Forecasting Multi-Step Soil Moisture with Three-Phase Hybrid Wavelet-Least Absolute Shrinkage Selection Operator-Long Short-Term Memory Network (moDWT-Lasso-LSTM) Model
Article
Article Title | Forecasting Multi-Step Soil Moisture with Three-Phase Hybrid Wavelet-Least Absolute Shrinkage Selection Operator-Long Short-Term Memory Network (moDWT-Lasso-LSTM) Model |
---|---|
Article Category | Article |
Authors | Jayasinghe, W. J. M. Lakmini Prarthana, Deo, Ravinesh C., Raj, Nawin, Ghimire, Sujan, Yaseen, Zaher Mundher, Nguyen-Huy, Thong and Ghahramani, Afshin |
Journal Title | Water |
Journal Citation | 16 (21), p. 3133 |
Number of Pages | 27 |
Year | 2024 |
Publisher | MDPI AG |
Place of Publication | Switzerland |
ISSN | 2073-4441 |
Digital Object Identifier (DOI) | https://doi.org/10.3390/w16213133 |
Web Address (URL) | https://www.mdpi.com/2073-4441/16/21/3133 |
Abstract | To develop agricultural risk management strategies, the early identification of water deficits during the growing cycle is critical. This research proposes a deep learning hybrid approach for multi-step soil moisture forecasting in the Bundaberg region in Queensland, Australia, with predictions made for 1-day, 14-day, and 30-day, intervals. The model integrates Geospatial Interactive Online Visualization and Analysis Infrastructure (Giovanni) satellite data with ground observations. Due to the periodicity, transience, and trends in soil moisture of the top layer, time series datasets were complex. Hence, the Maximum Overlap Discrete Wavelet Transform (moDWT) method was adopted for data decomposition to identify the best correlated wavelet and scaling coefficients of the predictor variables with the target top layer moisture. The proposed 3-phase hybrid moDWT-Lasso-LSTM model used the Least Absolute Shrinkage and Selection Operator (Lasso) method for feature selection. Optimal hyperparameters were identified using the Hyperopt algorithm with deep learning LSTM method. This proposed model’s performances were compared with benchmarked machine learning (ML) models. In total, nine models were developed, including three standalone models (e.g., LSTM), three integrated feature selection models (e.g., Lasso-LSTM), and three hybrid models incorporating wavelet decomposition and feature selection (e.g., moDWT-Lasso-LSTM). Compared to alternative models, the hybrid deep moDWT-Lasso-LSTM produced the superior predictive model across statistical performance metrics. For example, at 1-day forecast, The moDWT-Lasso-LSTM model exhibits the highest accuracy with the highest 𝑅2≈0.92469 and the lowest RMSE ≈0.97808, MAE ≈0.76623, and SMAPE ≈4.39700%, outperforming other models. The moDWT-Lasso-DNN model follows closely, while the Lasso-ANN and Lasso-DNN models show lower accuracy with higher RMSE and MAE values. The ANN and DNN models have the lowest performance, with higher error metrics and lower R2 values compared to the deep learning models incorporating moDWT and Lasso techniques. This research emphasizes the utility of the advanced complementary ML model, such as the developed moDWT-Lasso-LSTM 3-phase hybrid model, as a robust data-driven tool for early forecasting of soil moisture. |
Keywords | soil moisture model; deep learning; hybrid models; artificial intelligence; wavelet transform |
Contains Sensitive Content | Does not contain sensitive content |
ANZSRC Field of Research 2020 | 410402. Environmental assessment and monitoring |
370704. Surface water hydrology | |
460207. Modelling and simulation | |
Byline Affiliations | School of Mathematics, Physics and Computing |
King Fahd University of Petroleum and Minerals, Saudi Arabia | |
Centre for Applied Climate Sciences | |
Thanh Do University, Vietnam | |
Queensland Government, Queensland |
https://research.usq.edu.au/item/zq290/forecasting-multi-step-soil-moisture-with-three-phase-hybrid-wavelet-least-absolute-shrinkage-selection-operator-long-short-term-memory-network-modwt-lasso-lstm-model
