Black-white hole pattern: an investigation on the automated chronic neuropathic pain detection using EEG signals
Article
Tasci, Irem, Baygin, Mehmet, Barua, Prabal Datta, Hafeez-Baig, Abdul, Dogan, Sengul, Tuncer, Turker, Tan, Ru-San and Acharya, U. Rajendra. 2024. "Black-white hole pattern: an investigation on the automated chronic neuropathic pain detection using EEG signals." Cognitive Neurodynamics. 18 (5), pp. 2193-2210. https://doi.org/10.1007/s11571-024-10078-0
Article Title | Black-white hole pattern: an investigation on the automated chronic neuropathic pain detection using EEG signals |
---|---|
ERA Journal ID | 3179 |
Article Category | Article |
Authors | Tasci, Irem, Baygin, Mehmet, Barua, Prabal Datta, Hafeez-Baig, Abdul, Dogan, Sengul, Tuncer, Turker, Tan, Ru-San and Acharya, U. Rajendra |
Journal Title | Cognitive Neurodynamics |
Journal Citation | 18 (5), pp. 2193-2210 |
Number of Pages | 18 |
Year | 2024 |
Publisher | Springer |
Place of Publication | Netherlands |
ISSN | 1871-4080 |
1871-4099 | |
Digital Object Identifier (DOI) | https://doi.org/10.1007/s11571-024-10078-0 |
Web Address (URL) | https://link.springer.com/article/10.1007/s11571-024-10078-0 |
Abstract | Electroencephalography (EEG) signals provide information about the brain activities, this study bridges neuroscience and machine learning by introducing an astronomy-inspired feature extraction model. In this work, we developed a novel feature extraction function, black-white hole pattern (BWHPat) which dynamically selects the most suitable pattern from 14 options. We developed BWHPat in a four-phase feature engineering model, involving multileveled feature extraction, feature selection, classification, and cortex map generation. Textural and statistical features are extracted in the first phase, while tunable q-factor wavelet transform (TQWT) aids in multileveled feature extraction. The second phase employs iterative neighborhood component analysis (INCA) for feature selection, and the k-nearest neighbors (kNN) classifier is applied for classification, yielding channel-specific results. A new cortex map generation model highlights the most active channels using median and intersection functions. Our BWHPat-driven model consistently achieved over 99% classification accuracy across three scenarios using the publicly available EEG pain dataset. Furthermore, a semantic cortex map precisely identifies pain-affected brain regions. This study signifies the contribution to EEG signal classification and neuroscience. The BWHPat pattern establishes a unique link between astronomy and feature extraction, enhancing the understanding of brain activities. |
Keywords | Black-white hole pattern; EEG pain detection; Neuroscience; cortex map |
Contains Sensitive Content | Does not contain sensitive content |
ANZSRC Field of Research 2020 | 400306. Computational physiology |
Byline Affiliations | Firat University, Turkey |
Erzurum Technical University, Turkey | |
School of Business | |
School of Management and Enterprise | |
National Heart Centre, Singapore | |
Duke-NUS Medical School, Singapore | |
School of Mathematics, Physics and Computing |
Permalink -
https://research.usq.edu.au/item/z5vx1/black-white-hole-pattern-an-investigation-on-the-automated-chronic-neuropathic-pain-detection-using-eeg-signals
Download files
Explore this article
Change management and adoption of health information technology (HIT)/eHealth in public hospitals in Ghana: A qualitative study
Yusif, Salifu, Hafeez-Baig, Abdul and Soar, Jeffrey. 2022. "Change management and adoption of health information technology (HIT)/eHealth in public hospitals in Ghana: A qualitative study." Applied Computing and Informatics. 18 (3-4), pp. 279-289. https://doi.org/10.1016/j.aci.2019.11.007The effect of talent management processes on knowledge creation: a case of Australian higher education
Mohammed, Atheer Abdullah, Hafeez Baig, Abdul and Gururajan, Raj. 2019. "The effect of talent management processes on knowledge creation: a case of Australian higher education." Journal of Industry-University Collaboration. 1 (3), pp. 132-152. https://doi.org/10.1108/JIUC-05-2019-0010PLS-SEM path analysis to determine the predictive relevance of e-Health readiness assessment model
Yusif, Salifu, Hafeez-Baig, Abdul, Soar, Jeffrey and Ong Lai Teik, Derek. 2020. "PLS-SEM path analysis to determine the predictive relevance of e-Health readiness assessment model." Health And Technology. 10 (6), pp. 1497-1513. https://doi.org/10.1007/s12553-020-00484-9A model for evaluating eHealth preparedness – a case study approach
Yusif, Salifu, Hafeez-Baig, Abdul and Soar, Jeffrey. 2020. "A model for evaluating eHealth preparedness – a case study approach." Transforming Government: People, Process and Policy. 14 (3), pp. 561-587. https://doi.org/10.1108/TG-07-2019-0069An Exploratory Study of the Readiness of Public Healthcare Facilities in Developing Countries to Adopt Health Information Technology (HIT)/e-Health: the Case of Ghana
Yusif, Salifu, Hafeez-Baig, Abdul and Soar, Jeffrey. 2020. "An Exploratory Study of the Readiness of Public Healthcare Facilities in Developing Countries to Adopt Health Information Technology (HIT)/e-Health: the Case of Ghana." Journal of Healthcare Informatics Research. 4 (2), pp. 189-214. https://doi.org/10.1007/s41666-020-00070-8Cybersecurity Policy Compliance in Higher Education: A Theoretical Framework
Yusif, Salifu and Hafeez-Baig, Abdul. 2023. "Cybersecurity Policy Compliance in Higher Education: A Theoretical Framework." Journal of Applied Security Research. 18 (2), pp. 267-288. https://doi.org/10.1080/19361610.2021.1989271The role of image quality in telehealth: adoption challenges in the subcontinent
Chowdhury, Avijit, Hafeez-Baig, Abdul, Gururajan, Raj, McCubbin, Amanda, Sharif, Mirza Akmal and Miah, Shah J.. 2021. "The role of image quality in telehealth: adoption challenges in the subcontinent." Pacific Asia Journal of the Association for Information Systems. 13 (3), pp. 131-145. https://doi.org/10.17705/1pais.13307Effects of rubric quality on marker variation in higher education
Chakraborty, Subrata, Dann, Christopher, Mandal, Anuradha, Dann, Beverly, Paul, Manoranjan and Hafeez-Baig, Abdul. 2021. "Effects of rubric quality on marker variation in higher education." Studies in Educational Evaluation. 70, pp. 1-12. https://doi.org/10.1016/j.stueduc.2021.100997The hidden reasons of the Vietnamese parents for paying private tuition fees for public school teachers
Nguyen, The Cuong, Hafeez-Baig, Abdul, Gururajan, Raj and Nguyen, Nam C.. 2021. "The hidden reasons of the Vietnamese parents for paying private tuition fees for public school teachers." Social Sciences & Humanities Open. 3 (1), pp. 1-10. https://doi.org/10.1016/j.ssaho.2021.100105A conceptual model for cybersecurity governance
Yusif, Salifu and Hafeez-Baig, Abdul. 2021. "A conceptual model for cybersecurity governance." Journal of Applied Security Research. 16 (4), pp. 490-513. https://doi.org/10.1080/19361610.2021.1918995Development of a survey instrument to explore telehealth adoption in the healthcare domain
Chowdhury, Avijit, Hafeez-Baig, Abdul and Gururajan, Raj. 2021. "Development of a survey instrument to explore telehealth adoption in the healthcare domain." Yamamoto, Sakae and Mori, Hirohiko (ed.) 23RD International Conference on Human-Computer Interaction (HCII 2021). Washington DC, United States 24 - 29 Jul 2021 Switzerland. https://doi.org/10.1007/978-3-030-78361-7_16Discovering dynamic Virtual Team determinants through an interpretivist philosophical framework
Woods, Ash, Hafeez-Baig, Abdul and McCubbin, Amanda. 2021. "Discovering dynamic Virtual Team determinants through an interpretivist philosophical framework." 31st Australasian Conference on Information Systems (ACIS 2020). Wellington, New Zealand 01 - 04 Dec 2020 Wellington, New Zealand.The Adoption of Mobile Technologies in Healthcare: The Perceptions of Healthcare Professionals Regarding Knowledge Management Practices in Developing Countries
Chowdhury, Avijit, Hafeez-Baig, Abdul, Gururajan, Raj and Sharif, Mirza Akmal. 2020. "The Adoption of Mobile Technologies in Healthcare: The Perceptions of Healthcare Professionals Regarding Knowledge Management Practices in Developing Countries." Stephanidis, Constantine, Salvendy, Gavriel, Wei, June, Yamamoto, Sakae, Mori, Hirohiko, Meiselwitz, Gabriele, Nah, Fiona Fui-Hoon and Siau, Keng (ed.) 22nd Human-Computer Interaction International Conference (HCI International 2020). Copenhagen, Denmark 19 - 24 Jul 2020 Switzerland. https://doi.org/10.1007/978-3-030-60152-2_5An exploratory study to understand the phenomena of eye-tracking technology: a case of the education environment
