TMP19: A Novel Ternary Motif Pattern-Based ADHD Detection Model Using EEG Signals
Article
Article Title | TMP19: A Novel Ternary Motif Pattern-Based ADHD Detection Model Using EEG Signals |
---|---|
ERA Journal ID | 212275 |
Article Category | Article |
Authors | Barua, Prabal Datta, Dogan, Sengul, Baygin, Mehmet, Tuncer, Turker, Palmer, Elizabeth Emma, Ciaccio, Edward J. and Acharya, U. Rajendra |
Journal Title | Diagnostics |
Journal Citation | 12 (10) |
Article Number | 2544 |
Number of Pages | 14 |
Year | 2022 |
Publisher | MDPI AG |
Place of Publication | Switzerland |
ISSN | 2075-4418 |
Digital Object Identifier (DOI) | https://doi.org/10.3390/diagnostics12102544 |
Web Address (URL) | https://www.mdpi.com/2075-4418/12/10/2544 |
Abstract | Attention deficit hyperactivity disorder (ADHD) is a common neurodevelopmental condition worldwide. In this research, we used an ADHD electroencephalography (EEG) dataset containing more than 4000 EEG signals. Moreover, these EEGs are noisy signals. A new hand-modeled EEG classification model has been proposed to separate healthy versus ADHD individuals using the EEG signals. In this model, a new ternary motif pattern (TMP) has been incorporated. We have mimicked deep learning networks to create this hand-modeled classification method. The Tunable Q Wavelet Transform (TQWT) has been utilized to generate wavelet subbands. We applied the proposed TMP and statistics to construct informative features from both raw EEG signals and wavelet bands by generating TQWT. Herein, features have been generated by 18 subbands and the original EEG signal. Thus, this model is named TMP19. The most informative features have been chosen by deploying neighborhood component analysis (NCA), and the selected features have been classified using the k-nearest neighbor (kNN) classifier. The used ADHD EEG dataset has 14 channels. Thus, these three phases—(i) feature extraction with TQWT, TMP, and statistics; (ii) feature selection by deploying NCA; and (iii) classification with kNN—have been applied to each channel. Iterative hard majority voting (IHMV) has been applied to obtain a higher and more general classification response. Our model attained 95.57% and 77.93% classification accuracies by deploying 10-fold and leave one subject out (LOSO) cross-validations, respectively. |
Keywords | ADHD detection; EEG signal classification; signal processing; ternary motif pattern |
Contains Sensitive Content | Does not contain sensitive content |
ANZSRC Field of Research 2020 | 400306. Computational physiology |
Byline Affiliations | School of Business |
University of Technology Sydney | |
Firat University, Turkey | |
Ardahan University, Turkiye | |
Department of Health, New South Wales | |
University of New South Wales | |
Columbia University Irving Medical Center, United States | |
Ngee Ann Polytechnic, Singapore | |
Singapore University of Social Sciences (SUSS), Singapore | |
Asia University, Taiwan |
https://research.usq.edu.au/item/yyq28/tmp19-a-novel-ternary-motif-pattern-based-adhd-detection-model-using-eeg-signals
Download files
95
total views30
total downloads1
views this month0
downloads this month
Export as
Related outputs
Fibromyalgia Detection and Diagnosis: A Systematic Review of Data-Driven Approaches and Clinical Implications
