Hamlet-Pattern-Based Automated COVID-19 and Influenza Detection Model Using Protein Sequences
Article
Article Title | Hamlet-Pattern-Based Automated COVID-19 and Influenza Detection Model Using Protein Sequences |
---|---|
ERA Journal ID | 212275 |
Article Category | Article |
Authors | Erten, Mehmet, Acharya, Madhav R., Kamath, Aditya P., Sampathila, Niranjana, Bairy, G. Muralidhar, Aydemir, Emrah, Barua, Prabal Datta, Baygin, Mehmet, Tuncer, Ilknur, Dogan, Sengul and Tuncer, Turker |
Journal Title | Diagnostics |
Journal Citation | 12 (12) |
Article Number | 3181 |
Number of Pages | 15 |
Year | 2022 |
Publisher | MDPI AG |
Place of Publication | Switzerland |
ISSN | 2075-4418 |
Digital Object Identifier (DOI) | https://doi.org/10.3390/diagnostics12123181 |
Web Address (URL) | https://www.mdpi.com/2075-4418/12/12/3181 |
Abstract | SARS-CoV-2 and Influenza-A can present similar symptoms. Computer-aided diagnosis can help facilitate screening for the two conditions, and may be especially relevant and useful in the current COVID-19 pandemic because seasonal Influenza-A infection can still occur. We have developed a novel text-based classification model for discriminating between the two conditions using protein sequences of varying lengths. We downloaded viral protein sequences of SARS-CoV-2 and Influenza-A with varying lengths (all 100 or greater) from the NCBI database and randomly selected 16,901 SARS-CoV-2 and 19,523 Influenza-A sequences to form a two-class study dataset. We used a new feature extraction function based on a unique pattern, HamletPat, generated from the text of Shakespeare’s Hamlet, and a signum function to extract local binary pattern-like bits from overlapping fixed-length (27) blocks of the protein sequences. The bits were converted to decimal map signals from which histograms were extracted and concatenated to form a final feature vector of length 1280. The iterative Chi-square function selected the 340 most discriminative features to feed to an SVM with a Gaussian kernel for classification. The model attained 99.92% and 99.87% classification accuracy rates using hold-out (75:25 split ratio) and five-fold cross-validations, respectively. The excellent performance of the lightweight, handcrafted HamletPat-based classification model suggests that it can be a valuable tool for screening protein sequences to discriminate between SARS-CoV-2 and Influenza-A infections. |
Keywords | bioinformatics; Hamlet Pattern; protein sequence classification; SARS-CoV-2 |
Contains Sensitive Content | Does not contain sensitive content |
ANZSRC Field of Research 2020 | 3206. Medical biotechnology |
Byline Affiliations | Malatya Training and Research Hospital, Turkiye |
Manipal Academy of Higher Education, India | |
Brown University, United States | |
Sakarya University, Turkey | |
School of Business | |
University of Technology Sydney | |
Ardahan University, Turkiye | |
Government office in Elazig, Turkiye | |
Firat University, Turkey |
https://research.usq.edu.au/item/yywq4/hamlet-pattern-based-automated-covid-19-and-influenza-detection-model-using-protein-sequences
Download files
109
total views34
total downloads6
views this month2
downloads this month
Export as
Related outputs
Fibromyalgia Detection and Diagnosis: A Systematic Review of Data-Driven Approaches and Clinical Implications
