NFSDense201: microstructure image classification based on non-fixed size patch division with pre-trained DenseNet201 layers
Article
Barua, Prabal Datta, Dogan, Sengul, Kavuran, Gurkan, Tuncer, Turker, Tan, Ru-San and Acharya, U. Rajendra. 2023. "NFSDense201: microstructure image classification based on non-fixed size patch division with pre-trained DenseNet201 layers." Neural Computing and Applications. 35 (30), pp. 22253-22263. https://doi.org/10.1007/s00521-023-08825-1
Article Title | NFSDense201: microstructure image classification based on non-fixed size patch division with pre-trained DenseNet201 layers |
---|---|
Article Category | Article |
Authors | Barua, Prabal Datta, Dogan, Sengul, Kavuran, Gurkan, Tuncer, Turker, Tan, Ru-San and Acharya, U. Rajendra |
Journal Title | Neural Computing and Applications |
Journal Citation | 35 (30), pp. 22253-22263 |
Number of Pages | 11 |
Year | 2023 |
Place of Publication | United Kingdom |
Digital Object Identifier (DOI) | https://doi.org/10.1007/s00521-023-08825-1 |
Web Address (URL) | https://link.springer.com/article/10.1007/s00521-023-08825-1 |
Abstract | In the field of nanoscience, the scanning electron microscope (SEM) is widely employed to visualize the surface topography and composition of materials. In this study, we present a novel SEM image classification model called NFSDense201, which incorporates several key components. Firstly, we propose a unique nested patch division approach that divides each input image into four patches of varying dimensions. Secondly, we utilize DenseNet201, a deep neural network pretrained on ImageNet1k, to extract 2920 deep features from the last fully connected and global average pooling layers. Thirdly, we introduce an iterative neighborhood component analysis function to select the most discriminative features from the merged feature vector, which is formed by concatenating the four feature vectors extracted per input image. This process results in a final feature vector of optimal length 698. Lastly, we employ a standard shallow support vector machine classifier to perform the actual classification. To evaluate the performance of NFSDense201, we conducted experiments using a large public SEM image dataset. The dataset consists of 972, 162, 326, 4590, 3820, 3925, 4755, 181, 917, and 1624.jpeg images belonging to the following microstructural categories: “biological,” “fibers,” “film-coated surfaces,” “MEMS devices and electrodes,” “nanowires,” “particles,” “pattern surfaces,” “porous sponge,” “powder,” and “tips,” respectively. For both four-class and ten-class classification tasks, we evaluated NFSDense201 using subsets of the dataset containing 5080 and 21,272 images, respectively. The results demonstrate the superior performance of NFSDense201, achieving a four-class classification accuracy rate of 99.53% and a ten-class classification accuracy rate of 97.09%. These accuracy rates compare favorably against previously published SEM image classification models. Additionally, we report the performance of NFSDense201 for each class in the dataset. |
Keywords | Deep feature engineering; Non-fixed size patch division; SEM image classification ; DenseNet201 |
ANZSRC Field of Research 2020 | 400306. Computational physiology |
Public Notes | Files associated with this item cannot be displayed due to copyright restrictions. |
Byline Affiliations | School of Business |
University of Technology Sydney | |
Australian International Institute of Higher Education, Australia | |
University of New England | |
Taylor’s University, Malaysia | |
SRM Institute of Science and Technology, India | |
Kumamoto University, Japan | |
University of Sydney | |
Firat University, Turkey | |
Malatya Turgut Ozal University, Turkiye | |
National Heart Centre, Singapore | |
Duke-NUS Medical School, Singapore | |
School of Mathematics, Physics and Computing |
Permalink -
https://research.usq.edu.au/item/z1v63/nfsdense201-microstructure-image-classification-based-on-non-fixed-size-patch-division-with-pre-trained-densenet201-layers
