An automated earthquake classification model based on a new butterfly pattern using seismic signals
Article
Ozkaya, Suat Gokhan, Baygin, Mehmet, Barua, Prabal Datta, Tuncer, Turker, Dogan, Sengul, Chakraborty, Subrata and Acharya, U. Rajendra. 2024. "An automated earthquake classification model based on a new butterfly pattern using seismic signals." Expert Systems with Applications. 238 (Part D). https://doi.org/10.1016/j.eswa.2023.122079
Article Title | An automated earthquake classification model based on a new butterfly pattern using seismic signals |
---|---|
ERA Journal ID | 17852 |
Article Category | Article |
Authors | Ozkaya, Suat Gokhan, Baygin, Mehmet, Barua, Prabal Datta, Tuncer, Turker, Dogan, Sengul, Chakraborty, Subrata and Acharya, U. Rajendra |
Journal Title | Expert Systems with Applications |
Journal Citation | 238 (Part D) |
Article Number | 122079 |
Number of Pages | 13 |
Year | 2024 |
Publisher | Elsevier |
Place of Publication | United Kingdom |
ISSN | 0957-4174 |
1873-6793 | |
Digital Object Identifier (DOI) | https://doi.org/10.1016/j.eswa.2023.122079 |
Web Address (URL) | https://www.sciencedirect.com/science/article/pii/S0957417423025812 |
Abstract | Background: Seismic signals are useful for earthquake detection and classification. Therefore, various artificial intelligence (AI) models have been used with seismic signals to develop automated earthquake detection systems. Our primary goal is to present an accurate feature engineering model for earthquake detection and classification using seismic signals. Material and model: We have used a public dataset in this work containing three categories: (1) noise, (2) P waves, and (3) S waves. P and S waves are used to define earthquakes. We have presented two applied use cases using this dataset: (i) earthquake detection and (ii) wave classification. In this work, a new textural feature extractor has been presented by using a graph pattern similar to a butterfly. Thus, this feature extraction function is named Butterfly pattern (BFPat). We have created a new feature engineering architecture by deploying BFPat, statistics, and wavelet packet decomposition (WPD) functions. The recommended BFPat and statistics have been applied to the wavelet bands created by WPD and the raw seismic signals. Multilevel features have been extracted from both frequency and space domains. The used dataset contains signals with three channels. Using these three channels, seven signals have been created. Seven feature vectors have been created from 7 input signals used in this study. The most meaningful/informative features from the generated feature set are then selected using the iterative neighborhood component analysis feature selector method. Seven chosen feature vectors have been considered as inputs of the two shallow classifiers: k nearest neighbors (kNN) and support vector machine (SVM). A total of 14 (=7 × 2) results have been obtained in the classification phase. A majority voting process was applied in the last phase to choose the best results and improve the classification performance. Results: We have presented two use cases for our new BFPat method in this work to obtain superior results. Our model reached an accuracy of 99.58% in detecting the earthquake detection and 93.13% accuracy in 3-class classifications of waves. Conclusions: Our recommended model has achieved over 90% classification performance for both cases. Also, we have presented the most valuable channel and combinations in our work. Our developed system is ready to be tested with a bigger database. © 2023 Elsevier Ltd |
