers: scenarios and implications of the timing of scheduled duty periods in Queensland, Australia." Journal of Photochemistry and Photobiology, B: Biology. 213, pp. 1-10. https://doi.org/10.1016/j.jphotobiol.2020.112046Development of Flood Monitoring Index for daily flood risk evaluation: case studies in Fiji
Moishin, Mohammed, Deo, Ravinesh C., Prasad, Ramendra, Raj, Nawin and Abdulla, Shahab. 2021. "Development of Flood Monitoring Index for daily flood risk evaluation: case studies in Fiji." Stochastic Environmental Research and Risk Assessment. 35 (7), pp. 1387-1402. https://doi.org/10.1007/s00477-020-01899-6The modeling of human facial pain intensity based on Temporal Convolutional Networks trained with video frames in HSV color space
Bargshady, Ghazal, Zhou, Xujuan, Deo, Ravinesh C., Soar, Jeffrey, Whittaker, Frank and Wang, Hua. 2020. "The modeling of human facial pain intensity based on Temporal Convolutional Networks trained with video frames in HSV color space." Applied Soft Computing. 97 (Part A), pp. 1-14. https://doi.org/10.1016/j.asoc.2020.106805Predictive modelling of global solar radiation with artificial intelligence approaches using MODIS satellites and atmospheric reanalysis data for Australia
Ghimire, Sujan. 2019. Predictive modelling of global solar radiation with artificial intelligence approaches using MODIS satellites and atmospheric reanalysis data for Australia. PhD Thesis Doctor of Philosophy. University of Southern Queensland. https://doi.org/10.26192/9he0-h328Modeling wheat yield with data-intelligent algorithms: artificial neural network versus genetic programming and minimax probability machine regression
Ali, Mumtaz and Deo, Ravinesh C.. 2020. "Modeling wheat yield with data-intelligent algorithms: artificial neural network versus genetic programming and minimax probability machine regression." Samui, Pijush, Bui, Dieu Tien, Chakraborty, Subrata and Deo, Ravinesh C. (ed.) Handbook of probabilistic models. Oxford, United Kingdom. Elsevier. pp. 37-87MARS model for prediction of short- and long-term global solar radiation
Balalla, Dilki T., Nguyen-Huy, Thong and Deo, Ravinesh. 2021. "MARS model for prediction of short- and long-term global solar radiation." Deo, Ravinesh, Samui, Pijush and Roy, Sanjiban Sekhar (ed.) Predictive modelling for energy management and power systems engineering. Amsterdam, Netherlands. Elsevier. pp. 391-436Short-term electrical energy demand prediction under heat island effects using emotional neural network integrated with genetic algorithm
Karalasingham, Sagthitharan, Deo, Ravinesh and Prasad, Ramendra. 2021. "Short-term electrical energy demand prediction under heat island effects using emotional neural network integrated with genetic algorithm." Deo, Ravinesh, Samui, Pijush and Roy, Sanjiban Sekhar (ed.) Predictive modelling for energy management and power systems engineering. Amsterdam, Netherlands. Elsevier. pp. 271-298Hybrid multilayer perceptron-firefly optimizer algorithm for modelling photosynthetic active solar radiation for biofuel energy exploration
Goundar, Harshna, Yaseen, Zaher Mundher and Deo, Ravinesh. 2021. "Hybrid multilayer perceptron-firefly optimizer algorithm for modelling photosynthetic active solar radiation for biofuel energy exploration." Deo, Ravinesh, Samui, Pijush and Roy, Sanjiban Sekhar (ed.) Predictive modelling for energy management and power systems engineering. Amsterdam, Netherlands. Elsevier. pp. 191-232Design and performance of two decomposition paradigms in forecasting daily solar radiation with evolutionary polynomial regression: wavelet transform versus ensemble empirical mode decomposition
Rezaie-Balf, Mohammad, Kim, Sungwon, Ghaemi, Alireza and Deo, Ravinesh. 2021. "Design and performance of two decomposition paradigms in forecasting daily solar radiation with evolutionary polynomial regression: wavelet transform versus ensemble empirical mode decomposition." Deo, Ravinesh, Samui, Pijush and Roy, Sanjiban Sekhar (ed.) Predictive modelling for energy management and power systems engineering. Amsterdam, Netherlands. Elsevier. pp. 115-142Shade auditing in Queensland
Downs, Nathan, Parisi, Alfio, Baldwin, Louise and Harrison, Simone. 2019. "Shade auditing in Queensland." 6th Annual Brisbane Cancer Conference. Brisbane, Australia 28 - 29 Nov 2019Experimental Study on the Rainfall-Runoff Responses of Typical Urban Surfaces and Two Green Infrastructures Using Scale-Based Models
Liu, Wen, Feng, Qi, Deo, Ravinesh C., Yao, Lei and Wei, Wei. 2020. "Experimental Study on the Rainfall-Runoff Responses of Typical Urban Surfaces and Two Green Infrastructures Using Scale-Based Models ." Environmental Management (New York): an international journal for decision-makers, scientists and environmental auditors. 66 (4), pp. 683-693. https://doi.org/10.1007/s00267-020-01339-9Ensemble neural network approach detecting pain intensity from facial expressions
Bargshady, Ghazal, Zhou, Xujuan, Deo, Ravinesh C., Soar, Jeffrey, Whittaker, Frank and Wang, Hua. 2020. "Ensemble neural network approach detecting pain intensity from facial expressions." Artificial Intelligence in Medicine. 109, pp. 1-12. https://doi.org/10.1016/j.artmed.2020.101954A survey on text classification and its applications
Zhou, Xujuan, Gururajan, Raj, Li, Yuefeng, Venkataraman, Revathi, Tao, Xiaohui, Bargshady, Ghazala, Barua, Prabal D. and Kondalsamy-Chennakesavan, Srinivas. 2020. "A survey on text classification and its applications." Web Intelligence. 18 (2), pp. 205-216. https://doi.org/10.3233/WEB-200442Determination of factors influencing student engagement using a learning management system in a tertiary setting
Barua, Prabal Datta. 2020. Determination of factors influencing student engagement using a learning management system in a tertiary setting. PhD Thesis Doctor of Philosophy. University of Southern Queensland. https://doi.org/10.26192/Z9AS-4P42Daily flood forecasts with intelligent data analytic models: multivariate empirical mode decomposition-based modeling methods
Prasad, Ramendra, Charan, Dhrishna, Joseph, Lionel, Nguyen-Huy, Thong, Deo, Ravinesh C. and Singh, Sanjay. 2021. "Daily flood forecasts with intelligent data analytic models: multivariate empirical mode decomposition-based modeling methods." Deo, Ravinesh C., Samui, Pijush, Kisi, Ozgur and Yaseen, Zaher Mundher (ed.) Intelligent data analytics for decision-support systems in hazard mitigation: theory and practice of hazard mitigation. Singapore. Springer. pp. 359-381Artificial neural networks for prediction of Steadman Heat Index
Chand, Bhuwan, Nguyen-Huy, Thong and Deo, Ravinesh C.. 2021. "Artificial neural networks for prediction of Steadman Heat Index." Deo, Ravinesh C., Samui, Pijush, Kisi, Ozgur and Yaseen, Zaher Mundher (ed.) Intelligent data analytics for decision-support systems in hazard mitigation: theory and practice of hazard mitigation. Singapore. Springer. pp. 293-357Spatial prediction of landslide susceptibility using random forest algorithm
Rahmati, Omid, Kornejady, Aiding and Deo, Ravinesh C.. 2021. "Spatial prediction of landslide susceptibility using random forest algorithm." Deo, Ravinesh C., Samui, Pijush, Kisi, Ozgur and Yaseen, Zaher Mundher (ed.) Intelligent data analytics for decision-support systems in hazard mitigation: theory and practice of hazard mitigation. Singapore. Springer. pp. 281-292Intelligent data analytics for time series, trend analysis and drought indices comparison
Dayal, Kavina S., Deo, Ravinesh C. and Apan, Armando A.. 2021. "Intelligent data analytics for time series, trend analysis and drought indices comparison." Deo, Ravinesh C., Samui, Pijush, Kisi, Ozgur and Yaseen, Zaher Mundher (ed.) Intelligent data analytics for decision-support systems in hazard mitigation: theory and practice of hazard mitigation. Singapore. Springer. pp. 151-169Modulation of tropical cyclone genesis by Madden–Julian Oscillation in the Southern Hemisphere
Dayal, Kavina S., Wang, Bin and Deo, Ravinesh C.. 2021. "Modulation of tropical cyclone genesis by Madden–Julian Oscillation in the Southern Hemisphere." Deo, Ravinesh C., Samui, Pijush, Kisi, Ozgur and Yaseen, Zaher Mundher (ed.) Intelligent data analytics for decision-support systems in hazard mitigation: theory and practice of hazard mitigation. Singapore. Springer. pp. 127-150Bayesian Markov Chain Monte Carlo-based copulas: factoring the role of large-scale climate indices in monthly flood prediction
Nguyen-Huy, Thong, Deo, Ravinesh C., Yaseen, Zaher Mundher, Mushtaq, Shahbaz and Prasad, Ramendra. 2021. "Bayesian Markov Chain Monte Carlo-based copulas: factoring the role of large-scale climate indices in monthly flood prediction." Deo, Ravinesh C., Samui, Pijush, Kisi, Ozgur and Yaseen, Zaher Mundher (ed.) Intelligent data analytics for decision-support systems in hazard mitigation: theory and practice of hazard mitigation. Singapore. Springer. pp. 29-47Intelligent data analytics for decision-support systems in hazard mitigation: theory and practice of hazard mitigation
Deo, Ravinesh C., Samui, Pijush, Kisi, Ozgur and Yaseen, Zaher Mundher. 2021. Intelligent data analytics for decision-support systems in hazard mitigation: theory and practice of hazard mitigation. Singapore. Springer.Solar blue light radiation enhancement during mid to low solar elevation periods under cloud affected skies
Parisi, Alfio V., Igoe, Damien P., Amar, Abdurazaq and Downs, Nathan. 2020. "Solar blue light radiation enhancement during mid to low solar elevation periods under cloud affected skies." Sensors. 20 (15), pp. 1-13. https://doi.org/10.3390/s20154105Electronic Sun Journals: integrating calibrated field radiometers and traditional dosimeters for monitoring personal sun exposure behavior
Downs, Nathan. 2018. "Electronic Sun Journals: integrating calibrated field radiometers and traditional dosimeters for monitoring personal sun exposure behavior." 2018 National Cancer Institute Exercise Science and Skin Cancer Prevention Research Meeting. Rockville, United States 26 - 27 Sep 2018Near real-time significant wave height forecasting with hybridized multiple linear regression algorithms
Ali, Mumtaz, Prasad, Ramendra, Xiang, Yong and Deo, Ravinesh C.. 2020. "Near real-time significant wave height forecasting with hybridized multiple linear regression algorithms." Renewable and Sustainable Energy Reviews. 132. https://doi.org/10.1016/j.rser.2020.110003Electrical Energy Demand Forecasting Model Development and Evaluation with Maximum Overlap Discrete Wavelet Transform-Online Sequential Extreme Learning Machines Algorithms
Al-Musaylh, Mohanad S., Deo, Ravinesh C. and Li, Yan. 2020. "Electrical Energy Demand Forecasting Model Development and Evaluation with Maximum Overlap Discrete Wavelet Transform-Online Sequential Extreme Learning Machines Algorithms." Energies. 13 (9). https://doi.org/10.3390/en13092307Near real-time global solar radiation forecasting at multiple time-step horizons using the long short-term memory network
Huynh, Anh Ngoc‐Lan, Deo, Ravinesh C., An-Vo, Duc-Anh, Ali, Mumtaz, Raj, Nawin and Abdulla, Shahab. 2020. "Near real-time global solar radiation forecasting at multiple time-step horizons using the long short-term memory network." Energies. 13 (14). https://doi.org/10.3390/en13143517Adaptive boost LS-SVM classification approach for time-series signal classification in epileptic seizure diagnosis applications
Al-Hadeethi, Hanan, Abdulla, Shahab, Diykh, Mohammed, Deo, Ravinesh C. and Green, Jonathan H.. 2020. "Adaptive boost LS-SVM classification approach for time-series signal classification in epileptic seizure diagnosis applications." Expert Systems with Applications. 161. https://doi.org/10.1016/j.eswa.2020.113676Random forest predictive model development with uncertainty analysis capability for the estimation of evapotranspiration in an arid oasis region
