GCLP: An automated asthma detection model based on global chaotic logistic pattern using cough sounds
Article
Kilic, Mehmet, Barua, Prabal Datta, Keles, Tugce, Yildiz, Arif Metehan, Tuncer, Ilknur, Dogan, Sengul, Baygin, Mehmet, Tuncer, Turker, Kuluozturk, Mutlu, Tan, Ru-San and Acharya, U. Rajendra. 2024. "GCLP: An automated asthma detection model based on global chaotic logistic pattern using cough sounds." Engineering Applications of Artificial Intelligence. 127 (Part A). https://doi.org/10.1016/j.engappai.2023.107184
Article Title | GCLP: An automated asthma detection model based on global chaotic logistic pattern using cough sounds |
---|---|
ERA Journal ID | 32032 |
Article Category | Article |
Authors | Kilic, Mehmet, Barua, Prabal Datta, Keles, Tugce, Yildiz, Arif Metehan, Tuncer, Ilknur, Dogan, Sengul, Baygin, Mehmet, Tuncer, Turker, Kuluozturk, Mutlu, Tan, Ru-San and Acharya, U. Rajendra |
Journal Title | Engineering Applications of Artificial Intelligence |
Journal Citation | 127 (Part A) |
Article Number | 107184 |
Number of Pages | 13 |
Year | 2024 |
Publisher | Elsevier |
Place of Publication | United Kingdom |
ISSN | 0952-1976 |
1873-6769 | |
Digital Object Identifier (DOI) | https://doi.org/10.1016/j.engappai.2023.107184 |
Web Address (URL) | https://www.sciencedirect.com/science/article/pii/S0952197623013684 |
Abstract | Asthmatic patients suffer episodic attacks of breathing difficulty and cough. Cough sounds may be used to distinguish asthma from other lung conditions or normal, especially in the acute period. We aimed to develop a novel architecture using lightweight, handcrafted components that could still extract meaningful features. Cough sounds were recorded using mobile phones from 1428 asthmatic and healthy subjects. As cough sounds behave similarly to random noises, we used a global chaotic logistic pattern (GCLP) as a kernel for feature generation based on its ability to detect random relations among input signal values. Further, each cough sound was preprocessed to remove speech and silent periods before undergoing signal decomposition using tunable Q wavelet transformation (TQWT). The latter enabled downstream GCLP-based feature generation at multiple levels, which yielded four final feature vectors (which corresponded to the four types of TQWT-generated wavelet subbands), each of length 3584. Four feature selectors were applied to the latter, generating 16 selected feature vectors, each of length 256. A standard shallow cubic support vector machine was deployed to calculate 16 prediction vectors, which were collectively input to a mode-based iterative hard majority voting function to generate additional 15-voted results. Finally, a greedy algorithm automatically chose the best overall result, which made the model self-organized. Our model attained 99.44% classification accuracy for the collected dataset. Furthermore, our model obtained an accuracy of 98.53% with leave-one- subject-out cross-validation (LOSO CV) strategy, which justifies the robustness of the developed model. |
Keywords | Asthma detection; Chaotic feature extraction ; Cough sound classification ; Multiple parameter-based TQWT ; Feature selection |
Contains Sensitive Content | Does not contain sensitive content |
ANZSRC Field of Research 2020 | 420308. Health informatics and information systems |