Download files
4
total views1
total downloads4
views this month1
downloads this month
Export as
Related outputs
Explainable deeply-fused nets electricity demand prediction model: Factoring climate predictors for accuracy and deeper insights with probabilistic confidence interval and point-based forecasts
Ghimire, Sujan, AL-Musaylh, Mohanad S., Nguyen-Huy, Thong, Deo, Ravinesh C., Acharya, Rajendra, Casillas-Perez, David, Yaseen, Zaher Mundher and Salcedo-sanz, Sancho. 2025. "Explainable deeply-fused nets electricity demand prediction model: Factoring climate predictors for accuracy and deeper insights with probabilistic confidence interval and point-based forecasts." Applied Energy. 378 (Part A). https://doi.org/10.1016/j.apenergy.2024.124763Tree shade within urban parks and nature strips: standards and perspectives from measurement campaigns conducted in Queensland
Downs, Nathan, Antrobus, Jodie, Dekeyser, Stijn, Butler, Harry, Downs, Melanie, Hickmott, Darren, Madigan, Chris, Brown, Alexandra, Amar, Abdurazaq, Dearnaley, John, Parisi, Alfio V, Vanos, Jennifer, Raj, Nawin, Harrison, Simone and Igoe, Damien. 2024. "Tree shade within urban parks and nature strips: standards and perspectives from measurement campaigns conducted in Queensland ." 6th International conference UV and Skin Cancer Prevention. Brisbane, Australia 11 - 15 Sep 2024 Australia.Spatiotemporal performance evaluation of high-resolution multiple satellite and reanalysis precipitation products over the semiarid region of India
Devadarshini, Elangovan, Bhuvaneswari, Kulanthaivelu, Kumar, Shanmugam Mohan, Geethalakshmi, Vellingiri, Dhasarathan, Manickam, Senthil, Alagarsamy, Senthilraja, Kandasamy, Mushtaq, Shahbaz, Nguyen-Huy, Thong, Mai, Thanh and Kouadio, Louis. 2024. "Spatiotemporal performance evaluation of high-resolution multiple satellite and reanalysis precipitation products over the semiarid region of India." Environmental Monitoring and Assessment. 196. https://doi.org/10.1007/s10661-024-13152-6Harnessing a systems approach for sustainable adaptation in vulnerable mega-deltas: A case study of the dyke heightening program in the Vietnamese Mekong Delta's floodplains
Mai, Thanh, Mushtaq, Shahbaz, Tong, Yen Dan, Nguyen-Huy, Thong, Richards, Russell and Marcussen, Torben. 2024. "Harnessing a systems approach for sustainable adaptation in vulnerable mega-deltas: A case study of the dyke heightening program in the Vietnamese Mekong Delta's floodplains." Science of the Total Environment. 954. https://doi.org/10.1016/j.scitotenv.2024.176501Monitoring of Greenhouse Gas Emission Drivers in Atlantic Canadian Potato Production: A Robust Explainable Intelligent Glass-Box
Jamei, Mehdi, Hassan, Muhammad, Farooque, Aitazaz A., Ali, Mumtaz, Karbasi, Masoud, Randhawa, Gurjit, Yaseen, Zaher Mundher and Dwyer, Ross. 2024. "Monitoring of Greenhouse Gas Emission Drivers in Atlantic Canadian Potato Production: A Robust Explainable Intelligent Glass-Box." Results in Engineering. https://doi.org/10.1016/j.rineng.2024.103297Real-time prediction of the week-ahead flood index using hybrid deep learning algorithms with synoptic climate mode indices
Ahmed, A.A. Masrur, Akther, Shahida, Nguyen-Huy, Thong, Raj, Nawin, Jui (Student), S Janifer and S.Z., Farzana. 2024. "Real-time prediction of the week-ahead flood index using hybrid deep learning algorithms with synoptic climate mode indices." Journal of Hydro-Environment Research. 57, pp. 12-26. https://doi.org/10.1016/j.jher.2024.09.001Pyrolytic Pathway of Wheat Straw Pellet by the Thermogravimetric Analyzer
Nath, Bidhan, Bowtell, Les, Chen, Guangnan, Graham, Elizabeth and Nguyen-Huy, Thong. 2024. "Pyrolytic Pathway of Wheat Straw Pellet by the Thermogravimetric Analyzer." Energies. 17 (15). https://doi.org/10.3390/en17153693Half-hourly electricity price prediction with a hybrid convolution neural network-random vector functional link deep learning approach