Wu, Min, Feng, Qi, Wen, Xiaohu, Deo, Ravinesh C., Yin, Zhenliang, Yang, Linshan and Sheng, Danrui. 2020. "Random forest predictive model development with uncertainty analysis capability for the estimation of evapotranspiration in an arid oasis region." Hydrology Research: an international journal. 51 (4), pp. 648-665. https://doi.org/10.2166/nh.2020.012Measured UV Exposures of Ironman, Sprint and Olympic-Distance Triathlon Competitors
Downs, Nathan J., Axelsen, Taryn, Parisi, Alfio V., Schouten, Peter W. and Dexter, Ben R.. 2020. "Measured UV Exposures of Ironman, Sprint and Olympic-Distance Triathlon Competitors." Atmosphere. 11 (5), pp. 1-15. https://doi.org/10.3390/atmos11050440Evaluation of shade profiles while walking in urban environments: A case study from inner suburban Sydney, Australia
Igoe, Damien P., Downs, Nathan J., Parisi, Alfio V. and Amar, Abdurazaq. 2020. "Evaluation of shade profiles while walking in urban environments: A case study from inner suburban Sydney, Australia." Building and Environment. 177, pp. 1-7. https://doi.org/10.1016/j.buildenv.2020.106873Stormwater runoff and pollution retention performances of permeable pavements and the effects of structural factors
Liu, Wen, Feng, Qi, Chen, Weiping and Deo, Ravinesh C.. 2020. "Stormwater runoff and pollution retention performances of permeable pavements and the effects of structural factors." Environmental Science and Pollution Research. 27 (24), pp. 30831-30843. https://doi.org/10.1007/s11356-020-09220-2Spatial mapping of short-term solar radiation prediction incorporating geostationary satellite images coupled with deep convolutional LSTM networks for South Korea
Yeom, Jong-Min, Deo, Ravinesh C., Adamowski, Jan F., Park, Seonyoung and Lee, Chang-Suk. 2020. "Spatial mapping of short-term solar radiation prediction incorporating geostationary satellite images coupled with deep convolutional LSTM networks for South Korea." Environmental Research Letters. 15 (9), pp. 1-10. https://doi.org/10.1088/1748-9326/ab9467Development of Advanced Computer Aid Model for Shear Strength of Concrete Slender Beam Prediction
Sharafati, Ahmad, Haghbin, Masoud, Aldlemy, Mohammed Suleman, Mussa, Mohamed H., Al Zand, Ahmed W., Ali, Mumtaz, Bhagat, Suraj Kumar, Al-Ansari, Nadhir and Yaseen, Zaher Mundher. 2020. "Development of Advanced Computer Aid Model for Shear Strength of Concrete Slender Beam Prediction." Applied Sciences. 10 (11), pp. 1-25. https://doi.org/10.3390/app10113811Integrative stochastic model standardization with genetic algorithm for rainfall pattern forecasting in tropical and semi-arid environments
Salih, Sinan Q., Sharafati, Ahmad, Ebtehaj, Isa, Sanikhani, Hadi, Siddique, Ridwan, Deo, Ravinesh C., Bonakdari, Hossein, Shahid, Shamsuddin and Yaseen, Zaher Mundher. 2020. "Integrative stochastic model standardization with genetic algorithm for rainfall pattern forecasting in tropical and semi-arid environments." Hydrological Sciences Journal. 65 (7), pp. 1145-1157. https://doi.org/10.1080/02626667.2020.1734813Hybridized neural fuzzy ensembles for dust source modeling and prediction
Rahmati, Omid, Panahi, Mahdi, Ghiasi, Seid Saeid, Deo, Ravinesh C., Tiefenbacher, John P., Pradhan, Biswajeet, Jahani, Ali, Goshtasb, Hamid, Kornejady, Aiding, Shahabi, Himan, Shirzadi, Ataollah, Khosravi, Hassan, Moghaddam, Davoud Davoudi, Mohtashamian, Maryamsadat and Bui, Dieu Tien. 2020. "Hybridized neural fuzzy ensembles for dust source modeling and prediction." Atmospheric Environment. 224, pp. 1-11. https://doi.org/10.1016/j.atmosenv.2020.117320Global solar radiation estimation and climatic variability analysis using extreme learning machine based predictive model
Tao, Hai, Sharafati, Ahmad, Mohammed, Achite, Salih, Sinan Q., Deo, Ravinesh C., Al-Ansari, Nadhir and Yaseen, Zaher Mundher. 2020. "Global solar radiation estimation and climatic variability analysis using extreme learning machine based predictive model." IEEE Access. 8, pp. 12026-12042. https://doi.org/10.1109/ACCESS.2020.2965303Development and evaluation of the cascade correlation neural network and the random forest models for river stage and river flow prediction in Australia
Ghorbani, Mohammad Ali, Deo, Ravinesh C., Kim, Sungwon, Kashani, Mahasa Hasanpour, Karimi, Vahid and Izadkhah, Maryam. 2020. "Development and evaluation of the cascade correlation neural network and the random forest models for river stage and river flow prediction in Australia." Soft Computing. 24, pp. 12079-12090. https://doi.org/10.1007/s00500-019-04648-2Causality of climate, food production and conflict over the last two millennia in the Hexi Corridor, China
Yang, Linshan, Feng, Qi, Adamowski, Jan F., Deo, Ravinesh C., Yin, Zhenlaing, Wen, Xiaohu, Tang, Xia and Wu, Min. 2020. "Causality of climate, food production and conflict over the last two millennia in the Hexi Corridor, China." Science of the Total Environment. 713, pp. 1-11. https://doi.org/10.1016/j.scitotenv.2020.136587Mixing characteristics of a film-exciting flapping jet
Wu, M., Xu, M., Mi, J. and Deo, R. C.. 2020. "Mixing characteristics of a film-exciting flapping jet." International Journal of Heat and Fluid Flow. 82, pp. 1-9. https://doi.org/10.1016/j.ijheatfluidflow.2019.108532Influence of Clouds on OMI Satellite Total Daily UVA Exposure over a 12-year Period at a Southern Hemisphere Site
A Jebar, Mustapha A., Parisi, Alfio V., Downs, Nathan J. and Turner, Joanna. 2020. "Influence of Clouds on OMI Satellite Total Daily UVA Exposure over a 12-year Period at a Southern Hemisphere Site." International Journal of Remote Sensing. 41 (1), pp. 272-283. https://doi.org/10.1080/01431161.2019.1641243Enhanced deep learning algorithm development to detect pain intensity from facial expression images
Bargshady, Ghazal, Zhou, Xujuan, Deo, Ravinesh C., Soar, Jeffery, Whittaker, Frank and Wang, Hua. 2020. "Enhanced deep learning algorithm development to detect pain intensity from facial expression images." Expert Systems with Applications. 149, pp. 1-10. https://doi.org/10.1016/j.eswa.2020.113305A general extensible learning approach for multi-disease recommendations in a telehealth environment
Lafta, Raid, Zhang, Ji, Tao, Xiaohui, Zhu, Xiaodong, Li, Hongzhou, Chang, Liang and Deo, Ravinesh. 2020. "A general extensible learning approach for multi-disease recommendations in a telehealth environment ." Pattern Recognition Letters. 132, pp. 106-114. https://doi.org/10.1016/j.patrec.2018.11.006A hybrid air quality early-warning framework: an hourly forecasting model with online sequential extreme learning machines and empirical mode decomposition algorithms
Sharma, Ekta, Deo, Ravinesh C., Prasad, Ramendra and Parisi, Alfio V.. 2020. "A hybrid air quality early-warning framework: an hourly forecasting model with online sequential extreme learning machines and empirical mode decomposition algorithms." Science of the Total Environment. 709, pp. 1-23. https://doi.org/10.1016/j.scitotenv.2019.135934Machine learning approaches for spatial modeling of agricultural droughts in the south-east region of Queensland Australia
Rahmati, Omid, Falah, Fatemeh, Dayal, Kavina Shaanu, Deo, Ravinesh C., Mohammadi, Farnoush, Biggs, Trent, Moghaddam, Davoud Davoudi, Naghibi, Seyed Amir and Bui, Dieu Tien. 2020. "Machine learning approaches for spatial modeling of agricultural droughts in the south-east region of Queensland Australia." Science of the Total Environment. 699 (134230). https://doi.org/10.1016/j.scitotenv.2019.134230Complete ensemble empirical mode decomposition hybridized with random forest and kernel ridge regression model for monthly rainfall forecasts
Ali, Mumtaz, Prasad, Ramendra, Xiang, Yong and Yaseen, Z.. 2020. "Complete ensemble empirical mode decomposition hybridized with random forest and kernel ridge regression model for monthly rainfall forecasts." Journal of Hydrology. 584, pp. 1-15. https://doi.org/10.1016/j.jhydrol.2020.124647Preparing teachers to instruct students with autism in inclusive settings: Australian pre-service teachers’ and recent graduates’ perspectives - an exploratory case study
Devi, Aruna. 2019. Preparing teachers to instruct students with autism in inclusive settings: Australian pre-service teachers’ and recent graduates’ perspectives - an exploratory case study. Doctorate other than PhD Doctor of Education. University of Southern Queensland. https://doi.org/10.26192/64jd-sq48The Simulated Ocular and Whole-Body Distribution of Natural Sunlight to Kiteboarders: A High-Risk Case of UVR Exposure for Athletes Utilizing Water Surfaces in Sport
Downs, Nathan J., Parisi, Alfio V., Schouten, Peter W., Igoe, Damien P. and De Castro-Maqueda, Guillermo. 2020. "The Simulated Ocular and Whole-Body Distribution of Natural Sunlight to Kiteboarders: A High-Risk Case of UVR Exposure for Athletes Utilizing Water Surfaces in Sport." Photochemistry and Photobiology. 96 (4), pp. 926-935. https://doi.org/10.1111/PHP.13200Beyond the current Smartphone Application: Using smartphone hardware to measure UV radiation
Turner, J., Igoe, D. P., Parisi, A. V., Downs, N. J. and Amar, A.. 2018. "Beyond the current Smartphone Application: Using smartphone hardware to measure UV radiation." NIWA UV Workshop 2018 - UV Radiation: Effects on Human Health and the Environment. Wellington, New Zealand 04 - 06 Apr 2018 New Zealand.Projected spatial patterns in precipitation and air temperature for China's northwest region derived from high‐resolution regional climate models
Yin, Zhenliang, Feng, Qi, Yang, Linshan, Deo, Ravinesh C., Adamowski, Jan F., Wen, Xiaohu, Jia, Bing and Si, Jianhua. 2020. "Projected spatial patterns in precipitation and air temperature for China's northwest region derived from high‐resolution regional climate models." International Journal of Climatology. 40 (8), pp. 3922-3941. https://doi.org/10.1002/joc.6435Exploring solar and wind energy resources in North Korea with COMS MI geostationary satellite data coupled with numerical weather prediction reanalysis variables
Yeom, Jong-Min, Deo, Ravinesh, Adamowski, Jan F., Chae, Taebyeong, Kim, Dong-Su, Han, Kyung-Soo and Kim, Do-Yong. 2020. "Exploring solar and wind energy resources in North Korea with COMS MI geostationary satellite data coupled with numerical weather prediction reanalysis variables." Renewable and Sustainable Energy Reviews. 119, pp. 1-14. https://doi.org/10.1016/j.rser.2019.109570Capability and robustness of novel hybridized models used for drought hazard modeling in southeast Queensland, Australia
Rahmati, Omid, Panahi, Mahdi, Kalantari, Zahra, Soltani, Elinaz, Falah, Fatemeh, Dayal, Kavina S., Mohammadi, Farnoush, Deo, Ravinesh C., Tiefenbacher, John and Bui, Dieu Tien. 2020. "Capability and robustness of novel hybridized models used for drought hazard modeling in southeast Queensland, Australia." Science of the Total Environment. 718, pp. 1-17. https://doi.org/10.1016/j.scitotenv.2019.134656Regional hydrology heterogeneity and the response to climate and land surface changes in arid alpine basin, northwest China
Yang, Linshan, Feng, Qi, Yin, Zhenliang, Deo, Ravinesh C., Wen, Xiaohu, Si, Jianhua and Liu, Wen. 2020. "Regional hydrology heterogeneity and the response to climate and land surface changes in arid alpine basin, northwest China." Catena. 187. https://doi.org/10.1016/j.catena.2019.104345A site-specific standard for comparing dynamic solar ultraviolet protection characteristics of established tree canopies
Downs, N. J., Butler, H. J., Baldwin, L., Parisi, A. V., Amar, A., Vanos, J. and Harrison, S.. 2019. "A site-specific standard for comparing dynamic solar ultraviolet protection characteristics of established tree canopies." MethodsX. 6, pp. 1683-1693. https://doi.org/10.1016/j.mex.2019.07.013Seasonal Minimum and Maximum Solar Ultraviolet Exposure Measurements of Classroom Teachers Residing in Tropical North Queensland, Australia