Keywords | Butterfly pattern; Seismic wave classification ; Earthquake detection ; Information fusion ; Feature engineering |
Contains Sensitive Content | Does not contain sensitive content |
ANZSRC Field of Research 2020 | 400506. Earthquake engineering |
Public Notes | Files associated with this item cannot be displayed due to copyright restrictions. |
Byline Affiliations | Ardahan University, Turkiye |
Erzurum Technical University, Turkey | |
School of Business | |
Firat University, Turkey | |
University of New England | |
University of Technology Sydney | |
School of Mathematics, Physics and Computing |
Permalink -
https://research.usq.edu.au/item/z5v81/an-automated-earthquake-classification-model-based-on-a-new-butterfly-pattern-using-seismic-signals
108
total views0
total downloads4
views this month0
downloads this month
Export as
Related outputs
Fibromyalgia Detection and Diagnosis: A Systematic Review of Data-Driven Approaches and Clinical Implications
Atmakuru, Anirudh, Chakraborty, Subrata, Salvi, Massimo, Faust, Oliver, Barua, Prabal Datta, Kobayashi, Makiko, Tan, Ru San, Molinari, Filippo, Hafeez-Baig, Abdul and Acharya, U. Rajendra. 2025. "Fibromyalgia Detection and Diagnosis: A Systematic Review of Data-Driven Approaches and Clinical Implications." IEEE Access. 13, pp. 25026-25044. https://doi.org/10.1109/ACCESS.2025.3539196Current and future roles of artificial intelligence in retinopathy of prematurity
Jafarizadeh, Ali, Maleki, Shadi Farabi, Pouya, Parnia, Sobhi, Navid, Abdollahi, Mirsaeed, Pedrammehr, Siamak, Lim, Chee Peng, Asadi, Houshyar, Alizadehsani, Roohallah, Tan, Ru-San, Islam, Sheikh Mohammed Shariful and Acharya, U. Rajendra. 2025. "Current and future roles of artificial intelligence in retinopathy of prematurity." Artificial Intelligence Review. 58 (6). https://doi.org/10.1007/s10462-025-11153-6BAIoT-EMS: Consortium network for small-medium enterprises management system with blockchain and augmented intelligence of things
Khan, Abdullah Ayub, Yang, Jing, Laghari, Asif Ali, Baqasah, Abdullah M., Alroobaea, Roobaea, Ku, Chin Soon, Alizadehsani, Roohallah, Acharya, U. Rajendra and Por, Lip Yee. 2025. "BAIoT-EMS: Consortium network for small-medium enterprises management system with blockchain and augmented intelligence of things." Engineering Applications of Artificial Intelligence. 141. https://doi.org/10.1016/j.engappai.2024.109838Artificial Intelligence for Ovarian Cancer Detection with Medical Images: A Review of the Last Decade (2013–2023)
Naderi Yaghouti, Amir Reza, Shalbaf, Ahmad, Alizadehsani, Roohallah, Tan, Ru-San, Vijayananthan, Anushya, Yeong, Chai Hong and Acharya, U. Rajendra. 2025. "Artificial Intelligence for Ovarian Cancer Detection with Medical Images: A Review of the Last Decade (2013–2023)." Archives of Computational Methods in Engineering. https://doi.org/10.1007/s11831-025-10268-xZipper Pattern: An Investigation into Psychotic Criminal Detection Using EEG Signals
Tasci, Gulay, Barua, Prabal Datta, Tanko, Dahiru, Keles, Tugce, Tas, Suat, Tuncer, Ilknur, Kaya, Suheda, Yildirim, Kubra, Talu, Yunus, Tasci, Burak, Ozsoy, Filiz, Gonen, Nida, Tasci, Irem, Dogan, Sengul and Tuncer, Turker. 2025. "Zipper Pattern: An Investigation into Psychotic Criminal Detection Using EEG Signals." Diagnostics. 15 (2). https://doi.org/10.3390/diagnostics15020154FlexiCombFE: A flexible, combination-based feature engineering framework for brain tumor detection
