Primate brain pattern-based automated Alzheimer's disease detection model using EEG signals
Article
Dogan, Sengul, Baygin, Mehmet, Tasci, Burak, Loh, Hui Wen, Barua, Prabal D., Tuncer, Turker, Tan, Ru-San and Acharya, U. Rajendra. 2023. "Primate brain pattern-based automated Alzheimer's disease detection model using EEG signals." Cognitive Neurodynamics. 17 (3), pp. 647-659. https://doi.org/10.1007/s11571-022-09859-2
Article Title | Primate brain pattern-based automated Alzheimer's disease detection model using EEG signals |
---|---|
ERA Journal ID | 3179 |
Article Category | Article |
Authors | Dogan, Sengul, Baygin, Mehmet, Tasci, Burak, Loh, Hui Wen, Barua, Prabal D., Tuncer, Turker, Tan, Ru-San and Acharya, U. Rajendra |
Journal Title | Cognitive Neurodynamics |
Journal Citation | 17 (3), pp. 647-659 |
Number of Pages | 13 |
Year | 2023 |
Publisher | Springer |
Place of Publication | Netherlands |
ISSN | 1871-4080 |
1871-4099 | |
Digital Object Identifier (DOI) | https://doi.org/10.1007/s11571-022-09859-2 |
Web Address (URL) | https://link.springer.com/article/10.1007/s11571-022-09859-2 |
Abstract | Electroencephalography (EEG) may detect early changes in Alzheimer's disease (AD), a debilitating progressive neurodegenerative disease. We have developed an automated AD detection model using a novel directed graph for local texture feature extraction with EEG signals. The proposed graph was created from a topological map of the macroscopic connectome, i.e., neuronal pathways linking anatomo-functional brain segments involved in visual object recognition and motor response in the primate brain. This primate brain pattern (PBP)-based model was tested on a public AD EEG signal dataset. The dataset comprised 16-channel EEG signal recordings of 12 AD patients and 11 healthy controls. While PBP could generate 448 low-level features per one-dimensional EEG signal, combining it with tunable q-factor wavelet transform created a multilevel feature extractor (which mimicked deep models) to generate 8,512 (= 448 × 19) features per signal input. Iterative neighborhood component analysis was used to choose the most discriminative features (the number of optimal features varied among the individual EEG channels) to feed to a weighted k-nearest neighbor (KNN) classifier for binary classification into AD vs. healthy using both leave-one subject-out (LOSO) and tenfold cross-validations. Iterative majority voting was used to compute subject-level general performance results from the individual channel classification outputs. Channel-wise, as well as subject-level general results demonstrated exemplary performance. In addition, the model attained 100% and 92.01% accuracy for AD vs. healthy classification using the KNN classifier with tenfold and LOSO cross-validations, respectively. Our developed multilevel PBP-based model extracted discriminative features from EEG signals and paved the way for further development of models inspired by the brain connectome. |
Keywords | AD detection; Primate brain modelling; Feature engineering; Feature extraction; EEG signal classification |
ANZSRC Field of Research 2020 | 400306. Computational physiology |
Public Notes | Files associated with this item cannot be displayed due to copyright restrictions. |
Byline Affiliations | Firat University, Turkey |
Ardahan University, Turkiye | |
Singapore University of Social Sciences (SUSS), Singapore | |
School of Business | |
University of Technology Sydney | |
National Heart Centre, Singapore | |
Duke-NUS Medical School, Singapore | |
Ngee Ann Polytechnic, Singapore | |
Asia University, Taiwan |
Permalink -