Ghimire, Sujan, Deo, Ravinesh C., Casillas-Perez, David, Sharma, Ekta, Salcedo-sanz, Sancho, Barua, Prabal and Acharya, U. Rajendra. 2024. "Half-hourly electricity price prediction with a hybrid convolution neural network-random vector functional link deep learning approach." Applied Energy. 374. https://doi.org/10.1016/j.apenergy.2024.123920Towards next-generation federated learning: A case study on privacy attacks in artificial intelligence systems
Sharma, Ekta, Deo, Ravinesh C, Davey, Christopher P., Carter, Brad D. and Salcedo-sanz, Sancho. 2024. "Towards next-generation federated learning: A case study on privacy attacks in artificial intelligence systems." 2024 IEEE Conference on Artificial Intelligence (CAI 2024). Singapore 25 - 27 Jun 2024 United States. IEEE (Institute of Electrical and Electronics Engineers). https://doi.org/10.1109/CAI59869.2024.00259Poster: Cloud Computing with AI-empowered Trends in Software-Defined Radios: Challenges and Opportunities
Sharma, Ekta, Deo, Ravinesh C., Davey, Christopher P., Carter, Brad D. and Salcedo-sanz, Sancho. 2024. "Poster: Cloud Computing with AI-empowered Trends in Software-Defined Radios: Challenges and Opportunities." 2024 IEEE 25th International Symposium on a World of Wireless, Mobile and Multimedia Networks (WoWMoM 2024). Perth, Australia 04 - 07 Jun 2024 United States. IEEE (Institute of Electrical and Electronics Engineers). https://doi.org/10.1109/WoWMoM60985.2024.00054Academic Numeracies Framework – A tool to facilitate the systematic embedding of numeracies in university
Salmeron, Raquel, Galligan, Linda, Howarth, Debi and Raj, Nawin. 2024. "Academic Numeracies Framework – A tool to facilitate the systematic embedding of numeracies in university." 15th International Congress on Mathematical Education (ICME-15). Sydney, Australia 07 - 14 Jul 2024 Australia.Prediction of Sea Level Using Double Data Decomposition and Hybrid Deep Learning Model for Northern Territory, Australia
Raj, Nawin, Murali, Jaishukh, Singh-Peterson, Lila and Downs, Nathan. 2024. "Prediction of Sea Level Using Double Data Decomposition and Hybrid Deep Learning Model for Northern Territory, Australia." Mathematics. 12 (15). https://doi.org/10.3390/math12152376Robust drought forecasting in Eastern Canada: Leveraging EMD-TVF and ensemble deep RVFL for SPEI index forecasting
Karbasi, Masoud, Ali, Mumtaz, Farooque, Aitazaz Ahsan, Jamei, Mehdi, Khosravi, Khabat, Cheema, Saad Javed and Yaseen, Zaher Mundher. 2024. "Robust drought forecasting in Eastern Canada: Leveraging EMD-TVF and ensemble deep RVFL for SPEI index forecasting." Expert Systems with Applications. 256. https://doi.org/10.1016/j.eswa.2024.124900Atmospheric Visibility and Cloud Ceiling Predictions With Hybrid IIS-LSTM Integrated Model: Case Studies for Fiji's Aviation Industry
Raj, Shiveel, Deo, Ravinesh C., Sharma, Ekta, Prasad, Ramendra, Dinh, Toan and Salcedo-sanz, Sancho. 2024. "Atmospheric Visibility and Cloud Ceiling Predictions With Hybrid IIS-LSTM Integrated Model: Case Studies for Fiji's Aviation Industry." IEEE Access. 12, pp. 72530-72543. https://doi.org/10.1109/ACCESS.2024.3401091Point-based and probabilistic electricity demand prediction with a Neural Facebook Prophet and Kernel Density Estimation model
Ghimire, Sujan, Deo, Ravinesh C., Pourmousavi, S. Ali, Casillas-Perez, David and Salcedo-sanz, Sancho. 2024. "Point-based and probabilistic electricity demand prediction with a Neural Facebook Prophet and Kernel Density Estimation model." Engineering Applications of Artificial Intelligence. 135. https://doi.org/10.1016/j.engappai.2024.108702End-to-end learning of adaptive coded modulation schemes for resilient wireless communications
Davey, Christopher P., Shakeel, Ismail, Deo, Ravinesh C., Sharma, Ekta, Salcedo-sanz, Sancho and Soar, Jeffrey. 2024. "End-to-end learning of adaptive coded modulation schemes for resilient wireless communications." Applied Soft Computing. 159. https://doi.org/10.1016/j.asoc.2024.111672Deep Learning Based Over-the-Air Training of Wireless Communication Systems without Feedback