Atmakuru, Anirudh, Chakraborty, Subrata, Salvi, Massimo, Faust, Oliver, Barua, Prabal Datta, Kobayashi, Makiko, Tan, Ru San, Molinari, Filippo, Hafeez-Baig, Abdul and Acharya, U. Rajendra. 2025. "Fibromyalgia Detection and Diagnosis: A Systematic Review of Data-Driven Approaches and Clinical Implications." IEEE Access. 13, pp. 25026-25044. https://doi.org/10.1109/ACCESS.2025.3539196Zipper Pattern: An Investigation into Psychotic Criminal Detection Using EEG Signals
Tasci, Gulay, Barua, Prabal Datta, Tanko, Dahiru, Keles, Tugce, Tas, Suat, Tuncer, Ilknur, Kaya, Suheda, Yildirim, Kubra, Talu, Yunus, Tasci, Burak, Ozsoy, Filiz, Gonen, Nida, Tasci, Irem, Dogan, Sengul and Tuncer, Turker. 2025. "Zipper Pattern: An Investigation into Psychotic Criminal Detection Using EEG Signals." Diagnostics. 15 (2). https://doi.org/10.3390/diagnostics15020154FlexiCombFE: A flexible, combination-based feature engineering framework for brain tumor detection
Tuncer, Ilknur, Hafeez-Baig, Abdul, Barua, Prabal Datta, Hajiyeva, Rena, Massimo, Salvi, Dogan, Sengul, Tuncer, Turker and Acharya, U.R.. 2025. "FlexiCombFE: A flexible, combination-based feature engineering framework for brain tumor detection." Biomedical Signal Processing and Control. 104. https://doi.org/10.1016/j.bspc.2025.107538EEGConvNeXt: A novel convolutional neural network model for automated detection of Alzheimer's Disease and Frontotemporal Dementia using EEG signals
Acharya, Madhav, Deo, Ravinesh C, Barua, Prabal Datta, Devi, Aruna and Tao, Xiaohui. 2025. "EEGConvNeXt: A novel convolutional neural network model for automated detection of Alzheimer's Disease and Frontotemporal Dementia using EEG signals." Computer Methods and Programs in Biomedicine. 262. https://doi.org/10.1016/j.cmpb.2025.108652Application of transfer learning for biomedical signals: A comprehensive review of the last decade (2014–2024)
Jafari, Mahboobeh, Tao, Xiaohui, Barua, Prabal, Tan, Ru-San and Acharya, U.Rajendra. 2025. "Application of transfer learning for biomedical signals: A comprehensive review of the last decade (2014–2024)." Information Fusion. 118. https://doi.org/10.1016/j.inffus.2025.102982BrainNeXt: novel lightweight CNN model for the automated detection of brain disorders using MRI images
Poyraz, Melahat, Poyraz, Ahmet Kursad, Dogan, Yusuf, Gunes, Selva, Mir, Hasan S., Paul, Jose Kunnel, Barua, Prabal Datta, Baygin, Mehmet, Dogan, Sengul, Tuncer, Turker, Molinari, Filippo and Acharya, Rajendra. 2025. "BrainNeXt: novel lightweight CNN model for the automated detection of brain disorders using MRI images." Cognitive Neurodynamics. 19 (1). https://doi.org/10.1007/s11571-025-10235-zMobileTransNeXt: Integrating CNN, transformer, and BiLSTM for image classification
Ye, Peishun, Lin, Jiyan, Kang, Yaming, Kaya, Tolga, Yildirim, Kubra, Hafeez Baig, Abdul, Aydemir, Emrah, Dogan, Sengul and Tuncer, Turker. 2025. "MobileTransNeXt: Integrating CNN, transformer, and BiLSTM for image classification." Alexandria Engineering Journal. 123, pp. 460-470. https://doi.org/10.1016/j.aej.2025.03.048Application of artificial intelligence in air pollution monitoring and forecasting: A systematic review
Chadalavada, Sreeni, Faust, Oliver, Salvi, Massimo, Seoni, Silvia, Raj, Nawin, Raghavendra, U., Gudigar, Anjan, Barua, Prabal Datta, Molinari, Filippo and Acharya, Rajendra. 2025. "Application of artificial intelligence in air pollution monitoring and forecasting: A systematic review." Environmental Modelling and Software. 185. https://doi.org/10.1016/j.envsoft.2024.106312Half-hourly electricity price prediction model with explainable-decomposition hybrid deep learning approach