https://research.usq.edu.au/item/z1v82/primate-brain-pattern-based-automated-alzheimer-s-disease-detection-model-using-eeg-signals
92
total views0
total downloads5
views this month0
downloads this month
Export as
Related outputs
Fibromyalgia Detection and Diagnosis: A Systematic Review of Data-Driven Approaches and Clinical Implications
Atmakuru, Anirudh, Chakraborty, Subrata, Salvi, Massimo, Faust, Oliver, Barua, Prabal Datta, Kobayashi, Makiko, Tan, Ru San, Molinari, Filippo, Hafeez-Baig, Abdul and Acharya, U. Rajendra. 2025. "Fibromyalgia Detection and Diagnosis: A Systematic Review of Data-Driven Approaches and Clinical Implications." IEEE Access. 13, pp. 25026-25044. https://doi.org/10.1109/ACCESS.2025.3539196Current and future roles of artificial intelligence in retinopathy of prematurity
Jafarizadeh, Ali, Maleki, Shadi Farabi, Pouya, Parnia, Sobhi, Navid, Abdollahi, Mirsaeed, Pedrammehr, Siamak, Lim, Chee Peng, Asadi, Houshyar, Alizadehsani, Roohallah, Tan, Ru-San, Islam, Sheikh Mohammed Shariful and Acharya, U. Rajendra. 2025. "Current and future roles of artificial intelligence in retinopathy of prematurity." Artificial Intelligence Review. 58 (6). https://doi.org/10.1007/s10462-025-11153-6Artificial Intelligence for Ovarian Cancer Detection with Medical Images: A Review of the Last Decade (2013–2023)
Naderi Yaghouti, Amir Reza, Shalbaf, Ahmad, Alizadehsani, Roohallah, Tan, Ru-San, Vijayananthan, Anushya, Yeong, Chai Hong and Acharya, U. Rajendra. 2025. "Artificial Intelligence for Ovarian Cancer Detection with Medical Images: A Review of the Last Decade (2013–2023)." Archives of Computational Methods in Engineering. https://doi.org/10.1007/s11831-025-10268-xZipper Pattern: An Investigation into Psychotic Criminal Detection Using EEG Signals
Tasci, Gulay, Barua, Prabal Datta, Tanko, Dahiru, Keles, Tugce, Tas, Suat, Tuncer, Ilknur, Kaya, Suheda, Yildirim, Kubra, Talu, Yunus, Tasci, Burak, Ozsoy, Filiz, Gonen, Nida, Tasci, Irem, Dogan, Sengul and Tuncer, Turker. 2025. "Zipper Pattern: An Investigation into Psychotic Criminal Detection Using EEG Signals." Diagnostics. 15 (2). https://doi.org/10.3390/diagnostics15020154FlexiCombFE: A flexible, combination-based feature engineering framework for brain tumor detection
Tuncer, Ilknur, Hafeez-Baig, Abdul, Barua, Prabal Datta, Hajiyeva, Rena, Massimo, Salvi, Dogan, Sengul, Tuncer, Turker and Acharya, U.R.. 2025. "FlexiCombFE: A flexible, combination-based feature engineering framework for brain tumor detection." Biomedical Signal Processing and Control. 104. https://doi.org/10.1016/j.bspc.2025.107538EEGConvNeXt: A novel convolutional neural network model for automated detection of Alzheimer's Disease and Frontotemporal Dementia using EEG signals
Acharya, Madhav, Deo, Ravinesh C, Barua, Prabal Datta, Devi, Aruna and Tao, Xiaohui. 2025. "EEGConvNeXt: A novel convolutional neural network model for automated detection of Alzheimer's Disease and Frontotemporal Dementia using EEG signals." Computer Methods and Programs in Biomedicine. 262. https://doi.org/10.1016/j.cmpb.2025.108652Application of transfer learning for biomedical signals: A comprehensive review of the last decade (2014–2024)
Jafari, Mahboobeh, Tao, Xiaohui, Barua, Prabal, Tan, Ru-San and Acharya, U.Rajendra. 2025. "Application of transfer learning for biomedical signals: A comprehensive review of the last decade (2014–2024)." Information Fusion. 118. https://doi.org/10.1016/j.inffus.2025.102982BrainNeXt: novel lightweight CNN model for the automated detection of brain disorders using MRI images