70
total views0
total downloads2
views this month0
downloads this month
Export as
Related outputs
Fibromyalgia Detection and Diagnosis: A Systematic Review of Data-Driven Approaches and Clinical Implications
Atmakuru, Anirudh, Chakraborty, Subrata, Salvi, Massimo, Faust, Oliver, Barua, Prabal Datta, Kobayashi, Makiko, Tan, Ru San, Molinari, Filippo, Hafeez-Baig, Abdul and Acharya, U. Rajendra. 2025. "Fibromyalgia Detection and Diagnosis: A Systematic Review of Data-Driven Approaches and Clinical Implications." IEEE Access. 13, pp. 25026-25044. https://doi.org/10.1109/ACCESS.2025.3539196Current and future roles of artificial intelligence in retinopathy of prematurity
Jafarizadeh, Ali, Maleki, Shadi Farabi, Pouya, Parnia, Sobhi, Navid, Abdollahi, Mirsaeed, Pedrammehr, Siamak, Lim, Chee Peng, Asadi, Houshyar, Alizadehsani, Roohallah, Tan, Ru-San, Islam, Sheikh Mohammed Shariful and Acharya, U. Rajendra. 2025. "Current and future roles of artificial intelligence in retinopathy of prematurity." Artificial Intelligence Review. 58 (6). https://doi.org/10.1007/s10462-025-11153-6Artificial Intelligence for Ovarian Cancer Detection with Medical Images: A Review of the Last Decade (2013–2023)
Naderi Yaghouti, Amir Reza, Shalbaf, Ahmad, Alizadehsani, Roohallah, Tan, Ru-San, Vijayananthan, Anushya, Yeong, Chai Hong and Acharya, U. Rajendra. 2025. "Artificial Intelligence for Ovarian Cancer Detection with Medical Images: A Review of the Last Decade (2013–2023)." Archives of Computational Methods in Engineering. https://doi.org/10.1007/s11831-025-10268-xFlexiCombFE: A flexible, combination-based feature engineering framework for brain tumor detection
Tuncer, Ilknur, Hafeez-Baig, Abdul, Barua, Prabal Datta, Hajiyeva, Rena, Massimo, Salvi, Dogan, Sengul, Tuncer, Turker and Acharya, U.R.. 2025. "FlexiCombFE: A flexible, combination-based feature engineering framework for brain tumor detection." Biomedical Signal Processing and Control. 104. https://doi.org/10.1016/j.bspc.2025.107538EEGConvNeXt: A novel convolutional neural network model for automated detection of Alzheimer's Disease and Frontotemporal Dementia using EEG signals
Tao, Xiaohui, Acharya, Madhav, Deo, Ravinesh C, Barua, Prabal Datta and Devi, Aruna. 2025. "EEGConvNeXt: A novel convolutional neural network model for automated detection of Alzheimer's Disease and Frontotemporal Dementia using EEG signals." Computer Methods and Programs in Biomedicine. 262. https://doi.org/10.1016/j.cmpb.2025.108652BrainNeXt: novel lightweight CNN model for the automated detection of brain disorders using MRI images
Poyraz, Melahat, Poyraz, Ahmet Kursad, Dogan, Yusuf, Gunes, Selva, Mir, Hasan S., Paul, Jose Kunnel, Barua, Prabal Datta, Baygin, Mehmet, Dogan, Sengul, Tuncer, Turker, Molinari, Filippo and Acharya, Rajendra. 2025. "BrainNeXt: novel lightweight CNN model for the automated detection of brain disorders using MRI images." Cognitive Neurodynamics. 19 (1). https://doi.org/10.1007/s11571-025-10235-zA novel uncertainty-aware liquid neural network for noise-resilient time series forecasting and classification
Akpinar, Muhammed Halil, Atila, Orhan, Sengur, Abdulkadir, Salvi, Massimo and Acharya, U.R.. 2025. "A novel uncertainty-aware liquid neural network for noise-resilient time series forecasting and classification." Chaos, Solitons and Fractals. 193. https://doi.org/10.1016/j.chaos.2025.116130MobileTransNeXt: Integrating CNN, transformer, and BiLSTM for image classification
Ye, Peishun, Lin, Jiyan, Kang, Yaming, Kaya, Tolga, Yildirim, Kubra, Hafeez Baig, Abdul, Aydemir, Emrah, Dogan, Sengul and Tuncer, Turker. 2025. "MobileTransNeXt: Integrating CNN, transformer, and BiLSTM for image classification." Alexandria Engineering Journal. 123, pp. 460-470. https://doi.org/10.1016/j.aej.2025.03.048Electricity demand uncertainty modeling with Temporal Convolution Neural Network models