Syed, Ahmed, Chakraborty, Subrata, Hafeez-Baig, Abdul and Mandal, Anuradha. 2020. "An exploratory study to understand the phenomena of eye-tracking technology: a case of the education environment." Dann, Christopher Ewart and O'Neill, Shirley (ed.) Technology-enhanced formative assessment practices in higher education. United States. IGI Global. pp. 240-261Historical Virtual Team Learnings and a View to the New Normal Post-COVID-19
Woods, Ashley, Hafeez-Baig, Abdul and McCubbin, Amanda. 2022. "Historical Virtual Team Learnings and a View to the New Normal Post-COVID-19." 26th Pacific Asia Conference on Information Systems (PACIS 2022). Taipei, Taiwan 05 - 09 Jul 2022 Sydney, Australia.The potential influence of prior work experience on unfair dismissal arbitration decisions related to employee misconduct: an exploratory study of decision styles
Southey, Kim, Lynch, Bernadette, Rose, Dennis and Hafeez-Baig, Abdul. 2023. "The potential influence of prior work experience on unfair dismissal arbitration decisions related to employee misconduct: an exploratory study of decision styles ." Asia Pacific Journal of Human Resources. 61 (3), pp. 582-612. https://doi.org/10.1111/1744-7941.12366Factors influencing the adoption of electronic health records in the Australian environment
Ouheda, Salem, Hafeez-Baig, Abdul, Chakraborty, Subrata and Gururajan, Raj. 2019. "Factors influencing the adoption of electronic health records in the Australian environment." Kerr, Don, Blake, Jacqueline, Wang, Mingzhong and Tham, Aaron (ed.) 24th Annual Conference of the Asia Pacific DecisionSciences Institute: Technology Supporting People and Decision Making. Brisbane, Australia 15 - 18 Jul 2019 Brisbane, QLD, Australia.Towards effective knowledge application capability in ITSM through socialisation, externalisation, internalisation and combination
Fathi, Abdulazeez, Hafeez-Baig, Abdul and Gururajan, Raj. 2019. "Towards effective knowledge application capability in ITSM through socialisation, externalisation, internalisation and combination." Kerr, Don, Blake, Jacqueline, Wang, Mingzhong and Tham, Aaron (ed.) 24th Annual Conference of the Asia Pacific DecisionSciences Institute: Technology Supporting People and Decision Making. Brisbane, Australia 15 - 18 Jul 2019 Brisbane, QLD, Australia.An investigation into usability of big data analytics in the management of Type 2 Diabetes Mellitus
Bhotta, Dinakar, Hafeez-Baig, Abdul, Gururajan, Raj, Chakraborty, Subrata, Kavuri, Srinivas P. and Krishnan, Dharini. 2019. "An investigation into usability of big data analytics in the management of Type 2 Diabetes Mellitus." Kerr, Don, Blake, Jacqueline, Wang, Mingzhong and Tham, Aaron (ed.) 24th Annual Conference of the Asia Pacific DecisionSciences Institute: Technology Supporting People and Decision Making. Brisbane, Australia 15 - 18 Jul 2019 Brisbane, QLD, Australia.An exploratory qualitative research to address processes that are utilised for managing knowledge: a case study in a Queensland regional University
Mohammed, Atheer Abdullah, Hafeez-Baig, Abdul and Gururajan, Raj. 2019. "An exploratory qualitative research to address processes that are utilised for managing knowledge: a case study in a Queensland regional University." International Journal of Higher Education and Sustainability. 2 (3), pp. 173-196. https://doi.org/10.1504/IJHES.2019.101954Business model experimentation through technology and management innovation using cloud computing
Heaton, John, Hafeez-Baig, Abdul and Gururajan, Raj. 2019. "Business model experimentation through technology and management innovation using cloud computing." Kerr, Don, Blake, Jacqueline, Wang, Mingzhong and Tham, Aaron (ed.) 24th Annual Conference of the Asia Pacific DecisionSciences Institute: Technology Supporting People and Decision Making. Brisbane, Australia 15 - 18 Jul 2019 Brisbane, QLD, Australia.Understanding factors influencing adoption of mobile devices in telehealth: a quantitative study
Rani, Vasundhara, Hafeez-Baig, Abdul and Gururajan, Raj. 2019. "Understanding factors influencing adoption of mobile devices in telehealth: a quantitative study." 24th Asia Pacific DSI Conference Program (APDSI 2019). Brisbane, Australia 15 - 18 Jul 2019Conceptual framework for telehealth adoption in Indian healthcare
Chowdhury, Avijit, Hafeez-Baig, Abdul, Gururajan, Raj and Chakraborty, Subrata. 2019. "Conceptual framework for telehealth adoption in Indian healthcare." 24th Asia Pacific DSI Conference Program (APDSI 2019). Brisbane, Australia 15 - 18 Jul 2019A qualitative research to explore practices that are utilised for managing talent development in the higher education environment: a case study in six Australian universities
Mohammed, Atheer Abdullah, Hafeez-Baig, Abdul and Gururajan, Raj. 2019. "A qualitative research to explore practices that are utilised for managing talent development in the higher education environment: a case study in six Australian universities." Journal of Industry-University Collaboration. 1 (1), pp. 24-37. https://doi.org/10.1108/JIUC-02-2019-003Talent management as a core source of innovation and social development in higher education
Mohammed, Atheer Abdullah, Hafeez-Baig, Abdul and Gururajan, Raj. 2018. "Talent management as a core source of innovation and social development in higher education." Parrish, Dominique (ed.) Innovations in higher education: cases on transforming and advancing practice. London, United Kingdom. IntechOpen. pp. 1-31A qualitative research to explore processes that are utilized for managing talent: a case study in a Queensland regional university University
Mohammed, Atheer Abdullah, Hafeez-Baig, Abdul and Gururajan, Raj. 2018. "A qualitative research to explore processes that are utilized for managing talent: a case study in a Queensland regional university University." Australian Academy of Business and Economics Review. 4 (3), pp. 188-200.Understanding the factors that influence brand-image of a business school brand: a recruiters prospective
Kavuri, Srinivas Phani, Hafeez-Baig, Abdul, Gururajan, Raj and Bhotta, Dinakar. 2019. "Understanding the factors that influence brand-image of a business school brand: a recruiters prospective." Kerr, Don, Blake, Jacqueline, Wang, Mingzhong and Tham, Aaron (ed.) 24th Annual Conference of the Asia Pacific DecisionSciences Institute: Technology Supporting People and Decision Making. Brisbane, Australia 15 - 18 Jul 2019 Brisbane, QLD, Australia.Role of Accessory Right Inferior Hepatic Veins in evaluation of Liver Transplantation
Ahmed, Awais, Hafeez-Baig, Abdul, Sharif, Mirza Akmal, Ahmed, Umair, Ahmed, Shozab and Gururajan, Raj. 2017. "Role of Accessory Right Inferior Hepatic Veins in evaluation of Liver Transplantation." Annals of Clinical Gastroenterology and Hepatology. 1 (1), pp. 12-16. https://doi.org/10.29328/journal.acgh.1001004Consumer engagement with social media marketing in Australia
Deo, Kirtika, Chimhundu, Ranga and Hafeez-Baig, Abdul. 2018. "Consumer engagement with social media marketing in Australia." 2018 International Congress on Banking, Economics, Finance and Business (BEFB- Sydney 2018). Sydney, Australia 17 - 19 Dec 2018Exploring processes that are used for managing knowledge in the higher education environment: a case study in a Queensland regional university
Mohammed, Atheer Abdullah, Hafeez-Baig, Abdul and Gururajan, Raj. 2018. "Exploring processes that are used for managing knowledge in the higher education environment: a case study in a Queensland regional university." International Journal of Business and Economic Affairs. 3 (2), pp. 73-90. https://doi.org/10.24088/IJBEA-2018-32004e-Health readiness assessment factors and measuring tools: a systematic review
Yusif, Salifu, Hafeez-Baig, Abdul and Soar, Jeffrey. 2017. "e-Health readiness assessment factors and measuring tools: a systematic review." International Journal of Medical Informatics. 107, pp. 56-64. https://doi.org/10.1016/j.ijmedinf.2017.08.006Primarily investigating into the relationship between talent management and knowledge management in business environment
Mohammed, Atheer Abdullah, Gururajan, Raj and Hafeez-Baig, Abdul. 2017. "Primarily investigating into the relationship between talent management and knowledge management in business environment." 2017 IEEE/WIC/ACM International Conference on Web Intelligence (WI 2017). Leipzig, Germany 23 - 26 Aug 2017 New York, United States. https://doi.org/10.1145/3106426.3109444Objective Analysis of Marker Bias in Higher Education