Downs, Nathan J., Igoe, Damien P., Parisi, Alfio V., Taylor, Olivia, Lazzaroni, Shari L., Rawlings, Alex, Garzon-Chavez, Daniel R. and Harrison, Simone L.. 2019. "Seasonal Minimum and Maximum Solar Ultraviolet Exposure Measurements of Classroom Teachers Residing in Tropical North Queensland, Australia." Photochemistry and Photobiology. 95, pp. 1083-1093. https://doi.org/10.1111/php.13081An ensemble tree-based machine learning model for predicting the uniaxial compressive strength of travertine rocks
Barzegar, Rahim, Sattarpour, Masoud, Deo, Ravinesh, Fijani, Elham and Adamowski, Jan. 2020. "An ensemble tree-based machine learning model for predicting the uniaxial compressive strength of travertine rocks." Neural Computing and Applications. 32 (13), pp. 9065-9080. https://doi.org/10.1007/s00521-019-04418-zPrediction of evaporation in arid and semi-arid regions: a comparative study using different machine learning models
Yaseen, Zaher Mundher, Al-Juboori, Al-Juboori, Beyaztasc, Ufuk, Al-Ansari, Nadhir, Chau, Kwok-Wing, Qi, Chongchong, Ali, Mumtaz, Salih, Sinan Q. and Shahid, Shamsuddin. 2020. "Prediction of evaporation in arid and semi-arid regions: a comparative study using different machine learning models." Engineering Applications of Computational Fluid Mechanics. 14 (1), pp. 70-89. https://doi.org/10.1080/19942060.2019.1680576Modern artificial intelligence model development for undergraduate student performance prediction: an investigation on engineering mathematics courses
Deo, Ravinesh C., Yaseen, Zaher Mundher, Al-Ansari, Nadhir, Nguyen-Huy, Thong, Langlands, Trevor and Galligan, Linda. 2020. "Modern artificial intelligence model development for undergraduate student performance prediction: an investigation on engineering mathematics courses." IEEE Access. 8, pp. 136697-136724. https://doi.org/10.1109/ACCESS.2020.3010938Correcting satellite precipitation data and assimilating satellite-derived soil moisture data to generate ensemble hydrological forecasts within the HBV rainfall-runoff model
Ciupak, Maurycy, Ozga-Zielinski, Bogdan, Adamowski, Jan, Deo, Ravinesh C and Kochanek, Krzysztof. 2019. "Correcting satellite precipitation data and assimilating satellite-derived soil moisture data to generate ensemble hydrological forecasts within the HBV rainfall-runoff model." Water: an open access journal. 11 (10), pp. 1-31. https://doi.org/10.3390/w11102138Partitioning groundwater recharge sources in multiple aquifers system within a desert oasis environment: Implications for water resources management in endorheic basins
Guo, Xiaoyan, Feng, Qi, Si, Jianhua, Xi, Haiyang, Zhao, Yan and Deo, Ravinesh C.. 2019. "Partitioning groundwater recharge sources in multiple aquifers system within a desert oasis environment: Implications for water resources management in endorheic basins." Journal of Hydrology. 579, pp. 1-11. https://doi.org/10.1016/j.jhydrol.2019.124212Probabilistic seasonal rainfall forecasts using semiparametric d-vine copula-based quantile regression
Nguyen-Huy, Thong, Deo, Ravinesh C., Mushtaq, Shahbaz and Khan, Shahjahan. 2020. "Probabilistic seasonal rainfall forecasts using semiparametric d-vine copula-based quantile regression." Samui, Pijush, Bui, Dieu Tien, Chakraborty, Subrata and Deo, Ravinesh C. (ed.) Handbook of probabilistic models. Oxford, United Kingdom. Elsevier. pp. 203-227Monthly rainfall forecasting with Markov Chain Monte Carlo simulations integrated with statistical bivariate copulas
Ali, Mumtaz, Deo, Ravinesh C., Downs, Nathan J. and Maraseni, Tek. 2020. "Monthly rainfall forecasting with Markov Chain Monte Carlo simulations integrated with statistical bivariate copulas." Samui, Pijush, Bui, Dieu Tien, Chakraborty, Subrata and Deo, Ravinesh C. (ed.) Handbook of probabilistic models. Oxford, United Kingdom. Elsevier. pp. 89-105Development of copula statistical drought prediction model using the Standardized Precipitation-Evapotranspiration Index
Dayal, Kavina S., Deo, Ravinesh C. and Apan, Armando A.. 2020. "Development of copula statistical drought prediction model using the Standardized Precipitation-Evapotranspiration Index." Samui, Pijush, Bui, Dieu Tien, Chakraborty, Subrata and Deo, Ravinesh C. (ed.) Handbook of probabilistic models. Oxford, United Kingdom. Elsevier. pp. 141-178Soil organic carbon in semiarid alpine regions: the spatial distribution, stock estimation, and environmental controls
Zhu, Meng, Feng, Qi, Zhang, Mengxu, Liu, Wei, Deo, Ravinesh C., Zhang, Chengqi and Yang, Linshan. 2019. "Soil organic carbon in semiarid alpine regions: the spatial distribution, stock estimation, and environmental controls." Journal of Soils and Sediments: protection, risk assessment and remediation. 19 (10), pp. 3427-3441. https://doi.org/10.1007/s11368-019-02295-6Controlling factors of plant community composition with respect to the slope aspect gradient in the Qilian Mountains
Qin, Yanyan, Adamowski, Jan F., Deo, Ravinesh C., Hu, Zeyong, Cao, Jianjun, Zhu, Meng and Feng, Qi. 2019. "Controlling factors of plant community composition with respect to the slope aspect gradient in the Qilian Mountains." Ecosphere. 10 (9), pp. 1-13. https://doi.org/10.1002/ecs2.2851Grassland degradation on the Qinghai-Tibetan Plateau: reevaluation of causative factors
Cao, Jianjun, Adamowski, Jan F., Deo, Ravinesh C., Xu, Xueyun, Gong, Yifan and Feng, Qi. 2019. "Grassland degradation on the Qinghai-Tibetan Plateau: reevaluation of causative factors." Rangeland Ecology and Management. 72 (6), pp. 988-995. https://doi.org/10.1016/j.rama.2019.06.001Fractal dimension undirected correlation graph-based support vector machine model for identification of focal and non-focal electroencephalography signals
Diykh, Mohammed, Abdulla, Shahab, Saleh, Khalid and Deo, Ravinesh C.. 2019. "Fractal dimension undirected correlation graph-based support vector machine model for identification of focal and non-focal electroencephalography signals." Biomedical Signal Processing and Control. 54, pp. 1-10. https://doi.org/10.1016/j.bspc.2019.101611The impacts of substrate and vegetation on stormwater runoff quality from extensive green roofs
Liu, Wen, Wei, Wei, Chen, Weiping, Deo, Ravinesh C., Si, Jianhua, Xi, Haiyang, Li, Baofeng and Feng, Qi. 2019. "The impacts of substrate and vegetation on stormwater runoff quality from extensive green roofs." Journal of Hydrology. 576, pp. 575-582. https://doi.org/10.1016/j.jhydrol.2019.06.061Application of multivariate recursive nesting bias correction, multiscale wavelet entropy and AI-based models to improve future precipitation projection in upstream of the Heihe River, Northwest China
Yang, Linshan, Feng, Qi, Yin, Zhenliang, Wen, Xiaohu, Deo, Ravinesh C., Si, Jianhua and Li, Changbin. 2019. "Application of multivariate recursive nesting bias correction, multiscale wavelet entropy and AI-based models to improve future precipitation projection in upstream of the Heihe River, Northwest China." Theoretical and Applied Climatology. 137 (1-2), pp. 323-339. https://doi.org/10.1007/s00704-018-2598-yWavelet-based 3-phase hybrid SVR model trained with satellite-derived predictors, particle swarm optimization and maximum overlap discrete wavelet transform for solar radiation prediction
Ghimire, Sujan, Deo, Ravinesh C., Raj, Nawin and Mi, Jianchun. 2019. "Wavelet-based 3-phase hybrid SVR model trained with satellite-derived predictors, particle swarm optimization and maximum overlap discrete wavelet transform for solar radiation prediction." Renewable and Sustainable Energy Reviews. 113, pp. 1-19. https://doi.org/10.1016/j.rser.2019.109247Deep solar radiation forecasting with convolutional neural network and long short-term memory network algorithms
Ghimire, Sujan, Deo, Ravinesh C., Raj, Nawin and Mi, Jianchun. 2019. "Deep solar radiation forecasting with convolutional neural network and long short-term memory network algorithms." Applied Energy. 253, pp. 1-20. https://doi.org/10.1016/j.apenergy.2019.113541Sleep EEG signal analysis based on correlation graph similarity coupled with an ensemble extreme machine learning algorithm
Abdulla, Shahab, Diykh, Mohammed, Lafta, Raid Luaibi, Saleh, Khalid and Deo, Ravinesh C.. 2019. "Sleep EEG signal analysis based on correlation graph similarity coupled with an ensemble extreme machine learning algorithm." Expert Systems with Applications. 138, pp. 1-15. https://doi.org/10.1016/j.eswa.2019.07.007Short-term electricity demand forecasting using machine learning methods enriched with ground-based climate and ECMWF Reanalysis atmospheric predictors in southeast Queensland, Australia
Al-Musaylh, Mohanad S., Deo, Ravinesh C., Adamowski, Jan F. and Li, Yan. 2019. "Short-term electricity demand forecasting using machine learning methods enriched with ground-based climate and ECMWF Reanalysis atmospheric predictors in southeast Queensland, Australia." Renewable and Sustainable Energy Reviews. 113. https://doi.org/10.1016/j.rser.2019.109293Comparing the annualised dynamic shade characteristics of twenty-one tree canopies across twenty-six municipalities in a high ambient UV climate, Queensland - Australia
Downs, Nathan J., Baldwin, Louise, Parisi, Alfio V., Butler, Harry J., Vanos, Jennifer, Beckman, Melanie and Harrison, Simone. 2019. "Comparing the annualised dynamic shade characteristics of twenty-one tree canopies across twenty-six municipalities in a high ambient UV climate, Queensland - Australia." Applied Geography. 108, pp. 74-82. https://doi.org/10.1016/j.apgeog.2019.05.006Cloud segmentation property extraction from total sky image repositories using Python
Igoe, Damien P., Parisi, Alfio V. and Downs, Nathan J.. 2019. "Cloud segmentation property extraction from total sky image repositories using Python." Instrumentation Science and Technology. 47 (5), pp. 522-534. https://doi.org/10.1080/10739149.2019.1603996Designing a new data intelligence model for global solar radiation prediction: application of multivariate modeling scheme
Tao, Hai, Ebtehaj, Isa, Bonakdari, Hossein, Heddam, Salim, Voyant, Cyril, Al-Ansari, Nadhir, Deo, Ravinesh and Yaseen, Zaheer Mundher. 2019. "Designing a new data intelligence model for global solar radiation prediction: application of multivariate modeling scheme." Energies. 12 (7), pp. 1-24. https://doi.org/10.3390/en12071365Incorporating synoptic-scale climate signals for streamflow modelling over the Mediterranean region using machine learning models
Kisi, Ozgur, Choubin, Bahram, Deo, Ravinesh C. and Yaseen, Zaheer Mundher. 2019. "Incorporating synoptic-scale climate signals for streamflow modelling over the Mediterranean region using machine learning models." Hydrological Sciences Journal. 64 (10), pp. 1240-1252. https://doi.org/10.1080/02626667.2019.1632460Improving SPI-derived drought forecasts incorporating synoptic-scale climate indices in multi-phase multivariate empirical mode decomposition model hybridized with simulated annealing and kernel ridge regression algorithms
Ali, Mumtaz, Deo, Ravinesh C., Maraseni, Tek and Downs, Nathan J.. 2019. "Improving SPI-derived drought forecasts incorporating synoptic-scale climate indices in multi-phase multivariate empirical mode decomposition model hybridized with simulated annealing and kernel ridge regression algorithms." Journal of Hydrology. 576, pp. 164-184. https://doi.org/10.1016/j.jhydrol.2019.06.032Deep Learning Neural Networks Trained with MODIS Satellite-Derived Predictors for Long-Term Global Solar Radiation Prediction
Ghimire, Sujan, Deo, Ravinesh C., Raj, Nawin and Mi, Jianchun. 2019. "Deep Learning Neural Networks Trained with MODIS Satellite-Derived Predictors for Long-Term Global Solar Radiation Prediction." Energies. 12 (12), pp. 1-42. https://doi.org/10.3390/en12122407Situations, challenges and strategies of urban water management in Beijing under rapid urbanization effect
Liu, Wen, Chen, Weiping, Feng, Qi and Deo, Ravinesh C.. 2019. "Situations, challenges and strategies of urban water management in Beijing under rapid urbanization effect." Water Science and Technology: Water Supply. 19 (1), pp. 115-127. https://doi.org/10.2166/ws.2018.057A novel framework for distress detection through an automated speech processing system
