Automated characterization and detection of fibromyalgia using slow wave sleep EEG signals with glucose pattern and D’hondt pooling technique
Article
Aksalli, Isil Karabey, Baygin, Nursena, Hagiwara, Yuki, Paul, Jose Kunnel, Iype, Thomas, Barua, Prabal Datta, Koh, Joel E. W., Baygin, Mehmet, Dogan, Sengul, Tuncer, Turker and Acharya, U. Rajendra. 2024. "Automated characterization and detection of fibromyalgia using slow wave sleep EEG signals with glucose pattern and D’hondt pooling technique." Cognitive Neurodynamics. 18 (1), pp. 283-404. https://doi.org/10.1007/s11571-023-10005-9
Article Title | Automated characterization and detection of fibromyalgia using slow wave sleep EEG signals with glucose pattern and D’hondt pooling technique |
---|---|
ERA Journal ID | 3179 |
Article Category | Article |
Authors | Aksalli, Isil Karabey, Baygin, Nursena, Hagiwara, Yuki, Paul, Jose Kunnel, Iype, Thomas, Barua, Prabal Datta, Koh, Joel E. W., Baygin, Mehmet, Dogan, Sengul, Tuncer, Turker and Acharya, U. Rajendra |
Journal Title | Cognitive Neurodynamics |
Journal Citation | 18 (1), pp. 283-404 |
Number of Pages | 22 |
Year | 2024 |
Publisher | Springer |
Place of Publication | Netherlands |
ISSN | 1871-4080 |
1871-4099 | |
Digital Object Identifier (DOI) | https://doi.org/10.1007/s11571-023-10005-9 |
Web Address (URL) | https://link.springer.com/article/10.1007/s11571-023-10005-9 |
Abstract | Fibromyalgia is a soft tissue rheumatism with significant qualitative and quantitative impact on sleep macro and micro architecture. The primary objective of this study is to analyze and identify automatically healthy individuals and those with fibromyalgia using sleep electroencephalography (EEG) signals. The study focused on the automatic detection and interpretation of EEG signals obtained from fibromyalgia patients. In this work, the sleep EEG signals are divided into 15-s and a total of 5358 (3411 healthy control and 1947 fibromyalgia) EEG segments are obtained from 16 fibromyalgia and 16 normal subjects. Our developed model has advanced multilevel feature extraction architecture and hence, we used a new feature extractor called GluPat, inspired by the glucose chemical, with a new pooling approach inspired by the D’hondt selection system. Furthermore, our proposed method incorporated feature selection techniques using iterative neighborhood component analysis and iterative Chi2 methods. These selection mechanisms enabled the identification of discriminative features for accurate classification. In the classification phase, we employed a support vector machine and k-nearest neighbor algorithms to classify the EEG signals with leave-one-record-out (LORO) and tenfold cross-validation (CV) techniques. All results are calculated channel-wise and iterative majority voting is used to obtain generalized results. The best results were determined using the greedy algorithm. The developed model achieved a detection accuracy of 100% and 91.83% with a tenfold and LORO CV strategies, respectively using sleep stage (2 + 3) EEG signals. Our generated model is simple and has linear time complexity. |
Keywords | D′hondt pooling; Glucose pattern; Fibromyalgia; LORO |
Contains Sensitive Content | Does not contain sensitive content |
ANZSRC Field of Research 2020 | 400306. Computational physiology |
Public Notes | Files associated with this item cannot be displayed due to copyright restrictions. |
Byline Affiliations | Erzurum Technical University, Turkey |
Fraunhofer Institute for Cognitive Systems IKS, Germany | |
