Novel nested patch-based feature extraction model for automated Parkinson’s Disease symptom classification using MRI images
Article
Article Title | Novel nested patch-based feature extraction model for automated Parkinson’s Disease symptom classification using MRI images |
---|---|
ERA Journal ID | 5039 |
Article Category | Article |
Authors | Kaplan, Ela (Author), Altunisik, Erman (Author), Firat, Yasemin Ekmekyapar (Author), Barua, Prabal Datta (Author), Dogan, Sengul (Author), Baygin, Mehmet (Author), Demir, Fahrettin Burak (Author), Tuncer, Turker (Author), Palmer, Elizabeth (Author), Tan, Ru-San (Author), Yu, Ping (Author), Soar, Jeffrey (Author), Fujita, Hamido (Author) and Acharya, U. Rajendra (Author) |
Journal Title | Computer Methods and Programs in Biomedicine |
Journal Citation | 224, pp. 1-11 |
Article Number | 107030 |
Number of Pages | 11 |
Year | 2022 |
Publisher | Elsevier |
Place of Publication | Ireland |
ISSN | 0169-2607 |
1872-7565 | |
Digital Object Identifier (DOI) | https://doi.org/10.1016/j.cmpb.2022.107030 |
Web Address (URL) | https://www.sciencedirect.com/science/article/pii/S0169260722004126 |
Abstract | Objective: Parkinson's disease (PD) is a common neurological disorder with variable clinical manifestations and magnetic resonance imaging (MRI) findings. We propose an effective, handcrafted image classification model that can accurately (i) classify different PD stages, (ii) detect comorbid dementia, and (iii) discriminate PD-related motor symptoms. Methods: Selected image datasets from three PD studies were used to develop the classification model. Our proposed novel automated system was developed in four phases: (i) texture features are extracted from the non-fixed size patches. In the feature extraction phase, a pyramid histogram-oriented gradient (PHOG) image descriptor is used. (ii) In the feature selection phase, four feature selectors: neighborhood component analysis (NCA), Chi2, minimum redundancy maximum relevancy (mRMR), and ReliefF are used to generate four feature vectors. (iii) Two classifiers: k-nearest neighbor (kNN) and support vector machine (SVM) are used in the classification step. A ten-fold cross-validation technique is used to validate the results. (iv) Eight predicted vectors are generated using four selected feature vectors and two classifiers. Finally, iterative majority voting (IMV) is used to attain general classification results. Therefore, this model is named nested patch-PHOG-multiple feature selectors and multiple classifiers-IMV (NP-PHOG-MFSMCIMV). Results: Our presented NP-PHOG-MFSMCIMV model achieved 99.22%, 98.70%, and 99.53% accuracies for the collected PD stages, PD dementia, and PD symptoms classification datasets, respectively. Significance: The obtained accuracies (over 98% for all states) demonstrated the performance of developed NP-PHOG-MFSMCIMV model in automated PD state classification. |
Keywords | PD image classification; Nested patch division; Local binary pattern; Local phase quantization; Neighborhood component analysis; Image classification |
ANZSRC Field of Research 2020 | 460902. Decision support and group support systems |
Public Notes | Files associated with this item cannot be displayed due to copyright restrictions. |
Institution of Origin | University of Southern Queensland |
Byline Affiliations | Adıyaman Training and Research Hospital, Turkiye |
Adiyaman University, Turkiye | |
Sanko University, Turkiye | |
School of Business | |
Firat University, Turkey | |
Ardahan University, Turkiye | |
Bandirma Onyedi Eylul University, Turkiye | |
Department of Health, New South Wales | |
National Heart Centre, Singapore | |
University of Wollongong | |
HUTECH University of Technology, Vietnam | |