Chakraborty, Subrata, Zhou, Susan, Hafeez-Baig, Abdul, Gururajan, Raj, Paul, Manoranjan, Mandal, Anuradha, Chacko, Anila Elizabeth and Barua, Prabal D.. 2017. "Objective Analysis of Marker Bias in Higher Education." 5th IEEE International Conference on Teaching, Assessment, and Learning for Engineering (TALE 2016). Bangkok, Thailand 07 - 09 Dec 2016 Piscataway, United States. https://doi.org/10.1109/TALE.2016.7851839What factors determine healthcare professionals’ (HCPs) acceptance of mobile devices for telehealth: a qualitative study conducted in Queensland, Australia
Rani Sood, Vasundhara, Hafeez Baig, Abdul and Gururajan, Raj. 2016. "What factors determine healthcare professionals’ (HCPs) acceptance of mobile devices for telehealth: a qualitative study conducted in Queensland, Australia." 2nd National Rural & Remote Telehealth Conference. Toowoomba, Australia 05 - 06 Oct 2016 Toowoomba, Australia.What factors determine healthcare professionals’ (HCPs) acceptance of mobile devices for telehealth: a qualitative study conducted in Queensland, Australia
Rani Sood, Vasundhara, Gururajan, Raj and Hafeez Baig, Abdul. 2016. "What factors determine healthcare professionals’ (HCPs) acceptance of mobile devices for telehealth: a qualitative study conducted in Queensland, Australia." 2nd National Rural & Remote Telehealth Conference. Toowoomba, Australia 05 - 06 Oct 2016 Toowoomba, Australia.Adoption of mobile devices in the Australian healthcare: a conceptual framework approach
Rani Sood, Vasundhara, Gururajan, Raj, Hafeez-Baig, Abdul and Wickramasinghe, Nilmini. 2017. "Adoption of mobile devices in the Australian healthcare: a conceptual framework approach." Wickramasinghe, Nilmini (ed.) Handbook of research on healthcare administration and management. Hershey, PA, United States. IGI Global. pp. 662-685An experimental analysis of assessor specific bias in a programming assessment in multi-assessor scenarios utilizing an eye tracker
Debnath, Tanmoy, Paul, Manoranjan, Chakraborty, Subrata, Podder, Pallab Kanti, Gururajan, Raj and Hafeez-Baig, Abdul. 2016. "An experimental analysis of assessor specific bias in a programming assessment in multi-assessor scenarios utilizing an eye tracker." Shoniregun, Charles A. and Akmayeva, Galyna A. (ed.) London International Conference on Education (LICE-2016). London, United Kingdom 14 - 17 Nov 2016 London, United Kingdom.Assuring reliability in qualitative studies: a health informatics persective
Hafeez-Baig, Abdul, Gururajan, Raj and Chakraborty, Subrata. 2016. "Assuring reliability in qualitative studies: a health informatics persective." 20th Pacific Asia Conference on Information Systems (PACIS 2016). Chiayi, Taiwan 27 Jun - 01 Jul 2016 Chiayi, Taiwan.The impact of diet counselling on Type 2 Diabetes Mellitus: an Indian case study
Krishnan, Dharini, Gururajan, Raj, Hafeez-Baig, Abdul, Kondalasamy-Chennakesavan, Srinivas, Wickramasinghe, Nilmini and Gururajan, Rashmi. 2015. "The impact of diet counselling on Type 2 Diabetes Mellitus: an Indian case study." Journal of Diabetes and Metabolism. 6 (10). https://doi.org/10.4172/2155-6156.1000610Study to investigate factors influencing adoption of mobile devices in the health care environment
Rani Sood, Vasundhara, Hafeez-Baig, Abdul and Gururajan, Raj. 2016. "Study to investigate factors influencing adoption of mobile devices in the health care environment." European Conference on Information Systems (ECIS 2016). Istanbul, Turkey 12 - 15 Jun 2016Title of PhD project: study to investigate factors influencing adoption of mobile devices in the health care environment
Rani Sood, Vasundhara, Gururajan, Raj and Hafeez Baig, Abdul. 2015. "Title of PhD project: study to investigate factors influencing adoption of mobile devices in the health care environment." 26th Australasian Conference on Information Systems (ACIS 2015). Adelaide, Australia 30 Nov - 04 Dec 2015 Adelaide, South Australia.Older people, assistive technologies, and the barriers to adoption: a systematic review
Yusif, Salifu, Soar, Jeffrey and Hafeez-Baig, Abdul. 2016. "Older people, assistive technologies, and the barriers to adoption: a systematic review." International Journal of Medical Informatics. 94, pp. 112-116. https://doi.org/10.1016/j.ijmedinf.2016.07.004Exercise, Diet Counselling, and Management for the Adults with Diabetes Mellitus: An Indian Case Study
Pathy, Dharnini, Gururajan, Raj, Hafeez-Baig, Abdul, Wickramasinghe, Nilmini and Gururajan, Rashmi. 2016. "Exercise, Diet Counselling, and Management for the Adults with Diabetes Mellitus: An Indian Case Study ." Wickramasignhe, Nilmini, Troshani, Indrit and Tan, Joseph (ed.) Contemporary consumer health informatics. Switzerland. Springer. pp. 15-52Reliability of qualitative data using text analysis: a Queensland Health case study
Gururajan, Raj, Clark, Kevin, Moller, Suan, Sankaran, Prema, Hafeez Baig, Abdul, Wickramasinghe, Nilmini and Gururajan, Rashmi. 2016. "Reliability of qualitative data using text analysis: a Queensland Health case study." Wickramasinghe, Nilmini, Troshani, I. and Tan, J. (ed.) Contemporary Consumer Health Informatics. Switzerland. Springer. pp. 1-13An exploratory qualitative study to identify factors that influence the use of electronic patient journey boards in Queensland health
Gururajan, Raj, Hafeez Baig, Abdul, Sturgess, Julie, Clark, Kevin and Gururajan, Vijaya. 2015. "An exploratory qualitative study to identify factors that influence the use of electronic patient journey boards in Queensland health." Electronic Journal of Health Informatics. 9 (1).How do students use their mobile devices to support learning? A case study from an Australian regional university
Farley, Helen, Murphy, Angela, Johnson, Chris, Carter, Brad, Lane, Michael, Midgley, Warren, Hafeez-Baig, Abdul, Dekeyser, Stijn and Koronios, Andy. 2015. "How do students use their mobile devices to support learning? A case study from an Australian regional university." Journal of Interactive Media in Education. 15 (1), pp. 1-13. https://doi.org/10.5334/jime.arMoving towards the effective evaluation of mobile learning initiatives in higher education institutions
Farley, Helen, Murphy, Angela, Todd, Nicole Ann, Lane, Michael, Hafeez-Baig, Abdul, Midgley, Warren and Johnson, Chris. 2015. "Moving towards the effective evaluation of mobile learning initiatives in higher education institutions." Zhang, Yu (Aimee) (ed.) Handbook of mobile teaching and learning. Germany. Springer. pp. 721-740Mobile telehealth technology assessment: Queensland case study
Gururajan, Raj, Little, Alison, Hafeez Baig, Abdul, Chakraborty, Subrata and Wickramasignhe, Nilmini. 2015. "Mobile telehealth technology assessment: Queensland case study." 23rd Australian National Health Informatics Conference (HIC 2015). Brisbane, Australia 03 - 05 Aug 2015 Australia.Health text analysis: a Queensland Health case study
Gururajan, Raj, Hafeez-Baig, Abdul, Clark, Kevin, Moller, Susan and Sankaran, Prema. 2014. "Health text analysis: a Queensland Health case study." Mathirajan, M. (ed.) 2nd International Conference on Business Analytics and Intelligence (ICBAI 2014). Bangalore, India 18 - 20 Dec 2014 Bangalore, India.Reliability of qualitative data using text analysis: a Queensland Health case study
Gururajan, Raj, Hafeez-Baig, Abdul, Clark, Kevin, Moller, Susan and Sankaran, Prema. 2014. "Reliability of qualitative data using text analysis: a Queensland Health case study." 3rd International Conference on Eco-friendly Computing and Communication Systems (ICECCS 2014): Computing and Communication for Sustainable Development. Mangalore, India 18 - 21 Dec 2014 Piscataway, NJ. United States.Handheld wireless devices and opinions of physicians in healthcare environment: a case of Pakistan
Hafeez-Baig, Abdul and Gururajan, Raj. 2014. "Handheld wireless devices and opinions of physicians in healthcare environment: a case of Pakistan." 25th Australasian Conference on Information Systems (ACIS 2014). Auckland, New Zealand 08 - 10 Dec 2014 Palmerston North, New Zealand.An exploratory case study on employee health and wellbeing: Indian railways case study
Gururajan, Raj, Hafeez-Baig, Abdul, Chennakesav, Srinivas, Thomas, Anil, Prakash, Ram, Krishnan, Dharini and Sankaran, Prema. 2014. "An exploratory case study on employee health and wellbeing: Indian railways case study." Journal of Information Technology & Software Engineering. 4 (1), p. 125. https://doi.org/10.4172/2165-7866.1000125Mobile learning anytime, anywhere: what are our students doing?