Tuncer, Ilknur, Hafeez-Baig, Abdul, Barua, Prabal Datta, Hajiyeva, Rena, Massimo, Salvi, Dogan, Sengul, Tuncer, Turker and Acharya, U.R.. 2025. "FlexiCombFE: A flexible, combination-based feature engineering framework for brain tumor detection." Biomedical Signal Processing and Control. 104. https://doi.org/10.1016/j.bspc.2025.107538A dual-method approach using autoencoders and transductive learning for remaining useful life estimation
Yang, Jing, Boroojeni, Nika Anoosha, Chahardeh, Mehran Kazemi, Por, Lip Yee, Alizadehsani, Roohallah and Acharya, U. Rajendra. 2025. "A dual-method approach using autoencoders and transductive learning for remaining useful life estimation." Engineering Applications of Artificial Intelligence. 147. https://doi.org/10.1016/j.engappai.2025.110285EEGConvNeXt: A novel convolutional neural network model for automated detection of Alzheimer's Disease and Frontotemporal Dementia using EEG signals
Acharya, Madhav, Deo, Ravinesh C, Barua, Prabal Datta, Devi, Aruna and Tao, Xiaohui. 2025. "EEGConvNeXt: A novel convolutional neural network model for automated detection of Alzheimer's Disease and Frontotemporal Dementia using EEG signals." Computer Methods and Programs in Biomedicine. 262. https://doi.org/10.1016/j.cmpb.2025.108652A Dual-Stream Deep Learning Architecture With Adaptive Random Vector Functional Link for Multi-Center Ischemic Stroke Classification
Inamdar, Mahesh Anil, Gudigar, Anjan, Raghavendra, U., Salvi, Massimo, Aman, Raja Rizal Azman Bin Raja, Muhammad Gowdh, Nadia Fareeda, Ahir, Izzah Amirah Binti Mohd, Bin Kamaruddin, Mohd Salahuddin, Kadir, Khairul Azmi Abdul, Molinari, Filippo, Hegde, Ajay, Menon, Girish R. and Acharya, U. Rajendra. 2025. "A Dual-Stream Deep Learning Architecture With Adaptive Random Vector Functional Link for Multi-Center Ischemic Stroke Classification." IEEE Access. 13, pp. 46638-46658. https://doi.org/10.1109/ACCESS.2025.3550344Application of transfer learning for biomedical signals: A comprehensive review of the last decade (2014–2024)
Jafari, Mahboobeh, Tao, Xiaohui, Barua, Prabal, Tan, Ru-San and Acharya, U.Rajendra. 2025. "Application of transfer learning for biomedical signals: A comprehensive review of the last decade (2014–2024)." Information Fusion. 118. https://doi.org/10.1016/j.inffus.2025.102982BrainNeXt: novel lightweight CNN model for the automated detection of brain disorders using MRI images
Poyraz, Melahat, Poyraz, Ahmet Kursad, Dogan, Yusuf, Gunes, Selva, Mir, Hasan S., Paul, Jose Kunnel, Barua, Prabal Datta, Baygin, Mehmet, Dogan, Sengul, Tuncer, Turker, Molinari, Filippo and Acharya, Rajendra. 2025. "BrainNeXt: novel lightweight CNN model for the automated detection of brain disorders using MRI images." Cognitive Neurodynamics. 19 (1). https://doi.org/10.1007/s11571-025-10235-zA novel uncertainty-aware liquid neural network for noise-resilient time series forecasting and classification
Akpinar, Muhammed Halil, Atila, Orhan, Sengur, Abdulkadir, Salvi, Massimo and Acharya, U.R.. 2025. "A novel uncertainty-aware liquid neural network for noise-resilient time series forecasting and classification." Chaos, Solitons and Fractals. 193. https://doi.org/10.1016/j.chaos.2025.116130A novel complexity reduction technique using visibility relationship and perpendicular distance recursive refinement for physiological signals