Rana, Rajib, Gururajan, Raj, Mackenzie, Geraldine, Dunn, Jeff, Gray, Anthony, Zhou, Xujuan, Barua, Prabal Datta, Epps, Julien and Humphris, Gerald Michael. 2018. "A novel framework for distress detection through an automated speech processing system." 2018 IEEE/WIC/ACM International Conference on Web Intelligence (WI 2018). Santiago, Chile 03 - 06 Dec 2018 Los Alamitos, CA, United States. https://doi.org/10.1109/WI.2018.00-29Determination of factors influencing student engagement using a learning management system in a tertiary setting
Barua, Prabal Datta, Zhou, Xujuan, Gururajan, Raj and Chan, Ka C.. 2018. "Determination of factors influencing student engagement using a learning management system in a tertiary setting." 2018 IEEE/WIC/ACM International Conference on Web Intelligence (WI 2018). Santiago, Chile 03 - 06 Dec 2018 Los Alamitos, CA, United States. https://doi.org/10.1109/WI.2018.00-30Biologically effective solar ultraviolet exposures and the potential skin cancer risk for individual gold medalists of the 2020 Tokyo Summer Olympic Games
Downs, Nathan J., Axelsen, Taryn, Schouten, Peter, Igoe, Damien P., Parisi, Alfio V. and Vanos, Jennifer. 2019. "Biologically effective solar ultraviolet exposures and the potential skin cancer risk for individual gold medalists of the 2020 Tokyo Summer Olympic Games." Temperature. https://doi.org/10.1080/23328940.2019.1581427Suitable exclosure duration for the restoration of degraded alpine grasslands on the Qinghai-Tibetan Plateau
Cao, Jianjun, Li, Guangdong, Adamowski, Jan F., Holden, Nicolas M., Deo, Ravinesh C., Hu, Zeyong, Zhu, Guofeng, Xu, Xueyun and Feng, Qi. 2019. "Suitable exclosure duration for the restoration of degraded alpine grasslands on the Qinghai-Tibetan Plateau." Land Use Policy: the international journal covering all aspects of land use. 86, pp. 261-267. https://doi.org/10.1016/j.landusepol.2019.05.008Environmental and economic impacts and trade-offs from simultaneous management of soil constraints, nitrogen and water
Kodur, Shreevatsa, Shrestha, Uttam Babu, Maraseni, Tek Narayan and Deo, Ravinesh C.. 2019. "Environmental and economic impacts and trade-offs from simultaneous management of soil constraints, nitrogen and water." Journal of Cleaner Production. 222, pp. 960-970. https://doi.org/10.1016/j.jclepro.2019.03.079Land subsidence modelling using tree-based machine learning algorithms
Rahmati, Omid, Falah, Fatemeh, Naghibi, Seyed Amir, Biggs, Trent, Soltani, Milad, Deo, Ravinesh C., Cerda, Artemi, Mohammadi, Farnoush and Bui, Dieu Tien. 2019. "Land subsidence modelling using tree-based machine learning algorithms." Science of the Total Environment. 672, pp. 239-252. https://doi.org/10.1016/j.scitotenv.2019.03.496Weekly soil moisture forecasting with multivariate sequential, ensemble empirical mode decomposition and Boruta-random forest hybridizer algorithm approach
Prasad, Ramendra, Deo, Ravinesh C., Li, Yan and Maraseni, Tek. 2019. "Weekly soil moisture forecasting with multivariate sequential, ensemble empirical mode decomposition and Boruta-random forest hybridizer algorithm approach." Catena. 177, pp. 149-166. https://doi.org/10.1016/j.catena.2019.02.012Copula statistical models for analyzing stochastic dependencies of systemic drought risk and potential adaptation strategies
Nguyen-Huy, Thong, Deo, Ravinesh C., Mushtaq, Shahbaz, Kath, Jarrod and Khan, Shahjahan. 2019. "Copula statistical models for analyzing stochastic dependencies of systemic drought risk and potential adaptation strategies." Stochastic Environmental Research and Risk Assessment. 33 (3), pp. 779-799. https://doi.org/10.1007/s00477-019-01662-6Effects of stand age on carbon storage in dragon spruce forest ecosystems in the upper reaches of the Bailongjiang River basin, China
Cao, Jianjun, Gong, Yifan, Adamowski, Jan F., Deo, Ravinesh C., Zhu, Guofeng, Dong, Xiaogang, Zhang, Xiaofang, Liu, Haibo and Xin, Cunlin. 2019. "Effects of stand age on carbon storage in dragon spruce forest ecosystems in the upper reaches of the Bailongjiang River basin, China." Scientific Reports. 9 (1), pp. 1-11. https://doi.org/10.1038/s41598-019-39626-zThe role of topography in shaping the spatial patterns of soil organic carbon
Zhu, Meng, Feng, Qi, Qin, Yanyan, Cao, Jiajun, Zhang, Mengxu, Liu, Wei, Deo, Ravinesh C., Zhang, Chengqi, Li, Ruolin and Li, Baofeng. 2019. "The role of topography in shaping the spatial patterns of soil organic carbon." Catena. 176, pp. 296-305. https://doi.org/10.1016/j.catena.2019.01.029PMT: new analytical framework for automated evaluation of geo-environmental modelling approaches
Rahmati, Omid, Kornejady, Aiding, Samadi, Mahmood, Deo, Ravinesh C., Conoscenti, Christian, Lombardo, Luigi, Dayal, Kavina, Taghizadeh-Mehrjardi, Ruhollah, Pourghasemi, Hamid Reza, Kumar, Sandeep and Bui, Dieu Tien. 2019. "PMT: new analytical framework for automated evaluation of geo-environmental modelling approaches." Science of the Total Environment. 664, pp. 296-311. https://doi.org/10.1016/j.scitotenv.2019.02.017Universally deployable extreme learning machines integrated with remotely sensed MODIS satellite predictors over Australia to forecast global solar radiation: a new approach
Deo, Ravinesh C., Sahin, Mehmet, Adamowski, Jan F. and Mi, Jianchun. 2019. "Universally deployable extreme learning machines integrated with remotely sensed MODIS satellite predictors over Australia to forecast global solar radiation: a new approach." Renewable and Sustainable Energy Reviews. 104, pp. 235-261. https://doi.org/10.1016/j.rser.2019.01.009Two-phase extreme learning machines integrated with the complete ensemble empirical mode decomposition with adaptive noise algorithm for multi-scale runoff prediction problems
Wen, Xiaohu, Feng, Qi, Deo, Ravinesh C., Wu, Min, Yin, Zhenliang, Yang, Linshan and Singh, Vijay P.. 2019. "Two-phase extreme learning machines integrated with the complete ensemble empirical mode decomposition with adaptive noise algorithm for multi-scale runoff prediction problems." Journal of Hydrology. 570, pp. 167-184. https://doi.org/10.1016/j.jhydrol.2018.12.060New approach for sediment yield forecasting with a two-phase feed forward neuron network-particle swarm optimization model integrated with the gravitational search algorithm
Meshram, Sarita Gajbhiye, Ghorbani, M. A., Deo, Ravinesh C., Kashani, Mahsa Hasanpour, Meshram, Chandrashekhar and Karimi, Vahid. 2019. "New approach for sediment yield forecasting with a two-phase feed forward neuron network-particle swarm optimization model integrated with the gravitational search algorithm." Water Resources Management. 33 (7), pp. 2335-2356. https://doi.org/10.1007/s11269-019-02265-0Long-term modelling of wind speeds using six different heuristic artificial intelligence approaches
Maroufpoor, Saman, Sanikhani, Hadi, Kisi, Ozgur, Deo, Ravinesh C. and Yaseen, Zaher Mundher. 2019. "Long-term modelling of wind speeds using six different heuristic artificial intelligence approaches." International Journal of Climatology. 39 (8), pp. 3543-3557. https://doi.org/10.1002/joc.6037Development and evaluation of hybrid artificial neural network architectures for modeling spatio-temporal groundwater fluctuations in a complex aquifer system
Roshni, Thendiyath, Jha, Madan K., Deo, Ravinesh C. and Vandana, A.. 2019. "Development and evaluation of hybrid artificial neural network architectures for modeling spatio-temporal groundwater fluctuations in a complex aquifer system." Water Resources Management. 33 (7), pp. 2381-2397. https://doi.org/10.1007/s11269-019-02253-4An enhanced extreme learning machine model for river flow forecasting: state-of-the-art, practical applications in water resource engineering area and future research direction
Yaseen, Zaher Mundher, Sulaiman, Sadeq Oleiwi, Deo, Ravinesh C. and Chau, Kwok-Wing. 2019. "An enhanced extreme learning machine model for river flow forecasting: state-of-the-art, practical applications in water resource engineering area and future research direction." Journal of Hydrology. 569, pp. 387-408. https://doi.org/10.1016/j.jhydrol.2018.11.069The influence of structural factors on stormwater runoff retention of extensive green roofs: new evidence from scale-based models and real experiments
Liu, Wen, Feng, Qi, Chen, Weiping, Wei, Wei and Deo, Ravinesh C.. 2019. "The influence of structural factors on stormwater runoff retention of extensive green roofs: new evidence from scale-based models and real experiments." Journal of Hydrology. 569, pp. 230-238. https://doi.org/10.1016/j.jhydrol.2018.11.066Development of a model for calculating the solar ultraviolet protection factor of small to medium sized built shade structure
Parisi, Alfio V., Amar, Abdurazaq, Downs, Nathan J., Igoe, Damien P., Harrison, Simone L. and Turner, Joanna. 2019. "Development of a model for calculating the solar ultraviolet protection factor of small to medium sized built shade structure." Building and Environment. 147, pp. 415-421. https://doi.org/10.1016/j.buildenv.2018.10.010Direct and indirect impacts of ionic components of saline water on irrigated soil chemical and microbial processes
Chen, Lijuan, Li, Changsheng, Feng, Qi, Wei, Yongping, Zhao, Yan, Zhu, Meng and Deo, Ravinesh C.. 2019. "Direct and indirect impacts of ionic components of saline water on irrigated soil chemical and microbial processes." Catena. 172, pp. 581-589. https://doi.org/10.1016/j.catena.2018.09.030Global solar radiation prediction by ANN integrated with European Centre for medium range weather forecast fields in solar rich cities of Queensland Australia
Ghimire, Sujan, Deo, Ravinesh C., Downs, Nathan J. and Raj, Nawin. 2019. "Global solar radiation prediction by ANN integrated with European Centre for medium range weather forecast fields in solar rich cities of Queensland Australia." Journal of Cleaner Production. 216, pp. 288-310. https://doi.org/10.1016/j.jclepro.2019.01.158Future projection with an extreme-learning machine and support vector regression of reference evapotranspiration in a mountainous inland watershed in north-west China
Yin, Zhenliang, Feng, Qi, Yang, Linshan, Deo, Ravinesh C., Wen, Xiaohu, Si, Jianhua and Xiao, Shengchun. 2017. "Future projection with an extreme-learning machine and support vector regression of reference evapotranspiration in a mountainous inland watershed in north-west China." Water: an open access journal. 9 (11), pp. 880-902. https://doi.org/10.3390/w9110880The spatial and temporal contribution of glacier runoff to watershed discharge in the Yarkant River Basin, Northwest China
Yin, Zhenliang, Feng, Qi, Liu, Shiyin, Zhou, Songbing, Li, Jing, Yang, Linshan and Deo, Ravinesh C.. 2017. "The spatial and temporal contribution of glacier runoff to watershed discharge in the Yarkant River Basin, Northwest China." Water: an open access journal. 9 (3), pp. 159-178. https://doi.org/10.3390/w9030159A new nested ensemble technique for automated diagnosis of breast cancer
Abdar, Moloud, Zomorodi-Moghadam, Mariam, Zhou, Xujuan, Gururajan, Raj, Tao, Xiaohui, Barua, Prabal D. and Gururajan, Rashmi. 2020. "A new nested ensemble technique for automated diagnosis of breast cancer." Pattern Recognition Letters. https://doi.org/10.1016/j.patrec.2018.11.004A pilot observational study of environmental summertime health risk behaviour in central Brisbane, Queensland: opportunities to raise sun protection awareness in Australia’s Sunshine State
Dexter, Ben, King, Rachel, Harrison, Simone L., Parisi, Alfio V. and Downs, Nathan J.. 2019. "A pilot observational study of environmental summertime health risk behaviour in central Brisbane, Queensland: opportunities to raise sun protection awareness in Australia’s Sunshine State." Photochemistry and Photobiology. 95 (2), pp. 650-655. https://doi.org/10.1111/php.13011Global Solar Radiation Prediction Using Hybrid Online Sequential Extreme Learning Machine Model