Poyraz, Melahat, Poyraz, Ahmet Kursad, Dogan, Yusuf, Gunes, Selva, Mir, Hasan S., Paul, Jose Kunnel, Barua, Prabal Datta, Baygin, Mehmet, Dogan, Sengul, Tuncer, Turker, Molinari, Filippo and Acharya, Rajendra. 2025. "BrainNeXt: novel lightweight CNN model for the automated detection of brain disorders using MRI images." Cognitive Neurodynamics. 19 (1). https://doi.org/10.1007/s11571-025-10235-zA novel uncertainty-aware liquid neural network for noise-resilient time series forecasting and classification
Akpinar, Muhammed Halil, Atila, Orhan, Sengur, Abdulkadir, Salvi, Massimo and Acharya, U.R.. 2025. "A novel uncertainty-aware liquid neural network for noise-resilient time series forecasting and classification." Chaos, Solitons and Fractals. 193. https://doi.org/10.1016/j.chaos.2025.116130A novel complexity reduction technique using visibility relationship and perpendicular distance recursive refinement for physiological signals
Atila, Orhan, Akpinar, Muhammed Halil, Sengur, Abdulkadir and Acharya, U.R.. 2025. "A novel complexity reduction technique using visibility relationship and perpendicular distance recursive refinement for physiological signals." Communications in Nonlinear Science and Numerical Simulation. 145. https://doi.org/10.1016/j.cnsns.2025.108752MobileTransNeXt: Integrating CNN, transformer, and BiLSTM for image classification
Ye, Peishun, Lin, Jiyan, Kang, Yaming, Kaya, Tolga, Yildirim, Kubra, Hafeez Baig, Abdul, Aydemir, Emrah, Dogan, Sengul and Tuncer, Turker. 2025. "MobileTransNeXt: Integrating CNN, transformer, and BiLSTM for image classification." Alexandria Engineering Journal. 123, pp. 460-470. https://doi.org/10.1016/j.aej.2025.03.048Application of artificial intelligence in air pollution monitoring and forecasting: A systematic review
Chadalavada, Sreeni, Faust, Oliver, Salvi, Massimo, Seoni, Silvia, Raj, Nawin, Raghavendra, U., Gudigar, Anjan, Barua, Prabal Datta, Molinari, Filippo and Acharya, Rajendra. 2025. "Application of artificial intelligence in air pollution monitoring and forecasting: A systematic review." Environmental Modelling and Software. 185. https://doi.org/10.1016/j.envsoft.2024.106312Electricity demand uncertainty modeling with Temporal Convolution Neural Network models
Ghimire, Sujan, Deo, Ravinesh C., Casillas-Perez, David, Salcedo-Sanz, Sancho, Acharya, Rajendra and Dinh, Toan. 2025. "Electricity demand uncertainty modeling with Temporal Convolution Neural Network models." Renewable and Sustainable Energy Reviews. 209. https://doi.org/10.1016/j.rser.2024.115097Half-hourly electricity price prediction model with explainable-decomposition hybrid deep learning approach
Ghimire, Sujan, Deo, Ravinesh C., Hopf, Konstantin, Liu, Hangyue, Casillas-Perez, David, Helwig, Andreas, Prasad, Salvin S., Perez-Aracil, Jorge, Barua, Prabal Datta and Salcedo-Sanz, Sancho. 2025. "Half-hourly electricity price prediction model with explainable-decomposition hybrid deep learning approach." Energy and AI. 20. https://doi.org/10.1016/j.egyai.2025.100492Investigating Brain Lobe Biomarkers to Enhance Dementia Detection Using EEG Data
Siuly, Siuly, Tawhid, Md.Nurul Ahad, Li, Yan, Acharya, Rajendra, Sadiq, Muhammad Tariq and Wang, Hua. 2025. "Investigating Brain Lobe Biomarkers to Enhance Dementia Detection Using EEG Data." Cognitive Computation. 17 (2). https://doi.org/10.1007/s12559-025-10447-9Explainable hybrid deep learning framework for enhancing multi-step solar ultraviolet-B radiation predictions
Prasad, Salvin S., Joseph, Lionel P., Ghimire, Sujan, Deo, Ravinesh C., Downs, Nathan J., Acharya, Rajendra and Yaseen, Zaher M.. 2025. "Explainable hybrid deep learning framework for enhancing multi-step solar ultraviolet-B radiation predictions." Atmospheric Environment. 343. https://doi.org/10.1016/j.atmosenv.2024.120951CubicPat: Investigations on the Mental Performance and Stress Detection Using EEG Signals
