Decision support system for major depression detection using spectrogram and convolution neural network with EEG signals
Article
Article Title | Decision support system for major depression detection using spectrogram and convolution neural network with EEG signals |
---|---|
ERA Journal ID | 17851 |
Article Category | Article |
Authors | Loh, Hui Wen, Ooi, Chui Ping, Aydemir, Emrah, Tuncer, Turker, Dogan, Sengul and Acharya, U. Rajendra |
Journal Title | Expert Systems: the journal of knowledge engineering |
Journal Citation | 39 (3) |
Article Number | e12773 |
Number of Pages | 15 |
Year | 2022 |
Publisher | John Wiley & Sons |
Place of Publication | United Kingdom |
ISSN | 0266-4720 |
1468-0394 | |
Digital Object Identifier (DOI) | https://doi.org/10.1111/exsy.12773 |
Web Address (URL) | https://onlinelibrary.wiley.com/doi/10.1111/exsy.12773 |
Abstract | The number of Major Depressive Disorder (MDD) patients is rising rapidly these days following the incidence of COVID-19 pandemic. It is challenging to detect MDD through personal interviews and by observing electroencephalogram (EEG) signals. Hence, an automated MDD detection system developed using deep learning techniques can help reduce the workload of clinicians by diagnosing MDD accurately. In this study, we have proposed a novel deep learning model based on Convolutional Neural Network (CNN) and spectrogram images. In this work, Short-Time Fourier Transform (STFT) is first applied to the EEG signals to obtain spectrogram images of MDD patients and healthy subjects. These spectrogram images are then fed to the CNN model for automated detection of MDD patients and healthy subjects. The EEG signals used in this study were obtained from public database with 34 MDD patients and 30 healthy subjects. The highest classification accuracy, precision, sensitivity, specificity, and F1-score of 99.58%, 99.40%, 99.70%, 99.48%, and 99.55% respectively were obtained with hold-out validation. Our MDD detection model is highly accurate and needs to be validated with more diverse MDD database before it can be used in clinical settings. Also, we plan to use our developed prototype to detect depression using other physiological signals like electrocardiogram (ECG) and speech signals for accurate and faster diagnosis. |
Keywords | electroencephalogram (EEG); STFT; classification; CNN; deep learning; spectrograms; major depressive disorder (MDD) |
ANZSRC Field of Research 2020 | 400399. Biomedical engineering not elsewhere classified |
Public Notes | File reproduced in accordance with the copyright policy of the publisher/author. |
Byline Affiliations | Singapore University of Social Sciences (SUSS), Singapore |
Sakarya University, Turkey | |
Firat University, Turkey | |
Ngee Ann Polytechnic, Singapore | |
School of Management and Enterprise |
https://research.usq.edu.au/item/z025v/decision-support-system-for-major-depression-detection-using-spectrogram-and-convolution-neural-network-with-eeg-signals
Download files
130
total views247
total downloads8
views this month15
downloads this month
Export as
Related outputs
Fibromyalgia Detection and Diagnosis: A Systematic Review of Data-Driven Approaches and Clinical Implications
Atmakuru, Anirudh, Chakraborty, Subrata, Salvi, Massimo, Faust, Oliver, Barua, Prabal Datta, Kobayashi, Makiko, Tan, Ru San, Molinari, Filippo, Hafeez-Baig, Abdul and Acharya, U. Rajendra. 2025. "Fibromyalgia Detection and Diagnosis: A Systematic Review of Data-Driven Approaches and Clinical Implications." IEEE Access. 13, pp. 25026-25044. https://doi.org/10.1109/ACCESS.2025.3539196Current and future roles of artificial intelligence in retinopathy of prematurity