Hou, Muzhou, Zhang, Tianle, Weng, Futian, Ali, Mumtaz, Al-Ansari, Nadhir and Yaseen, Zaher Mundher. 2018. "Global Solar Radiation Prediction Using Hybrid Online Sequential Extreme Learning Machine Model." Energies. 11 (12), pp. 1-19. https://doi.org/10.3390/en11123415Evaluated UVA irradiances over a twelve year period at a sub-tropical site from ozone monitoring instrument data including the influence of cloud
A Jebar, Mustapha A., Parisi, Alfio V., Downs, Nathan J. and Turner, Joanna F.. 2018. "Evaluated UVA irradiances over a twelve year period at a sub-tropical site from ozone monitoring instrument data including the influence of cloud." Photochemistry and Photobiology. 94 (6), pp. 1281-1288. https://doi.org/10.1111/php.12948Particle swarm optimized–support vector regression hybrid model for daily horizon electricity demand forecasting using climate dataset
Al-Musaylh, Mohanad S., Deo, Ravinesh C. and Li, Yan. 2018. "Particle swarm optimized–support vector regression hybrid model for daily horizon electricity demand forecasting using climate dataset." Huang, Q. and Kolhe, M. (ed.) 3rd International Conference on Power and Renewable Energy (ICPRE 2018). Berlin, Germany 21 - 24 Sep 2018 Germany. https://doi.org/10.1051/e3sconf/20186408001Effects of topography on soil organic carbon stocks in grasslands of a semiarid alpine region, northwestern China
Zhu, Meng, Feng, Qi, Zhang, Mengxu, Liu, Wei, Qin, Yanyan, Deo, Ravinesh C. and Zhang, Chengqi. 2019. "Effects of topography on soil organic carbon stocks in grasslands of a semiarid alpine region, northwestern China." Journal of Soils and Sediments: protection, risk assessment and remediation. 19 (4), pp. 1640-1650. https://doi.org/10.1007/s11368-018-2203-0A joint deep neural network model for pain recognition from face
Bargshady, Ghazal, Soar, Jeffrey, Zhou, Xujuan, Deo, Ravinesh, Whittaker, Frank and Wang, Hua. 2019. "A joint deep neural network model for pain recognition from face." 4th IEEE International Conference on Computer and Communication Systems (ICCCS 2019). Singapore 23 - 25 Feb 2019 Singapore. pp. 52-56 https://doi.org/10.1109/CCOMS.2019.8821779Effects of Afforestation on Soil Bulk Density and pH in the Loess Plateau, China
Zhang, Xiaofang, Adamowski, Jan F., Deo, Ravinesh C., Xu, Xueyun, Zhu, Guofeng and Cao, Jianjun. 2018. "Effects of Afforestation on Soil Bulk Density and pH in the Loess Plateau, China." Water: an open access journal. 10 (12), pp. 1-15. https://doi.org/10.3390/w10121710Assessment of soil salinisation in the Ejina Oasis located in the lower reaches of Heihe River, Northwestern China
Zhao, Yu, Feng, Qi, Lu, Aigang and Deo, Ravinesh C.. 2019. "Assessment of soil salinisation in the Ejina Oasis located in the lower reaches of Heihe River, Northwestern China." Chemistry and Ecology. 35 (4), pp. 330-343. https://doi.org/10.1080/02757540.2018.1554060Design and implementation of a hybrid MLP-GSA model with multilayer perceptron-gravitational search algorithm for monthly lake water level forecasting
Ghorbani, Mohammad Ali, Deo, Ravinesh C., Karimi, Vahid, Kashani, Mahsa H. and Ghorbani, Shahryar. 2018. "Design and implementation of a hybrid MLP-GSA model with multilayer perceptron-gravitational search algorithm for monthly lake water level forecasting." Stochastic Environmental Research and Risk Assessment. 33 (1), pp. 125-147. https://doi.org/10.1007/s00477-018-1630-1Monitoring paddy productivity in North Korea employing geostationary satellite images integrated with GRAMI-rice model
Yeom, Jong-min, Jeong, Seungtaek, Jeong, Gwanyong, Ng, Chi Tim, Deo, Ravinesh C. and Ko, Jonghan. 2018. "Monitoring paddy productivity in North Korea employing geostationary satellite images integrated with GRAMI-rice model." Scientific Reports. 8, pp. 1-15. https://doi.org/10.1038/s41598-018-34550-0Artificial intelligence approach for the prediction of Robusta coffee yield using soil fertility properties
Kouadio, Louis, Deo, Ravinesh C., Byrareddy, Vivekananda, Adamowski, Jan F., Mushtaq, Shahbaz and Nguyen, Van Phuong. 2018. "Artificial intelligence approach for the prediction of Robusta coffee yield using soil fertility properties." Computers and Electronics in Agriculture. 155, pp. 324-338. https://doi.org/10.1016/j.compag.2018.10.014Artificial intelligence-based fast and efficient hybrid approach for spatial modelling of soil electrical conductivity
Ghorbani, Mohammad Ali, Deo, Ravinesh C., Kashani, Mahsa H., Shahabi, Mahmoud and Ghorbani, Shahryar. 2019. "Artificial intelligence-based fast and efficient hybrid approach for spatial modelling of soil electrical conductivity." Soil and Tillage Research. 186, pp. 152-164. https://doi.org/10.1016/j.still.2018.09.012Quantifying flood events in Bangladesh with a daily-step flood monitoring index based on the concept of daily effective precipitation
Deo, Ravinesh C., Adamowski, Jan F., Begum, Khaleda, Salcedo-sanz, Sancho, Kim, Do-Woo, Dayal, Kavina S. and Byun, Hi-Ryong. 2019. "Quantifying flood events in Bangladesh with a daily-step flood monitoring index based on the concept of daily effective precipitation." Theoretical and Applied Climatology. 137, pp. 1201-1215. https://doi.org/10.1007/s00704-018-2657-4Shear strength prediction of steel fiber reinforced concrete beam using hybrid intelligence models: a new approach
Yaseen, Zaheer Munder, Tran, Minh Tung, Kim, Sungwon, Bakhshpoori, Taha and Deo, Ravinesh C.. 2018. "Shear strength prediction of steel fiber reinforced concrete beam using hybrid intelligence models: a new approach." Engineering Structures. 177, pp. 244-255. https://doi.org/10.1016/j.engstruct.2018.09.074Adaptive Neuro-Fuzzy Inference System integrated with solar zenith angle for forecasting sub-tropical photosynthetically active radiation
Deo, Ravinesh C., Downs, Nathan J., Adamowski, Jan F. and Parisi, Alfio V.. 2018. "Adaptive Neuro-Fuzzy Inference System integrated with solar zenith angle for forecasting sub-tropical photosynthetically active radiation." Food and Energy Security. 8 (1). https://doi.org/10.1002/fes3.151Cotton yield prediction with Markov Chain Monte Carlo-based simulation model integrated with genetic programing algorithm: a new hybrid copula-driven approach
Ali, Mumtaz, Deo, Ravinesh C., Downs, Nathan J. and Maraseni, Tek. 2018. "Cotton yield prediction with Markov Chain Monte Carlo-based simulation model integrated with genetic programing algorithm: a new hybrid copula-driven approach." Agricultural and Forest Meteorology. 263, pp. 428-448. https://doi.org/10.1016/j.agrformet.2018.09.002Weekly heat wave death prediction model using zero-inflated regression approach
Kim, Do-Woo, Deo, Ravinesh C., Park, Sang-Jin, Lee, Jong-Seol and Lee, Woo-Seop. 2019. "Weekly heat wave death prediction model using zero-inflated regression approach." Theoretical and Applied Climatology. 137 (1-2), pp. 823-838. https://doi.org/10.1007/s00704-018-2636-9A comparative study of temperature and precipitation‑based aridity indices and their trends in Mongolia
Nyamtseren, Mandakh, Feng, Qi and Deo, Ravinesh C.. 2018. "A comparative study of temperature and precipitation‑based aridity indices and their trends in Mongolia." International Journal of Environmental Research. 12, pp. 887-899. https://doi.org/10.1007/s41742-018-0143-6Can individual land ownership reduce grassland degradation and favor socioeconomic sustainability on the Qinghai-Tibetan Plateau?
Cao, J. J., Holden, N. M., Adamowski, J. F., Deo, R. C., Xu, X. Y. and Feng, Q.. 2018. "Can individual land ownership reduce grassland degradation and favor socioeconomic sustainability on the Qinghai-Tibetan Plateau?" Environmental Science and Policy. 89, pp. 192-197. https://doi.org/10.1016/j.envsci.2018.08.003Copula-based agricultural conditional value-at-risk modelling for geographical diversifications in wheat farming portfolio management
Nguyen-Huy, Thong, Deo, Ravinesh C., Mushtaq, Shahbaz, Kath, Jarrod and Khan, Shahjahan. 2018. "Copula-based agricultural conditional value-at-risk modelling for geographical diversifications in wheat farming portfolio management." Weather and Climate Extremes. 21, pp. 76-89. https://doi.org/10.1016/j.wace.2018.07.002Design and evaluation of SVR, MARS and M5Tree models for 1, 2 and 3-day lead time forecasting of river flow data in a semiarid mountainous catchment
Yin, Zhenliang, Feng, Qi, Wen, Xiaohu, Deo, Ravinesh C., Yang, Linshan, Si, Jianhua and He, Zhibin. 2018. "Design and evaluation of SVR, MARS and M5Tree models for 1, 2 and 3-day lead time forecasting of river flow data in a semiarid mountainous catchment." Stochastic Environmental Research and Risk Assessment. 32 (9), pp. 2457-2476. https://doi.org/10.1007/s00477-018-1585-2Mapping hypothermia death vulnerability in Korea
Park, Sang-Jin, Kim, Do-Woo, Deo, Ravinesh C. and Lee, Jong-Seol. 2018. "Mapping hypothermia death vulnerability in Korea." International Journal of Disaster Risk Reduction. 31, pp. 668-678. https://doi.org/10.1016/j.ijdrr.2018.06.016Multi-stage committee based extreme learning machine model incorporating the influence of climate parameters and seasonality on drought forecasting
Ali, Mumtaz, Deo, Ravinesh C., Downs, Nathan J. and Maraseni, Tek. 2018. "Multi-stage committee based extreme learning machine model incorporating the influence of climate parameters and seasonality on drought forecasting." Computers and Electronics in Agriculture. 152, pp. 149-165. https://doi.org/10.1016/j.compag.2018.07.013A real-time hourly water index for flood risk monitoring: pilot studies in Brisbane, Australia, and Dobong Observatory, South Korea
Deo, Ravinesh C., Byun, Hi-Ryong, Kim, Ga-Byn and Adamowski, Jan F.. 2018. "A real-time hourly water index for flood risk monitoring: pilot studies in Brisbane, Australia, and Dobong Observatory, South Korea." Environmental Monitoring and Assessment. 190 (450). https://doi.org/10.1007/s10661-018-6806-0Multi-stage hybridized online sequential extreme learning machine integrated with Markov Chain Monte Carlo copula-Bat algorithm for rainfall forecasting
Ali, Mumtaz, Deo, Ravinesh C., Downs, Nathan J. and Maraseni, Tek. 2018. "Multi-stage hybridized online sequential extreme learning machine integrated with Markov Chain Monte Carlo copula-Bat algorithm for rainfall forecasting." Atmospheric Research. 213, pp. 450-464. https://doi.org/10.1016/j.atmosres.2018.07.005Influence of stand type and stand age on soil carbon storage in China’s arid and semi-arid regions
Cao, Jianjun, Zhang, Xiaofang, Deo, Ravinesh, Gong, Yifan and Feng, Qi. 2018. "Influence of stand type and stand age on soil carbon storage in China’s arid and semi-arid regions." Land Use Policy: the international journal covering all aspects of land use. 78, pp. 258-265. https://doi.org/10.1016/j.landusepol.2018.07.002The geospatial relationship of pterygium and senile cataract to ambient solar ultraviolet in tropical Ecuador
Garzon-Chavez, Daniel R., Quentin, Emmanuelle, Harrison, Simone L., Parisi, Alfio V., Butler, Harry J. and Downs, Nathan J.. 2018. "The geospatial relationship of pterygium and senile cataract to ambient solar ultraviolet in tropical Ecuador." Photochemical and Photobiological Sciences. 17 (8), pp. 1075-1083. https://doi.org/10.1039/C8PP00023ADesign and implementation of a hybrid model based on two-layer decomposition method coupled with extreme learning machines to support real-time environmental monitoring of water quality parameters
Fijani, Elham, Barzegar, Rahim, Deo, Ravinesh, Tziritis, Evangelos and Konstantinos, Skordas. 2019. "Design and implementation of a hybrid model based on two-layer decomposition method coupled with extreme learning machines to support real-time environmental monitoring of water quality parameters." Science of the Total Environment. 648, pp. 839-853. https://doi.org/10.1016/j.scitotenv.2018.08.221Triathlon and the potential for solar ultraviolet exposure risk assessed during the swim, bicycle and running legs of competitive Australian Ironman events