Murphy, Angela, Farley, Helen, Lane, Michael, Hafeez-Baig, Abdul and Carter, Brad. 2014. "Mobile learning anytime, anywhere: what are our students doing?" Australasian Journal of Information Systems. 18 (3), pp. 331-345.An empirical study to determine factors that motivate and limit the implementation of ICT in healthcare environment
Gururajan, Raj and Hafeez-Baig, Abdul. 2014. "An empirical study to determine factors that motivate and limit the implementation of ICT in healthcare environment." BMC Medical Informatics and Decision Making. 14 (98), pp. 1-8. https://doi.org/10.1186/1472-6947-14-98An exploratory study to identify factors that influence the use of electronic patient boards in Queensland Health
Gururajan, Raj, Hafeez-Baig, Abdul, Sturgess, Julie, Clark, Kevin, Gururajan, Vijaya and Moller, Susan. 2013. "An exploratory study to identify factors that influence the use of electronic patient boards in Queensland Health." International Journal of Management & Information Technology. 7 (2), pp. 1033-1041.Listening to the student voice: how are students really using mobile technologies for learning?
Murphy, Angela, Farley, Helen, Johnson, Chris, Lane, Michael, Carter, Brad, Hafeez-Baig, Abdul, Midgley, Warren, Dekeyser, Stijn, Rees, Sharon, Mitchell, Maxine, Doyle, Joanne and Koronios, Andy. 2013. "Listening to the student voice: how are students really using mobile technologies for learning?" Carter, Helen, Gosper, Maree and Hedberg, John (ed.) 30th Annual Conference of the Australasian Society for Computers in Learning in Tertiary Education (ASCILITE 2013). Sydney, Australia 01 - 04 Dec 2013An exploration of access to and use of mobile technologies for learning activities among higher education students
Murphy, Angela, Farley, Helen, Midgley, Warren, Carter, Brad, Lane, Michael and Hafeez-Baig, Abdul. 2013. "An exploration of access to and use of mobile technologies for learning activities among higher education students." Research Colloquia and Showcase 2013. Springfield, Australia 12 - 13 Jun 2013 Queensland, Australia.Critical role of 'T-shaped skills & incentive rewards' as determinants for knowledge management enablers: a case of Indian study
Hafeez-Baig, Abdul and Gururajan, Raj. 2012. "Critical role of 'T-shaped skills & incentive rewards' as determinants for knowledge management enablers: a case of Indian study." Kalloniatis, Christos (ed.) Modern information systems. Rijeka, Croatia. InTech Europe. pp. 133-146Wireless technology and clinical influences in healthcare setting: an Indian case study
Hafeez-Baig, Abdul and Gururajan, Raj. 2011. "Wireless technology and clinical influences in healthcare setting: an Indian case study." Guah, Matthew (ed.) Healthcare delivery reform and new technologies: organizational initiatives. Hershey, PA. United States. IGI Global. pp. 55-74An empirical study to determine factors that motivate and limit the implementation of ICT in healthcare environments
Hafeez-Baig, Abdul and Gururajan, Raj. 2013. "An empirical study to determine factors that motivate and limit the implementation of ICT in healthcare environments." Deng, Hepu and Standing, Craig (ed.) 24th Australasian Conference on Information Systems (ACIS 2013). Melbourne, Australia 04 - 06 Dec 2013 Melbourne, Australia.Mobile learning anytime, anywhere: what are our students doing?
Murphy, Angela, Farley, Helen, Lane, Michael, Hafeez-Baig, Abdul and Carter, Brad. 2013. "Mobile learning anytime, anywhere: what are our students doing?" Aisyah, Hepu and Standing, Craig (ed.) 24th Australasian Conference on Information Systems (ACIS 2013). Melbourne, Australia 04 - 06 Dec 2013 Melbourne, Australia.Role of wireless handheld technology by nurses in healthcare environment
Hafeez-Baig, Abdul and Gururajan, Raj. 2012. "Role of wireless handheld technology by nurses in healthcare environment." Computer Technology and Application. 3 (8), pp. 550-557.Acoustical design of digital stethoscope for improved performance
Thiagarajan, Raj C., Sankaran, Prema, Hafeez-Baig, Abdul, Elangovan, Kodeeswari and Gururajan, Raj. 2012. "Acoustical design of digital stethoscope for improved performance." Singh, Lakhvinder (ed.) COMSOL Conference India 2012. Bangalore, India 02 - 03 Nov 2012 Burlington, MA. United States.Does information and communication technology (ICT) facilitate knowledge management activities in the 21st century?
Hafeez-Baig, Abdul and Gururajan, Raj. 2012. "Does information and communication technology (ICT) facilitate knowledge management activities in the 21st century?" Journal of Software. 7 (11), pp. 2437-2442. https://doi.org/10.4304/jsw.7.11.2437-2442Wireless technology and job performance attributes in a healthcare environment
Hafeez-Baig, Abdul and Gururajan, Raj. 2012. "Wireless technology and job performance attributes in a healthcare environment." Su, Ying (ed.) 7th International Conference on Cooperation and Promotion of Information Resources in Science and Technology (COINFO 2012): Information Sharing in the Cloud. Nanjing, China 23 - 25 Nov 2012 Irvine, CA. United States.Expectations, usability, and job satisfaction as determinants for the perceived benefits for the use of wireless technology in healthcare
Hafeez-Baig, Abdul and Gururajan, Raj. 2013. "Expectations, usability, and job satisfaction as determinants for the perceived benefits for the use of wireless technology in healthcare." Bali, Rajeev K., Troshani, Indrit, Goldberg, Steve and Wickramasinghe, Nilmini (ed.) Pervasive health knowledge management. New York, NY. United States. Springer. pp. 305-316Phenomena of adoption of wireless handheld devices: a case of healthcare setting
Hafeez-Baig, Abdul and Gururajan, Raj. 2013. "Phenomena of adoption of wireless handheld devices: a case of healthcare setting." Muga, Helen E. and Thomas, Ken D. (ed.) Cases on the diffusion and adoption of sustainable development practices. Hershey, PA. United States. IGI Global. pp. 20-43Feeding back and enhancing authentic learning in quality course assessment design: locating information systems education in rigorous educational research
Hafeez-Baig, Abdul, Gururajan, Raj, Jones, David and Danaher, P. A.. 2012. "Feeding back and enhancing authentic learning in quality course assessment design: locating information systems education in rigorous educational research." Lamp, John (ed.) 23rd Australasian Conference on Information Systems (ACIS 2012). Geelong, Australia 03 - 05 Dec 2012 Geelong, Australia.Principles and pressures in managing student attitudes to innovative mobile learning: a view from an Australian distance-education-intensive university
Hafeez-Baig, Abdul, Gururajan, Raj, Danaher, P. A. and De George-Walker, Linda. 2013. "Principles and pressures in managing student attitudes to innovative mobile learning: a view from an Australian distance-education-intensive university." International Journal of Management in Education. 7 (1/2), pp. 149-162. https://doi.org/10.1504/IJMIE.2013.050818Deploying mobile data services: an Australian case study
Tsai, Heng-Sheng, Gururajan, Raj, Kong, Eric, Hafeez-Baig, Abdul and Lin, Meng Kuan. 2012. "Deploying mobile data services: an Australian case study." IT Professional: technology solutions for the enterprise. 14 (5), pp. 22-27. https://doi.org/10.1109/MITP.2012.47Student perceptions and uses of wireless handheld devices: implications for implementing blended and mobile learning in an Australian university
Gururajan, Raj, Hafeez-Baig, Abdul, Danaher, P. A. and De George-Walker, Linda. 2011. "Student perceptions and uses of wireless handheld devices: implications for implementing blended and mobile learning in an Australian university." Kitchenham, Andrew (ed.) Models for interdisciplinary mobile learning: delivering information to students. Hershey, PA. United States. IGI Global. pp. 231-246Challenges and opportunities for academics adopting an online peer review innovation
Hafeez-Baig, Abdul, De George-Walker, Linda, Gururajan, Raj and Danaher, P. A.. 2011. "Challenges and opportunities for academics adopting an online peer review innovation." Studies in Learning Evaluation Innovation and Development. 8 (1), pp. 26-37.A confirmatory factor analysis on the views and constructs for knowledge management in India