Atmakuru, Anirudh, Chakraborty, Subrata, Salvi, Massimo, Faust, Oliver, Barua, Prabal Datta, Kobayashi, Makiko, Tan, Ru San, Molinari, Filippo, Hafeez-Baig, Abdul and Acharya, U. Rajendra. 2025. "Fibromyalgia Detection and Diagnosis: A Systematic Review of Data-Driven Approaches and Clinical Implications." IEEE Access. 13, pp. 25026-25044. https://doi.org/10.1109/ACCESS.2025.3539196Automated hip dysplasia detection using novel FlexiLBPHOG model with ultrasound images
Key, Sefa, Kurum, Huseyin, Esmez, Omer, Hafeez-Baig, Abdul, Hajiyeva, Rena, Dogan, Sengul and Tuncer, Turker. 2025. "Automated hip dysplasia detection using novel FlexiLBPHOG model with ultrasound images." Ain Shams Engineering Journal. 16 (1). https://doi.org/10.1016/j.asej.2024.103235Artificial Intelligence-Based Suicide Prevention and Prediction: A Systematic Review (2019-2023)
Atmakuru, Anirudh, Shahini, Alen, Chakraborty, Subrata, Seoni, Silvia, Salvi, Massimo, Hafeez-Baig, Abdul, Rashid, Sadaf, Tan, Ru San, Barua, Prabal Datta, Molinari, Filippo and Acharya, U Rajendra. 2025. "Artificial Intelligence-Based Suicide Prevention and Prediction: A Systematic Review (2019-2023)." Information Fusion. 114. https://doi.org/10.1016/j.inffus.2024.102673Explainable deeply-fused nets electricity demand prediction model: Factoring climate predictors for accuracy and deeper insights with probabilistic confidence interval and point-based forecasts
Ghimire, Sujan, AL-Musaylh, Mohanad S., Nguyen-Huy, Thong, Deo, Ravinesh C., Acharya, Rajendra, Casillas-Perez, David, Yaseen, Zaher Mundher and Salcedo-sanz, Sancho. 2025. "Explainable deeply-fused nets electricity demand prediction model: Factoring climate predictors for accuracy and deeper insights with probabilistic confidence interval and point-based forecasts." Applied Energy. 378 (Part A). https://doi.org/10.1016/j.apenergy.2024.124763AttentionPoolMobileNeXt: An automated construction damage detection model based on a new convolutional neural network and deep feature engineering models
Aydin, Mehmet, Barua, Prabal Datta, Chadalavada, Sreenivasulu, Dogan, Sengul, Tuncer, Turker, Chakraborty, Subrata and Acharya, Rajendra U.. 2025. "AttentionPoolMobileNeXt: An automated construction damage detection model based on a new convolutional neural network and deep feature engineering models." Multimedia Tools and Applications. 84 (4), pp. 1821-1843. https://doi.org/10.1007/s11042-024-19163-2Directed Lobish-based explainable feature engineering model with TTPat and CWINCA for EEG artifact classification
Tuncer, Turker, Dogan, Sengul, Baygin, Mehmet, Tasci, Irem, Mungen, Bulent, Tasci, Burak, Barua, Prabal Datta and Acharya, U.R.. 2024. "Directed Lobish-based explainable feature engineering model with TTPat and CWINCA for EEG artifact classification." Knowledge-Based Systems. 305. https://doi.org/10.1016/j.knosys.2024.112555Retinal Health Screening Using Artificial Intelligence with Digital Fundus Images: A Review of the Last Decade (2012-2023)
Islam, Saad, Deo, Ravinesh C., Barua, Prabal Datta, Soar, Jeffrey, Yu, Ping and Acharya, U. Rajendra. 2024. "Retinal Health Screening Using Artificial Intelligence with Digital Fundus Images: A Review of the Last Decade (2012-2023)." IEEE Access. 12, pp. 176630-176685. https://doi.org/10.1109/ACCESS.2024.3477420Automated EEG-based language detection using directed quantum pattern technique
Dogan, Sengul, Tuncer, Turker, Barua, Prabal Datta and Acharya, U.R.. 2024. "Automated EEG-based language detection using directed quantum pattern technique." Applied Soft Computing. 167 (Part A). https://doi.org/10.1016/j.asoc.2024.112301A Novel Hybrid Model for Automatic Non-Small Cell Lung Cancer Classification Using Histopathological Images