Atila, Orhan, Akpinar, Muhammed Halil, Sengur, Abdulkadir and Acharya, U.R.. 2025. "A novel complexity reduction technique using visibility relationship and perpendicular distance recursive refinement for physiological signals." Communications in Nonlinear Science and Numerical Simulation. 145. https://doi.org/10.1016/j.cnsns.2025.108752MobileTransNeXt: Integrating CNN, transformer, and BiLSTM for image classification
Ye, Peishun, Lin, Jiyan, Kang, Yaming, Kaya, Tolga, Yildirim, Kubra, Hafeez Baig, Abdul, Aydemir, Emrah, Dogan, Sengul and Tuncer, Turker. 2025. "MobileTransNeXt: Integrating CNN, transformer, and BiLSTM for image classification." Alexandria Engineering Journal. 123, pp. 460-470. https://doi.org/10.1016/j.aej.2025.03.048Application of artificial intelligence in air pollution monitoring and forecasting: A systematic review
Chadalavada, Sreeni, Faust, Oliver, Salvi, Massimo, Seoni, Silvia, Raj, Nawin, Raghavendra, U., Gudigar, Anjan, Barua, Prabal Datta, Molinari, Filippo and Acharya, Rajendra. 2025. "Application of artificial intelligence in air pollution monitoring and forecasting: A systematic review." Environmental Modelling and Software. 185. https://doi.org/10.1016/j.envsoft.2024.106312Electricity demand uncertainty modeling with Temporal Convolution Neural Network models
Ghimire, Sujan, Deo, Ravinesh C., Casillas-Perez, David, Salcedo-Sanz, Sancho, Acharya, Rajendra and Dinh, Toan. 2025. "Electricity demand uncertainty modeling with Temporal Convolution Neural Network models." Renewable and Sustainable Energy Reviews. 209. https://doi.org/10.1016/j.rser.2024.115097Half-hourly electricity price prediction model with explainable-decomposition hybrid deep learning approach
Ghimire, Sujan, Deo, Ravinesh C., Hopf, Konstantin, Liu, Hangyue, Casillas-Perez, David, Helwig, Andreas, Prasad, Salvin S., Perez-Aracil, Jorge, Barua, Prabal Datta and Salcedo-Sanz, Sancho. 2025. "Half-hourly electricity price prediction model with explainable-decomposition hybrid deep learning approach." Energy and AI. 20. https://doi.org/10.1016/j.egyai.2025.100492Investigating Brain Lobe Biomarkers to Enhance Dementia Detection Using EEG Data
Siuly, Siuly, Tawhid, Md.Nurul Ahad, Li, Yan, Acharya, Rajendra, Sadiq, Muhammad Tariq and Wang, Hua. 2025. "Investigating Brain Lobe Biomarkers to Enhance Dementia Detection Using EEG Data." Cognitive Computation. 17 (2). https://doi.org/10.1007/s12559-025-10447-9Explainable hybrid deep learning framework for enhancing multi-step solar ultraviolet-B radiation predictions
Prasad, Salvin S., Joseph, Lionel P., Ghimire, Sujan, Deo, Ravinesh C., Downs, Nathan J., Acharya, Rajendra and Yaseen, Zaher M.. 2025. "Explainable hybrid deep learning framework for enhancing multi-step solar ultraviolet-B radiation predictions." Atmospheric Environment. 343. https://doi.org/10.1016/j.atmosenv.2024.120951CubicPat: Investigations on the Mental Performance and Stress Detection Using EEG Signals
Ince, Ugur, Talu, Yunus, Duz, Aleyna, Tas, Suat, Tanko, Dahiru, Tasci, Irem, Dogan, Sengul, Hafeez-Baig, Abdul, Aydemir, Emrah and Tuncer, Turker. 2025. "CubicPat: Investigations on the Mental Performance and Stress Detection Using EEG Signals." Diagnostics. 15 (3). https://doi.org/10.3390/diagnostics15030363Preserving privacy in healthcare: A systematic review of deep learning approaches for synthetic data generation
Li, Yintong, Acharya, U. Rajendra and Tan, Jen Hong. 2025. "Preserving privacy in healthcare: A systematic review of deep learning approaches for synthetic data generation." Computer Methods and Programs in Biomedicine. 260. https://doi.org/10.1016/j.cmpb.2024.108571Automated hip dysplasia detection using novel FlexiLBPHOG model with ultrasound images