Jafarizadeh, Ali, Maleki, Shadi Farabi, Pouya, Parnia, Sobhi, Navid, Abdollahi, Mirsaeed, Pedrammehr, Siamak, Lim, Chee Peng, Asadi, Houshyar, Alizadehsani, Roohallah, Tan, Ru-San, Islam, Sheikh Mohammed Shariful and Acharya, U. Rajendra. 2025. "Current and future roles of artificial intelligence in retinopathy of prematurity." Artificial Intelligence Review. 58 (6). https://doi.org/10.1007/s10462-025-11153-6Artificial Intelligence for Ovarian Cancer Detection with Medical Images: A Review of the Last Decade (2013–2023)
Naderi Yaghouti, Amir Reza, Shalbaf, Ahmad, Alizadehsani, Roohallah, Tan, Ru-San, Vijayananthan, Anushya, Yeong, Chai Hong and Acharya, U. Rajendra. 2025. "Artificial Intelligence for Ovarian Cancer Detection with Medical Images: A Review of the Last Decade (2013–2023)." Archives of Computational Methods in Engineering. https://doi.org/10.1007/s11831-025-10268-xFlexiCombFE: A flexible, combination-based feature engineering framework for brain tumor detection
Tuncer, Ilknur, Hafeez-Baig, Abdul, Barua, Prabal Datta, Hajiyeva, Rena, Massimo, Salvi, Dogan, Sengul, Tuncer, Turker and Acharya, U.R.. 2025. "FlexiCombFE: A flexible, combination-based feature engineering framework for brain tumor detection." Biomedical Signal Processing and Control. 104. https://doi.org/10.1016/j.bspc.2025.107538BrainNeXt: novel lightweight CNN model for the automated detection of brain disorders using MRI images
Poyraz, Melahat, Poyraz, Ahmet Kursad, Dogan, Yusuf, Gunes, Selva, Mir, Hasan S., Paul, Jose Kunnel, Barua, Prabal Datta, Baygin, Mehmet, Dogan, Sengul, Tuncer, Turker, Molinari, Filippo and Acharya, Rajendra. 2025. "BrainNeXt: novel lightweight CNN model for the automated detection of brain disorders using MRI images." Cognitive Neurodynamics. 19 (1). https://doi.org/10.1007/s11571-025-10235-zA novel uncertainty-aware liquid neural network for noise-resilient time series forecasting and classification
Akpinar, Muhammed Halil, Atila, Orhan, Sengur, Abdulkadir, Salvi, Massimo and Acharya, U.R.. 2025. "A novel uncertainty-aware liquid neural network for noise-resilient time series forecasting and classification." Chaos, Solitons and Fractals. 193. https://doi.org/10.1016/j.chaos.2025.116130MobileTransNeXt: Integrating CNN, transformer, and BiLSTM for image classification
Ye, Peishun, Lin, Jiyan, Kang, Yaming, Kaya, Tolga, Yildirim, Kubra, Hafeez Baig, Abdul, Aydemir, Emrah, Dogan, Sengul and Tuncer, Turker. 2025. "MobileTransNeXt: Integrating CNN, transformer, and BiLSTM for image classification." Alexandria Engineering Journal. 123, pp. 460-470. https://doi.org/10.1016/j.aej.2025.03.048Electricity demand uncertainty modeling with Temporal Convolution Neural Network models
Ghimire, Sujan, Deo, Ravinesh C., Casillas-Perez, David, Salcedo-Sanz, Sancho, Acharya, Rajendra and Dinh, Toan. 2025. "Electricity demand uncertainty modeling with Temporal Convolution Neural Network models." Renewable and Sustainable Energy Reviews. 209. https://doi.org/10.1016/j.rser.2024.115097Investigating Brain Lobe Biomarkers to Enhance Dementia Detection Using EEG Data
Siuly, Siuly, Tawhid, Md.Nurul Ahad, Li, Yan, Acharya, Rajendra, Sadiq, Muhammad Tariq and Wang, Hua. 2025. "Investigating Brain Lobe Biomarkers to Enhance Dementia Detection Using EEG Data." Cognitive Computation. 17 (2). https://doi.org/10.1007/s12559-025-10447-9Explainable hybrid deep learning framework for enhancing multi-step solar ultraviolet-B radiation predictions
