MobileTransNeXt: Integrating CNN, transformer, and BiLSTM for image classification
Article
Article Title | MobileTransNeXt: Integrating CNN, transformer, and BiLSTM for image classification |
---|---|
ERA Journal ID | 44460 |
Article Category | Article |
Authors | Ye, Peishun, Lin, Jiyan, Kang, Yaming, Kaya, Tolga, Yildirim, Kubra, Hafeez Baig, Abdul, Aydemir, Emrah, Dogan, Sengul and Tuncer, Turker |
Journal Title | Alexandria Engineering Journal |
Journal Citation | 123, pp. 460-470 |
Number of Pages | 11 |
Year | 2025 |
Publisher | Elsevier |
Place of Publication | Egypt |
ISSN | 1110-0168 |
2090-2670 | |
Digital Object Identifier (DOI) | https://doi.org/10.1016/j.aej.2025.03.048 |
Web Address (URL) | https://www.sciencedirect.com/science/article/pii/S1110016825003540 |
Abstract | Transformers have become popular in computer vision by competing with convolutional neural networks (CNNs). However, CNNs have high potential in deep learning, and models based on transformer and CNN collaboration need to be proposed to achieve better performance. This study presents a new hybrid model that achieves high classification accuracy with fewer learnable parameters, and the main goal is to achieve high classification accuracy with fewer learnable parameters. In this research, first, an innovative deep learning architecture consisting of CNN and transformer collaboration is proposed, and in this model is termed MobileTransNeXt. The recommended MobileTransNeXt is obtained by integrating a transformer into MobileNetV2. MobileTransNeXt creates a feature map using MobileNetV2 and uses a transformer to classify the created feature map. In addition, a MobileTransNeXt-based deep feature engineering (DFE) approach is proposed to take the test classification ability to the next level and show high transfer learning ability. The presented models were tested on two datasets (UC-Merced and NWPU-RESISC45). MobileTransNeXt achieved 96.90 % and 95.18 % accuracy, while the DFE model performed even better, reaching 98.81 % and 95.29 %. These results clearly show that MobileTransNeXt is a new computer vision solution. |
Keywords | Deep feature engineering; MobileTransNeXt; Iterative feature selection; Remote sensing image; Patch-based feature extraction |
Contains Sensitive Content | Does not contain sensitive content |
ANZSRC Field of Research 2020 | 460999. Information systems not elsewhere classified |
Byline Affiliations | Yulin University, China |
Turkish Aerospace Industries (TUSAS), Turkey | |
Firat University, Turkey | |
School of Management and Enterprise | |
Sakarya University, Turkey |
https://research.usq.edu.au/item/zx15y/mobiletransnext-integrating-cnn-transformer-and-bilstm-for-image-classification
Download files
39
total views12
total downloads6
views this month8
downloads this month
Export as
Related outputs
Dr Abdul Hafeez-Baig
Hafeez-Baig, A.. Dr Abdul Hafeez-Baig.Fibromyalgia Detection and Diagnosis: A Systematic Review of Data-Driven Approaches and Clinical Implications
Atmakuru, Anirudh, Chakraborty, Subrata, Salvi, Massimo, Faust, Oliver, Barua, Prabal Datta, Kobayashi, Makiko, Tan, Ru San, Molinari, Filippo, Hafeez-Baig, Abdul and Acharya, U. Rajendra. 2025. "Fibromyalgia Detection and Diagnosis: A Systematic Review of Data-Driven Approaches and Clinical Implications." IEEE Access. 13, pp. 25026-25044. https://doi.org/10.1109/ACCESS.2025.3539196Zipper Pattern: An Investigation into Psychotic Criminal Detection Using EEG Signals
