Exemplar Darknet19 feature generation technique for automated kidney stone detection with coronal CT images
Article
Article Title | Exemplar Darknet19 feature generation technique for automated kidney stone detection with coronal CT images |
---|---|
ERA Journal ID | 5031 |
Article Category | Article |
Authors | Baygin, Mehmet, Yaman, Orhan, Barua, Prabal Datta, Dogan, Sengul, Tuncer, Turker and Acharya, U. Rajendra |
Journal Title | Artificial Intelligence in Medicine |
Journal Citation | 127 |
Article Number | 102274 |
Number of Pages | 8 |
Year | 2022 |
Publisher | Elsevier |
Place of Publication | Netherlands |
ISSN | 0933-3657 |
1873-2860 | |
Digital Object Identifier (DOI) | https://doi.org/10.1016/j.artmed.2022.102274 |
Web Address (URL) | https://www.sciencedirect.com/science/article/pii/S0933365722000392 |
Abstract | Kidney stone is a commonly seen ailment and is usually detected by urologists using computed tomography (CT) images. It is difficult and time-consuming to detect small stones in CT images. Hence, an automated system can help clinicians to detect kidney stones accurately. In this work, a novel transfer learning-based image classification method (ExDark19) has been proposed to detect kidney stones using CT images. The iterative neighborhood component analysis (INCA) is employed to select the most informative feature vectors and these selected features vectors are fed to the k nearest neighbor (kNN) classifier to detect kidney stones with a ten-fold cross-validation (CV) strategy. The proposed ExDark19 model yielded an accuracy of 99.22% with 10-fold CV and 99.71% using the hold-out validation method. Our results demonstrate that the proposed ExDark19 detect kidney stones over 99% accuracies for two validation techniques. This developed automated system can assist the urologists to validate their manual screening of kidney stones and hence reduce the possible human error. |
Keywords | Biomedical image classification; ExDark19; INCA; Kidney stone detection; Pre-trained model; Transfer learning |
Contains Sensitive Content | Does not contain sensitive content |
ANZSRC Field of Research 2020 | 400306. Computational physiology |
Public Notes | Files associated with this item cannot be displayed due to copyright restrictions. |
Byline Affiliations | Ngee Ann Polytechnic, Singapore |
Singapore University of Social Sciences (SUSS), Singapore | |
Asia University, Taiwan | |
Ardahan University, Turkiye | |
Firat University, Turkey | |
School of Business | |
University of Technology Sydney | |
Cogninet Australia, Australia |
https://research.usq.edu.au/item/yyw99/exemplar-darknet19-feature-generation-technique-for-automated-kidney-stone-detection-with-coronal-ct-images
179
total views2
total downloads0
views this month0
downloads this month
Export as
Related outputs
Fibromyalgia Detection and Diagnosis: A Systematic Review of Data-Driven Approaches and Clinical Implications
Atmakuru, Anirudh, Chakraborty, Subrata, Salvi, Massimo, Faust, Oliver, Barua, Prabal Datta, Kobayashi, Makiko, Tan, Ru San, Molinari, Filippo, Hafeez-Baig, Abdul and Acharya, U. Rajendra. 2025. "Fibromyalgia Detection and Diagnosis: A Systematic Review of Data-Driven Approaches and Clinical Implications." IEEE Access. 13, pp. 25026-25044. https://doi.org/10.1109/ACCESS.2025.3539196Current and future roles of artificial intelligence in retinopathy of prematurity