Prasad, Salvin S., Joseph, Lionel P., Ghimire, Sujan, Deo, Ravinesh C., Downs, Nathan J., Acharya, Rajendra and Yaseen, Zaher M.. 2025. "Explainable hybrid deep learning framework for enhancing multi-step solar ultraviolet-B radiation predictions." Atmospheric Environment. 343. https://doi.org/10.1016/j.atmosenv.2024.120951Automated hip dysplasia detection using novel FlexiLBPHOG model with ultrasound images
Key, Sefa, Kurum, Huseyin, Esmez, Omer, Hafeez-Baig, Abdul, Hajiyeva, Rena, Dogan, Sengul and Tuncer, Turker. 2025. "Automated hip dysplasia detection using novel FlexiLBPHOG model with ultrasound images." Ain Shams Engineering Journal. 16 (1). https://doi.org/10.1016/j.asej.2024.103235Artificial Intelligence-Based Suicide Prevention and Prediction: A Systematic Review (2019-2023)
Atmakuru, Anirudh, Shahini, Alen, Chakraborty, Subrata, Seoni, Silvia, Salvi, Massimo, Hafeez-Baig, Abdul, Rashid, Sadaf, Tan, Ru San, Barua, Prabal Datta, Molinari, Filippo and Acharya, U Rajendra. 2025. "Artificial Intelligence-Based Suicide Prevention and Prediction: A Systematic Review (2019-2023)." Information Fusion. 114. https://doi.org/10.1016/j.inffus.2024.102673Explainable deeply-fused nets electricity demand prediction model: Factoring climate predictors for accuracy and deeper insights with probabilistic confidence interval and point-based forecasts
Ghimire, Sujan, AL-Musaylh, Mohanad S., Nguyen-Huy, Thong, Deo, Ravinesh C., Acharya, Rajendra, Casillas-Perez, David, Yaseen, Zaher Mundher and Salcedo-sanz, Sancho. 2025. "Explainable deeply-fused nets electricity demand prediction model: Factoring climate predictors for accuracy and deeper insights with probabilistic confidence interval and point-based forecasts." Applied Energy. 378 (Part A). https://doi.org/10.1016/j.apenergy.2024.124763AttentionPoolMobileNeXt: An automated construction damage detection model based on a new convolutional neural network and deep feature engineering models
Aydin, Mehmet, Barua, Prabal Datta, Chadalavada, Sreenivasulu, Dogan, Sengul, Tuncer, Turker, Chakraborty, Subrata and Acharya, Rajendra U.. 2025. "AttentionPoolMobileNeXt: An automated construction damage detection model based on a new convolutional neural network and deep feature engineering models." Multimedia Tools and Applications. 84 (4), pp. 1821-1843. https://doi.org/10.1007/s11042-024-19163-2Directed Lobish-based explainable feature engineering model with TTPat and CWINCA for EEG artifact classification
Tuncer, Turker, Dogan, Sengul, Baygin, Mehmet, Tasci, Irem, Mungen, Bulent, Tasci, Burak, Barua, Prabal Datta and Acharya, U.R.. 2024. "Directed Lobish-based explainable feature engineering model with TTPat and CWINCA for EEG artifact classification." Knowledge-Based Systems. 305. https://doi.org/10.1016/j.knosys.2024.112555Retinal Health Screening Using Artificial Intelligence with Digital Fundus Images: A Review of the Last Decade (2012-2023)
Islam, Saad, Deo, Ravinesh C., Barua, Prabal Datta, Soar, Jeffrey, Yu, Ping and Acharya, U. Rajendra. 2024. "Retinal Health Screening Using Artificial Intelligence with Digital Fundus Images: A Review of the Last Decade (2012-2023)." IEEE Access. 12, pp. 176630-176685. https://doi.org/10.1109/ACCESS.2024.3477420Automated EEG-based language detection using directed quantum pattern technique