Axelsen, Taryn, Parisi, Alfio V. and Downs, Nathan J.. 2018. "Triathlon and the potential for solar ultraviolet exposure risk assessed during the swim, bicycle and running legs of competitive Australian Ironman events." 4th International Conference on UV & Skin Cancer Prevention 2018. Toronto, Canada 01 - 04 May 2018Reflecting on fraction addition: using the mirror formula to visualise unit fraction addition
Igoe, Damien, Boucher, Nicholas, Clark, Iain, Parisi, Alfio and Downs, Nathan. 2018. "Reflecting on fraction addition: using the mirror formula to visualise unit fraction addition." Australian Mathematics Teacher. 74 (2), pp. 3-9.Optimization of windspeed prediction using an artificial neural network compared with a genetic programming model
Deo, Ravinesh C., Ghimire, Sujan, Downs, Nathan J. and Raj, Nawin. 2018. "Optimization of windspeed prediction using an artificial neural network compared with a genetic programming model." Kim, Dookie, Roy, Sanjiban Sekhar, Lansivaara, Tim, Deo, Ravinesh C. and Samui, Pijush (ed.) Handbook of research on predictive modeling and optimization methods in science and engineering. Hershey, United States. IGI Global. pp. 328-359Hybrid data intelligent models and applications for water level prediction
Yaseen, Zaher Mundher, Deo, Ravinesh C., Ebtehaj, Isa and Bonakdari, Hossein. 2018. "Hybrid data intelligent models and applications for water level prediction." Kim, Dookie, Roy, Sanjiban Sekhar, Länsivaara, Tim, Deo, Ravinesh C. and Samui, Pijush (ed.) Handbook of research on predictive modeling and optimization methods in science and engineering. Hershey, United States. IGI Global. pp. 121-139Selection of representative feature training sets with self-organized maps for optimized time series modeling and prediction: application to forecasting daily drought conditions with ARIMA and neural network models
McCarthy, Elizabeth, Deo, Ravinesh C., Li, Yan and Maraseni, Tek. 2018. "Selection of representative feature training sets with self-organized maps for optimized time series modeling and prediction: application to forecasting daily drought conditions with ARIMA and neural network models." Kim, Dookie, Roy, Sanjiban Sekhar, Lansivaara, Tim, Deo, Ravinesh C. and Samui, Pijush (ed.) Handbook of research on predictive modeling and optimization methods in science and engineering. Hershey, United States. IGI Global. pp. 446-464Survey of different data-intelligent modeling strategies for forecasting air temperature using geographic information as model predictors
Sanikhani, Hadi, Deo, Ravinesh C., Samui, Pijush, Kisi, Ozgur, Mert, Chian, Mirabbasi, Rasoul, Gavili, Siavash and Yaseen, Zaher Mundher. 2018. "Survey of different data-intelligent modeling strategies for forecasting air temperature using geographic information as model predictors." Computers and Electronics in Agriculture. 152, pp. 242-260. https://doi.org/10.1016/j.compag.2018.07.008Soil moisture forecasting by a hybrid machine learning technique: ELM integrated with ensemble empirical mode decomposition
Prasad, Ramendra, Deo, Ravinesh C., Li, Yan and Maraseni, Tek. 2018. "Soil moisture forecasting by a hybrid machine learning technique: ELM integrated with ensemble empirical mode decomposition." Geoderma. 330, pp. 136-161. https://doi.org/10.1016/j.geoderma.2018.05.035Modeling the joint influence of multiple synoptic-scale, climate mode indices on Australian wheat yield using a vine copula-based approach
Nguyen-Huy, Thong, Deo, Ravinesh C., Mushtaq, Shahbaz, An-Vo, Duc-Anh and Khan, Shahjahan. 2018. "Modeling the joint influence of multiple synoptic-scale, climate mode indices on Australian wheat yield using a vine copula-based approach." European Journal of Agronomy. 98, pp. 65-81. https://doi.org/10.1016/j.eja.2018.05.006Non-tuned data intelligent model for soil temperature estimation: a new approach
Sanikhani, Hadi, Deo, Ravinesh C., Yaseen, Zaher Mundheer, Eray, Okan and Kisi, Ozgur. 2018. "Non-tuned data intelligent model for soil temperature estimation: a new approach." Geoderma. 330, pp. 52-64. https://doi.org/10.1016/j.geoderma.2018.05.030Drought prediction with standardized precipitation and evapotranspiration index and support vector regression models
Deo, Ravinesh C., Salcedo-sanz, Sancho, Carro-Calvo, Leopoldo and Saavedra-Moreno, Beatriz. 2018. "Drought prediction with standardized precipitation and evapotranspiration index and support vector regression models." Samui, Pijush, Kim, Dookie and Ghosh, Chandan (ed.) Integrating disaster science and management. Amsterdam, Netherlands. Elsevier. pp. 151-174Drought modelling based on artificial intelligence and neural network algorithms: a case study in Queensland, Australia
Dayal, Kavina, Deo, Ravinesh and Apan, Armando A.. 2017. "Drought modelling based on artificial intelligence and neural network algorithms: a case study in Queensland, Australia." Filho, Walter Leal (ed.) Climate change adaptation in Pacific countries:fostering resilience and improving the quality of life. Springer. pp. 177-198The inverse relationship between the incidence of solar induced eye disease and reported skin cancer rates at high elevation in the tropical Andes, Ecuador, South America
Downs, Nathan J., Garzon Chavez, Daniel R., Quentin, Emmanuelle, Harrison, Simone L., Parisi, Alfio V. and Butler, Harry. 2018. "The inverse relationship between the incidence of solar induced eye disease and reported skin cancer rates at high elevation in the tropical Andes, Ecuador, South America." 4th International Conference on UV & Skin Cancer Prevention 2018. Toronto, Canada 01 - 04 May 2018 Canada.The Garment Protection Factor (GPF): an index for sun-protective clothing that combines garment coverage and UVR transmittance
Harrison, Simone L., Downs, Nathan J., Rawlings, Alex T. and Parisi, Alfio V.. 2018. "The Garment Protection Factor (GPF): an index for sun-protective clothing that combines garment coverage and UVR transmittance." 4th International Conference on UV & Skin Cancer Prevention 2018. Toronto, Canada 01 - 04 May 2018 Canada.Input selection and data-driven model performance optimization to predict the Standardized Precipitation and Evaporation Index in a drought-prone region
Mouatadid, Soukayna, Raj, Nawin, Deo, Ravinesh C. and Adamowski, Jan F.. 2018. "Input selection and data-driven model performance optimization to predict the Standardized Precipitation and Evaporation Index in a drought-prone region." Atmospheric Research. 212, pp. 130-149. https://doi.org/10.1016/j.atmosres.2018.05.012Re-imagining standard timescales in forecasting precipitation events for Queensland’s grazing enterprises
McCarthy, E., Deo, R. C., Li, Y. and Maraseni, T.. 2017. "Re-imagining standard timescales in forecasting precipitation events for Queensland’s grazing enterprises." Syme, G., Hatton MacDonald, D., Fulton, B. and Piantadosi, J. (ed.) 22nd International Congress on Modelling and Simulation (MODSIM2017). Hobart, Australia 03 - 08 Dec 2017 Australia. Modelling and Simulation Society of Australia and New Zealand . https://doi.org/10.36334/modsim.2017.L1.mccarthySelf-adaptive differential evolutionary extreme learning machines for long-term solar radiation prediction with remotely-sensed MODIS satellite and Reanalysis atmospheric products in solar-rich cities
Ghimire, Sujan, Deo, Ravinesh C., Downs, Nathan J. and Raj, Nawin. 2018. "Self-adaptive differential evolutionary extreme learning machines for long-term solar radiation prediction with remotely-sensed MODIS satellite and Reanalysis atmospheric products in solar-rich cities." Remote Sensing of Environment: an interdisciplinary journal. 212, pp. 176-198. https://doi.org/10.1016/j.rse.2018.05.003An ensemble-ANFIS based uncertainty assessment model for forecasting multi-scalar standardized precipitation index
Ali, Mumtaz, Deo, Ravinesh C., Downs, Nathan J. and Maraseni, Tek. 2018. "An ensemble-ANFIS based uncertainty assessment model for forecasting multi-scalar standardized precipitation index." Atmospheric Research. 207, pp. 155-180. https://doi.org/10.1016/j.atmosres.2018.02.024Wavelet analysis–artificial neural network conjunction models for multi-scale monthly groundwater level predicting in an arid inland river basin, northwestern China
Wen, Xiaohu, Feng, Qi, Deo, Ravinesh C., Wu, Min and Si, Jianhua. 2017. "Wavelet analysis–artificial neural network conjunction models for multi-scale monthly groundwater level predicting in an arid inland river basin, northwestern China." Hydrology Research: an international journal. 48 (6), pp. 1710-1729. https://doi.org/10.2166/nh.2016.396Ensemble committee-based data intelligent approach for generating soil moisture forecasts with multivariate hydro-meteorological predictors
Prasad, Ramendra, Deo, Ravinesh C., Li, Yan and Maraseni, Tek. 2018. "Ensemble committee-based data intelligent approach for generating soil moisture forecasts with multivariate hydro-meteorological predictors." Soil and Tillage Research. 181, pp. 63-81. https://doi.org/10.1016/j.still.2018.03.021Comparison of social-ecological resilience between two grassland management patterns driven by grassland land contract policy in the Maqu, Qinghai-Tibetan Plateau
Cao, Jianjun, Li, Mengtian, Deo, Ravinesh C., Adamowski, Jan F, Cerda, Artemi, Feng, Qi, Liu, Minxia, Zhang, Jian, Zhu, Guofeng, Zhang, Xuebin, Xu, Xueyun, Yang, Shurong and Gong, Yifan. 2018. "Comparison of social-ecological resilience between two grassland management patterns driven by grassland land contract policy in the Maqu, Qinghai-Tibetan Plateau." Land Use Policy: the international journal covering all aspects of land use. 74, pp. 88-96. https://doi.org/10.1016/j.landusepol.2017.07.027Spatio-temporal drought risk mapping approach and its application in the drought-prone region of south-east Queensland, Australia
Dayal, Kavina S., Deo, Ravinesh C. and Apan, Armando A.. 2018. "Spatio-temporal drought risk mapping approach and its application in the drought-prone region of south-east Queensland, Australia." Natural Hazards. 93 (2), pp. 823-847. https://doi.org/10.1007/s11069-018-3326-8A new approach to predict daily pH in rivers based on the 'a trous' redundant wavelet transform algorithm
Rajaee, Taher, Ravansalar, Masoud, Adamowski, Jan F. and Deo, Ravinesh C.. 2018. "A new approach to predict daily pH in rivers based on the 'a trous' redundant wavelet transform algorithm." Water, Air and Soil Pollution: an international journal of environmental pollution. 229 (3). https://doi.org/10.1007/s11270-018-3715-3Computational intelligence approaches for energy load forecasting in smart energy management grids: state of the art, future challenges, and research directions and Research Directions
Fallah, Seyedeh Narjes, Deo, Ravinesh Chand, Shojafar, Mohammad, Conti, Mauro and Shamshirband, Shahaboddin. 2018. "Computational intelligence approaches for energy load forecasting in smart energy management grids: state of the art, future challenges, and research directions and Research Directions." Energies. 11 (3). https://doi.org/10.3390/en11030596Two-phase particle swarm optimized-support vector regression hybrid model integrated with improved empirical mode decomposition with adaptive noise for multiple-horizon electricity demand forecasting
Al-Musaylh, Mohanad S., Deo, Ravinesh C., Li, Yan and Adamowski, Jan F.. 2018. "Two-phase particle swarm optimized-support vector regression hybrid model integrated with improved empirical mode decomposition with adaptive noise for multiple-horizon electricity demand forecasting." Applied Energy. 217, pp. 422-439. https://doi.org/10.1016/j.apenergy.2018.02.140Comparative signal to noise ratio as a determinant to select smartphone image sensor colour channels for analysis in the UVB