Public Notes | Files associated with this item cannot be displayed due to copyright restrictions. |
Byline Affiliations | Firat University, Turkey |
School of Business | |
Interior Ministry, Turkiye | |
Erzurum Technical University, Turkey | |
National Heart Centre, Singapore | |
Duke-NUS Medical Centre, Singapore | |
School of Mathematics, Physics and Computing | |
Kumamoto University, Japan |
Permalink -
https://research.usq.edu.au/item/z5v38/gclp-an-automated-asthma-detection-model-based-on-global-chaotic-logistic-pattern-using-cough-sounds
143
total views0
total downloads0
views this month0
downloads this month
Export as
Related outputs
Spectrogram-based Deep Learning Approach for Anomaly Detection from Cough Sounds
Keles, Tugce, Dogan, Sengul, Hafeez-Baig, Abdul and Tuncer, Turker. 2025. "Spectrogram-based Deep Learning Approach for Anomaly Detection from Cough Sounds." International Journal of Information Technology and Computer Science (IJITCS). 17 (3), pp. 1-12. https://doi.org/10.5815/ijitcs.2025.03.01MuRAt-CAP-Net: A novel multi-input residual attention network for automated detection of A-phases and subtypes in cyclic alternating patterns
Yaman, Suleyman, Güler, Hasan, Sengur, Abdulkadir, Hafeez-Baig, Abdul and Acharya, U. Rajendra. 2025. "MuRAt-CAP-Net: A novel multi-input residual attention network for automated detection of A-phases and subtypes in cyclic alternating patterns." Biomedical Signal Processing and Control. 110 (Part A). https://doi.org/10.1016/j.bspc.2025.108221AirQuaNet: A Convolutional Neural Network Model With Multi-Scale Feature Learning and Attention Mechanisms for Air Quality-Based Health Impact Prediction
Chadalavada, Sreeni, Yaman, Suleyman, Sengur, Abdulkadir, Deo, Ravinesh C., Hafeez-Baig, Abdul, Kolbe-Alexander, Tracy, Sampathila, Niranjana and Acharya, U. Rajendra. 2025. "AirQuaNet: A Convolutional Neural Network Model With Multi-Scale Feature Learning and Attention Mechanisms for Air Quality-Based Health Impact Prediction." IEEE Access. 13, pp. 96261-96276. https://doi.org/10.1109/ACCESS.2025.3574722Gated-LNN: Gated Liquid Neural Networks for Accurate Water Quality Index Prediction and Classification
Chadalavada, Sreeni, Yaman, Süleyman, Yaman, Suleyman, Sengur, Abdulkadi, Hafeez-Baig, Abdul, Tan, Ru-San, Barua, Prabal Datta, Deo, Ravinesh C., Kobayashi, Makiko and Acharya, U. Rajendra. 2025. "Gated-LNN: Gated Liquid Neural Networks for Accurate Water Quality Index Prediction and Classification." IEEE Access. 13, pp. 69500-69512. https://doi.org/10.1109/ACCESS.2025.3561593Artificial intelligence for early detection of diabetes mellitus complications via retinal imaging
Sobhi, Navid, SaDeghi-Bazargani, Y., Mirzaei, M., Abdollahi, M., Jafarizadeh, A., Pedrammehr, S., Alizadehsani, R., Tan, R., Islam, S. and Acharya, U. Rajendra. 2025. "Artificial intelligence for early detection of diabetes mellitus complications via retinal imaging." Journal of Diabetes and Metabolic Disorders. 24. https://doi.org/10.1007/s40200-025-01596-7MobileTurkerNeXt: investigating the detection of Bankart and SLAP lesions using magnetic resonance images
Gurger, Murat, Esmez, Omer, Key, Sefa, Hafeez-Baig, Abdul, Dogan, Sengul and Tuncer, Turker. 2025. "MobileTurkerNeXt: investigating the detection of Bankart and SLAP lesions using magnetic resonance images." Radiological Physics and Technology. https://doi.org/10.1007/s12194-025-00918-xStrokeNeXt: an automated stroke classification model using computed tomography and magnetic resonance images