Tasci, Gulay, Barua, Prabal Datta, Tanko, Dahiru, Keles, Tugce, Tas, Suat, Tuncer, Ilknur, Kaya, Suheda, Yildirim, Kubra, Talu, Yunus, Tasci, Burak, Ozsoy, Filiz, Gonen, Nida, Tasci, Irem, Dogan, Sengul and Tuncer, Turker. 2025. "Zipper Pattern: An Investigation into Psychotic Criminal Detection Using EEG Signals." Diagnostics. 15 (2). https://doi.org/10.3390/diagnostics15020154FlexiCombFE: A flexible, combination-based feature engineering framework for brain tumor detection
Tuncer, Ilknur, Hafeez-Baig, Abdul, Barua, Prabal Datta, Hajiyeva, Rena, Massimo, Salvi, Dogan, Sengul, Tuncer, Turker and Acharya, U.R.. 2025. "FlexiCombFE: A flexible, combination-based feature engineering framework for brain tumor detection." Biomedical Signal Processing and Control. 104. https://doi.org/10.1016/j.bspc.2025.107538BrainNeXt: novel lightweight CNN model for the automated detection of brain disorders using MRI images
Poyraz, Melahat, Poyraz, Ahmet Kursad, Dogan, Yusuf, Gunes, Selva, Mir, Hasan S., Paul, Jose Kunnel, Barua, Prabal Datta, Baygin, Mehmet, Dogan, Sengul, Tuncer, Turker, Molinari, Filippo and Acharya, Rajendra. 2025. "BrainNeXt: novel lightweight CNN model for the automated detection of brain disorders using MRI images." Cognitive Neurodynamics. 19 (1). https://doi.org/10.1007/s11571-025-10235-zCubicPat: Investigations on the Mental Performance and Stress Detection Using EEG Signals
Ince, Ugur, Talu, Yunus, Duz, Aleyna, Tas, Suat, Tanko, Dahiru, Tasci, Irem, Dogan, Sengul, Hafeez-Baig, Abdul, Aydemir, Emrah and Tuncer, Turker. 2025. "CubicPat: Investigations on the Mental Performance and Stress Detection Using EEG Signals." Diagnostics. 15 (3). https://doi.org/10.3390/diagnostics15030363Automated hip dysplasia detection using novel FlexiLBPHOG model with ultrasound images
Key, Sefa, Kurum, Huseyin, Esmez, Omer, Hafeez-Baig, Abdul, Hajiyeva, Rena, Dogan, Sengul and Tuncer, Turker. 2025. "Automated hip dysplasia detection using novel FlexiLBPHOG model with ultrasound images." Ain Shams Engineering Journal. 16 (1). https://doi.org/10.1016/j.asej.2024.103235Artificial Intelligence-Based Suicide Prevention and Prediction: A Systematic Review (2019-2023)
Atmakuru, Anirudh, Shahini, Alen, Chakraborty, Subrata, Seoni, Silvia, Salvi, Massimo, Hafeez-Baig, Abdul, Rashid, Sadaf, Tan, Ru San, Barua, Prabal Datta, Molinari, Filippo and Acharya, U Rajendra. 2025. "Artificial Intelligence-Based Suicide Prevention and Prediction: A Systematic Review (2019-2023)." Information Fusion. 114. https://doi.org/10.1016/j.inffus.2024.102673AttentionPoolMobileNeXt: An automated construction damage detection model based on a new convolutional neural network and deep feature engineering models
Aydin, Mehmet, Barua, Prabal Datta, Chadalavada, Sreenivasulu, Dogan, Sengul, Tuncer, Turker, Chakraborty, Subrata and Acharya, Rajendra U.. 2025. "AttentionPoolMobileNeXt: An automated construction damage detection model based on a new convolutional neural network and deep feature engineering models." Multimedia Tools and Applications. 84 (4), pp. 1821-1843. https://doi.org/10.1007/s11042-024-19163-2Directed Lobish-based explainable feature engineering model with TTPat and CWINCA for EEG artifact classification
Tuncer, Turker, Dogan, Sengul, Baygin, Mehmet, Tasci, Irem, Mungen, Bulent, Tasci, Burak, Barua, Prabal Datta and Acharya, U.R.. 2024. "Directed Lobish-based explainable feature engineering model with TTPat and CWINCA for EEG artifact classification." Knowledge-Based Systems. 305. https://doi.org/10.1016/j.knosys.2024.112555Automated EEG-based language detection using directed quantum pattern technique
Dogan, Sengul, Tuncer, Turker, Barua, Prabal Datta and Acharya, U.R.. 2024. "Automated EEG-based language detection using directed quantum pattern technique." Applied Soft Computing. 167 (Part A). https://doi.org/10.1016/j.asoc.2024.112301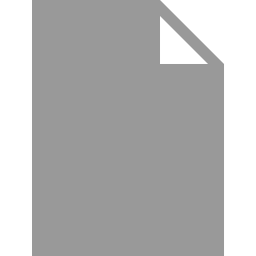