Davey, Christopher P., Shakeel, Ismail, Deo, Ravinesh C. and Salcedo-sanz, Sancho. 2024. "Deep Learning Based Over-the-Air Training of Wireless Communication Systems without Feedback." Sensors. 24 (10). https://doi.org/10.3390/s24102993Pyrolysis of wheat straw pellets in a pilot-scale reactor: Effect of temperature and residence time
Nath, Bidhan, Chen, Guangnan, Bowtell, Les and Nguyen-Huy, Thong. 2024. "Pyrolysis of wheat straw pellets in a pilot-scale reactor: Effect of temperature and residence time." Energy Science and Engineering. 12 (8), pp. 3524-3539. https://doi.org/10.1002/ese3.1833Integrating rainfall index-based insurance with optimal crop management strategies can reduce financial risks for Australian dryland cotton farmers
Nguyen-Huy, Thong, Kath, Jarrod, Kouadio, Louis, King, Rachel, Mushtaq, Shahbaz and Barratt, Jonathan. 2024. "Integrating rainfall index-based insurance with optimal crop management strategies can reduce financial risks for Australian dryland cotton farmers." Sustainable Futures. 8. https://doi.org/10.1016/j.sftr.2024.100249Multi-step ahead forecasting of electrical conductivity in rivers by using a hybrid Convolutional Neural Network-Long Short-Term Memory (CNN-LSTM) model enhanced by Boruta-XGBoost feature selection algorithm
Karbasi, Masoud, Ali, Mumtaz, Bateni, Sayed M., Jun, Changhyun, Jamei, Mehdi, Farooque, AitazazAhsan and Yaseen, Zaher Mundher. 2024. "Multi-step ahead forecasting of electrical conductivity in rivers by using a hybrid Convolutional Neural Network-Long Short-Term Memory (CNN-LSTM) model enhanced by Boruta-XGBoost feature selection algorithm." Scientific Reports. 14. https://doi.org/https://doi.org/10.1038/s41598-024-65837-0A playground shade index for standardising ultraviolet protection assessments of open, mixed and protected outdoor recreational spaces
Downs, Nathan, Butler, Harry, Dexter, Benjamin, Raj, Nawin, Downs, Melanie, Turner, Joanna, Dekeyser, Stijn, Deo, Ravinesh, Vanos, Jennifer, Igoe, Damien and Parisi, Alfio V. 2024. "A playground shade index for standardising ultraviolet protection assessments of open, mixed and protected outdoor recreational spaces." 6th International conference UV and Skin Cancer Prevention. Brisbane, Australia 11 - 15 Sep 2024 Australia.The mitigating effect of street trees, urban flora, and the suburban environment on seasonal peak UV indices: A case study from Brisbane, Australia
Downs, Nathan James, Amar, Abdurazaq, Dearnaley, John, Butler, Harry, Dekeyser, Stijn, Igoe, Damien, Parisi, Alfio V., Raj, Nawin, Deo, Ravinesh and Turner, Joanna. 2024. "The mitigating effect of street trees, urban flora, and the suburban environment on seasonal peak UV indices: A case study from Brisbane, Australia." Photochemistry and Photobiology. https://doi.org/10.1111/php.13988Multi-Step-Ahead Wind Speed Forecast System: Hybrid Multivariate Decomposition and Feature Selection-Based Gated Additive Tree Ensemble Model
Joseph, Lionel P., Deo, Ravinesh C., Casillas-Perez, David, Prasad, Ramendra, Raj, Nawin and Salcedo-sanz, Sancho. 2024. "Multi-Step-Ahead Wind Speed Forecast System: Hybrid Multivariate Decomposition and Feature Selection-Based Gated Additive Tree Ensemble Model." IEEE Access. 12, pp. 58750-58777. https://doi.org/10.1109/ACCESS.2024.3392899Deep Learning-Assisted Sensitive 3C-SiC Sensor for Long-Term Monitoring of Physical Respiration
Tran, Thi Lap, Nguyen, Duy Van, Nguyen, Hung, Nguyen, Thi Phuoc Van, Song, Pingan, Deo, Ravinesh C, Moloney, Clint, Dao, Viet Dung, Nguyen, Nam-Trung and Dinh, Toan. 2024. "Deep Learning-Assisted Sensitive 3C-SiC Sensor for Long-Term Monitoring of Physical Respiration." Advanced Sensor Research. 3 (8). https://doi.org/10.1002/adsr.202300159Copula-Probabilistic Flood Risk Analysis with an Hourly Flood Monitoring Index