Jafarizadeh, Ali, Maleki, Shadi Farabi, Pouya, Parnia, Sobhi, Navid, Abdollahi, Mirsaeed, Pedrammehr, Siamak, Lim, Chee Peng, Asadi, Houshyar, Alizadehsani, Roohallah, Tan, Ru-San, Islam, Sheikh Mohammed Shariful and Acharya, U. Rajendra. 2025. "Current and future roles of artificial intelligence in retinopathy of prematurity." Artificial Intelligence Review. 58 (6). https://doi.org/10.1007/s10462-025-11153-6Artificial Intelligence for Ovarian Cancer Detection with Medical Images: A Review of the Last Decade (2013–2023)
Naderi Yaghouti, Amir Reza, Shalbaf, Ahmad, Alizadehsani, Roohallah, Tan, Ru-San, Vijayananthan, Anushya, Yeong, Chai Hong and Acharya, U. Rajendra. 2025. "Artificial Intelligence for Ovarian Cancer Detection with Medical Images: A Review of the Last Decade (2013–2023)." Archives of Computational Methods in Engineering. https://doi.org/10.1007/s11831-025-10268-xZipper Pattern: An Investigation into Psychotic Criminal Detection Using EEG Signals
Tasci, Gulay, Barua, Prabal Datta, Tanko, Dahiru, Keles, Tugce, Tas, Suat, Tuncer, Ilknur, Kaya, Suheda, Yildirim, Kubra, Talu, Yunus, Tasci, Burak, Ozsoy, Filiz, Gonen, Nida, Tasci, Irem, Dogan, Sengul and Tuncer, Turker. 2025. "Zipper Pattern: An Investigation into Psychotic Criminal Detection Using EEG Signals." Diagnostics. 15 (2). https://doi.org/10.3390/diagnostics15020154FlexiCombFE: A flexible, combination-based feature engineering framework for brain tumor detection
Tuncer, Ilknur, Hafeez-Baig, Abdul, Barua, Prabal Datta, Hajiyeva, Rena, Massimo, Salvi, Dogan, Sengul, Tuncer, Turker and Acharya, U.R.. 2025. "FlexiCombFE: A flexible, combination-based feature engineering framework for brain tumor detection." Biomedical Signal Processing and Control. 104. https://doi.org/10.1016/j.bspc.2025.107538EEGConvNeXt: A novel convolutional neural network model for automated detection of Alzheimer's Disease and Frontotemporal Dementia using EEG signals
Acharya, Madhav, Deo, Ravinesh C, Barua, Prabal Datta, Devi, Aruna and Tao, Xiaohui. 2025. "EEGConvNeXt: A novel convolutional neural network model for automated detection of Alzheimer's Disease and Frontotemporal Dementia using EEG signals." Computer Methods and Programs in Biomedicine. 262. https://doi.org/10.1016/j.cmpb.2025.108652Application of transfer learning for biomedical signals: A comprehensive review of the last decade (2014–2024)
Jafari, Mahboobeh, Tao, Xiaohui, Barua, Prabal, Tan, Ru-San and Acharya, U.Rajendra. 2025. "Application of transfer learning for biomedical signals: A comprehensive review of the last decade (2014–2024)." Information Fusion. 118. https://doi.org/10.1016/j.inffus.2025.102982BrainNeXt: novel lightweight CNN model for the automated detection of brain disorders using MRI images
Poyraz, Melahat, Poyraz, Ahmet Kursad, Dogan, Yusuf, Gunes, Selva, Mir, Hasan S., Paul, Jose Kunnel, Barua, Prabal Datta, Baygin, Mehmet, Dogan, Sengul, Tuncer, Turker, Molinari, Filippo and Acharya, Rajendra. 2025. "BrainNeXt: novel lightweight CNN model for the automated detection of brain disorders using MRI images." Cognitive Neurodynamics. 19 (1). https://doi.org/10.1007/s11571-025-10235-zA novel uncertainty-aware liquid neural network for noise-resilient time series forecasting and classification
Akpinar, Muhammed Halil, Atila, Orhan, Sengur, Abdulkadir, Salvi, Massimo and Acharya, U.R.. 2025. "A novel uncertainty-aware liquid neural network for noise-resilient time series forecasting and classification." Chaos, Solitons and Fractals. 193. https://doi.org/10.1016/j.chaos.2025.116130A novel complexity reduction technique using visibility relationship and perpendicular distance recursive refinement for physiological signals