Katar, Oguzhan, Yildirim, Ozal, Tan, Ru-San and Acharya, U Rajendra. 2024. "A Novel Hybrid Model for Automatic Non-Small Cell Lung Cancer Classification Using Histopathological Images." Diagnostics. 14 (22). https://doi.org/10.3390/diagnostics14222497Synthetic Data Generation via Generative Adversarial Networks in Healthcare: A Systematic Review of Image- and Signal-Based Studies
Akpinar, Muhammed Halil, Sengur, Abdulkadir, Salvi, Massimo, Seoni, Silvia, Faust, Oliver, Mir, Hasan, Molinari,Filippo and Acharya, U. Rajendra. 2024. "Synthetic Data Generation via Generative Adversarial Networks in Healthcare: A Systematic Review of Image- and Signal-Based Studies." IEEE Open Journal of Engineering in Medicine and Biology. 6, pp. 183-192. https://doi.org/10.1109/OJEMB.2024.3508472RECOMED: A comprehensive pharmaceutical recommendation system
Zomorodi, Mariam, Ghodsollahee, Ismail, Martin, Jennifer H, Talley, Nicholas J, Salari, Vahid, Pławiak, Paweł, Rahimi, Kazem and Acharya, U.R.. 2024. "RECOMED: A comprehensive pharmaceutical recommendation system." Artificial Intelligence in Medicine. 157. https://doi.org/10.1016/j.artmed.2024.102981Artificial intelligence in assessing cardiovascular diseases and risk factors via retinal fundus images: A review of the last decade
Abdollahi, Mirsaeed, Jafarizadeh, Ali, Ghafouri-Asbagh, Amirhosein, Sobhi, Navid, Pourmoghtader, Keysan, Pedrammehr, Siamak, Asadi, Houshyar, Tan, Ru-San, Alizadehsani, Roohallah and Acharya, U. Rajendra. 2024. "Artificial intelligence in assessing cardiovascular diseases and risk factors via retinal fundus images: A review of the last decade." WIREs Data Mining and Knowledge Discovery. 14 (6). https://doi.org/10.1002/widm.1560Early prediction of sudden cardiac death using multimodal fusion of ECG Features extracted from Hilbert–Huang and wavelet transforms with explainable vision transformer and CNN models
Telangore, Hardik, Azad, Victor, Sharma, Manish, Bhurane, Ankit, Tan, Ru San and Acharya, U. Rajendra. 2024. "Early prediction of sudden cardiac death using multimodal fusion of ECG Features extracted from Hilbert–Huang and wavelet transforms with explainable vision transformer and CNN models." Computer Methods and Programs in Biomedicine. 257. https://doi.org/10.1016/j.cmpb.2024.108455A Pragmatic Approach to Fetal Monitoring via Cardiotocography Using Feature Elimination and Hyperparameter Optimization
Hardalac, Firat, Akmal, Haad, Ayturan, Kubilay, Acharya, U. Rajendra and Tan, Ru-San. 2024. "A Pragmatic Approach to Fetal Monitoring via Cardiotocography Using Feature Elimination and Hyperparameter Optimization." Interdisciplinary Sciences: Computational Life Sciences. 16 (4), pp. 882-906. https://doi.org/10.1007/s12539-024-00647-6Automated System for the Detection of Heart Anomalies Using Phonocardiograms: A Systematic Review
Gudigar, Anjan, Raghavendra, U., Maithri, M., Samanth, Jyothi, Inamdar, Mahesh Anil, Vidhya, V., Vicnesh, Jahmunah, Prabhu, Mukund A., Tan, Ru-San, Yeong, Chai Hong, Molinari, Filippo and Acharya, U. R.. 2024. "Automated System for the Detection of Heart Anomalies Using Phonocardiograms: A Systematic Review." IEEE Access. 12, pp. 138399-138428. https://doi.org/10.1109/ACCESS.2024.3465511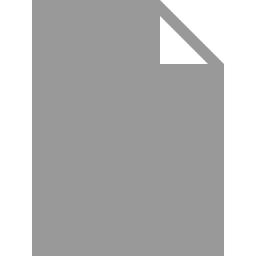