Automated Detection of Gastrointestinal Diseases Using Resnet50*-Based Explainable Deep Feature Engineering Model with Endoscopy Images
Article
Article Title | Automated Detection of Gastrointestinal Diseases Using Resnet50*-Based Explainable Deep Feature Engineering Model with Endoscopy Images |
---|---|
ERA Journal ID | 34304 |
Article Category | Article |
Authors | Cambay, Veysel Yusu, Barua, Prabal Dat, Hafeez Baig, Abdul, Dogan, Sengul, Baygin, Mehmet, Tuncer, Turker and Acharya, U. R. |
Journal Title | Sensors |
Journal Citation | 24 (23) |
Number of Pages | 23 |
Year | 2024 |
Publisher | MDPI AG |
Place of Publication | Switzerland |
ISSN | 1424-8220 |
1424-8239 | |
Digital Object Identifier (DOI) | https://doi.org/https://doi.org/10.3390/s24237710 |
Web Address (URL) | https://www.mdpi.com/1424-8220/24/23/7710 |
Abstract | This work aims to develop a novel convolutional neural network (CNN) named ResNet50* to detect various gastrointestinal diseases using a new ResNet50*-based deep feature engineering model with endoscopy images. The novelty of this work is the development of ResNet50*, a new variant of the ResNet model, featuring convolution-based residual blocks and a pooling-based attention mechanism similar to PoolFormer. Using ResNet50*, a gastrointestinal image dataset was trained, and an explainable deep feature engineering (DFE) model was developed. This DFE model comprises four primary stages: (i) feature extraction, (ii) iterative feature selection, (iii) classification using shallow classifiers, and (iv) information fusion. The DFE model is self-organizing, producing 14 different outcomes (8 classifier-specific and 6 voted) and selecting the most effective result as the final decision. During feature extraction, heatmaps are identified using gradient-weighted class activation mapping (Grad-CAM) with features derived from these regions via the final global average pooling layer of the pretrained ResNet50*. Four iterative feature selectors are employed in the feature selection stage to obtain distinct feature vectors. The classifiers k-nearest neighbors (kNN) and support vector machine (SVM) are used to produce specific outcomes. Iterative majority voting is employed in the final stage to obtain voted outcomes using the top result determined by the greedy algorithm based on classification accuracy. The presented ResNet50* was trained on an augmented version of the Kvasir dataset, and its performance was tested using Kvasir, Kvasir version 2, and wireless capsule endoscopy (WCE) curated colon disease image datasets. Our proposed ResNet50* model demonstrated a classification accuracy of more than 92% for all three datasets and a remarkable 99.13% accuracy for the WCE dataset. These findings affirm the superior classification ability of the ResNet50* model and confirm the generalizability of the developed architecture, showing consistent performance across all three distinct datasets. |
Keywords | ResNet50*; colon disease classification; deep feature engineering; multiple iterative feature selection |
Contains Sensitive Content | Does not contain sensitive content |
ANZSRC Field of Research 2020 | 420302. Digital health |
Byline Affiliations | Firat University, Turkey |
Mus Alparslan University, Turkey | |
School of Business | |
School of Management and Enterprise | |
Erzurum Technical University, Turkey | |
School of Mathematics, Physics and Computing |
https://research.usq.edu.au/item/zqqv7/automated-detection-of-gastrointestinal-diseases-using-resnet50-based-explainable-deep-feature-engineering-model-with-endoscopy-images
Download files
4
total views1
total downloads4
views this month1
downloads this month
Export as
Related outputs
Automated hip dysplasia detection using novel FlexiLBPHOG model with ultrasound images
Key, Sefa, Kurum, Huseyin, Esmez, Omer, Hafeez-Baig, Abdul, Hajiyeva, Rena, Dogan, Sengul and Tuncer, Turker. 2025. "Automated hip dysplasia detection using novel FlexiLBPHOG model with ultrasound images." Ain Shams Engineering Journal. 16 (1). https://doi.org/10.1016/j.asej.2024.103235Artificial Intelligence-Based Suicide Prevention and Prediction: A Systematic Review (2019-2023)
Atmakuru, Anirudh, Shahini, Alen, Chakraborty, Subrata, Seoni, Silvia, Salvi, Massimo, Hafeez-Baig, Abdul, Rashid, Sadaf, Tan, Ru San, Barua, Prabal Datta, Molinari, Filippo and Acharya, U Rajendra. 2025. "Artificial Intelligence-Based Suicide Prevention and Prediction: A Systematic Review (2019-2023)." Information Fusion. 114. https://doi.org/10.1016/j.inffus.2024.102673Explainable deeply-fused nets electricity demand prediction model: Factoring climate predictors for accuracy and deeper insights with probabilistic confidence interval and point-based forecasts
Ghimire, Sujan, AL-Musaylh, Mohanad S., Nguyen-Huy, Thong, Deo, Ravinesh C., Acharya, Rajendra, Casillas-Perez, David, Yaseen, Zaher Mundher and Salcedo-sanz, Sancho. 2025. "Explainable deeply-fused nets electricity demand prediction model: Factoring climate predictors for accuracy and deeper insights with probabilistic confidence interval and point-based forecasts." Applied Energy. 378 (Part A). https://doi.org/10.1016/j.apenergy.2024.124763Dr Abdul Hafeez-Baig
Hafeez-Baig, A.. Dr Abdul Hafeez-Baig.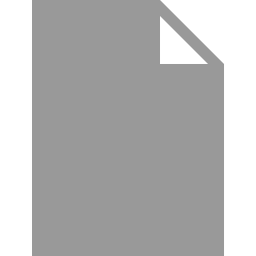