Ekingen, Evren, Yildirim, Ferhat, Bayar, Ozgur, Akbal, Erhan, Sercek, Ilknur, Hafeez-Baig, Abdul, Dogan, Sengul and Tuncer, Turker. 2025. "StrokeNeXt: an automated stroke classification model using computed tomography and magnetic resonance images." BMC Medical Imaging. 25 (1). https://doi.org/10.1186/s12880-025-01721-1Fibromyalgia Detection and Diagnosis: A Systematic Review of Data-Driven Approaches and Clinical Implications
Atmakuru, Anirudh, Chakraborty, Subrata, Salvi, Massimo, Faust, Oliver, Barua, Prabal Datta, Kobayashi, Makiko, Tan, Ru San, Molinari, Filippo, Hafeez-Baig, Abdul and Acharya, U. Rajendra. 2025. "Fibromyalgia Detection and Diagnosis: A Systematic Review of Data-Driven Approaches and Clinical Implications." IEEE Access. 13, pp. 25026-25044. https://doi.org/10.1109/ACCESS.2025.3539196Current and future roles of artificial intelligence in retinopathy of prematurity
Jafarizadeh, Ali, Maleki, Shadi Farabi, Pouya, Parnia, Sobhi, Navid, Abdollahi, Mirsaeed, Pedrammehr, Siamak, Lim, Chee Peng, Asadi, Houshyar, Alizadehsani, Roohallah, Tan, Ru-San, Islam, Sheikh Mohammed Shariful and Acharya, U. Rajendra. 2025. "Current and future roles of artificial intelligence in retinopathy of prematurity." Artificial Intelligence Review. 58 (6). https://doi.org/10.1007/s10462-025-11153-6BAIoT-EMS: Consortium network for small-medium enterprises management system with blockchain and augmented intelligence of things
Khan, Abdullah Ayub, Yang, Jing, Laghari, Asif Ali, Baqasah, Abdullah M., Alroobaea, Roobaea, Ku, Chin Soon, Alizadehsani, Roohallah, Acharya, U. Rajendra and Por, Lip Yee. 2025. "BAIoT-EMS: Consortium network for small-medium enterprises management system with blockchain and augmented intelligence of things." Engineering Applications of Artificial Intelligence. 141. https://doi.org/10.1016/j.engappai.2024.109838Artificial Intelligence for Ovarian Cancer Detection with Medical Images: A Review of the Last Decade (2013–2023)
Naderi Yaghouti, Amir Reza, Shalbaf, Ahmad, Alizadehsani, Roohallah, Tan, Ru-San, Vijayananthan, Anushya, Yeong, Chai Hong and Acharya, U. Rajendra. 2025. "Artificial Intelligence for Ovarian Cancer Detection with Medical Images: A Review of the Last Decade (2013–2023)." Archives of Computational Methods in Engineering. https://doi.org/10.1007/s11831-025-10268-xZipper Pattern: An Investigation into Psychotic Criminal Detection Using EEG Signals
Tasci, Gulay, Barua, Prabal Datta, Tanko, Dahiru, Keles, Tugce, Tas, Suat, Tuncer, Ilknur, Kaya, Suheda, Yildirim, Kubra, Talu, Yunus, Tasci, Burak, Ozsoy, Filiz, Gonen, Nida, Tasci, Irem, Dogan, Sengul and Tuncer, Turker. 2025. "Zipper Pattern: An Investigation into Psychotic Criminal Detection Using EEG Signals." Diagnostics. 15 (2). https://doi.org/10.3390/diagnostics15020154FlexiCombFE: A flexible, combination-based feature engineering framework for brain tumor detection
Tuncer, Ilknur, Hafeez-Baig, Abdul, Barua, Prabal Datta, Hajiyeva, Rena, Massimo, Salvi, Dogan, Sengul, Tuncer, Turker and Acharya, U.R.. 2025. "FlexiCombFE: A flexible, combination-based feature engineering framework for brain tumor detection." Biomedical Signal Processing and Control. 104. https://doi.org/10.1016/j.bspc.2025.107538A dual-method approach using autoencoders and transductive learning for remaining useful life estimation