Atila, Orhan, Akpinar, Muhammed Halil, Sengur, Abdulkadir and Acharya, U.R.. 2025. "A novel complexity reduction technique using visibility relationship and perpendicular distance recursive refinement for physiological signals." Communications in Nonlinear Science and Numerical Simulation. 145. https://doi.org/10.1016/j.cnsns.2025.108752MobileTransNeXt: Integrating CNN, transformer, and BiLSTM for image classification
Ye, Peishun, Lin, Jiyan, Kang, Yaming, Kaya, Tolga, Yildirim, Kubra, Hafeez Baig, Abdul, Aydemir, Emrah, Dogan, Sengul and Tuncer, Turker. 2025. "MobileTransNeXt: Integrating CNN, transformer, and BiLSTM for image classification." Alexandria Engineering Journal. 123, pp. 460-470. https://doi.org/10.1016/j.aej.2025.03.048Application of artificial intelligence in air pollution monitoring and forecasting: A systematic review
Chadalavada, Sreeni, Faust, Oliver, Salvi, Massimo, Seoni, Silvia, Raj, Nawin, Raghavendra, U., Gudigar, Anjan, Barua, Prabal Datta, Molinari, Filippo and Acharya, Rajendra. 2025. "Application of artificial intelligence in air pollution monitoring and forecasting: A systematic review." Environmental Modelling and Software. 185. https://doi.org/10.1016/j.envsoft.2024.106312Electricity demand uncertainty modeling with Temporal Convolution Neural Network models
Ghimire, Sujan, Deo, Ravinesh C., Casillas-Perez, David, Salcedo-Sanz, Sancho, Acharya, Rajendra and Dinh, Toan. 2025. "Electricity demand uncertainty modeling with Temporal Convolution Neural Network models." Renewable and Sustainable Energy Reviews. 209. https://doi.org/10.1016/j.rser.2024.115097Half-hourly electricity price prediction model with explainable-decomposition hybrid deep learning approach
Ghimire, Sujan, Deo, Ravinesh C., Hopf, Konstantin, Liu, Hangyue, Casillas-Perez, David, Helwig, Andreas, Prasad, Salvin S., Perez-Aracil, Jorge, Barua, Prabal Datta and Salcedo-Sanz, Sancho. 2025. "Half-hourly electricity price prediction model with explainable-decomposition hybrid deep learning approach." Energy and AI. 20. https://doi.org/10.1016/j.egyai.2025.100492Investigating Brain Lobe Biomarkers to Enhance Dementia Detection Using EEG Data
Siuly, Siuly, Tawhid, Md.Nurul Ahad, Li, Yan, Acharya, Rajendra, Sadiq, Muhammad Tariq and Wang, Hua. 2025. "Investigating Brain Lobe Biomarkers to Enhance Dementia Detection Using EEG Data." Cognitive Computation. 17 (2). https://doi.org/10.1007/s12559-025-10447-9Explainable hybrid deep learning framework for enhancing multi-step solar ultraviolet-B radiation predictions
Prasad, Salvin S., Joseph, Lionel P., Ghimire, Sujan, Deo, Ravinesh C., Downs, Nathan J., Acharya, Rajendra and Yaseen, Zaher M.. 2025. "Explainable hybrid deep learning framework for enhancing multi-step solar ultraviolet-B radiation predictions." Atmospheric Environment. 343. https://doi.org/10.1016/j.atmosenv.2024.120951CubicPat: Investigations on the Mental Performance and Stress Detection Using EEG Signals
Ince, Ugur, Talu, Yunus, Duz, Aleyna, Tas, Suat, Tanko, Dahiru, Tasci, Irem, Dogan, Sengul, Hafeez-Baig, Abdul, Aydemir, Emrah and Tuncer, Turker. 2025. "CubicPat: Investigations on the Mental Performance and Stress Detection Using EEG Signals." Diagnostics. 15 (3). https://doi.org/10.3390/diagnostics15030363Preserving privacy in healthcare: A systematic review of deep learning approaches for synthetic data generation
