DSWIN: Automated hunger detection model based on hand-crafted decomposed shifted windows architecture using EEG signals
Article
Kirik, Serkan, Tasci, Irem, Barua, Prabal D., Yildiz, Arif Metehan, Keles, Tugce, Baygin, Mehmet, Tuncer, Ilknur, Dogan, Sengul, Tuncer, Turker, Devi, Aruna, Tan, Ru-San and Acharya, U.R.. 2024. "DSWIN: Automated hunger detection model based on hand-crafted decomposed shifted windows architecture using EEG signals." Knowledge-Based Systems. 300. https://doi.org/10.1016/j.knosys.2024.112150
Article Title | DSWIN: Automated hunger detection model based on hand-crafted decomposed shifted windows architecture using EEG signals |
---|---|
ERA Journal ID | 18062 |
Article Category | Article |
Authors | Kirik, Serkan, Tasci, Irem, Barua, Prabal D., Yildiz, Arif Metehan, Keles, Tugce, Baygin, Mehmet, Tuncer, Ilknur, Dogan, Sengul, Tuncer, Turker, Devi, Aruna, Tan, Ru-San and Acharya, U.R. |
Journal Title | Knowledge-Based Systems |
Journal Citation | 300 |
Article Number | 112150 |
Number of Pages | 13 |
Year | 2024 |
Publisher | Elsevier |
ISSN | 0950-7051 |
1872-7409 | |
Digital Object Identifier (DOI) | https://doi.org/10.1016/j.knosys.2024.112150 |
Web Address (URL) | https://www.sciencedirect.com/science/article/abs/pii/S0950705124007846 |
Abstract | Hunger is a physiological state that arises from complex interactions of multiple factors, including higher brain center control. We purposed to develop an accurate and efficient machine-learning model for the automated detection of hunger using EEG signals. We prospectively acquired 14-channel EEG (sampling frequency 128 Hz) from 43 and 48 fasted and post-prandial healthy subjects (hungry vs. control groups, respectively) using the EMOTIV EPOC+ mobile brain cap system. To augment the hunger response, fasted subjects were also shown video images of food during EEG recording. The EEG signals were divided into 15-second segments. 877 and 852 participants/subjects were in the hungry and control groups. We created a novel handcrafted architecture—decomposed shifted window (DSWIN)—that combined swin patch division with tunable Q-factor wavelet transform-based signal decomposition for multilevel feature extraction of EEG signals. Textural and statistical features were extracted from the multiple patches and decomposed signals using a novel penta pattern-based extractor and statistical moments, respectively, and then merged. Iterative neighborhood component analysis (INCA) and iterative ReliefF (IRF) were applied. Twenty-eight selected feature vectors were generated, which were then fed to a shallow k-nearest neighbors (kNN) classifier to calculate channel-wise prediction vectors. From the 28 channel-wise prediction vectors, another 26 modes of function-based voted results were calculated using iterative hard majority voting, and the best overall model result was selected using a greedy algorithm. Our model attained 99.54% and 82.71% binary classification accuracies of hungry status vs. control using 10-fold and leave-one-subject-out cross-validations, respectively. |
Keywords | DSWIN architecture; EEG; Hunger detection ; Penta pattern ; Neuroscience |
Contains Sensitive Content | Does not contain sensitive content |
ANZSRC Field of Research 2020 | 460207. Modelling and simulation |
Public Notes | Files associated with this item cannot be displayed due to copyright restrictions. |
Byline Affiliations | Elazig Fethi Sekin City Hospital, Turkey |
Firat University, Turkey | |
School of Business | |
Erzurum Technical University, Turkey | |