Gururajan, Raj, Hafeez-Baig, Abdul and Tsai, Heng-Sheng. 2012. "A confirmatory factor analysis on the views and constructs for knowledge management in India." Computer Technology and Application. 3 (1), pp. 98-104.Pakistani study on the determinants of wireless technology in healthcare
Hafeez-Baig, Abdul and Gururajan, Raj. 2012. "Pakistani study on the determinants of wireless technology in healthcare." Computer Technology and Application. 3 (2), pp. 194-200.Preliminary study to investigation the determinants that effect IS/IT outsourcing
Hafeez-Baig, Abdul and Gururajan, Raj. 2011. "Preliminary study to investigation the determinants that effect IS/IT outsourcing." International Journal of Information and Communication Technology Research. 1 (2), pp. 48-54.An exploratory study to investigate the effectiveness of PDAs in healthcare: a case of Queensland nurses
Hafeez-Baig, Abdul and Gururajan, Raj. 2011. "An exploratory study to investigate the effectiveness of PDAs in healthcare: a case of Queensland nurses." 22nd Australasian Conference on Information Systems (ACIS 2011). Sydney, Australia 29 Nov - 02 Dec 2011 Atlanta, GA. United States.Clinicians perceptions towards wireless handheld devices: an Australian case study
Hafeez-Baig, Abdul and Gururajan, Raj. 2011. "Clinicians perceptions towards wireless handheld devices: an Australian case study." Su, Ying and Al-Hakim, Latif (ed.) COINFO 2011: 6th International Conference on Cooperation and Promotion of Information Resources in Science and Technology: Coordinative Innovation and Open Sharing. Hangzhou, China 12 - 14 Nov 2011 Irvine, CA, United States.Clinicians' perception of using digital stethoscopes in telehealth platform: Queensland telehealth preliminary study
Soroush, Layla, Hafeez-Baig, Abdul and Gururajan, Raj. 2010. "Clinicians' perception of using digital stethoscopes in telehealth platform: Queensland telehealth preliminary study." Rosemann, Michael (ed.) 21st Australasian Conference on Information Systems (ACIS 2010). Brisbane, Australia 01 - 03 Dec 2010 Brisbane, Australia.Study to investigate the adoption of wireless technology in the Australian healthcare system
Hafeez-Baig, Abdul. 2010. Study to investigate the adoption of wireless technology in the Australian healthcare system. PhD Thesis Doctor of Philosophy. University of Southern Queensland.Knowledge sharing and management for better organisational outcomes: an Indian study
Gururajan, Raj, Tsai, Heng-Sheng, Hafeez-Baig, Abdul and Lin, Meng-Kuan. 2010. "Knowledge sharing and management for better organisational outcomes: an Indian study." Chen, Hong-Mei and Lin, Fu-ren (ed.) 14th Pacific Asia Conference on Information Systems (PACIS 2010): Service Science in Information Systems Research. Taipei, Taiwan 09 - 12 Jul 2010 Kaohsiung, Taiwan.Exploratory study to explore the role of ICT in the process of knowledge management in an Indian business environment
Hafeez-Baig, Abdul, Gururajan, Raj, Heng Sheng, Tasi and Sankaran, Prema. 2010. "Exploratory study to explore the role of ICT in the process of knowledge management in an Indian business environment." Xiang, Yang, Samarati, Pierangela, Hu, Jiankun, Zhou, Wanlei and Sadeghi, Ahmad-Reza (ed.) NSS 2010: 4th International Conference on Network and System Security . Melbourne, Australia 01 - 03 Sep 2010 Los Alamitos, CA. United States. https://doi.org/10.1109/NSS.2010.89A web-based ECG decision support system using multi-touch function to support cardiac diagnosis
Lin, Meng-Kuan, Gururajan, Raj, Mula, Joseph M., Leis, John W. and Hafeez-Baig, Abdul. 2010. "A web-based ECG decision support system using multi-touch function to support cardiac diagnosis." 5th International Conference on Cooperation and Promotion of Information Resources in Science and Technology: Information Sharing in U-Era (COINFO'10). Beijing, China 27 - 29 Nov 2010Key common determinants for adoption of wireless technology in healthcare for India and Pakistan: development of a conceptual model
Hafeez-Baig, Abdul and Gururajan, Raj. 2010. "Key common determinants for adoption of wireless technology in healthcare for India and Pakistan: development of a conceptual model." Safran, Charles, Marin, Heimer F. and Reti, Shane R. (ed.) 13th World Congress on Medical and Health Informatics (MEDINFO 2010). Cape Town, South Africa 12 - 15 Sep 2010 Amsterdam, Netherlands. https://doi.org/10.3233/978-1-60750-588-4-342Wireless handheld device and LAN security issues: a case study
Gururajan, Raj and Hafeez-Baig, Abdul. 2011. "Wireless handheld device and LAN security issues: a case study." Kerr, Don, Gammack, John G. and Bryant, Kay (ed.) Digital business security development: management technologies. Hershey, PA, USA. IGI Global. pp. 129-151Development of a tele-stethoscope: Indian perspective
Sankaran, Prema, Chandrasekaran, K., Hafeez Baig, Abdul and Moll, Chris Lynton. 2010. "Development of a tele-stethoscope: Indian perspective." Li, Yan, Yang, Jiajia, Wen, Peng and Wu, Jinglong (ed.) 2010 IEEE/ICME International Conference on Complex Medical Engineering (ICME 2010). Gold Coast, Australia 13 - 15 Jul 2010 Piscataway, NJ. United States. https://doi.org/10.1109/ICCME.2010.5558838Experiences and perceptions of learner engagement in blended learning environments: the case of an Australian university
De George-Walker, Linda, Hafeez-Baig, Abdul, Gururajan, Raj and Danaher, Patrick Alan. 2010. "Experiences and perceptions of learner engagement in blended learning environments: the case of an Australian university." Inoue, Yukiko (ed.) Cases on online blended learning technologies in higher education: concepts and practices. Hershey, PA, USA. IGI Global. pp. 23-43Efficacy and feasibility of a mobile ECG decision support system - a preliminary conceptual model
Lin, Meng-Kuan, Mula, Joseph M., Gururajan, Raj, Leis, John W. and Hafeez-Baig, Abdul. 2010. "Efficacy and feasibility of a mobile ECG decision support system - a preliminary conceptual model." Chen, Houn-Gee and Liang, Ting-Peng (ed.) 14th Pacific Asia Conference on Information Systems (PACIS 2010): Service Science in Information Systems Research. Taipei, Taiwan 09 - 12 Jul 2010 Kaohsiung, Taiwan.An Exploratory Study to Understand the Drivers and Inhibitors for the Successful Adoption of Wireless Technology in Australian Healthcare Systems
Hafeez-Baig, Abdul and Gururajan, Raj. 2010. "An Exploratory Study to Understand the Drivers and Inhibitors for the Successful Adoption of Wireless Technology in Australian Healthcare Systems." Pease, Wayne, Cooper, Malcolm and Gururajan, Raj (ed.) Biomedical Knowledge Management: Infrastructures and Processes for E-Health Systems. Hershey, PA, USA. Medical Information Science Reference (IGI Global). pp. 267-278Selected influences on students' uses of wireless handheld devices: an Iranian exploratory case study
Hafeez-Baig, Abdul, Gururajan, Raj, Nazemi, Shamsodin, De George-Walker, Linda and Danaher, P. A.. 2010. "Selected influences on students' uses of wireless handheld devices: an Iranian exploratory case study." Abas, Zoraini Wati, Jung, Insung and Luca, Joseph (ed.) Global Learn Asia Pacific 2010. Penang, Malaysia 17 - 20 May 2010 Chesapeake, VA, United States.Views and constructs for knowledge management in India: a confirmatory factor analysis
Hafeez-Baig, Abdul, Gururajan, Raj and Tsai, Heng-Sheng. 2010. "Views and constructs for knowledge management in India: a confirmatory factor analysis." Chen, Houn-Gee and Liang, Ting-Peng (ed.) 14th Pacific Asia Conference on Information Systems (PACIS 2010): Service Science in Information Systems Research. Taipei, Taiwan 09 - 12 Jul 2010 Kaohsiung, Taiwan.A discussion on the role of knowledge management in aged care
Gururajan, Vijaya, Gururajan, Raj and Hafeez-Baig, Abdul. 2004. "A discussion on the role of knowledge management in aged care." Conrick, Moya and Soar, Jeffrey (ed.) 1st Australian Aged and Community Care Informatics Conference (ACCIC'04). Brisbane, Australia 24 - 25 Aug 2004 Brunswick East, Australia.Organizational, technical and perceived readiness as adoption factors for wireless technology in healthcare: an Australian case study