Dogan, Sengul, Tuncer, Turker, Barua, Prabal Datta and Acharya, U.R.. 2024. "Automated EEG-based language detection using directed quantum pattern technique." Applied Soft Computing. 167 (Part A). https://doi.org/10.1016/j.asoc.2024.112301A Novel Hybrid Model for Automatic Non-Small Cell Lung Cancer Classification Using Histopathological Images
Katar, Oguzhan, Yildirim, Ozal, Tan, Ru-San and Acharya, U Rajendra. 2024. "A Novel Hybrid Model for Automatic Non-Small Cell Lung Cancer Classification Using Histopathological Images." Diagnostics. 14 (22). https://doi.org/10.3390/diagnostics14222497Synthetic Data Generation via Generative Adversarial Networks in Healthcare: A Systematic Review of Image- and Signal-Based Studies
Akpinar, Muhammed Halil, Sengur, Abdulkadir, Salvi, Massimo, Seoni, Silvia, Faust, Oliver, Mir, Hasan, Molinari,Filippo and Acharya, U. Rajendra. 2024. "Synthetic Data Generation via Generative Adversarial Networks in Healthcare: A Systematic Review of Image- and Signal-Based Studies." IEEE Open Journal of Engineering in Medicine and Biology. 6, pp. 183-192. https://doi.org/10.1109/OJEMB.2024.3508472RECOMED: A comprehensive pharmaceutical recommendation system
Zomorodi, Mariam, Ghodsollahee, Ismail, Martin, Jennifer H, Talley, Nicholas J, Salari, Vahid, Pławiak, Paweł, Rahimi, Kazem and Acharya, U.R.. 2024. "RECOMED: A comprehensive pharmaceutical recommendation system." Artificial Intelligence in Medicine. 157. https://doi.org/10.1016/j.artmed.2024.102981Artificial intelligence in assessing cardiovascular diseases and risk factors via retinal fundus images: A review of the last decade
Abdollahi, Mirsaeed, Jafarizadeh, Ali, Ghafouri-Asbagh, Amirhosein, Sobhi, Navid, Pourmoghtader, Keysan, Pedrammehr, Siamak, Asadi, Houshyar, Tan, Ru-San, Alizadehsani, Roohallah and Acharya, U. Rajendra. 2024. "Artificial intelligence in assessing cardiovascular diseases and risk factors via retinal fundus images: A review of the last decade." WIREs Data Mining and Knowledge Discovery. 14 (6). https://doi.org/10.1002/widm.1560Early prediction of sudden cardiac death using multimodal fusion of ECG Features extracted from Hilbert–Huang and wavelet transforms with explainable vision transformer and CNN models
Telangore, Hardik, Azad, Victor, Sharma, Manish, Bhurane, Ankit, Tan, Ru San and Acharya, U. Rajendra. 2024. "Early prediction of sudden cardiac death using multimodal fusion of ECG Features extracted from Hilbert–Huang and wavelet transforms with explainable vision transformer and CNN models." Computer Methods and Programs in Biomedicine. 257. https://doi.org/10.1016/j.cmpb.2024.108455A Pragmatic Approach to Fetal Monitoring via Cardiotocography Using Feature Elimination and Hyperparameter Optimization
Hardalac, Firat, Akmal, Haad, Ayturan, Kubilay, Acharya, U. Rajendra and Tan, Ru-San. 2024. "A Pragmatic Approach to Fetal Monitoring via Cardiotocography Using Feature Elimination and Hyperparameter Optimization." Interdisciplinary Sciences: Computational Life Sciences. 16 (4), pp. 882-906. https://doi.org/10.1007/s12539-024-00647-6Automated System for the Detection of Heart Anomalies Using Phonocardiograms: A Systematic Review
Gudigar, Anjan, Raghavendra, U., Maithri, M., Samanth, Jyothi, Inamdar, Mahesh Anil, Vidhya, V., Vicnesh, Jahmunah, Prabhu, Mukund A., Tan, Ru-San, Yeong, Chai Hong, Molinari, Filippo and Acharya, U. R.. 2024. "Automated System for the Detection of Heart Anomalies Using Phonocardiograms: A Systematic Review." IEEE Access. 12, pp. 138399-138428. https://doi.org/10.1109/ACCESS.2024.3465511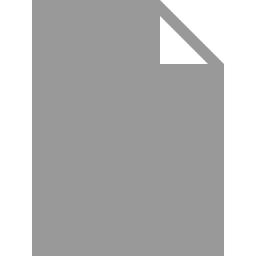