School of Mathematics, Physics and Computing | |
Institute for Life Sciences and the Environment | |
Centre for Health Research | |
Institute for Life Sciences and the Environment | |
Kumamoto University, Japan |
https://research.usq.edu.au/item/q78w1/novel-nested-patch-based-feature-extraction-model-for-automated-parkinson-s-disease-symptom-classification-using-mri-images
180
total views2
total downloads3
views this month0
downloads this month
Export as
Related outputs
Automated hip dysplasia detection using novel FlexiLBPHOG model with ultrasound images
Key, Sefa, Kurum, Huseyin, Esmez, Omer, Hafeez-Baig, Abdul, Hajiyeva, Rena, Dogan, Sengul and Tuncer, Turker. 2025. "Automated hip dysplasia detection using novel FlexiLBPHOG model with ultrasound images." Ain Shams Engineering Journal. 16 (1). https://doi.org/10.1016/j.asej.2024.103235Artificial Intelligence-Based Suicide Prevention and Prediction: A Systematic Review (2019-2023)
Atmakuru, Anirudh, Shahini, Alen, Chakraborty, Subrata, Seoni, Silvia, Salvi, Massimo, Hafeez-Baig, Abdul, Rashid, Sadaf, Tan, Ru San, Barua, Prabal Datta, Molinari, Filippo and Acharya, U Rajendra. 2025. "Artificial Intelligence-Based Suicide Prevention and Prediction: A Systematic Review (2019-2023)." Information Fusion. 114. https://doi.org/10.1016/j.inffus.2024.102673Explainable deeply-fused nets electricity demand prediction model: Factoring climate predictors for accuracy and deeper insights with probabilistic confidence interval and point-based forecasts
Ghimire, Sujan, AL-Musaylh, Mohanad S., Nguyen-Huy, Thong, Deo, Ravinesh C., Acharya, Rajendra, Casillas-Perez, David, Yaseen, Zaher Mundher and Salcedo-sanz, Sancho. 2025. "Explainable deeply-fused nets electricity demand prediction model: Factoring climate predictors for accuracy and deeper insights with probabilistic confidence interval and point-based forecasts." Applied Energy. 378 (Part A). https://doi.org/10.1016/j.apenergy.2024.124763AttentionPoolMobileNeXt: An automated construction damage detection model based on a new convolutional neural network and deep feature engineering models
Aydin, Mehmet, Barua, Prabal Datta, Chadalavada, Sreenivasulu, Dogan, Sengul, Tuncer, Turker, Chakraborty, Subrata and Acharya, Rajendra U.. 2025. "AttentionPoolMobileNeXt: An automated construction damage detection model based on a new convolutional neural network and deep feature engineering models." Multimedia Tools and Applications. 84 (4), pp. 1821-1843. https://doi.org/10.1007/s11042-024-19163-2Exploring veganism in Australia, France, Spain, and Portugal: a netnographic study using the transtheoretical model
Saleh, Gelareh, Ward, Aletha, Dillon-Murray, Angela, Canseco-Lopez, Fatima and Soar, Jeffrey. 2025. "Exploring veganism in Australia, France, Spain, and Portugal: a netnographic study using the transtheoretical model." Food, Culture, and Society. 28 (1), pp. 102-13. https://doi.org/10.1080/15528014.2024.2351661Directed Lobish-based explainable feature engineering model with TTPat and CWINCA for EEG artifact classification
Tuncer, Turker, Dogan, Sengul, Baygin, Mehmet, Tasci, Irem, Mungen, Bulent, Tasci, Burak, Barua, Prabal Datta and Acharya, U.R.. 2024. "Directed Lobish-based explainable feature engineering model with TTPat and CWINCA for EEG artifact classification." Knowledge-Based Systems. 305. https://doi.org/10.1016/j.knosys.2024.112555Retinal Health Screening Using Artificial Intelligence with Digital Fundus Images: A Review of the Last Decade (2012-2023)
Islam, Saad, Deo, Ravinesh C., Barua, Prabal Datta, Soar, Jeffrey, Yu, Ping and Acharya, U. Rajendra. 2024. "Retinal Health Screening Using Artificial Intelligence with Digital Fundus Images: A Review of the Last Decade (2012-2023)." IEEE Access. 12, pp. 176630-176685. https://doi.org/10.1109/ACCESS.2024.3477420Automated EEG-based language detection using directed quantum pattern technique