Interior Ministry, Turkiye | |
University of the Sunshine Coast | |
National Heart Centre, Singapore | |
Duke-NUS Medical Centre, Singapore | |
School of Mathematics, Physics and Computing | |
Centre for Health Research |
Permalink -
https://research.usq.edu.au/item/z850w/dswin-automated-hunger-detection-model-based-on-hand-crafted-decomposed-shifted-windows-architecture-using-eeg-signals
45
total views0
total downloads0
views this month0
downloads this month
Export as
Related outputs
Automated hip dysplasia detection using novel FlexiLBPHOG model with ultrasound images
Key, Sefa, Kurum, Huseyin, Esmez, Omer, Hafeez-Baig, Abdul, Hajiyeva, Rena, Dogan, Sengul and Tuncer, Turker. 2025. "Automated hip dysplasia detection using novel FlexiLBPHOG model with ultrasound images." Ain Shams Engineering Journal. 16 (1). https://doi.org/10.1016/j.asej.2024.103235Artificial Intelligence-Based Suicide Prevention and Prediction: A Systematic Review (2019-2023)
Atmakuru, Anirudh, Shahini, Alen, Chakraborty, Subrata, Seoni, Silvia, Salvi, Massimo, Hafeez-Baig, Abdul, Rashid, Sadaf, Tan, Ru San, Barua, Prabal Datta, Molinari, Filippo and Acharya, U Rajendra. 2025. "Artificial Intelligence-Based Suicide Prevention and Prediction: A Systematic Review (2019-2023)." Information Fusion. 114. https://doi.org/10.1016/j.inffus.2024.102673Explainable deeply-fused nets electricity demand prediction model: Factoring climate predictors for accuracy and deeper insights with probabilistic confidence interval and point-based forecasts
Ghimire, Sujan, AL-Musaylh, Mohanad S., Nguyen-Huy, Thong, Deo, Ravinesh C., Acharya, Rajendra, Casillas-Perez, David, Yaseen, Zaher Mundher and Salcedo-sanz, Sancho. 2025. "Explainable deeply-fused nets electricity demand prediction model: Factoring climate predictors for accuracy and deeper insights with probabilistic confidence interval and point-based forecasts." Applied Energy. 378 (Part A). https://doi.org/10.1016/j.apenergy.2024.124763AttentionPoolMobileNeXt: An automated construction damage detection model based on a new convolutional neural network and deep feature engineering models
Aydin, Mehmet, Barua, Prabal Datta, Chadalavada, Sreenivasulu, Dogan, Sengul, Tuncer, Turker, Chakraborty, Subrata and Acharya, Rajendra U.. 2025. "AttentionPoolMobileNeXt: An automated construction damage detection model based on a new convolutional neural network and deep feature engineering models." Multimedia Tools and Applications. 84 (4), pp. 1821-1843. https://doi.org/10.1007/s11042-024-19163-2Directed Lobish-based explainable feature engineering model with TTPat and CWINCA for EEG artifact classification
Tuncer, Turker, Dogan, Sengul, Baygin, Mehmet, Tasci, Irem, Mungen, Bulent, Tasci, Burak, Barua, Prabal Datta and Acharya, U.R.. 2024. "Directed Lobish-based explainable feature engineering model with TTPat and CWINCA for EEG artifact classification." Knowledge-Based Systems. 305. https://doi.org/10.1016/j.knosys.2024.112555Retinal Health Screening Using Artificial Intelligence with Digital Fundus Images: A Review of the Last Decade (2012-2023)
Islam, Saad, Deo, Ravinesh C., Barua, Prabal Datta, Soar, Jeffrey, Yu, Ping and Acharya, U. Rajendra. 2024. "Retinal Health Screening Using Artificial Intelligence with Digital Fundus Images: A Review of the Last Decade (2012-2023)." IEEE Access. 12, pp. 176630-176685. https://doi.org/10.1109/ACCESS.2024.3477420Automated EEG-based language detection using directed quantum pattern technique