Yang, Jing, Boroojeni, Nika Anoosha, Chahardeh, Mehran Kazemi, Por, Lip Yee, Alizadehsani, Roohallah and Acharya, U. Rajendra. 2025. "A dual-method approach using autoencoders and transductive learning for remaining useful life estimation." Engineering Applications of Artificial Intelligence. 147. https://doi.org/10.1016/j.engappai.2025.110285EEGConvNeXt: A novel convolutional neural network model for automated detection of Alzheimer's Disease and Frontotemporal Dementia using EEG signals
Acharya, Madhav, Deo, Ravinesh C, Barua, Prabal Datta, Devi, Aruna and Tao, Xiaohui. 2025. "EEGConvNeXt: A novel convolutional neural network model for automated detection of Alzheimer's Disease and Frontotemporal Dementia using EEG signals." Computer Methods and Programs in Biomedicine. 262. https://doi.org/10.1016/j.cmpb.2025.108652A Dual-Stream Deep Learning Architecture With Adaptive Random Vector Functional Link for Multi-Center Ischemic Stroke Classification
Inamdar, Mahesh Anil, Gudigar, Anjan, Raghavendra, U., Salvi, Massimo, Aman, Raja Rizal Azman Bin Raja, Muhammad Gowdh, Nadia Fareeda, Ahir, Izzah Amirah Binti Mohd, Bin Kamaruddin, Mohd Salahuddin, Kadir, Khairul Azmi Abdul, Molinari, Filippo, Hegde, Ajay, Menon, Girish R. and Acharya, U. Rajendra. 2025. "A Dual-Stream Deep Learning Architecture With Adaptive Random Vector Functional Link for Multi-Center Ischemic Stroke Classification." IEEE Access. 13, pp. 46638-46658. https://doi.org/10.1109/ACCESS.2025.3550344Application of transfer learning for biomedical signals: A comprehensive review of the last decade (2014–2024)
Jafari, Mahboobeh, Tao, Xiaohui, Barua, Prabal, Tan, Ru-San and Acharya, U.Rajendra. 2025. "Application of transfer learning for biomedical signals: A comprehensive review of the last decade (2014–2024)." Information Fusion. 118. https://doi.org/10.1016/j.inffus.2025.102982BrainNeXt: novel lightweight CNN model for the automated detection of brain disorders using MRI images
Poyraz, Melahat, Poyraz, Ahmet Kursad, Dogan, Yusuf, Gunes, Selva, Mir, Hasan S., Paul, Jose Kunnel, Barua, Prabal Datta, Baygin, Mehmet, Dogan, Sengul, Tuncer, Turker, Molinari, Filippo and Acharya, Rajendra. 2025. "BrainNeXt: novel lightweight CNN model for the automated detection of brain disorders using MRI images." Cognitive Neurodynamics. 19 (1). https://doi.org/10.1007/s11571-025-10235-zA novel uncertainty-aware liquid neural network for noise-resilient time series forecasting and classification
Akpinar, Muhammed Halil, Atila, Orhan, Sengur, Abdulkadir, Salvi, Massimo and Acharya, U.R.. 2025. "A novel uncertainty-aware liquid neural network for noise-resilient time series forecasting and classification." Chaos, Solitons and Fractals. 193. https://doi.org/10.1016/j.chaos.2025.116130A novel complexity reduction technique using visibility relationship and perpendicular distance recursive refinement for physiological signals
Atila, Orhan, Akpinar, Muhammed Halil, Sengur, Abdulkadir and Acharya, U.R.. 2025. "A novel complexity reduction technique using visibility relationship and perpendicular distance recursive refinement for physiological signals." Communications in Nonlinear Science and Numerical Simulation. 145. https://doi.org/10.1016/j.cnsns.2025.108752MobileTransNeXt: Integrating CNN, transformer, and BiLSTM for image classification