Hafeez-Baig, Abdul and Gururajan, Raj. 2009. "Organizational, technical and perceived readiness as adoption factors for wireless technology in healthcare: an Australian case study." Sintchenko, Vitali and Croll, Peter (ed.) Health Informatics Conference (HIC 2009): Frontiers of Health Informatics - Redefining Healthcare. Canberra, Australia 19 - 21 Aug 2009 Melbourne, Australia.The trial of PDAs in Queensland public hospitals and its effect on patient safety
Bruggemann, Glenn, Gurney, Tiana, Hafeez-Baig, Abdul, March, Judy and Weale, Greg. 2009. "The trial of PDAs in Queensland public hospitals and its effect on patient safety." 2nd Passionate about Practice Conference. Brisbane, Australia 29 - 30 Jul 2009The practical issues in using existing digital stethoscopes for telehealth
Gurney, Tiana, Stenhouse, Shayne, Gururajan, Raj and Hafeez-Baig, Abdul. 2009. "The practical issues in using existing digital stethoscopes for telehealth." Annual International Conference on Successes and Failures in Telehealth 2009 (SFT-09). Brisbane, Australia 10 - 11 Dec 2009 Brisbane, Australia.Confirmatory factor analysis to establish determinants of wireless technology in the Indian healthcare
Gururajan, Raj, Gurney, Tiana and Hafeez-Baig, Abdul. 2009. "Confirmatory factor analysis to establish determinants of wireless technology in the Indian healthcare." International Journal of Healthcare Delivery Reform Initiatives. 1 (3), pp. 19-37.Study to investigate the determinants for the use of wireless technology in healthcare setting: a case of Pakistan
Hafeez-Baig, Abdul, Gururajan, Raj, Mula, Joseph M. and Lin, Meng-Kuan. 2009. "Study to investigate the determinants for the use of wireless technology in healthcare setting: a case of Pakistan." Peng, Jie, Al-Hakim, Latif and Chen, Guoqing (ed.) COINFO 2009: Managing Virtual Organisations and Information in the Era of Globalisation. Beijing, China 21 - 23 Nov 2009 Los Alamitos, CA. United States. https://doi.org/10.1109/COINFO.2009.52Legal and regulatory issues of implementation of electronic signatures
Gururajan, Raj and Hafeez-Baig, Abdul. 2004. "Legal and regulatory issues of implementation of electronic signatures." 2004 AusCERT: Computer Security: Are we there yet?. Gold Coast, Australia 23 - 27 May 2004 Brisbane, Australia.Implementation difficulties of electronic signatures in the m-commerce environment
Gururajan, Raj and Hafeez-Baig, Abdul. 2004. "Implementation difficulties of electronic signatures in the m-commerce environment." 3rd International Conference on Information and Management Sciences (IMS2004). Dunhuang, China 05 - 10 Jun 2004 Beijing, China.Exploratory study to investigate the applicability of existing digital stethoscopes in a telehealth setting
Gurney, T., Hafeez-Baig, A. and Gururajan, R.. 2009. "Exploratory study to investigate the applicability of existing digital stethoscopes in a telehealth setting." Neelakanta, P. S. (ed.) OCOBIAHC 2009: International Conference on Biomedical Instrumentation and Healthcare Engineering. Chennai, India 06 - 08 Aug 2009 Piscataway, NJ, USA.Trialling wireless handheld devices in a clinical setting: a Queensland case study
Gurney, Tiana, Hafeez-Baig, Abdul and Gururajan, Raj. 2009. "Trialling wireless handheld devices in a clinical setting: a Queensland case study." Dhillon, Gurpreet (ed.) CISTM 2009: 7th Annual Conference on Information Science, Technology and Management. Gurgaon, India 13 - 15 Jul 2009 Finland.Exploratory study to understand the phenomena of adoption of wireless handheld devices in the Australian healthcare system
Hafeez-Baig, Abdul and Gururajan, Raj. 2009. "Exploratory study to understand the phenomena of adoption of wireless handheld devices in the Australian healthcare system." Journal of Systems and Information Technology. 11 (1), pp. 43-56. https://doi.org/10.1108/13287260910932403Online learning with peer-review: an initial study
Gururajan, Raj, Hafeez-Baig, Abdul and Khan, Shamim. 2008. "Online learning with peer-review: an initial study." 2008 World Conference on E-Learning in Corporate, Government, Healthcare & Higher Education (E-Learn 2008). Las Vegas, United States of America 17 - 21 Nov 2008 Chesapeake, VA, United States.Time management, improved patient expectations and added value contributing to the perceived acceptance of using wireless hand held devices in the Indian healthcare
Hafeez-Baig, Abdul and Gururajan, Raj. 2008. "Time management, improved patient expectations and added value contributing to the perceived acceptance of using wireless hand held devices in the Indian healthcare." Mills, Annette and Huff, Sid (ed.) 19th Australasian Conference on Information Systems (ACIS 2008). Christchurch, New Zealand 03 - 05 Dec 2008 Christchurch, New Zealand.Pakastani healthcare professionals views and opinions about use of wireless handheld devices in healthcare environment
Gururajan, Raj, Hafeez-Baig, Abdul and Kerr, Don. 2008. "Pakastani healthcare professionals views and opinions about use of wireless handheld devices in healthcare environment." Electronic Journal of Health Informatics. 3 (2), pp. 1-10.Transforming the practice of mobile learning: promoting pedagogical innovation through educational principles and strategies that work
Danaher, Patrick, Gururajan, Raj and Hafeez-Baig, Abdul. 2009. "Transforming the practice of mobile learning: promoting pedagogical innovation through educational principles and strategies that work." Hokyoung, Ryu and Parsons, D. (ed.) Innovative mobile learning: techniques and technologies. Hershey PA, USA. IGI Global. pp. 21-46Clinical factors and technological barriers as determinants for the intention to use wireless handheld technology in healthcare environment: an Indian case study
Gururajan, Raj, Gururajan, Vijaya and Hafeez-Baig, Abdul. 2008. "Clinical factors and technological barriers as determinants for the intention to use wireless handheld technology in healthcare environment: an Indian case study." Willie, Golden, Thomas, Acton and Kieran, Conboy (ed.) 16th European Conference on Information Systems: Information Systems in an Innovative Knowledge-based Society (ECIS 2008). Galway, Ireland 09 - 11 Jun 2008 Galway, Ireland.Challenges and opportunities in facilitating student engagement and empowerment: perspectives from information systems and education courses at the University of Southern Queensland, Australia
Hafeez-Baig, Abdul. 2007. "Challenges and opportunities in facilitating student engagement and empowerment: perspectives from information systems and education courses at the University of Southern Queensland, Australia." Montgomerie, Craig and Seale, Jane (ed.) ED-MEDIA 2007: World Conference on Educational Multimedia, Hypermedia and Telecommunications 2007. Vancouver, Canada 25 - 29 Jun 2007 Chesapeake, VA, Canada.An exploratory study to identify the determinants of the wireless handheld devices in higher education: a case of USQ
Hafeez-Baig, Abdul. 2007. "An exploratory study to identify the determinants of the wireless handheld devices in higher education: a case of USQ." Montgomerie, Craig and Seale, Jane (ed.) ED-MEDIA 2007: World Conference on Educational Multimedia, Hypermedia and Telecommunications 2007. Vancouver, Canada 25 - 29 Jun 2007 Chesapeake, VA, United States.Reactions and perceptions of healthcare professional towards wireless devices in healthcare environment in the developing world: a case of Pakistan
Gururajan, Raj, Hafeez-Baig, Abdul and Kerr, Don. 2007. "Reactions and perceptions of healthcare professional towards wireless devices in healthcare environment in the developing world: a case of Pakistan." Toleman, Mark, Cater-Steel, Aileen and Roberts, Dave (ed.) 18th Australasian Conference on Information Systems (ACIS 2007). Toowoomba, Australia 05 - 07 Dec 2007 Toowoomba, Australia.Global implementation of digital stethoscope for telehealth or telemedicine: an Australian case study
Hafeez-Baig, Abdul, Scott, Annette, Sarquis, Colleen, Cockfield, Glenys and Gururajan, Raj. 2007. "Global implementation of digital stethoscope for telehealth or telemedicine: an Australian case study." 5th International Symposium on Management of Technology (ISMOT 2007). Hangzhou, China 01 - 03 Jun 2007 China.Clinical usefulness is the key common determinant of adoption of wireless technology in healthcare for India and Australia