Ghimire, Sujan, Deo, Ravinesh C., Casillas-Perez, David, Salcedo-Sanz, Sancho, Acharya, Rajendra and Dinh, Toan. 2025. "Electricity demand uncertainty modeling with Temporal Convolution Neural Network models." Renewable and Sustainable Energy Reviews. 209. https://doi.org/10.1016/j.rser.2024.115097Half-hourly electricity price prediction model with explainable-decomposition hybrid deep learning approach
Ghimire, Sujan, Deo, Ravinesh C., Hopf, Konstantin, Liu, Hangyue, Casillas-Perez, David, Helwig, Andreas, Prasad, Salvin S., Perez-Aracil, Jorge, Barua, Prabal Datta and Salcedo-Sanz, Sancho. 2025. "Half-hourly electricity price prediction model with explainable-decomposition hybrid deep learning approach." Energy and AI. 20. https://doi.org/10.1016/j.egyai.2025.100492Investigating Brain Lobe Biomarkers to Enhance Dementia Detection Using EEG Data
Siuly, Siuly, Tawhid, Md.Nurul Ahad, Li, Yan, Acharya, Rajendra, Sadiq, Muhammad Tariq and Wang, Hua. 2025. "Investigating Brain Lobe Biomarkers to Enhance Dementia Detection Using EEG Data." Cognitive Computation. 17 (2). https://doi.org/10.1007/s12559-025-10447-9Explainable hybrid deep learning framework for enhancing multi-step solar ultraviolet-B radiation predictions
Prasad, Salvin S., Joseph, Lionel P., Ghimire, Sujan, Deo, Ravinesh C., Downs, Nathan J., Acharya, Rajendra and Yaseen, Zaher M.. 2025. "Explainable hybrid deep learning framework for enhancing multi-step solar ultraviolet-B radiation predictions." Atmospheric Environment. 343. https://doi.org/10.1016/j.atmosenv.2024.120951Automated hip dysplasia detection using novel FlexiLBPHOG model with ultrasound images
Key, Sefa, Kurum, Huseyin, Esmez, Omer, Hafeez-Baig, Abdul, Hajiyeva, Rena, Dogan, Sengul and Tuncer, Turker. 2025. "Automated hip dysplasia detection using novel FlexiLBPHOG model with ultrasound images." Ain Shams Engineering Journal. 16 (1). https://doi.org/10.1016/j.asej.2024.103235Artificial Intelligence-Based Suicide Prevention and Prediction: A Systematic Review (2019-2023)
Atmakuru, Anirudh, Shahini, Alen, Chakraborty, Subrata, Seoni, Silvia, Salvi, Massimo, Hafeez-Baig, Abdul, Rashid, Sadaf, Tan, Ru San, Barua, Prabal Datta, Molinari, Filippo and Acharya, U Rajendra. 2025. "Artificial Intelligence-Based Suicide Prevention and Prediction: A Systematic Review (2019-2023)." Information Fusion. 114. https://doi.org/10.1016/j.inffus.2024.102673Explainable deeply-fused nets electricity demand prediction model: Factoring climate predictors for accuracy and deeper insights with probabilistic confidence interval and point-based forecasts
Ghimire, Sujan, AL-Musaylh, Mohanad S., Nguyen-Huy, Thong, Deo, Ravinesh C., Acharya, Rajendra, Casillas-Perez, David, Yaseen, Zaher Mundher and Salcedo-sanz, Sancho. 2025. "Explainable deeply-fused nets electricity demand prediction model: Factoring climate predictors for accuracy and deeper insights with probabilistic confidence interval and point-based forecasts." Applied Energy. 378 (Part A). https://doi.org/10.1016/j.apenergy.2024.124763AttentionPoolMobileNeXt: An automated construction damage detection model based on a new convolutional neural network and deep feature engineering models
Aydin, Mehmet, Barua, Prabal Datta, Chadalavada, Sreenivasulu, Dogan, Sengul, Tuncer, Turker, Chakraborty, Subrata and Acharya, Rajendra U.. 2025. "AttentionPoolMobileNeXt: An automated construction damage detection model based on a new convolutional neural network and deep feature engineering models." Multimedia Tools and Applications. 84 (4), pp. 1821-1843. https://doi.org/10.1007/s11042-024-19163-2Directed Lobish-based explainable feature engineering model with TTPat and CWINCA for EEG artifact classification
Tuncer, Turker, Dogan, Sengul, Baygin, Mehmet, Tasci, Irem, Mungen, Bulent, Tasci, Burak, Barua, Prabal Datta and Acharya, U.R.. 2024. "Directed Lobish-based explainable feature engineering model with TTPat and CWINCA for EEG artifact classification." Knowledge-Based Systems. 305. https://doi.org/10.1016/j.knosys.2024.112555Retinal Health Screening Using Artificial Intelligence with Digital Fundus Images: A Review of the Last Decade (2012-2023)