Government Medical College, India | |
School of Business | |
Ngee Ann Polytechnic, Singapore | |
Firat University, Turkey | |
School of Mathematics, Physics and Computing |
Permalink -
https://research.usq.edu.au/item/z1vqz/automated-characterization-and-detection-of-fibromyalgia-using-slow-wave-sleep-eeg-signals-with-glucose-pattern-and-d-hondt-pooling-technique
81
total views0
total downloads0
views this month0
downloads this month
Export as
Related outputs
Automated hip dysplasia detection using novel FlexiLBPHOG model with ultrasound images
Key, Sefa, Kurum, Huseyin, Esmez, Omer, Hafeez-Baig, Abdul, Hajiyeva, Rena, Dogan, Sengul and Tuncer, Turker. 2025. "Automated hip dysplasia detection using novel FlexiLBPHOG model with ultrasound images." Ain Shams Engineering Journal. 16 (1). https://doi.org/10.1016/j.asej.2024.103235Artificial Intelligence-Based Suicide Prevention and Prediction: A Systematic Review (2019-2023)
Atmakuru, Anirudh, Shahini, Alen, Chakraborty, Subrata, Seoni, Silvia, Salvi, Massimo, Hafeez-Baig, Abdul, Rashid, Sadaf, Tan, Ru San, Barua, Prabal Datta, Molinari, Filippo and Acharya, U Rajendra. 2025. "Artificial Intelligence-Based Suicide Prevention and Prediction: A Systematic Review (2019-2023)." Information Fusion. 114. https://doi.org/10.1016/j.inffus.2024.102673Explainable deeply-fused nets electricity demand prediction model: Factoring climate predictors for accuracy and deeper insights with probabilistic confidence interval and point-based forecasts
Ghimire, Sujan, AL-Musaylh, Mohanad S., Nguyen-Huy, Thong, Deo, Ravinesh C., Acharya, Rajendra, Casillas-Perez, David, Yaseen, Zaher Mundher and Salcedo-sanz, Sancho. 2025. "Explainable deeply-fused nets electricity demand prediction model: Factoring climate predictors for accuracy and deeper insights with probabilistic confidence interval and point-based forecasts." Applied Energy. 378 (Part A). https://doi.org/10.1016/j.apenergy.2024.124763AttentionPoolMobileNeXt: An automated construction damage detection model based on a new convolutional neural network and deep feature engineering models
Aydin, Mehmet, Barua, Prabal Datta, Chadalavada, Sreenivasulu, Dogan, Sengul, Tuncer, Turker, Chakraborty, Subrata and Acharya, Rajendra U.. 2025. "AttentionPoolMobileNeXt: An automated construction damage detection model based on a new convolutional neural network and deep feature engineering models." Multimedia Tools and Applications. 84 (4), pp. 1821-1843. https://doi.org/10.1007/s11042-024-19163-2Directed Lobish-based explainable feature engineering model with TTPat and CWINCA for EEG artifact classification
Tuncer, Turker, Dogan, Sengul, Baygin, Mehmet, Tasci, Irem, Mungen, Bulent, Tasci, Burak, Barua, Prabal Datta and Acharya, U.R.. 2024. "Directed Lobish-based explainable feature engineering model with TTPat and CWINCA for EEG artifact classification." Knowledge-Based Systems. 305. https://doi.org/10.1016/j.knosys.2024.112555Retinal Health Screening Using Artificial Intelligence with Digital Fundus Images: A Review of the Last Decade (2012-2023)
Islam, Saad, Deo, Ravinesh C., Barua, Prabal Datta, Soar, Jeffrey, Yu, Ping and Acharya, U. Rajendra. 2024. "Retinal Health Screening Using Artificial Intelligence with Digital Fundus Images: A Review of the Last Decade (2012-2023)." IEEE Access. 12, pp. 176630-176685. https://doi.org/10.1109/ACCESS.2024.3477420Automated EEG-based language detection using directed quantum pattern technique