Ghimire, Sujan, Deo, Ravinesh C., Hopf, Konstantin, Liu, Hangyue, Casillas-Perez, David, Helwig, Andreas, Prasad, Salvin S., Perez-Aracil, Jorge, Barua, Prabal Datta and Salcedo-Sanz, Sancho. 2025. "Half-hourly electricity price prediction model with explainable-decomposition hybrid deep learning approach." Energy and AI. 20. https://doi.org/10.1016/j.egyai.2025.100492CubicPat: Investigations on the Mental Performance and Stress Detection Using EEG Signals
Ince, Ugur, Talu, Yunus, Duz, Aleyna, Tas, Suat, Tanko, Dahiru, Tasci, Irem, Dogan, Sengul, Hafeez-Baig, Abdul, Aydemir, Emrah and Tuncer, Turker. 2025. "CubicPat: Investigations on the Mental Performance and Stress Detection Using EEG Signals." Diagnostics. 15 (3). https://doi.org/10.3390/diagnostics15030363Automated hip dysplasia detection using novel FlexiLBPHOG model with ultrasound images
Key, Sefa, Kurum, Huseyin, Esmez, Omer, Hafeez-Baig, Abdul, Hajiyeva, Rena, Dogan, Sengul and Tuncer, Turker. 2025. "Automated hip dysplasia detection using novel FlexiLBPHOG model with ultrasound images." Ain Shams Engineering Journal. 16 (1). https://doi.org/10.1016/j.asej.2024.103235Artificial Intelligence-Based Suicide Prevention and Prediction: A Systematic Review (2019-2023)
Atmakuru, Anirudh, Shahini, Alen, Chakraborty, Subrata, Seoni, Silvia, Salvi, Massimo, Hafeez-Baig, Abdul, Rashid, Sadaf, Tan, Ru San, Barua, Prabal Datta, Molinari, Filippo and Acharya, U Rajendra. 2025. "Artificial Intelligence-Based Suicide Prevention and Prediction: A Systematic Review (2019-2023)." Information Fusion. 114. https://doi.org/10.1016/j.inffus.2024.102673AttentionPoolMobileNeXt: An automated construction damage detection model based on a new convolutional neural network and deep feature engineering models
Aydin, Mehmet, Barua, Prabal Datta, Chadalavada, Sreenivasulu, Dogan, Sengul, Tuncer, Turker, Chakraborty, Subrata and Acharya, Rajendra U.. 2025. "AttentionPoolMobileNeXt: An automated construction damage detection model based on a new convolutional neural network and deep feature engineering models." Multimedia Tools and Applications. 84 (4), pp. 1821-1843. https://doi.org/10.1007/s11042-024-19163-2Directed Lobish-based explainable feature engineering model with TTPat and CWINCA for EEG artifact classification
Tuncer, Turker, Dogan, Sengul, Baygin, Mehmet, Tasci, Irem, Mungen, Bulent, Tasci, Burak, Barua, Prabal Datta and Acharya, U.R.. 2024. "Directed Lobish-based explainable feature engineering model with TTPat and CWINCA for EEG artifact classification." Knowledge-Based Systems. 305. https://doi.org/10.1016/j.knosys.2024.112555Retinal Health Screening Using Artificial Intelligence with Digital Fundus Images: A Review of the Last Decade (2012-2023)
Islam, Saad, Deo, Ravinesh C., Barua, Prabal Datta, Soar, Jeffrey, Yu, Ping and Acharya, U. Rajendra. 2024. "Retinal Health Screening Using Artificial Intelligence with Digital Fundus Images: A Review of the Last Decade (2012-2023)." IEEE Access. 12, pp. 176630-176685. https://doi.org/10.1109/ACCESS.2024.3477420Automated EEG-based language detection using directed quantum pattern technique
Dogan, Sengul, Tuncer, Turker, Barua, Prabal Datta and Acharya, U.R.. 2024. "Automated EEG-based language detection using directed quantum pattern technique." Applied Soft Computing. 167 (Part A). https://doi.org/10.1016/j.asoc.2024.112301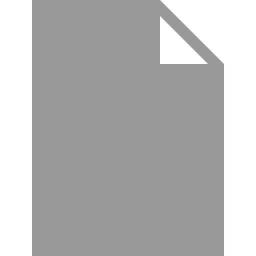