Li, Yintong, Acharya, U. Rajendra and Tan, Jen Hong. 2025. "Preserving privacy in healthcare: A systematic review of deep learning approaches for synthetic data generation." Computer Methods and Programs in Biomedicine. 260. https://doi.org/10.1016/j.cmpb.2024.108571Automated hip dysplasia detection using novel FlexiLBPHOG model with ultrasound images
Key, Sefa, Kurum, Huseyin, Esmez, Omer, Hafeez-Baig, Abdul, Hajiyeva, Rena, Dogan, Sengul and Tuncer, Turker. 2025. "Automated hip dysplasia detection using novel FlexiLBPHOG model with ultrasound images." Ain Shams Engineering Journal. 16 (1). https://doi.org/10.1016/j.asej.2024.103235Artificial Intelligence-Based Suicide Prevention and Prediction: A Systematic Review (2019-2023)
Atmakuru, Anirudh, Shahini, Alen, Chakraborty, Subrata, Seoni, Silvia, Salvi, Massimo, Hafeez-Baig, Abdul, Rashid, Sadaf, Tan, Ru San, Barua, Prabal Datta, Molinari, Filippo and Acharya, U Rajendra. 2025. "Artificial Intelligence-Based Suicide Prevention and Prediction: A Systematic Review (2019-2023)." Information Fusion. 114. https://doi.org/10.1016/j.inffus.2024.102673Explainable deeply-fused nets electricity demand prediction model: Factoring climate predictors for accuracy and deeper insights with probabilistic confidence interval and point-based forecasts
Ghimire, Sujan, AL-Musaylh, Mohanad S., Nguyen-Huy, Thong, Deo, Ravinesh C., Acharya, Rajendra, Casillas-Perez, David, Yaseen, Zaher Mundher and Salcedo-sanz, Sancho. 2025. "Explainable deeply-fused nets electricity demand prediction model: Factoring climate predictors for accuracy and deeper insights with probabilistic confidence interval and point-based forecasts." Applied Energy. 378 (Part A). https://doi.org/10.1016/j.apenergy.2024.124763AttentionPoolMobileNeXt: An automated construction damage detection model based on a new convolutional neural network and deep feature engineering models
Aydin, Mehmet, Barua, Prabal Datta, Chadalavada, Sreenivasulu, Dogan, Sengul, Tuncer, Turker, Chakraborty, Subrata and Acharya, Rajendra U.. 2025. "AttentionPoolMobileNeXt: An automated construction damage detection model based on a new convolutional neural network and deep feature engineering models." Multimedia Tools and Applications. 84 (4), pp. 1821-1843. https://doi.org/10.1007/s11042-024-19163-2Directed Lobish-based explainable feature engineering model with TTPat and CWINCA for EEG artifact classification
Tuncer, Turker, Dogan, Sengul, Baygin, Mehmet, Tasci, Irem, Mungen, Bulent, Tasci, Burak, Barua, Prabal Datta and Acharya, U.R.. 2024. "Directed Lobish-based explainable feature engineering model with TTPat and CWINCA for EEG artifact classification." Knowledge-Based Systems. 305. https://doi.org/10.1016/j.knosys.2024.112555Retinal Health Screening Using Artificial Intelligence with Digital Fundus Images: A Review of the Last Decade (2012-2023)
Islam, Saad, Deo, Ravinesh C., Barua, Prabal Datta, Soar, Jeffrey, Yu, Ping and Acharya, U. Rajendra. 2024. "Retinal Health Screening Using Artificial Intelligence with Digital Fundus Images: A Review of the Last Decade (2012-2023)." IEEE Access. 12, pp. 176630-176685. https://doi.org/10.1109/ACCESS.2024.3477420Automated EEG-based language detection using directed quantum pattern technique