Ye, Peishun, Lin, Jiyan, Kang, Yaming, Kaya, Tolga, Yildirim, Kubra, Hafeez Baig, Abdul, Aydemir, Emrah, Dogan, Sengul and Tuncer, Turker. 2025. "MobileTransNeXt: Integrating CNN, transformer, and BiLSTM for image classification." Alexandria Engineering Journal. 123, pp. 460-470. https://doi.org/10.1016/j.aej.2025.03.048Application of artificial intelligence in air pollution monitoring and forecasting: A systematic review
Chadalavada, Sreeni, Faust, Oliver, Salvi, Massimo, Seoni, Silvia, Raj, Nawin, Raghavendra, U., Gudigar, Anjan, Barua, Prabal Datta, Molinari, Filippo and Acharya, Rajendra. 2025. "Application of artificial intelligence in air pollution monitoring and forecasting: A systematic review." Environmental Modelling and Software. 185. https://doi.org/10.1016/j.envsoft.2024.106312Electricity demand uncertainty modeling with Temporal Convolution Neural Network models
Ghimire, Sujan, Deo, Ravinesh C., Casillas-Perez, David, Salcedo-Sanz, Sancho, Acharya, Rajendra and Dinh, Toan. 2025. "Electricity demand uncertainty modeling with Temporal Convolution Neural Network models." Renewable and Sustainable Energy Reviews. 209. https://doi.org/10.1016/j.rser.2024.115097Half-hourly electricity price prediction model with explainable-decomposition hybrid deep learning approach
Ghimire, Sujan, Deo, Ravinesh C., Hopf, Konstantin, Liu, Hangyue, Casillas-Perez, David, Helwig, Andreas, Prasad, Salvin S., Perez-Aracil, Jorge, Barua, Prabal Datta and Salcedo-Sanz, Sancho. 2025. "Half-hourly electricity price prediction model with explainable-decomposition hybrid deep learning approach." Energy and AI. 20. https://doi.org/10.1016/j.egyai.2025.100492Investigating Brain Lobe Biomarkers to Enhance Dementia Detection Using EEG Data
Siuly, Siuly, Tawhid, Md.Nurul Ahad, Li, Yan, Acharya, Rajendra, Sadiq, Muhammad Tariq and Wang, Hua. 2025. "Investigating Brain Lobe Biomarkers to Enhance Dementia Detection Using EEG Data." Cognitive Computation. 17 (2). https://doi.org/10.1007/s12559-025-10447-9Explainable hybrid deep learning framework for enhancing multi-step solar ultraviolet-B radiation predictions
Prasad, Salvin S., Joseph, Lionel P., Ghimire, Sujan, Deo, Ravinesh C., Downs, Nathan J., Acharya, Rajendra and Yaseen, Zaher M.. 2025. "Explainable hybrid deep learning framework for enhancing multi-step solar ultraviolet-B radiation predictions." Atmospheric Environment. 343. https://doi.org/10.1016/j.atmosenv.2024.120951CubicPat: Investigations on the Mental Performance and Stress Detection Using EEG Signals
Ince, Ugur, Talu, Yunus, Duz, Aleyna, Tas, Suat, Tanko, Dahiru, Tasci, Irem, Dogan, Sengul, Hafeez-Baig, Abdul, Aydemir, Emrah and Tuncer, Turker. 2025. "CubicPat: Investigations on the Mental Performance and Stress Detection Using EEG Signals." Diagnostics. 15 (3). https://doi.org/10.3390/diagnostics15030363Preserving privacy in healthcare: A systematic review of deep learning approaches for synthetic data generation
Li, Yintong, Acharya, U. Rajendra and Tan, Jen Hong. 2025. "Preserving privacy in healthcare: A systematic review of deep learning approaches for synthetic data generation." Computer Methods and Programs in Biomedicine. 260. https://doi.org/10.1016/j.cmpb.2024.108571Automated hip dysplasia detection using novel FlexiLBPHOG model with ultrasound images