Grist, Shelly, Hafeez-Baig, Abdul, Gururajan, Raj and Khan, Shamim. 2007. "Clinical usefulness is the key common determinant of adoption of wireless technology in healthcare for India and Australia." Archer, Norm, Hassanein, Khaled and Yuan, Yufei (ed.) 6th International Conference on the Management of Mobile Business (ICMB 2007). Toronto, Canada 09 - 11 Jul 2007 Washington, United States.Technology management, data management, improved outcomes, efficiency and software limitation influencing the use of wireless technology for healthcare in Pakistan
Hafeez-Baig, Abdul, Grist, Shelly and Gururajan, Raj. 2007. "Technology management, data management, improved outcomes, efficiency and software limitation influencing the use of wireless technology for healthcare in Pakistan." Lee, Roger, Chowdhury, Morshed U., Ray, Sid and Lee, Thuy (ed.) 6th IEEE/ACIS International Conference on Computer and Information Science (ICIS 2007). Melbourne, Australia 11 - 13 Jul 2007 Los Alamitos, CA. United States. https://doi.org/10.1109/ICIS.2007.177Meanings emerging in the practice of wireless technologies: findings from two academic focus groups at the University of Southern Queensland
Danaher, Patrick Alan and Hafeez-Baig, Abdul. 2007. "Meanings emerging in the practice of wireless technologies: findings from two academic focus groups at the University of Southern Queensland." Fogarty, Roderick (ed.) 3rd International Conference on Pedagogies and Learning. Brisbane, Australia 26 - 28 Sep 2007 Toowoomba, Australia.An exploratory study of mobile learning for tertiary education: a discussion with students
Hafeez-Baig, Abdul, Gururajan, Raj and Gururajan, Vijaya. 2006. "An exploratory study of mobile learning for tertiary education: a discussion with students." International Journal of Pedagogies and Learning. 2 (1), pp. 76-88.Future possibilities for mobile learning technologies and applications at the University of Southern Queensland, Australia: lessons from an academic focus group
Hafeez-Baig, Abdul and Danaher, Patrick Alan. 2007. "Future possibilities for mobile learning technologies and applications at the University of Southern Queensland, Australia: lessons from an academic focus group." Parsons, D. and Ryu, Hokyoung (ed.) 1st International Conference on Mobile Learning Technologies and Applications. Auckland, New Zealand 19 Feb 2007 Auckland, New Zealand.A framework for the adoption of wireless technology in healthcare: an Indian study
Howard, Angela, Hafeez-Baig, Abdul, Howard, Srecko and Gururajan, Raj. 2006. "A framework for the adoption of wireless technology in healthcare: an Indian study." Spencer, S. and Jenkins, A. (ed.) 17th Australasian Conference on Information Systems (ACIS 2006). Adelaide, Australia 06 - 08 Dec 2006 Australia.Wireless technologies and lifelong learning pedagogies: institutional challenges and opportunities at the University of Southern Queensland
Hafeez-Baig, Abdul and Danaher, Patrick Alan. 2006. "Wireless technologies and lifelong learning pedagogies: institutional challenges and opportunities at the University of Southern Queensland." Orr, Debbie, Nouwens, Fons, Macpherson, Colin, Harreveld, R. E. (Bobby) and Danaher, Patrick Alan (ed.) 4th International Lifelong Learning Conference: Lifelong Learning: Partners, Pathways and Pedagogies. Yeppoon, Australia 13 - 16 Jun 2006 Rockhampton, Australia.Preliminary investigation to explore perceptions of security issues associated with wireless technology in healthcare in Australia
Tiong, I. C., Hafeez-Baig, Abdul, Gururajan, Raj and Soar, Jeffrey. 2006. "Preliminary investigation to explore perceptions of security issues associated with wireless technology in healthcare in Australia." Westbrook, J. and Callen, J. (ed.) Health Infomatics Conference 2006: Bridging the Digital Divide: Clinician, Consumer and Computer (HIC 2006). Sydney, Australia 20 - 22 Aug 2006 Melbourne, Australia.Wireless ehealth applications: are we on the right track?
Gururajan, Raj and Hafeez-Baig, Abdul. 2005. "Wireless ehealth applications: are we on the right track?" Isaias, Pedro, Nunes, Miguel Baptista and Reis, Antonio Palma dos (ed.) IADIS Virtual Multi Conference on Computer Science and Information Systems 2005 (IADIS 2005). Virtual 11 - 29 Apr 2005 United States.Adoption of wireless handheld technology: a case of Queensland healthcare
Gururajan, Raj, Hafeez-Baig, Abdul and Moloney, Clint. 2005. "Adoption of wireless handheld technology: a case of Queensland healthcare." Yeung, Jeff (ed.) 5th International Conference on Electronic Business: Enhancing Logistics & Supply Chain Competence Through E-Business (ICEB 2005). Hong Kong, China 05 - 09 Dec 2005 Hong Kong, China.Legal issues and risks impacting wireless workforce
Hafeez-Baig, Abdul and Gururajan, Raj. 2005. "Legal issues and risks impacting wireless workforce." Li, Eldon (ed.) APDSI 2005. Taipei, Taiwan 28 Jun - 02 Jul 2005 Taiwan.An exploratory study to determine factors impacting outsourcing of information systems in healthcare
Hafeez-Baig, Abdul and Gururajan, Raj. 2005. "An exploratory study to determine factors impacting outsourcing of information systems in healthcare." Eldon, Li (ed.) APDSI 2005. Taipei, Taiwan 28 Jun - 02 Jul 2005 Taiwan.Easing into mobile learning
Murphy, Angela and Farley, Helen. 2015. "Easing into mobile learning." Reiners, Torsten, Von Konsky, B. R., Gibson, D., Chang, V., Irving, L. and Clarke, K. (ed.) 32nd Annual Conference of the Australasian Society for Computers in Learning in Tertiary Education (ASCILITE 2015) . Perth, Australia 29 Nov - 02 Dec 2015 Perth, Australia.Study to investigate factors influencing adoption of mobile devices in the healthcare environment
Rani, Vasundhara. 2018. Study to investigate factors influencing adoption of mobile devices in the healthcare environment. PhD Thesis Doctor of Philosophy. University of Southern Queensland. https://doi.org/10.26192/5c0da36df69cdAn investigation into the relationship between talent management processes and knowledge management processes: a case of the higher education sector in Queensland, Australia
Mohammed, Atheer Abdullah. 2018. An investigation into the relationship between talent management processes and knowledge management processes: a case of the higher education sector in Queensland, Australia. PhD Thesis Doctor of Philosophy. University of Southern Queensland. https://doi.org/10.26192/5f64349dce229Data quality in community health: an Indonesian study
Aisyah, Maulidyati and Cockcroft, Sophie. 2013. "Data quality in community health: an Indonesian study." Deng, Hepu and Standing, Craig (ed.) 24th Australasian Conference on Information Systems (ACIS 2013). Melbourne, Australia 04 - 06 Dec 2013 Melbourne, Australia.Determinants of telehealth adoption in the Indian healthcare domain: an exploratory study
Chowdhury, Avijit. 2021. Determinants of telehealth adoption in the Indian healthcare domain: an exploratory study. PhD Thesis Doctor of Philosophy. University of Southern Queensland. https://doi.org/10.26192/q6v72Determination of factors influencing student engagement using a learning management system in a tertiary setting
Barua, Prabal Datta. 2020. Determination of factors influencing student engagement using a learning management system in a tertiary setting. PhD Thesis Doctor of Philosophy. University of Southern Queensland. https://doi.org/10.26192/Z9AS-4P42Consumer engagement with social media marketing: an empirical investigation in Australia
Deo, Kirtika. 2019. Consumer engagement with social media marketing: an empirical investigation in Australia. Doctorate other than PhD Doctor of Business Administation. University of Southern Queensland. https://doi.org/10.26192/fxdj-qk37Internet governance and cyber-security: a systematic literature review
Yusif, Salifu, Hafeez-Baig, Abdul and Anachanser, Charles. 2024. "Internet governance and cyber-security: a systematic literature review." Information Security Journal: a Global Perspective. 33 (2), pp. 158-171. https://doi.org/10.1080/19393555.2023.2268608Wavelet scattering- and object detection-based computer vision for identifying dengue from peripheral blood microscopy