Key, Sefa, Kurum, Huseyin, Esmez, Omer, Hafeez-Baig, Abdul, Hajiyeva, Rena, Dogan, Sengul and Tuncer, Turker. 2025. "Automated hip dysplasia detection using novel FlexiLBPHOG model with ultrasound images." Ain Shams Engineering Journal. 16 (1). https://doi.org/10.1016/j.asej.2024.103235Artificial Intelligence-Based Suicide Prevention and Prediction: A Systematic Review (2019-2023)
Atmakuru, Anirudh, Shahini, Alen, Chakraborty, Subrata, Seoni, Silvia, Salvi, Massimo, Hafeez-Baig, Abdul, Rashid, Sadaf, Tan, Ru San, Barua, Prabal Datta, Molinari, Filippo and Acharya, U Rajendra. 2025. "Artificial Intelligence-Based Suicide Prevention and Prediction: A Systematic Review (2019-2023)." Information Fusion. 114. https://doi.org/10.1016/j.inffus.2024.102673Explainable deeply-fused nets electricity demand prediction model: Factoring climate predictors for accuracy and deeper insights with probabilistic confidence interval and point-based forecasts
Ghimire, Sujan, AL-Musaylh, Mohanad S., Nguyen-Huy, Thong, Deo, Ravinesh C., Acharya, Rajendra, Casillas-Perez, David, Yaseen, Zaher Mundher and Salcedo-sanz, Sancho. 2025. "Explainable deeply-fused nets electricity demand prediction model: Factoring climate predictors for accuracy and deeper insights with probabilistic confidence interval and point-based forecasts." Applied Energy. 378 (Part A). https://doi.org/10.1016/j.apenergy.2024.124763AttentionPoolMobileNeXt: An automated construction damage detection model based on a new convolutional neural network and deep feature engineering models
Aydin, Mehmet, Barua, Prabal Datta, Chadalavada, Sreenivasulu, Dogan, Sengul, Tuncer, Turker, Chakraborty, Subrata and Acharya, Rajendra U.. 2025. "AttentionPoolMobileNeXt: An automated construction damage detection model based on a new convolutional neural network and deep feature engineering models." Multimedia Tools and Applications. 84 (4), pp. 1821-1843. https://doi.org/10.1007/s11042-024-19163-2Directed Lobish-based explainable feature engineering model with TTPat and CWINCA for EEG artifact classification
Tuncer, Turker, Dogan, Sengul, Baygin, Mehmet, Tasci, Irem, Mungen, Bulent, Tasci, Burak, Barua, Prabal Datta and Acharya, U.R.. 2024. "Directed Lobish-based explainable feature engineering model with TTPat and CWINCA for EEG artifact classification." Knowledge-Based Systems. 305. https://doi.org/10.1016/j.knosys.2024.112555Retinal Health Screening Using Artificial Intelligence with Digital Fundus Images: A Review of the Last Decade (2012-2023)
Islam, Saad, Deo, Ravinesh C., Barua, Prabal Datta, Soar, Jeffrey, Yu, Ping and Acharya, U. Rajendra. 2024. "Retinal Health Screening Using Artificial Intelligence with Digital Fundus Images: A Review of the Last Decade (2012-2023)." IEEE Access. 12, pp. 176630-176685. https://doi.org/10.1109/ACCESS.2024.3477420Automated EEG-based language detection using directed quantum pattern technique
Dogan, Sengul, Tuncer, Turker, Barua, Prabal Datta and Acharya, U.R.. 2024. "Automated EEG-based language detection using directed quantum pattern technique." Applied Soft Computing. 167 (Part A). https://doi.org/10.1016/j.asoc.2024.112301A Novel Hybrid Model for Automatic Non-Small Cell Lung Cancer Classification Using Histopathological Images