Ince, Ugur, Talu, Yunus, Duz, Aleyna, Tas, Suat, Tanko, Dahiru, Tasci, Irem, Dogan, Sengul, Hafeez-Baig, Abdul, Aydemir, Emrah and Tuncer, Turker. 2025. "CubicPat: Investigations on the Mental Performance and Stress Detection Using EEG Signals." Diagnostics. 15 (3). https://doi.org/10.3390/diagnostics15030363Preserving privacy in healthcare: A systematic review of deep learning approaches for synthetic data generation
Li, Yintong, Acharya, U. Rajendra and Tan, Jen Hong. 2025. "Preserving privacy in healthcare: A systematic review of deep learning approaches for synthetic data generation." Computer Methods and Programs in Biomedicine. 260. https://doi.org/10.1016/j.cmpb.2024.108571Automated hip dysplasia detection using novel FlexiLBPHOG model with ultrasound images
Key, Sefa, Kurum, Huseyin, Esmez, Omer, Hafeez-Baig, Abdul, Hajiyeva, Rena, Dogan, Sengul and Tuncer, Turker. 2025. "Automated hip dysplasia detection using novel FlexiLBPHOG model with ultrasound images." Ain Shams Engineering Journal. 16 (1). https://doi.org/10.1016/j.asej.2024.103235Artificial Intelligence-Based Suicide Prevention and Prediction: A Systematic Review (2019-2023)
Atmakuru, Anirudh, Shahini, Alen, Chakraborty, Subrata, Seoni, Silvia, Salvi, Massimo, Hafeez-Baig, Abdul, Rashid, Sadaf, Tan, Ru San, Barua, Prabal Datta, Molinari, Filippo and Acharya, U Rajendra. 2025. "Artificial Intelligence-Based Suicide Prevention and Prediction: A Systematic Review (2019-2023)." Information Fusion. 114. https://doi.org/10.1016/j.inffus.2024.102673Explainable deeply-fused nets electricity demand prediction model: Factoring climate predictors for accuracy and deeper insights with probabilistic confidence interval and point-based forecasts
Ghimire, Sujan, AL-Musaylh, Mohanad S., Nguyen-Huy, Thong, Deo, Ravinesh C., Acharya, Rajendra, Casillas-Perez, David, Yaseen, Zaher Mundher and Salcedo-sanz, Sancho. 2025. "Explainable deeply-fused nets electricity demand prediction model: Factoring climate predictors for accuracy and deeper insights with probabilistic confidence interval and point-based forecasts." Applied Energy. 378 (Part A). https://doi.org/10.1016/j.apenergy.2024.124763AttentionPoolMobileNeXt: An automated construction damage detection model based on a new convolutional neural network and deep feature engineering models
Aydin, Mehmet, Barua, Prabal Datta, Chadalavada, Sreenivasulu, Dogan, Sengul, Tuncer, Turker, Chakraborty, Subrata and Acharya, Rajendra U.. 2025. "AttentionPoolMobileNeXt: An automated construction damage detection model based on a new convolutional neural network and deep feature engineering models." Multimedia Tools and Applications. 84 (4), pp. 1821-1843. https://doi.org/10.1007/s11042-024-19163-2Directed Lobish-based explainable feature engineering model with TTPat and CWINCA for EEG artifact classification
Tuncer, Turker, Dogan, Sengul, Baygin, Mehmet, Tasci, Irem, Mungen, Bulent, Tasci, Burak, Barua, Prabal Datta and Acharya, U.R.. 2024. "Directed Lobish-based explainable feature engineering model with TTPat and CWINCA for EEG artifact classification." Knowledge-Based Systems. 305. https://doi.org/10.1016/j.knosys.2024.112555Retinal Health Screening Using Artificial Intelligence with Digital Fundus Images: A Review of the Last Decade (2012-2023)
Islam, Saad, Deo, Ravinesh C., Barua, Prabal Datta, Soar, Jeffrey, Yu, Ping and Acharya, U. Rajendra. 2024. "Retinal Health Screening Using Artificial Intelligence with Digital Fundus Images: A Review of the Last Decade (2012-2023)." IEEE Access. 12, pp. 176630-176685. https://doi.org/10.1109/ACCESS.2024.3477420Automated EEG-based language detection using directed quantum pattern technique