Dsilva, Liora Rosvin, Tantri, Shivani Harish, Sampathila, Niranjana, Mayrose, Hilda, Bairy, G. Muralidhar, Belurkar, Sushma, Saravu, Kavitha, Chadaga, Krishnaraj and Hafeez-Baig, Abdul. 2024. "Wavelet scattering- and object detection-based computer vision for identifying dengue from peripheral blood microscopy." International Journal of Imaging Systems and Technology. 34 (1). https://doi.org/10.1002/ima.23020Novel tiny textural motif pattern-based RNA virus protein sequence classification model
Erten, Mehmet, Aydemir, Emrah, Barua, Prabal Datta, Baygin, Mehmet, Dogan, Sengul, Tuncer, Turker, Tan, Ru-San, Hafeez-Baig, Abdul and Acharya, U. Rajendra. 2024. "Novel tiny textural motif pattern-based RNA virus protein sequence classification model." Expert Systems with Applications. 242. https://doi.org/10.1016/j.eswa.2023.122781267
total views24
total downloads10
views this month2
downloads this month
Export as
Related outputs
Dr Abdul Hafeez-Baig
Hafeez-Baig, A.. Dr Abdul Hafeez-Baig.Fibromyalgia Detection and Diagnosis: A Systematic Review of Data-Driven Approaches and Clinical Implications
Atmakuru, Anirudh, Chakraborty, Subrata, Salvi, Massimo, Faust, Oliver, Barua, Prabal Datta, Kobayashi, Makiko, Tan, Ru San, Molinari, Filippo, Hafeez-Baig, Abdul and Acharya, U. Rajendra. 2025. "Fibromyalgia Detection and Diagnosis: A Systematic Review of Data-Driven Approaches and Clinical Implications." IEEE Access. 13, pp. 25026-25044. https://doi.org/10.1109/ACCESS.2025.3539196Automated hip dysplasia detection using novel FlexiLBPHOG model with ultrasound images
Key, Sefa, Kurum, Huseyin, Esmez, Omer, Hafeez-Baig, Abdul, Hajiyeva, Rena, Dogan, Sengul and Tuncer, Turker. 2025. "Automated hip dysplasia detection using novel FlexiLBPHOG model with ultrasound images." Ain Shams Engineering Journal. 16 (1). https://doi.org/10.1016/j.asej.2024.103235Artificial Intelligence-Based Suicide Prevention and Prediction: A Systematic Review (2019-2023)
Atmakuru, Anirudh, Shahini, Alen, Chakraborty, Subrata, Seoni, Silvia, Salvi, Massimo, Hafeez-Baig, Abdul, Rashid, Sadaf, Tan, Ru San, Barua, Prabal Datta, Molinari, Filippo and Acharya, U Rajendra. 2025. "Artificial Intelligence-Based Suicide Prevention and Prediction: A Systematic Review (2019-2023)." Information Fusion. 114. https://doi.org/10.1016/j.inffus.2024.102673Explainable deeply-fused nets electricity demand prediction model: Factoring climate predictors for accuracy and deeper insights with probabilistic confidence interval and point-based forecasts
Ghimire, Sujan, AL-Musaylh, Mohanad S., Nguyen-Huy, Thong, Deo, Ravinesh C., Acharya, Rajendra, Casillas-Perez, David, Yaseen, Zaher Mundher and Salcedo-sanz, Sancho. 2025. "Explainable deeply-fused nets electricity demand prediction model: Factoring climate predictors for accuracy and deeper insights with probabilistic confidence interval and point-based forecasts." Applied Energy. 378 (Part A). https://doi.org/10.1016/j.apenergy.2024.124763AttentionPoolMobileNeXt: An automated construction damage detection model based on a new convolutional neural network and deep feature engineering models
Aydin, Mehmet, Barua, Prabal Datta, Chadalavada, Sreenivasulu, Dogan, Sengul, Tuncer, Turker, Chakraborty, Subrata and Acharya, Rajendra U.. 2025. "AttentionPoolMobileNeXt: An automated construction damage detection model based on a new convolutional neural network and deep feature engineering models." Multimedia Tools and Applications. 84 (4), pp. 1821-1843. https://doi.org/10.1007/s11042-024-19163-2Directed Lobish-based explainable feature engineering model with TTPat and CWINCA for EEG artifact classification
Tuncer, Turker, Dogan, Sengul, Baygin, Mehmet, Tasci, Irem, Mungen, Bulent, Tasci, Burak, Barua, Prabal Datta and Acharya, U.R.. 2024. "Directed Lobish-based explainable feature engineering model with TTPat and CWINCA for EEG artifact classification." Knowledge-Based Systems. 305. https://doi.org/10.1016/j.knosys.2024.112555Retinal Health Screening Using Artificial Intelligence with Digital Fundus Images: A Review of the Last Decade (2012-2023)
Islam, Saad, Deo, Ravinesh C., Barua, Prabal Datta, Soar, Jeffrey, Yu, Ping and Acharya, U. Rajendra. 2024. "Retinal Health Screening Using Artificial Intelligence with Digital Fundus Images: A Review of the Last Decade (2012-2023)." IEEE Access. 12, pp. 176630-176685. https://doi.org/10.1109/ACCESS.2024.3477420Automated EEG-based language detection using directed quantum pattern technique
Dogan, Sengul, Tuncer, Turker, Barua, Prabal Datta and Acharya, U.R.. 2024. "Automated EEG-based language detection using directed quantum pattern technique." Applied Soft Computing. 167 (Part A). https://doi.org/10.1016/j.asoc.2024.112301A Novel Hybrid Model for Automatic Non-Small Cell Lung Cancer Classification Using Histopathological Images
Katar, Oguzhan, Yildirim, Ozal, Tan, Ru-San and Acharya, U Rajendra. 2024. "A Novel Hybrid Model for Automatic Non-Small Cell Lung Cancer Classification Using Histopathological Images." Diagnostics. 14 (22). https://doi.org/10.3390/diagnostics14222497Synthetic Data Generation via Generative Adversarial Networks in Healthcare: A Systematic Review of Image- and Signal-Based Studies
Akpinar, Muhammed Halil, Sengur, Abdulkadir, Salvi, Massimo, Seoni, Silvia, Faust, Oliver, Mir, Hasan, Molinari,Filippo and Acharya, U. Rajendra. 2024. "Synthetic Data Generation via Generative Adversarial Networks in Healthcare: A Systematic Review of Image- and Signal-Based Studies." IEEE Open Journal of Engineering in Medicine and Biology. 6, pp. 183-192. https://doi.org/10.1109/OJEMB.2024.3508472RECOMED: A comprehensive pharmaceutical recommendation system
Zomorodi, Mariam, Ghodsollahee, Ismail, Martin, Jennifer H, Talley, Nicholas J, Salari, Vahid, Pławiak, Paweł, Rahimi, Kazem and Acharya, U.R.. 2024. "RECOMED: A comprehensive pharmaceutical recommendation system." Artificial Intelligence in Medicine. 157. https://doi.org/10.1016/j.artmed.2024.102981Artificial intelligence in assessing cardiovascular diseases and risk factors via retinal fundus images: A review of the last decade
Abdollahi, Mirsaeed, Jafarizadeh, Ali, Ghafouri-Asbagh, Amirhosein, Sobhi, Navid, Pourmoghtader, Keysan, Pedrammehr, Siamak, Asadi, Houshyar, Tan, Ru-San, Alizadehsani, Roohallah and Acharya, U. Rajendra. 2024. "Artificial intelligence in assessing cardiovascular diseases and risk factors via retinal fundus images: A review of the last decade." WIREs Data Mining and Knowledge Discovery. 14 (6). https://doi.org/10.1002/widm.1560Early prediction of sudden cardiac death using multimodal fusion of ECG Features extracted from Hilbert–Huang and wavelet transforms with explainable vision transformer and CNN models
Telangore, Hardik, Azad, Victor, Sharma, Manish, Bhurane, Ankit, Tan, Ru San and Acharya, U. Rajendra. 2024. "Early prediction of sudden cardiac death using multimodal fusion of ECG Features extracted from Hilbert–Huang and wavelet transforms with explainable vision transformer and CNN models." Computer Methods and Programs in Biomedicine. 257. https://doi.org/10.1016/j.cmpb.2024.108455A Pragmatic Approach to Fetal Monitoring via Cardiotocography Using Feature Elimination and Hyperparameter Optimization
Hardalac, Firat, Akmal, Haad, Ayturan, Kubilay, Acharya, U. Rajendra and Tan, Ru-San. 2024. "A Pragmatic Approach to Fetal Monitoring via Cardiotocography Using Feature Elimination and Hyperparameter Optimization." Interdisciplinary Sciences: Computational Life Sciences. 16 (4), pp. 882-906. https://doi.org/10.1007/s12539-024-00647-6Automated System for the Detection of Heart Anomalies Using Phonocardiograms: A Systematic Review
Gudigar, Anjan, Raghavendra, U., Maithri, M., Samanth, Jyothi, Inamdar, Mahesh Anil, Vidhya, V., Vicnesh, Jahmunah, Prabhu, Mukund A., Tan, Ru-San, Yeong, Chai Hong, Molinari, Filippo and Acharya, U. R.. 2024. "Automated System for the Detection of Heart Anomalies Using Phonocardiograms: A Systematic Review." IEEE Access. 12, pp. 138399-138428. https://doi.org/10.1109/ACCESS.2024.3465511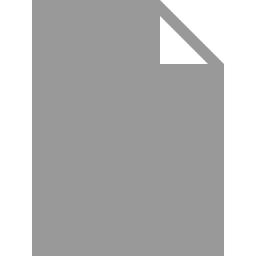