Katar, Oguzhan, Yildirim, Ozal, Tan, Ru-San and Acharya, U Rajendra. 2024. "A Novel Hybrid Model for Automatic Non-Small Cell Lung Cancer Classification Using Histopathological Images." Diagnostics. 14 (22). https://doi.org/10.3390/diagnostics14222497Synthetic Data Generation via Generative Adversarial Networks in Healthcare: A Systematic Review of Image- and Signal-Based Studies
Akpinar, Muhammed Halil, Sengur, Abdulkadir, Salvi, Massimo, Seoni, Silvia, Faust, Oliver, Mir, Hasan, Molinari,Filippo and Acharya, U. Rajendra. 2024. "Synthetic Data Generation via Generative Adversarial Networks in Healthcare: A Systematic Review of Image- and Signal-Based Studies." IEEE Open Journal of Engineering in Medicine and Biology. 6, pp. 183-192. https://doi.org/10.1109/OJEMB.2024.3508472RECOMED: A comprehensive pharmaceutical recommendation system
Zomorodi, Mariam, Ghodsollahee, Ismail, Martin, Jennifer H, Talley, Nicholas J, Salari, Vahid, Pławiak, Paweł, Rahimi, Kazem and Acharya, U.R.. 2024. "RECOMED: A comprehensive pharmaceutical recommendation system." Artificial Intelligence in Medicine. 157. https://doi.org/10.1016/j.artmed.2024.102981Artificial intelligence in assessing cardiovascular diseases and risk factors via retinal fundus images: A review of the last decade
Abdollahi, Mirsaeed, Jafarizadeh, Ali, Ghafouri-Asbagh, Amirhosein, Sobhi, Navid, Pourmoghtader, Keysan, Pedrammehr, Siamak, Asadi, Houshyar, Tan, Ru-San, Alizadehsani, Roohallah and Acharya, U. Rajendra. 2024. "Artificial intelligence in assessing cardiovascular diseases and risk factors via retinal fundus images: A review of the last decade." WIREs Data Mining and Knowledge Discovery. 14 (6). https://doi.org/10.1002/widm.1560Early prediction of sudden cardiac death using multimodal fusion of ECG Features extracted from Hilbert–Huang and wavelet transforms with explainable vision transformer and CNN models
Telangore, Hardik, Azad, Victor, Sharma, Manish, Bhurane, Ankit, Tan, Ru San and Acharya, U. Rajendra. 2024. "Early prediction of sudden cardiac death using multimodal fusion of ECG Features extracted from Hilbert–Huang and wavelet transforms with explainable vision transformer and CNN models." Computer Methods and Programs in Biomedicine. 257. https://doi.org/10.1016/j.cmpb.2024.108455A Pragmatic Approach to Fetal Monitoring via Cardiotocography Using Feature Elimination and Hyperparameter Optimization
Hardalac, Firat, Akmal, Haad, Ayturan, Kubilay, Acharya, U. Rajendra and Tan, Ru-San. 2024. "A Pragmatic Approach to Fetal Monitoring via Cardiotocography Using Feature Elimination and Hyperparameter Optimization." Interdisciplinary Sciences: Computational Life Sciences. 16 (4), pp. 882-906. https://doi.org/10.1007/s12539-024-00647-6Automated System for the Detection of Heart Anomalies Using Phonocardiograms: A Systematic Review
Gudigar, Anjan, Raghavendra, U., Maithri, M., Samanth, Jyothi, Inamdar, Mahesh Anil, Vidhya, V., Vicnesh, Jahmunah, Prabhu, Mukund A., Tan, Ru-San, Yeong, Chai Hong, Molinari, Filippo and Acharya, U. R.. 2024. "Automated System for the Detection of Heart Anomalies Using Phonocardiograms: A Systematic Review." IEEE Access. 12, pp. 138399-138428. https://doi.org/10.1109/ACCESS.2024.3465511Exploring the perspectives of Australian primary school teachers on students learning about project management
Delle-Vergini, Sante, Eacersall, Douglas, Dann, Chris, Ally, Mustafa and Chakraborty, Subrata. 2024. "Exploring the perspectives of Australian primary school teachers on students learning about project management." Issues in Educational Research. 34 (3), pp. 928-952.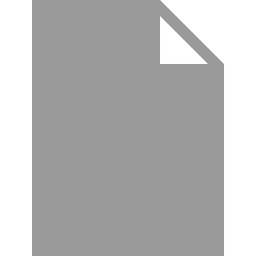