Islam, Saad, Deo, Ravinesh C., Barua, Prabal Datta, Soar, Jeffrey, Yu, Ping and Acharya, U. Rajendra. 2024. "Retinal Health Screening Using Artificial Intelligence with Digital Fundus Images: A Review of the Last Decade (2012-2023)." IEEE Access. 12, pp. 176630-176685. https://doi.org/10.1109/ACCESS.2024.3477420Automated EEG-based language detection using directed quantum pattern technique
Dogan, Sengul, Tuncer, Turker, Barua, Prabal Datta and Acharya, U.R.. 2024. "Automated EEG-based language detection using directed quantum pattern technique." Applied Soft Computing. 167 (Part A). https://doi.org/10.1016/j.asoc.2024.112301A Novel Hybrid Model for Automatic Non-Small Cell Lung Cancer Classification Using Histopathological Images
Katar, Oguzhan, Yildirim, Ozal, Tan, Ru-San and Acharya, U Rajendra. 2024. "A Novel Hybrid Model for Automatic Non-Small Cell Lung Cancer Classification Using Histopathological Images." Diagnostics. 14 (22). https://doi.org/10.3390/diagnostics14222497Synthetic Data Generation via Generative Adversarial Networks in Healthcare: A Systematic Review of Image- and Signal-Based Studies
Akpinar, Muhammed Halil, Sengur, Abdulkadir, Salvi, Massimo, Seoni, Silvia, Faust, Oliver, Mir, Hasan, Molinari,Filippo and Acharya, U. Rajendra. 2024. "Synthetic Data Generation via Generative Adversarial Networks in Healthcare: A Systematic Review of Image- and Signal-Based Studies." IEEE Open Journal of Engineering in Medicine and Biology. 6, pp. 183-192. https://doi.org/10.1109/OJEMB.2024.3508472RECOMED: A comprehensive pharmaceutical recommendation system
Zomorodi, Mariam, Ghodsollahee, Ismail, Martin, Jennifer H, Talley, Nicholas J, Salari, Vahid, Pławiak, Paweł, Rahimi, Kazem and Acharya, U.R.. 2024. "RECOMED: A comprehensive pharmaceutical recommendation system." Artificial Intelligence in Medicine. 157. https://doi.org/10.1016/j.artmed.2024.102981Artificial intelligence in assessing cardiovascular diseases and risk factors via retinal fundus images: A review of the last decade
Abdollahi, Mirsaeed, Jafarizadeh, Ali, Ghafouri-Asbagh, Amirhosein, Sobhi, Navid, Pourmoghtader, Keysan, Pedrammehr, Siamak, Asadi, Houshyar, Tan, Ru-San, Alizadehsani, Roohallah and Acharya, U. Rajendra. 2024. "Artificial intelligence in assessing cardiovascular diseases and risk factors via retinal fundus images: A review of the last decade." WIREs Data Mining and Knowledge Discovery. 14 (6). https://doi.org/10.1002/widm.1560Early prediction of sudden cardiac death using multimodal fusion of ECG Features extracted from Hilbert–Huang and wavelet transforms with explainable vision transformer and CNN models
Telangore, Hardik, Azad, Victor, Sharma, Manish, Bhurane, Ankit, Tan, Ru San and Acharya, U. Rajendra. 2024. "Early prediction of sudden cardiac death using multimodal fusion of ECG Features extracted from Hilbert–Huang and wavelet transforms with explainable vision transformer and CNN models." Computer Methods and Programs in Biomedicine. 257. https://doi.org/10.1016/j.cmpb.2024.108455A Pragmatic Approach to Fetal Monitoring via Cardiotocography Using Feature Elimination and Hyperparameter Optimization
Hardalac, Firat, Akmal, Haad, Ayturan, Kubilay, Acharya, U. Rajendra and Tan, Ru-San. 2024. "A Pragmatic Approach to Fetal Monitoring via Cardiotocography Using Feature Elimination and Hyperparameter Optimization." Interdisciplinary Sciences: Computational Life Sciences. 16 (4), pp. 882-906. https://doi.org/10.1007/s12539-024-00647-6Automated System for the Detection of Heart Anomalies Using Phonocardiograms: A Systematic Review
Gudigar, Anjan, Raghavendra, U., Maithri, M., Samanth, Jyothi, Inamdar, Mahesh Anil, Vidhya, V., Vicnesh, Jahmunah, Prabhu, Mukund A., Tan, Ru-San, Yeong, Chai Hong, Molinari, Filippo and Acharya, U. R.. 2024. "Automated System for the Detection of Heart Anomalies Using Phonocardiograms: A Systematic Review." IEEE Access. 12, pp. 138399-138428. https://doi.org/10.1109/ACCESS.2024.3465511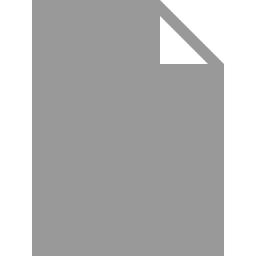