Dogan, Sengul, Tuncer, Turker, Barua, Prabal Datta and Acharya, U.R.. 2024. "Automated EEG-based language detection using directed quantum pattern technique." Applied Soft Computing. 167 (Part A). https://doi.org/10.1016/j.asoc.2024.112301A Novel Hybrid Model for Automatic Non-Small Cell Lung Cancer Classification Using Histopathological Images
Katar, Oguzhan, Yildirim, Ozal, Tan, Ru-San and Acharya, U Rajendra. 2024. "A Novel Hybrid Model for Automatic Non-Small Cell Lung Cancer Classification Using Histopathological Images." Diagnostics. 14 (22). https://doi.org/10.3390/diagnostics14222497Synthetic Data Generation via Generative Adversarial Networks in Healthcare: A Systematic Review of Image- and Signal-Based Studies
Akpinar, Muhammed Halil, Sengur, Abdulkadir, Salvi, Massimo, Seoni, Silvia, Faust, Oliver, Mir, Hasan, Molinari,Filippo and Acharya, U. Rajendra. 2024. "Synthetic Data Generation via Generative Adversarial Networks in Healthcare: A Systematic Review of Image- and Signal-Based Studies." IEEE Open Journal of Engineering in Medicine and Biology. 6, pp. 183-192. https://doi.org/10.1109/OJEMB.2024.3508472RECOMED: A comprehensive pharmaceutical recommendation system
Zomorodi, Mariam, Ghodsollahee, Ismail, Martin, Jennifer H, Talley, Nicholas J, Salari, Vahid, Pławiak, Paweł, Rahimi, Kazem and Acharya, U.R.. 2024. "RECOMED: A comprehensive pharmaceutical recommendation system." Artificial Intelligence in Medicine. 157. https://doi.org/10.1016/j.artmed.2024.102981Artificial intelligence in assessing cardiovascular diseases and risk factors via retinal fundus images: A review of the last decade
Abdollahi, Mirsaeed, Jafarizadeh, Ali, Ghafouri-Asbagh, Amirhosein, Sobhi, Navid, Pourmoghtader, Keysan, Pedrammehr, Siamak, Asadi, Houshyar, Tan, Ru-San, Alizadehsani, Roohallah and Acharya, U. Rajendra. 2024. "Artificial intelligence in assessing cardiovascular diseases and risk factors via retinal fundus images: A review of the last decade." WIREs Data Mining and Knowledge Discovery. 14 (6). https://doi.org/10.1002/widm.1560Early prediction of sudden cardiac death using multimodal fusion of ECG Features extracted from Hilbert–Huang and wavelet transforms with explainable vision transformer and CNN models
Telangore, Hardik, Azad, Victor, Sharma, Manish, Bhurane, Ankit, Tan, Ru San and Acharya, U. Rajendra. 2024. "Early prediction of sudden cardiac death using multimodal fusion of ECG Features extracted from Hilbert–Huang and wavelet transforms with explainable vision transformer and CNN models." Computer Methods and Programs in Biomedicine. 257. https://doi.org/10.1016/j.cmpb.2024.108455A Pragmatic Approach to Fetal Monitoring via Cardiotocography Using Feature Elimination and Hyperparameter Optimization
Hardalac, Firat, Akmal, Haad, Ayturan, Kubilay, Acharya, U. Rajendra and Tan, Ru-San. 2024. "A Pragmatic Approach to Fetal Monitoring via Cardiotocography Using Feature Elimination and Hyperparameter Optimization." Interdisciplinary Sciences: Computational Life Sciences. 16 (4), pp. 882-906. https://doi.org/10.1007/s12539-024-00647-6Automated System for the Detection of Heart Anomalies Using Phonocardiograms: A Systematic Review
Gudigar, Anjan, Raghavendra, U., Maithri, M., Samanth, Jyothi, Inamdar, Mahesh Anil, Vidhya, V., Vicnesh, Jahmunah, Prabhu, Mukund A., Tan, Ru-San, Yeong, Chai Hong, Molinari, Filippo and Acharya, U. R.. 2024. "Automated System for the Detection of Heart Anomalies Using Phonocardiograms: A Systematic Review." IEEE Access. 12, pp. 138399-138428. https://doi.org/10.1109/ACCESS.2024.3465511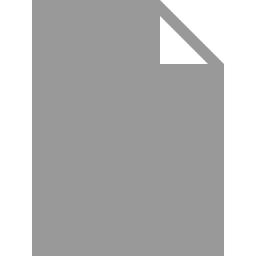