Dogan, Sengul, Tuncer, Turker, Barua, Prabal Datta and Acharya, U.R.. 2024. "Automated EEG-based language detection using directed quantum pattern technique." Applied Soft Computing. 167 (Part A). https://doi.org/10.1016/j.asoc.2024.112301A Novel Hybrid Model for Automatic Non-Small Cell Lung Cancer Classification Using Histopathological Images
Katar, Oguzhan, Yildirim, Ozal, Tan, Ru-San and Acharya, U Rajendra. 2024. "A Novel Hybrid Model for Automatic Non-Small Cell Lung Cancer Classification Using Histopathological Images." Diagnostics. 14 (22). https://doi.org/10.3390/diagnostics14222497Synthetic Data Generation via Generative Adversarial Networks in Healthcare: A Systematic Review of Image- and Signal-Based Studies
Akpinar, Muhammed Halil, Sengur, Abdulkadir, Salvi, Massimo, Seoni, Silvia, Faust, Oliver, Mir, Hasan, Molinari,Filippo and Acharya, U. Rajendra. 2024. "Synthetic Data Generation via Generative Adversarial Networks in Healthcare: A Systematic Review of Image- and Signal-Based Studies." IEEE Open Journal of Engineering in Medicine and Biology. 6, pp. 183-192. https://doi.org/10.1109/OJEMB.2024.3508472RECOMED: A comprehensive pharmaceutical recommendation system
Zomorodi, Mariam, Ghodsollahee, Ismail, Martin, Jennifer H, Talley, Nicholas J, Salari, Vahid, Pławiak, Paweł, Rahimi, Kazem and Acharya, U.R.. 2024. "RECOMED: A comprehensive pharmaceutical recommendation system." Artificial Intelligence in Medicine. 157. https://doi.org/10.1016/j.artmed.2024.102981Artificial intelligence in assessing cardiovascular diseases and risk factors via retinal fundus images: A review of the last decade
Abdollahi, Mirsaeed, Jafarizadeh, Ali, Ghafouri-Asbagh, Amirhosein, Sobhi, Navid, Pourmoghtader, Keysan, Pedrammehr, Siamak, Asadi, Houshyar, Tan, Ru-San, Alizadehsani, Roohallah and Acharya, U. Rajendra. 2024. "Artificial intelligence in assessing cardiovascular diseases and risk factors via retinal fundus images: A review of the last decade." WIREs Data Mining and Knowledge Discovery. 14 (6). https://doi.org/10.1002/widm.1560Early prediction of sudden cardiac death using multimodal fusion of ECG Features extracted from Hilbert–Huang and wavelet transforms with explainable vision transformer and CNN models
Telangore, Hardik, Azad, Victor, Sharma, Manish, Bhurane, Ankit, Tan, Ru San and Acharya, U. Rajendra. 2024. "Early prediction of sudden cardiac death using multimodal fusion of ECG Features extracted from Hilbert–Huang and wavelet transforms with explainable vision transformer and CNN models." Computer Methods and Programs in Biomedicine. 257. https://doi.org/10.1016/j.cmpb.2024.108455A Pragmatic Approach to Fetal Monitoring via Cardiotocography Using Feature Elimination and Hyperparameter Optimization
Hardalac, Firat, Akmal, Haad, Ayturan, Kubilay, Acharya, U. Rajendra and Tan, Ru-San. 2024. "A Pragmatic Approach to Fetal Monitoring via Cardiotocography Using Feature Elimination and Hyperparameter Optimization." Interdisciplinary Sciences: Computational Life Sciences. 16 (4), pp. 882-906. https://doi.org/10.1007/s12539-024-00647-6Automated System for the Detection of Heart Anomalies Using Phonocardiograms: A Systematic Review
Gudigar, Anjan, Raghavendra, U., Maithri, M., Samanth, Jyothi, Inamdar, Mahesh Anil, Vidhya, V., Vicnesh, Jahmunah, Prabhu, Mukund A., Tan, Ru-San, Yeong, Chai Hong, Molinari, Filippo and Acharya, U. R.. 2024. "Automated System for the Detection of Heart Anomalies Using Phonocardiograms: A Systematic Review." IEEE Access. 12, pp. 138399-138428. https://doi.org/10.1109/ACCESS.2024.3465511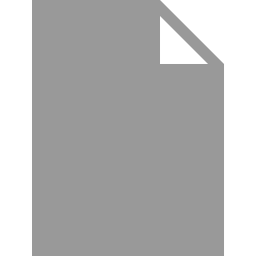