Dogan, Sengul, Tuncer, Turker, Barua, Prabal Datta and Acharya, U.R.. 2024. "Automated EEG-based language detection using directed quantum pattern technique." Applied Soft Computing. 167 (Part A). https://doi.org/10.1016/j.asoc.2024.112301A Novel Hybrid Model for Automatic Non-Small Cell Lung Cancer Classification Using Histopathological Images
Katar, Oguzhan, Yildirim, Ozal, Tan, Ru-San and Acharya, U Rajendra. 2024. "A Novel Hybrid Model for Automatic Non-Small Cell Lung Cancer Classification Using Histopathological Images." Diagnostics. 14 (22). https://doi.org/10.3390/diagnostics14222497Synthetic Data Generation via Generative Adversarial Networks in Healthcare: A Systematic Review of Image- and Signal-Based Studies
Akpinar, Muhammed Halil, Sengur, Abdulkadir, Salvi, Massimo, Seoni, Silvia, Faust, Oliver, Mir, Hasan, Molinari,Filippo and Acharya, U. Rajendra. 2024. "Synthetic Data Generation via Generative Adversarial Networks in Healthcare: A Systematic Review of Image- and Signal-Based Studies." IEEE Open Journal of Engineering in Medicine and Biology. 6, pp. 183-192. https://doi.org/10.1109/OJEMB.2024.3508472RECOMED: A comprehensive pharmaceutical recommendation system
Zomorodi, Mariam, Ghodsollahee, Ismail, Martin, Jennifer H, Talley, Nicholas J, Salari, Vahid, Pławiak, Paweł, Rahimi, Kazem and Acharya, U.R.. 2024. "RECOMED: A comprehensive pharmaceutical recommendation system." Artificial Intelligence in Medicine. 157. https://doi.org/10.1016/j.artmed.2024.102981Artificial intelligence in assessing cardiovascular diseases and risk factors via retinal fundus images: A review of the last decade
Abdollahi, Mirsaeed, Jafarizadeh, Ali, Ghafouri-Asbagh, Amirhosein, Sobhi, Navid, Pourmoghtader, Keysan, Pedrammehr, Siamak, Asadi, Houshyar, Tan, Ru-San, Alizadehsani, Roohallah and Acharya, U. Rajendra. 2024. "Artificial intelligence in assessing cardiovascular diseases and risk factors via retinal fundus images: A review of the last decade." WIREs Data Mining and Knowledge Discovery. 14 (6). https://doi.org/10.1002/widm.1560Early prediction of sudden cardiac death using multimodal fusion of ECG Features extracted from Hilbert–Huang and wavelet transforms with explainable vision transformer and CNN models
Telangore, Hardik, Azad, Victor, Sharma, Manish, Bhurane, Ankit, Tan, Ru San and Acharya, U. Rajendra. 2024. "Early prediction of sudden cardiac death using multimodal fusion of ECG Features extracted from Hilbert–Huang and wavelet transforms with explainable vision transformer and CNN models." Computer Methods and Programs in Biomedicine. 257. https://doi.org/10.1016/j.cmpb.2024.108455A Pragmatic Approach to Fetal Monitoring via Cardiotocography Using Feature Elimination and Hyperparameter Optimization
Hardalac, Firat, Akmal, Haad, Ayturan, Kubilay, Acharya, U. Rajendra and Tan, Ru-San. 2024. "A Pragmatic Approach to Fetal Monitoring via Cardiotocography Using Feature Elimination and Hyperparameter Optimization." Interdisciplinary Sciences: Computational Life Sciences. 16 (4), pp. 882-906. https://doi.org/10.1007/s12539-024-00647-6Automated System for the Detection of Heart Anomalies Using Phonocardiograms: A Systematic Review
Gudigar, Anjan, Raghavendra, U., Maithri, M., Samanth, Jyothi, Inamdar, Mahesh Anil, Vidhya, V., Vicnesh, Jahmunah, Prabhu, Mukund A., Tan, Ru-San, Yeong, Chai Hong, Molinari, Filippo and Acharya, U. R.. 2024. "Automated System for the Detection of Heart Anomalies Using Phonocardiograms: A Systematic Review." IEEE Access. 12, pp. 138399-138428. https://doi.org/10.1109/ACCESS.2024.3465511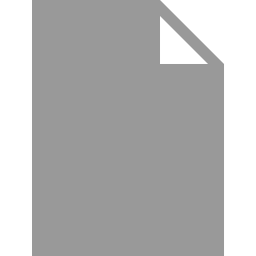