Igoe, D. P., Parisi, A. V., Downs, N. J., Amar, A. and Turner, J.. 2018. "Comparative signal to noise ratio as a determinant to select smartphone image sensor colour channels for analysis in the UVB." Sensors and Actuators A: Physical. 272, pp. 125-133. https://doi.org/10.1016/j.sna.2018.01.057Atmospheric total ozone column evaluation with a smartphone image sensor
Igoe, Damien P., Parisi, Alfio V., Amar, Abdurazaq, Downs, Nathan J. and Turner, Joanna. 2018. "Atmospheric total ozone column evaluation with a smartphone image sensor." International Journal of Remote Sensing. 39 (9), pp. 2766-2783. https://doi.org/10.1080/01431161.2018.1433895Application of the hybrid artificial neural network coupled with rolling mechanism and grey model algorithms for streamflow forecasting over multiple time horizons
Yaseen, Zaher Mundher, Fu, Minglei, Wang, Chen, Mohtar, Wan Hanna Melini Wan, Deo, Ravinesh C. and El-Shafie, Ahmed. 2018. "Application of the hybrid artificial neural network coupled with rolling mechanism and grey model algorithms for streamflow forecasting over multiple time horizons." Water Resources Management. 32 (5), pp. 1883-1899. https://doi.org/10.1007/s11269-018-1909-5Multi-household grazing management pattern maintains better soil fertility
Cao, Jianjun, Xu, Xueyun, Deo, Ravinesh C., Holden, Nicholas M., Adamowski, Jan F., Gong, Yifan, Feng, Qi, Yang, Shurong, Li, Mengtian, Zhou, Junju, Zhang, Jian and Liu, Minxia. 2018. "Multi-household grazing management pattern maintains better soil fertility." Agronomy for Sustainable Development: sciences des productions vegetales et de l'environnement. 38 (1). https://doi.org/10.1007/s13593-017-0482-2Short-term electricity demand forecasting with MARS, SVR and ARIMA models using aggregated demand data in Queensland, Australia
Al-Musaylh, Mohanad S., Deo, Ravinesh C., Adamowski, Jan F. and Li, Yan. 2018. "Short-term electricity demand forecasting with MARS, SVR and ARIMA models using aggregated demand data in Queensland, Australia." Advanced Engineering Informatics: the science of supporting knowledge-intensive activities. 35 (C), pp. 1-16. https://doi.org/10.1016/j.aei.2017.11.002Mapping groundwater contamination risk of multiple aquifers using multi-model ensemble of machine learning algorithms
Barzegar, Rahim, Moghaddam, Asghar Asghari, Deo, Ravinesh, Fijani, Elham and Tziritis, Evangelos. 2018. "Mapping groundwater contamination risk of multiple aquifers using multi-model ensemble of machine learning algorithms." Science of the Total Environment. 621, pp. 697-712. https://doi.org/10.1016/j.scitotenv.2017.11.185Investigating drought duration-severity-intensity characteristics using the Standardized Precipitation-Evapotranspiration Index: case studies in drought-prone Southeast Queensland
Dayal, Kavina S., Deo, Ravinesh C. and Apan, Armando A.. 2018. "Investigating drought duration-severity-intensity characteristics using the Standardized Precipitation-Evapotranspiration Index: case studies in drought-prone Southeast Queensland." Journal of Hydrologic Engineering. 23 (1), p. 05017029. https://doi.org/10.1061/(ASCE)HE.1943-5584.0001593An efficient neuro-evolutionary hybrid modelling mechanism for the estimation of daily global solar radiation in the Sunshine State of Australia
Salcedo-sanz, Sancho, Deo, Ravinesh C., Cornejo-Bueno, Laura, Camacho-Gomez, Carlos and Ghimire, Sujan. 2018. "An efficient neuro-evolutionary hybrid modelling mechanism for the estimation of daily global solar radiation in the Sunshine State of Australia." Applied Energy. 209, pp. 79-94. https://doi.org/10.1016/j.apenergy.2017.10.076Multi-layer perceptron hybrid model integrated with the firefly optimizer algorithm for windspeed prediction of target site using a limited set of neighboring reference station data
Deo, Ravinesh C., Ghorbani, Mohammad Ali, Samadianfard, Saeed, Maraseni, Tek, Bilgili, Mehmet and Biazar, Mustafa. 2018. "Multi-layer perceptron hybrid model integrated with the firefly optimizer algorithm for windspeed prediction of target site using a limited set of neighboring reference station data." Renewable Energy. 116 (Part A), pp. 309-323. https://doi.org/10.1016/j.renene.2017.09.078A comprehensive approach to evaluating and classifying sun-protective clothing
Downs, N. J. and Harrison, S. L.. 2018. "A comprehensive approach to evaluating and classifying sun-protective clothing." British Journal of Dermatology. 178 (4), pp. 958-964. https://doi.org/10.1111/bjd.15938Identifying separate impacts of climate and land use/cover change on hydrological processes in upper stream of Heihe River, northwest China
Yang, Linshan, Feng, Qi, Yin, Zhenliang, Wen, Xiaohu, Si, Jianhua, Li, Changbin and Deo, Ravinesh C.. 2017. "Identifying separate impacts of climate and land use/cover change on hydrological processes in upper stream of Heihe River, northwest China." Hydrological Processes. 31 (5), pp. 1100-1112. https://doi.org/10.1002/hyp.11098Reservoir inflow forecasting with a modified coactive neuro-fuzzy inference system: a case study for a semi-arid region
Allawi, Mohammed Falah, Jaafar, Othman, Hamzah, Firdaus Mohamad, Mohd, Nuruol Syuhadaa, Deo, Ravinesh C. and El-Shafie, Ahmed. 2018. "Reservoir inflow forecasting with a modified coactive neuro-fuzzy inference system: a case study for a semi-arid region." Theoretical and Applied Climatology. 134 (1-2), pp. 545-563. https://doi.org/10.1007/s00704-017-2292-5Implementation of a hybrid MLP-FFA model for water level prediction of Lake Egirdir, Turkey
Ghorbani, Mohammad Ali, Deo, Ravinesh C., Karimi, Vahid, Yaseen, Zaher Mundher and Terz, Ozlem. 2018. "Implementation of a hybrid MLP-FFA model for water level prediction of Lake Egirdir, Turkey." Stochastic Environmental Research and Risk Assessment. 32 (6), pp. 1683-1697. https://doi.org/10.1007/s00477-017-1474-0Pan evaporation prediction using a hybrid multilayer perceptron-firefly algorithm (MLP-FFA) model: case study in North Iran
Ghorbani, M. A., Deo, Ravinesh C., Yaseen, Zaher Mundher, Kashani, Mahsa H. and Mohammadi, Babak. 2018. "Pan evaporation prediction using a hybrid multilayer perceptron-firefly algorithm (MLP-FFA) model: case study in North Iran." Theoretical and Applied Climatology. 133 (3-4), pp. 1119-1131. https://doi.org/10.1007/s00704-017-2244-0Impact of grassland contract policy on soil organic carbon losses from alpine grassland on the Qinghai–Tibetan Plateau
Cao, J., Gong, Y., Yeh, E. T., Holden, N. M., Adamowski, J. F., Deo, R. C., Liu, M., Zhou, J., Zhang, J., Zhang, S., Sheng, D., Yang, S., Xu, X., Li, M. and Feng, Q.. 2017. "Impact of grassland contract policy on soil organic carbon losses from alpine grassland on the Qinghai–Tibetan Plateau." Soil Use and Management. 33 (4), pp. 633-671. https://doi.org/10.1111/sum.12387Effects of ecological water transport on photosynthesis and chlorophyll fluorescence of Populus euphratica
Zhao, Chun Yan, Si, Jian Hua, Feng, Qi, Yu, Teng Fei, Deo, Ravinesh C. and Luo, Huan. 2018. "Effects of ecological water transport on photosynthesis and chlorophyll fluorescence of Populus euphratica." Water Science and Technology: Water Supply. 18 (5), pp. 1747-1756. https://doi.org/10.2166/ws.2017.236Trend analysis of Water Poverty Index for assessment of water stress and water management polices: a case study in the Hexi Corridor, China
Huang, Shan, Feng, Qi, Lu, Zhixiang, Wen, Xiaohu and Deo, Ravinesh C.. 2017. "Trend analysis of Water Poverty Index for assessment of water stress and water management polices: a case study in the Hexi Corridor, China." Sustainability. 9 (5), pp. 756-772. https://doi.org/10.3390/su9050756Forecasting evaporative loss by least-square support-vector regression and evaluation with genetic programming, Gaussian process, and minimax probability machine regression: case study of Brisbane City
Deo, Ravinesh C. and Samui, Pijush. 2017. "Forecasting evaporative loss by least-square support-vector regression and evaluation with genetic programming, Gaussian process, and minimax probability machine regression: case study of Brisbane City." Journal of Hydrologic Engineering. 22 (6), pp. 1-15. https://doi.org/10.1061/(ASCE)HE.1943-5584.0001506Statistical evaluation of rainfall time series in concurrence with agriculture and water resources of Ken River basin, Central India (1901–2010)
Meshram, Sarita Gajbhiye, Singh, Sudhir Kumar, Meshram, Chandrashekhar, Deo, Ravinesh C. and Ambade, Balram. 2018. "Statistical evaluation of rainfall time series in concurrence with agriculture and water resources of Ken River basin, Central India (1901–2010)." Theoretical and Applied Climatology. 134, pp. 1231-1243. https://doi.org/10.1007/s00704-017-2335-yAn international comparison of rice consumption behaviours and greenhouse gas emissions from rice production
Maraseni, Tek Narayan, Deo, Ravinesh C., Qu, Jiansheng, Gentle, Popular and Neupane, Prem Raj. 2018. "An international comparison of rice consumption behaviours and greenhouse gas emissions from rice production." Journal of Cleaner Production. 172, pp. 2288-2300. https://doi.org/10.1016/j.jclepro.2017.11.182An Inexpensive High-Temporal Resolution Electronic Sun Journal for Monitoring Personal Day to Day Sun Exposure Patterns
Downs, Nathan J., Parisi, Alfio V., Butler, Harry, Rawlings, Alex and Elrahoumi, Raja Salem. 2017. "An Inexpensive High-Temporal Resolution Electronic Sun Journal for Monitoring Personal Day to Day Sun Exposure Patterns." Frontiers in Public Health. 5, pp. 1-9. https://doi.org/10.3389/fpubh.2017.00310Physiological response to salinity stress and tolerance mechanics of Populus euphratica
Zhao, Chun Yan, Si, Jian Hua, Feng, Qi, Deo, Ravinesh C., Yu, Teng Fei and Li, Pei Du. 2017. "Physiological response to salinity stress and tolerance mechanics of Populus euphratica." Environmental Monitoring and Assessment. 189 (11), pp. 533-543. https://doi.org/10.1007/s10661-017-6257-zCarbon dioxide fluxes and their environmental controls in a riparian forest within the hyper-arid region of Northwest China
Ma, Xiaohong, Feng, Qi, Yu, Tengfei, Su, Yonghong and Deo, Ravinesh C.. 2017. "Carbon dioxide fluxes and their environmental controls in a riparian forest within the hyper-arid region of Northwest China." Forests. 8 (10), pp. 1-17. https://doi.org/10.3390/f8100379Comparative study of hybrid-wavelet artificial intelligence models for monthly groundwater depth forecasting in extreme arid regions, Northwest China
Yu, Haijiao, Wen, Xiaohu, Feng, Qi, Deo, Ravinesh C., Si, Jianhua and Wu, Min. 2018. "Comparative study of hybrid-wavelet artificial intelligence models for monthly groundwater depth forecasting in extreme arid regions, Northwest China." Water Resources Management. 32 (1), pp. 301-323. https://doi.org/10.1007/s11269-017-1811-6Predicting compressive strength of lightweight foamed concrete using extreme learning machine model
Yaseen, Zaher Mundher, Deo, Ravinesh C., Hilal, Ameer, Abd, Abbas M., Bueno, Laura Cornejo, Salcedo-sanz, Sancho and Nehdi, Moncef L.. 2018. "Predicting compressive strength of lightweight foamed concrete using extreme learning machine model." Advances in Engineering Software. 115, pp. 112-125. https://doi.org/10.1016/j.advengsoft.2017.09.004Novel approach for streamflow forecasting using a hybrid ANFIS-FFA model
Yaseen, Zaher Mundher, Ebtehaj, Isa, Bonakdari, Hossein, Deo, Ravinesh C., Mehr, Ali Danandeh, Mohtar, Wan Hanna Melini Wan, Diop, Lamine, El-Shafie, Ahmed and Singh, Vijay P.. 2017. "Novel approach for streamflow forecasting using a hybrid ANFIS-FFA model." Journal of Hydrology. 554, pp. 263-276. https://doi.org/10.1016/j.jhydrol.2017.09.007Rainfall Pattern Forecasting Using Novel Hybrid Intelligent Model Based ANFIS-FFA