Dogan, Sengul, Tuncer, Turker, Barua, Prabal Datta and Acharya, U.R.. 2024. "Automated EEG-based language detection using directed quantum pattern technique." Applied Soft Computing. 167 (Part A). https://doi.org/10.1016/j.asoc.2024.112301A Novel Hybrid Model for Automatic Non-Small Cell Lung Cancer Classification Using Histopathological Images
Katar, Oguzhan, Yildirim, Ozal, Tan, Ru-San and Acharya, U Rajendra. 2024. "A Novel Hybrid Model for Automatic Non-Small Cell Lung Cancer Classification Using Histopathological Images." Diagnostics. 14 (22). https://doi.org/10.3390/diagnostics14222497Synthetic Data Generation via Generative Adversarial Networks in Healthcare: A Systematic Review of Image- and Signal-Based Studies
Akpinar, Muhammed Halil, Sengur, Abdulkadir, Salvi, Massimo, Seoni, Silvia, Faust, Oliver, Mir, Hasan, Molinari,Filippo and Acharya, U. Rajendra. 2024. "Synthetic Data Generation via Generative Adversarial Networks in Healthcare: A Systematic Review of Image- and Signal-Based Studies." IEEE Open Journal of Engineering in Medicine and Biology. 6, pp. 183-192. https://doi.org/10.1109/OJEMB.2024.3508472RECOMED: A comprehensive pharmaceutical recommendation system
Zomorodi, Mariam, Ghodsollahee, Ismail, Martin, Jennifer H, Talley, Nicholas J, Salari, Vahid, Pławiak, Paweł, Rahimi, Kazem and Acharya, U.R.. 2024. "RECOMED: A comprehensive pharmaceutical recommendation system." Artificial Intelligence in Medicine. 157. https://doi.org/10.1016/j.artmed.2024.102981Artificial intelligence in assessing cardiovascular diseases and risk factors via retinal fundus images: A review of the last decade
Abdollahi, Mirsaeed, Jafarizadeh, Ali, Ghafouri-Asbagh, Amirhosein, Sobhi, Navid, Pourmoghtader, Keysan, Pedrammehr, Siamak, Asadi, Houshyar, Tan, Ru-San, Alizadehsani, Roohallah and Acharya, U. Rajendra. 2024. "Artificial intelligence in assessing cardiovascular diseases and risk factors via retinal fundus images: A review of the last decade." WIREs Data Mining and Knowledge Discovery. 14 (6). https://doi.org/10.1002/widm.1560Early prediction of sudden cardiac death using multimodal fusion of ECG Features extracted from Hilbert–Huang and wavelet transforms with explainable vision transformer and CNN models
Telangore, Hardik, Azad, Victor, Sharma, Manish, Bhurane, Ankit, Tan, Ru San and Acharya, U. Rajendra. 2024. "Early prediction of sudden cardiac death using multimodal fusion of ECG Features extracted from Hilbert–Huang and wavelet transforms with explainable vision transformer and CNN models." Computer Methods and Programs in Biomedicine. 257. https://doi.org/10.1016/j.cmpb.2024.108455A Pragmatic Approach to Fetal Monitoring via Cardiotocography Using Feature Elimination and Hyperparameter Optimization
Hardalac, Firat, Akmal, Haad, Ayturan, Kubilay, Acharya, U. Rajendra and Tan, Ru-San. 2024. "A Pragmatic Approach to Fetal Monitoring via Cardiotocography Using Feature Elimination and Hyperparameter Optimization." Interdisciplinary Sciences: Computational Life Sciences. 16 (4), pp. 882-906. https://doi.org/10.1007/s12539-024-00647-6Automated System for the Detection of Heart Anomalies Using Phonocardiograms: A Systematic Review
Gudigar, Anjan, Raghavendra, U., Maithri, M., Samanth, Jyothi, Inamdar, Mahesh Anil, Vidhya, V., Vicnesh, Jahmunah, Prabhu, Mukund A., Tan, Ru-San, Yeong, Chai Hong, Molinari, Filippo and Acharya, U. R.. 2024. "Automated System for the Detection of Heart Anomalies Using Phonocardiograms: A Systematic Review." IEEE Access. 12, pp. 138399-138428. https://doi.org/10.1109/ACCESS.2024.3465511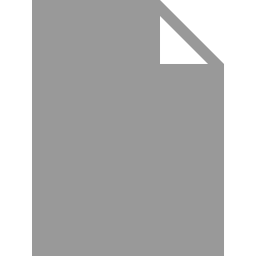