Key, Sefa, Kurum, Huseyin, Esmez, Omer, Hafeez-Baig, Abdul, Hajiyeva, Rena, Dogan, Sengul and Tuncer, Turker. 2025. "Automated hip dysplasia detection using novel FlexiLBPHOG model with ultrasound images." Ain Shams Engineering Journal. 16 (1). https://doi.org/10.1016/j.asej.2024.103235Artificial Intelligence-Based Suicide Prevention and Prediction: A Systematic Review (2019-2023)
Atmakuru, Anirudh, Shahini, Alen, Chakraborty, Subrata, Seoni, Silvia, Salvi, Massimo, Hafeez-Baig, Abdul, Rashid, Sadaf, Tan, Ru San, Barua, Prabal Datta, Molinari, Filippo and Acharya, U Rajendra. 2025. "Artificial Intelligence-Based Suicide Prevention and Prediction: A Systematic Review (2019-2023)." Information Fusion. 114. https://doi.org/10.1016/j.inffus.2024.102673Explainable deeply-fused nets electricity demand prediction model: Factoring climate predictors for accuracy and deeper insights with probabilistic confidence interval and point-based forecasts
Ghimire, Sujan, AL-Musaylh, Mohanad S., Nguyen-Huy, Thong, Deo, Ravinesh C., Acharya, Rajendra, Casillas-Perez, David, Yaseen, Zaher Mundher and Salcedo-sanz, Sancho. 2025. "Explainable deeply-fused nets electricity demand prediction model: Factoring climate predictors for accuracy and deeper insights with probabilistic confidence interval and point-based forecasts." Applied Energy. 378 (Part A). https://doi.org/10.1016/j.apenergy.2024.124763AttentionPoolMobileNeXt: An automated construction damage detection model based on a new convolutional neural network and deep feature engineering models
Aydin, Mehmet, Barua, Prabal Datta, Chadalavada, Sreenivasulu, Dogan, Sengul, Tuncer, Turker, Chakraborty, Subrata and Acharya, Rajendra U.. 2025. "AttentionPoolMobileNeXt: An automated construction damage detection model based on a new convolutional neural network and deep feature engineering models." Multimedia Tools and Applications. 84 (4), pp. 1821-1843. https://doi.org/10.1007/s11042-024-19163-2Directed Lobish-based explainable feature engineering model with TTPat and CWINCA for EEG artifact classification
Tuncer, Turker, Dogan, Sengul, Baygin, Mehmet, Tasci, Irem, Mungen, Bulent, Tasci, Burak, Barua, Prabal Datta and Acharya, U.R.. 2024. "Directed Lobish-based explainable feature engineering model with TTPat and CWINCA for EEG artifact classification." Knowledge-Based Systems. 305. https://doi.org/10.1016/j.knosys.2024.112555Retinal Health Screening Using Artificial Intelligence with Digital Fundus Images: A Review of the Last Decade (2012-2023)
Islam, Saad, Deo, Ravinesh C., Barua, Prabal Datta, Soar, Jeffrey, Yu, Ping and Acharya, U. Rajendra. 2024. "Retinal Health Screening Using Artificial Intelligence with Digital Fundus Images: A Review of the Last Decade (2012-2023)." IEEE Access. 12, pp. 176630-176685. https://doi.org/10.1109/ACCESS.2024.3477420Automated EEG-based language detection using directed quantum pattern technique
Dogan, Sengul, Tuncer, Turker, Barua, Prabal Datta and Acharya, U.R.. 2024. "Automated EEG-based language detection using directed quantum pattern technique." Applied Soft Computing. 167 (Part A). https://doi.org/10.1016/j.asoc.2024.112301A Novel Hybrid Model for Automatic Non-Small Cell Lung Cancer Classification Using Histopathological Images