Chand, Ravinesh, Nguyen-Huy, Thong, Deo, Ravinesh C., Ghimire, Sujan, Ali, Mumtaz and Ghahramani, Afshin. 2024. "Copula-Probabilistic Flood Risk Analysis with an Hourly Flood Monitoring Index." Water. 16 (11). https://doi.org/10.3390/w16111560From conceptualization to practice: enhancing economic resilience at the local government level in Australia
Singh-Peterson, Lila, Kassulke, Anna and Raj, Nawin. 2024. "From conceptualization to practice: enhancing economic resilience at the local government level in Australia." Journal of Environmental Policy and Planning. 26 (3), pp. 295-310. https://doi.org/https://doi.org/10.1080/1523908X.2024.2334709Short-term wind speed forecasting using an optimized three-phase convolutional neural network fused with bidirectional long short-term memory network model
Joseph, Lionel P., Deo, Ravinesh C., Casillas-Perez, David, Prasad, Ramendra, Raj, Nawin and Salcedo-sanz, Sancho. 2024. "Short-term wind speed forecasting using an optimized three-phase convolutional neural network fused with bidirectional long short-term memory network model." Applied Energy. 359. https://doi.org/10.1016/j.apenergy.2024.122624Probabilistic-based electricity demand forecasting with hybrid convolutional neural network-extreme learning machine model
Ghimire, Sujan, Deo, Ravinesh C., Casillas-Perez, David, Salcedo-sanz, Sancho, Pourmousavi, S. Ali and Acharya, U. Rajendra. 2024. "Probabilistic-based electricity demand forecasting with hybrid convolutional neural network-extreme learning machine model." Engineering Applications of Artificial Intelligence. 132. https://doi.org/10.1016/j.engappai.2024.107918Electricity demand error corrections with attention bi-directional neural networks
Ghimire, Sujan, Deo, Ravinesh C., Casillas-Perez, David and Salcedo-sanz, Sancho. 2024. "Electricity demand error corrections with attention bi-directional neural networks." Energy. 291. https://doi.org/10.1016/j.energy.2023.129938Micromachined Mechanical Resonant Sensors: From Materials, Structural Designs to Applications
Dinh, Toan, Rais-Zadeh, Mina, Nguyen, Thanh, Phan, Hoang-Phuong, Song, Pingan, Deo, Ravinesh, Dao, Dzung, Nguyen, Nam-Trung and Bell, John. 2024. "Micromachined Mechanical Resonant Sensors: From Materials, Structural Designs to Applications." Advanced Materials Technologies. 9 (2). https://doi.org/10.1002/admt.202300913Assessment and prediction of significant wave height using hybrid CNN-BiLSTM deep learning model for sustainable wave energy in Australia
Raj, Nawin and Prakash, Reema. 2024. "Assessment and prediction of significant wave height using hybrid CNN-BiLSTM deep learning model for sustainable wave energy in Australia." Sustainable Horizons . 11. https://doi.org/10.1016/j.horiz.2024.100098Assessment and Prediction of Sea Level and Coastal Wetland Changes in Small Islands Using Remote Sensing and Artificial Intelligence
Raj, Nawin and Pasfield-Neofitou, Sarah. 2024. "Assessment and Prediction of Sea Level and Coastal Wetland Changes in Small Islands Using Remote Sensing and Artificial Intelligence." Remote Sensing. 16 (3), p. 551. https://doi.org/https://doi.org/10.3390/rs16030551Machine learning for expediting next-generation of fire-retardant polymer composites
Jafari, Pooya, Zhang, Ruoran, Huo, Siqi, Wang, Qingsheng, Yong, Jianming, Hong, Min, Deo, Ravinesh, Wang, Hao and Song, Pingan. 2024. "Machine learning for expediting next-generation of fire-retardant polymer composites." Composites Communications. 45. https://doi.org/10.1016/j.coco.2023.101806Quantitative improvement of streamflow forecasting accuracy in the Atlantic zones of Canada based on hydro-meteorological signals: A multi-level advanced intelligent expert framework
Jamei, Mozhdeh, Jamei, Mehdi, Ali, Mozhdeh, Karbasi, Masoud, Farooque, Aitazaz A., Malik, Anurag, Cheema, Saad Javed, Esau, Travis J. and Yaseen, Zaher Mundher. 2024. "Quantitative improvement of streamflow forecasting accuracy in the Atlantic zones of Canada based on hydro-meteorological signals: A multi-level advanced intelligent expert framework." Ecological Informatics. 80. https://doi.org/10.1016/j.ecoinf.2023.102455Short-term drought Index forecasting for hot and semi-humid climate Regions: A novel empirical Fourier decomposition-based ensemble Deep-Random vector functional link strategy