Yaseen, Zaher Mundher, Ghareb, Mazen Ismaeel, Ebtehaj, Isa, Bonakdari, Hossein, Siddique, Ridwan, Heddam, Sali, Yusif, Ali A. and Deo, Ravinesh. 2018. "Rainfall Pattern Forecasting Using Novel Hybrid Intelligent Model Based ANFIS-FFA." Water Resources Management. 32 (1), pp. 105-122. https://doi.org/10.1007/s11269-017-1797-0Synthetic retrieval of hourly net ecosystem exchange using the neural network model with combined MI and GOCI geostationary sensor datasets and ground-based measurements
Yeom, Jong-Min, Deo, Ravinesh, Chun, Junghwa, Hong, Jinkyu, Kim, Dong-Su, Han, Kyung-Soo and Cho, Jaeil. 2017. "Synthetic retrieval of hourly net ecosystem exchange using the neural network model with combined MI and GOCI geostationary sensor datasets and ground-based measurements." International Journal of Remote Sensing. 38 (23), pp. 7441-7456. https://doi.org/10.1080/01431161.2017.1375573Separation of the Climatic and Land Cover Impacts on the Flow Regime Changes in Two Watersheds of Northeastern Tibetan Plateau
Yang, Linshan, Feng, Qi, Yin, Zhenliang, Deo, Ravinesh C., Wen, Xiaohu, Si, Jianhua and Li, Changbin. 2017. "Separation of the Climatic and Land Cover Impacts on the Flow Regime Changes in Two Watersheds of Northeastern Tibetan Plateau." Advances in Meteorology. 2017, pp. 1-15. https://doi.org/10.1155/2017/6310401Changes in climatic elements in the Pan-Hexi region during 1960–2014 and responses to global climatic changes
Wei, Liu, Feng, Qi and Deo, Ravinesh C.. 2018. "Changes in climatic elements in the Pan-Hexi region during 1960–2014 and responses to global climatic changes." Theoretical and Applied Climatology. 133 (1-2), pp. 405-420. https://doi.org/10.1007/s00704-017-2194-6Mapping heatwave vulnerability in Korea
Kim, Do-Woo, Deo, Ravinesh C., Lee, Jong-Seol and Yeom, Jong-Min. 2017. "Mapping heatwave vulnerability in Korea." Natural Hazards. 89 (1), pp. 35-55. https://doi.org/10.1007/s11069-017-2951-yCopula-statistical precipitation forecasting model in Australia’s agro-ecological zones
Nguyen-Huy, Thong, Deo, Ravinesh C., An-Vo, Duc-Anh, Mushtaq, Shahbaz and Khan, Shahjahan. 2017. "Copula-statistical precipitation forecasting model in Australia’s agro-ecological zones." Agricultural Water Management. 191, pp. 153-172. https://doi.org/10.1016/j.agwat.2017.06.010Input selection and performance optimization of ANN-based streamflow forecasts in the drought-prone Murray Darling Basin region using IIS and MODWT algorithm
Prasad, Ramendra, Deo, Ravinesh C., Li, Yan and Maraseni, Tek. 2017. "Input selection and performance optimization of ANN-based streamflow forecasts in the drought-prone Murray Darling Basin region using IIS and MODWT algorithm." Atmospheric Research. 197, pp. 42-63. https://doi.org/10.1016/j.atmosres.2017.06.014Very short-term reactive forecasting of the solar ultraviolet index using an extreme learning machine integrated with the solar zenith angle
Deo, Ravinesh C., Downs, Nathan, Parisi, Alfio V., Adamowski, Jan F. and Quilty, John M.. 2017. "Very short-term reactive forecasting of the solar ultraviolet index using an extreme learning machine integrated with the solar zenith angle." Environmental Research. 155, pp. 141-166. https://doi.org/10.1016/j.envres.2017.01.035Association between plant species diversity and edaphic factors in the lower reaches of the Heihe River, northwestern China
Zhao, Yu, Feng, Qi, Xi, Haiyang, Li, Huiya, Yang, Huaide and Deo, Ravinesh C.. 2017. "Association between plant species diversity and edaphic factors in the lower reaches of the Heihe River, northwestern China." Chemistry and Ecology. 33 (3), pp. 181-195. https://doi.org/10.1080/02757540.2017.1287904Forecasting long-term global solar radiation with an ANN algorithm coupled with satellite-derived (MODIS) land surface temperature (LST) for regional locations in Queensland
Deo, Ravinesh C. and Sahin, Mehmet. 2017. "Forecasting long-term global solar radiation with an ANN algorithm coupled with satellite-derived (MODIS) land surface temperature (LST) for regional locations in Queensland." Renewable and Sustainable Energy Reviews. 72, pp. 828-848. https://doi.org/10.1016/j.rser.2017.01.114Computational intelligence approach for modeling hydrogen production: a review
Faizollahzadeh, Sina, Najafi, Bahman, Shamshirband, Shahaboddin, Bidgoli, Behrouz Minaei, Deo, Ravinesh Chand and Chau, Kwok-wing. 2018. "Computational intelligence approach for modeling hydrogen production: a review." Engineering Applications of Computational Fluid Mechanics. 12 (1), pp. 438-458. https://doi.org/10.1080/19942060.2018.1452296Stream-flow forecasting using extreme learning machines: a case study in a semi-arid region in Iraq
Yaseen, Zaher Mundher, Jaafar, Othman, Deo, Ravinesh C., Kisi, Ozgur, Adamowski, Jan, Quilty, John and El-Shafie, Ahmed. 2016. "Stream-flow forecasting using extreme learning machines: a case study in a semi-arid region in Iraq." Journal of Hydrology. 542, pp. 603-614. https://doi.org/10.1016/j.jhydrol.2016.09.035Quantitative definition and spatiotemporal distribution of little water season (LIWAS) in Korea
Kim, Su-Jeong, Byun, Hi-Ryong and Deo, Ravinesh C.. 2016. "Quantitative definition and spatiotemporal distribution of little water season (LIWAS) in Korea." Asia-Pacific Journal of Atmospheric Sciences. 52 (4), pp. 379-393. https://doi.org/10.1007/s13143-016-0012-1Validation of ozone monitoring instrument UV satellite data using spectral and broadband surface based measurements at a Queensland site
A Jebar, Mustapha A., Parisi, Alfio V., Downs, Nathan J. and Turner, Joanna F.. 2017. "Validation of ozone monitoring instrument UV satellite data using spectral and broadband surface based measurements at a Queensland site." Photochemistry and Photobiology. 93 (5), pp. 1289-1293. https://doi.org/10.1111/php.12784Solar ultraviolet and the occupational radiant exposure of Queensland school teachers: a comparative study between teaching classifications and behavior patterns
Downs, Nathan J., Harrison, Simone L., Garzon Chavez, Daniel R. and Parisi, Alfio V.. 2016. "Solar ultraviolet and the occupational radiant exposure of Queensland school teachers: a comparative study between teaching classifications and behavior patterns." Journal of Photochemistry and Photobiology, B: Biology. 158, pp. 105-112. https://doi.org/10.1016/j.jphotobiol.2016.02.018Dual calibrated dosimeter for simultaneous measurements of erythemal and vitamin D effective solar ultraviolet radiation
Wainwright, L., Parisi, A. V. and Downs, N.. 2016. "Dual calibrated dosimeter for simultaneous measurements of erythemal and vitamin D effective solar ultraviolet radiation." Journal of Photochemistry and Photobiology, B: Biology. 157, pp. 15-21. https://doi.org/10.1016/j.jphotobiol.2016.02.003An extreme learning machine model for the simulation of monthly mean streamflow water level in eastern Queensland
Deo, Ravinesh C. and Sahin, Mehmet. 2016. "An extreme learning machine model for the simulation of monthly mean streamflow water level in eastern Queensland." Environmental Monitoring and Assessment. 188 (90). https://doi.org/10.1007/s10661-016-5094-9Forecasting effective drought index using a wavelet extreme learning machine (W-ELM) model
Deo, Ravinesh C., Tiwari, Mukesh K., Adamowski, Jan F. and Quilty, John M.. 2017. "Forecasting effective drought index using a wavelet extreme learning machine (W-ELM) model." Stochastic Environmental Research and Risk Assessment. 31 (5), pp. 1211-1240. https://doi.org/10.1007/s00477-016-1265-zDrought forecasting in eastern Australia using multivariate adaptive regression spline, least square support vector machine and M5Tree model
Deo, Ravinesh C., Kisi, Ogzur and Singh, Vijay P.. 2017. "Drought forecasting in eastern Australia using multivariate adaptive regression spline, least square support vector machine and M5Tree model." Atmospheric Research. 184, pp. 149-175. https://doi.org/10.1016/j.atmosres.2016.10.004A wavelet-coupled support vector machine model for forecasting global incident solar radiation using limited meteorological dataset
Deo, Ravinesh C., Wen, Xiaohu and Feng, Qi. 2016. "A wavelet-coupled support vector machine model for forecasting global incident solar radiation using limited meteorological dataset." Applied Energy. 168, pp. 568-593. https://doi.org/10.1016/j.apenergy.2016.01.130Concurrent evaluation of personal damaging and beneficial UV exposures over an extended period
Wainwright, L. K., Parisi, A. V. and Downs, N. J.. 2017. "Concurrent evaluation of personal damaging and beneficial UV exposures over an extended period." Journal of Photochemistry and Photobiology, B: Biology. 170, pp. 188-196. https://doi.org/10.1016/j.jphotobiol.2017.04.013Comparison of GOME-2 UVA satellite data to ground-based spectroradiometer measurements at a subtropical site
Parisi, Alfio V., Downs, Nathan, Turner, Joanna and King, Rachel. 2017. "Comparison of GOME-2 UVA satellite data to ground-based spectroradiometer measurements at a subtropical site." IEEE Transactions on Geoscience and Remote Sensing. 55 (6), pp. 3145-3149. https://doi.org/10.1109/TGRS.2017.2662714Educating the younger generation (and the public) in UV effects with a simple experiment
Turner, Joanna, Parisi, Alfio V. and Downs, Nathan. 2013. "Educating the younger generation (and the public) in UV effects with a simple experiment." Byrne, Scott and Chen, Min (ed.) 6th Asia & Oceania Conference on Photobiology (AOCP 2013): Life on a Sun-Drenched Planet. Sydney, Australia 10 - 13 Nov 2013In-situ measurements of solar exposure distribution on a coral reef: preliminary findings relating to reef aspect, water turbidity and dosimeter design
Downs, N. J., Schouten, P. W. and Parisi, A. V.. 2016. "In-situ measurements of solar exposure distribution on a coral reef: preliminary findings relating to reef aspect, water turbidity and dosimeter design." 13th International Coral Reef Symposium. Honolulu, Hawaii 19 - 24 Jun 2016Application of hybrid artificial neural network algorithm for the prediction of Standardized Precipitation Index
Dayal, Kavina S., Deo, Ravinesh C. and Apan, Armando A.. 2016. "Application of hybrid artificial neural network algorithm for the prediction of Standardized Precipitation Index." 2016 IEEE Region 10 International Conference: Technologies for Smart Nation (TENCON 2016). Singapore 22 - 25 Nov 2016 Singapore. https://doi.org/10.1109/TENCON.2016.7848588Solar ultraviolet attenuation during the Australian (Red Dawn) dust event of 23 September 2009
Downs, Nathan, Butler, Harry and Parisi, Alfio. 2016. "Solar ultraviolet attenuation during the Australian (Red Dawn) dust event of 23 September 2009." Bulletin of the American Meteorological Society. 97 (11), pp. 2039-2050. https://doi.org/10.1175/BAMS-D-15-00053.1Characterization of cloud cover with a smartphone camera
Parisi, A. V., Downs, N., Igoe, D. and Turner, J.. 2016. "Characterization of cloud cover with a smartphone camera." Instrumentation Science and Technology. 44 (1), pp. 23-34. https://doi.org/10.1080/10739149.2015.1055577Solar radiation and the UV index: an application of numerical integration, trigonometric functions, online education and the modelling process
Downs, Nathan, Parisi, Alfio, Galligan, Linda, Turner, Joanna, Amar, Abdurazaq, King, Rachel, Ultra, Filipina and Butler, Harry. 2016. "Solar radiation and the UV index: an application of numerical integration, trigonometric functions, online education and the modelling process." International Journal of Research in Education and Science. 2 (1), pp. 179-189.Objective Analysis of Marker Bias in Higher Education
Chakraborty, Subrata, Zhou, Susan, Hafeez-Baig, Abdul, Gururajan, Raj, Paul, Manoranjan, Mandal, Anuradha, Chacko, Anila Elizabeth and Barua, Prabal D.. 2017. "Objective Analysis of Marker Bias in Higher Education." 5th IEEE International Conference on Teaching, Assessment, and Learning for Engineering (TALE 2016). Bangkok, Thailand 07 - 09 Dec 2016 Piscataway, United States. https://doi.org/10.1109/TALE.2016.7851839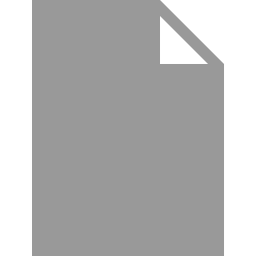