Dogan, Sengul, Tuncer, Turker, Barua, Prabal Datta and Acharya, U.R.. 2024. "Automated EEG-based language detection using directed quantum pattern technique." Applied Soft Computing. 167 (Part A). https://doi.org/10.1016/j.asoc.2024.112301A Novel Hybrid Model for Automatic Non-Small Cell Lung Cancer Classification Using Histopathological Images
Katar, Oguzhan, Yildirim, Ozal, Tan, Ru-San and Acharya, U Rajendra. 2024. "A Novel Hybrid Model for Automatic Non-Small Cell Lung Cancer Classification Using Histopathological Images." Diagnostics. 14 (22). https://doi.org/10.3390/diagnostics14222497Synthetic Data Generation via Generative Adversarial Networks in Healthcare: A Systematic Review of Image- and Signal-Based Studies
Akpinar, Muhammed Halil, Sengur, Abdulkadir, Salvi, Massimo, Seoni, Silvia, Faust, Oliver, Mir, Hasan, Molinari,Filippo and Acharya, U. Rajendra. 2024. "Synthetic Data Generation via Generative Adversarial Networks in Healthcare: A Systematic Review of Image- and Signal-Based Studies." IEEE Open Journal of Engineering in Medicine and Biology. 6, pp. 183-192. https://doi.org/10.1109/OJEMB.2024.3508472RECOMED: A comprehensive pharmaceutical recommendation system
Zomorodi, Mariam, Ghodsollahee, Ismail, Martin, Jennifer H, Talley, Nicholas J, Salari, Vahid, Pławiak, Paweł, Rahimi, Kazem and Acharya, U.R.. 2024. "RECOMED: A comprehensive pharmaceutical recommendation system." Artificial Intelligence in Medicine. 157. https://doi.org/10.1016/j.artmed.2024.102981Artificial intelligence in assessing cardiovascular diseases and risk factors via retinal fundus images: A review of the last decade
Abdollahi, Mirsaeed, Jafarizadeh, Ali, Ghafouri-Asbagh, Amirhosein, Sobhi, Navid, Pourmoghtader, Keysan, Pedrammehr, Siamak, Asadi, Houshyar, Tan, Ru-San, Alizadehsani, Roohallah and Acharya, U. Rajendra. 2024. "Artificial intelligence in assessing cardiovascular diseases and risk factors via retinal fundus images: A review of the last decade." WIREs Data Mining and Knowledge Discovery. 14 (6). https://doi.org/10.1002/widm.1560Early prediction of sudden cardiac death using multimodal fusion of ECG Features extracted from Hilbert–Huang and wavelet transforms with explainable vision transformer and CNN models
Telangore, Hardik, Azad, Victor, Sharma, Manish, Bhurane, Ankit, Tan, Ru San and Acharya, U. Rajendra. 2024. "Early prediction of sudden cardiac death using multimodal fusion of ECG Features extracted from Hilbert–Huang and wavelet transforms with explainable vision transformer and CNN models." Computer Methods and Programs in Biomedicine. 257. https://doi.org/10.1016/j.cmpb.2024.108455A Pragmatic Approach to Fetal Monitoring via Cardiotocography Using Feature Elimination and Hyperparameter Optimization
Hardalac, Firat, Akmal, Haad, Ayturan, Kubilay, Acharya, U. Rajendra and Tan, Ru-San. 2024. "A Pragmatic Approach to Fetal Monitoring via Cardiotocography Using Feature Elimination and Hyperparameter Optimization." Interdisciplinary Sciences: Computational Life Sciences. 16 (4), pp. 882-906. https://doi.org/10.1007/s12539-024-00647-6Automated System for the Detection of Heart Anomalies Using Phonocardiograms: A Systematic Review
Gudigar, Anjan, Raghavendra, U., Maithri, M., Samanth, Jyothi, Inamdar, Mahesh Anil, Vidhya, V., Vicnesh, Jahmunah, Prabhu, Mukund A., Tan, Ru-San, Yeong, Chai Hong, Molinari, Filippo and Acharya, U. R.. 2024. "Automated System for the Detection of Heart Anomalies Using Phonocardiograms: A Systematic Review." IEEE Access. 12, pp. 138399-138428. https://doi.org/10.1109/ACCESS.2024.3465511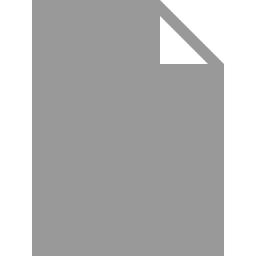