Katar, Oguzhan, Yildirim, Ozal, Tan, Ru-San and Acharya, U Rajendra. 2024. "A Novel Hybrid Model for Automatic Non-Small Cell Lung Cancer Classification Using Histopathological Images." Diagnostics. 14 (22). https://doi.org/10.3390/diagnostics14222497Synthetic Data Generation via Generative Adversarial Networks in Healthcare: A Systematic Review of Image- and Signal-Based Studies
Akpinar, Muhammed Halil, Sengur, Abdulkadir, Salvi, Massimo, Seoni, Silvia, Faust, Oliver, Mir, Hasan, Molinari,Filippo and Acharya, U. Rajendra. 2024. "Synthetic Data Generation via Generative Adversarial Networks in Healthcare: A Systematic Review of Image- and Signal-Based Studies." IEEE Open Journal of Engineering in Medicine and Biology. 6, pp. 183-192. https://doi.org/10.1109/OJEMB.2024.3508472RECOMED: A comprehensive pharmaceutical recommendation system
Zomorodi, Mariam, Ghodsollahee, Ismail, Martin, Jennifer H, Talley, Nicholas J, Salari, Vahid, Pławiak, Paweł, Rahimi, Kazem and Acharya, U.R.. 2024. "RECOMED: A comprehensive pharmaceutical recommendation system." Artificial Intelligence in Medicine. 157. https://doi.org/10.1016/j.artmed.2024.102981Artificial intelligence in assessing cardiovascular diseases and risk factors via retinal fundus images: A review of the last decade
Abdollahi, Mirsaeed, Jafarizadeh, Ali, Ghafouri-Asbagh, Amirhosein, Sobhi, Navid, Pourmoghtader, Keysan, Pedrammehr, Siamak, Asadi, Houshyar, Tan, Ru-San, Alizadehsani, Roohallah and Acharya, U. Rajendra. 2024. "Artificial intelligence in assessing cardiovascular diseases and risk factors via retinal fundus images: A review of the last decade." WIREs Data Mining and Knowledge Discovery. 14 (6). https://doi.org/10.1002/widm.1560Early prediction of sudden cardiac death using multimodal fusion of ECG Features extracted from Hilbert–Huang and wavelet transforms with explainable vision transformer and CNN models
Telangore, Hardik, Azad, Victor, Sharma, Manish, Bhurane, Ankit, Tan, Ru San and Acharya, U. Rajendra. 2024. "Early prediction of sudden cardiac death using multimodal fusion of ECG Features extracted from Hilbert–Huang and wavelet transforms with explainable vision transformer and CNN models." Computer Methods and Programs in Biomedicine. 257. https://doi.org/10.1016/j.cmpb.2024.108455A Pragmatic Approach to Fetal Monitoring via Cardiotocography Using Feature Elimination and Hyperparameter Optimization
Hardalac, Firat, Akmal, Haad, Ayturan, Kubilay, Acharya, U. Rajendra and Tan, Ru-San. 2024. "A Pragmatic Approach to Fetal Monitoring via Cardiotocography Using Feature Elimination and Hyperparameter Optimization." Interdisciplinary Sciences: Computational Life Sciences. 16 (4), pp. 882-906. https://doi.org/10.1007/s12539-024-00647-6Automated System for the Detection of Heart Anomalies Using Phonocardiograms: A Systematic Review
Gudigar, Anjan, Raghavendra, U., Maithri, M., Samanth, Jyothi, Inamdar, Mahesh Anil, Vidhya, V., Vicnesh, Jahmunah, Prabhu, Mukund A., Tan, Ru-San, Yeong, Chai Hong, Molinari, Filippo and Acharya, U. R.. 2024. "Automated System for the Detection of Heart Anomalies Using Phonocardiograms: A Systematic Review." IEEE Access. 12, pp. 138399-138428. https://doi.org/10.1109/ACCESS.2024.3465511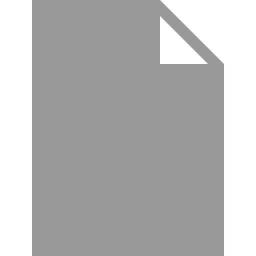