Jamei, Mehdi, Ali, Mumtaz, Bateni, Sayed M., Jun, Changhyun, Karbasi, Masoud, Malik, Anurag, Jamei, Mozhdeh and Yaseen, Zaher Mundher. 2024. "Short-term drought Index forecasting for hot and semi-humid climate Regions: A novel empirical Fourier decomposition-based ensemble Deep-Random vector functional link strategy ." Computers and Electronics in Agriculture. 217. https://doi.org/10.1016/j.compag.2023.108609Very short-term solar ultraviolet-A radiation forecasting system with cloud cover images and a Bayesian optimized interpretable artificial intelligence model
Prasad, Salvin Sanjesh, Deo, Ravinesh Chand, Downs, Nathan James, Casillas-Perez, David, Salcedo-sanz, Sancho and Parisi, Alfio Venerando. 2024. "Very short-term solar ultraviolet-A radiation forecasting system with cloud cover images and a Bayesian optimized interpretable artificial intelligence model ." Expert Systems with Applications. 236. https://doi.org/10.1016/j.eswa.2023.121273Boruta extra tree-bidirectional long short-term memory model development for Pan evaporation forecasting: Investigation of arid climate condition
Karbasi, Masoud, Ali, Mumtaz, Bateni, Sayed M., Jun, Changhyun, Jamei, Mehdi and Yaseen, Zaher Mundher. 2024. "Boruta extra tree-bidirectional long short-term memory model development for Pan evaporation forecasting: Investigation of arid climate condition." Alexandria Engineering Journal. 86, pp. 425-442. https://doi.org/10.1016/j.aej.2023.11.061Two-step deep learning framework with error compensation technique for short-term, half-hourly electricity price forecasting
Ghimire, Sujan, Deo, Ravinesh C., Casillas-Perez, David and Salcedo-sanz, Sancho. 2024. "Two-step deep learning framework with error compensation technique for short-term, half-hourly electricity price forecasting." Applied Energy. 353 (Part A). https://doi.org/10.1016/j.apenergy.2023.122059Hybrid Deep Learning Model for Wave Height Prediction in Australia's Wave Energy Region
Ahmed, Abul Abrar Masrur, Jui, S Janifer Jabin, AL-Musaylh, Mohanad S., Raj, Nawin, Saha, Reepa, Deo, Ravinesh C and Saha, Sanjoy Kumar. 2024. "Hybrid Deep Learning Model for Wave Height Prediction in Australia's Wave Energy Region." Applied Soft Computing. 150. https://doi.org/10.1016/j.asoc.2023.111003Gully erosion mapping susceptibility in a Mediterranean environment: A hybrid decision-making model
Hitouri, Sliman, Meriame, Mohajane, Ajim, Ali Sk, Pacheco, Quevedo Renata, Nguyen-Huy, Thong, Pham, Quoc Bao, ElKhrachy, Ismail and Varasano, Antonietta. 2024. "Gully erosion mapping susceptibility in a Mediterranean environment: A hybrid decision-making model." International Soil and Water Conservation Research. 12 (2), pp. 279-297. https://doi.org/10.1016/j.iswcr.2023.09.008Modelling future spatial distribution of peanut crops in Australia under climate change scenarios
Haerani, Haerani, Apan, Armando, Nguyen-Huy, Thong and Basnet, Badri. 2023. "Modelling future spatial distribution of peanut crops in Australia under climate change scenarios." Geo-spatial Information Science. https://doi.org/10.1080/10095020.2022.2155255Accurate Image Multi-Class Classification Neural Network Model with Quantum Entanglement Approach
Riaz, Farina, Abdulla, Shahab, Suzuki, Hajime, Ganguly, Srinjoy, Deo, Ravinesh C. and Hopkins, Susan. 2023. "Accurate Image Multi-Class Classification Neural Network Model with Quantum Entanglement Approach." Sensors. 23 (5), pp. 1-11. https://doi.org/10.3390/s23052753Channel-Agnostic Training of Transmitter and Receiver for Wireless Communications
Davey, Christopher P., Shakeel, Ismail, Deo, Ravinesh C. and Salcedo-sanz, Sancho. 2023. "Channel-Agnostic Training of Transmitter and Receiver for Wireless Communications ." Sensors. 23 (24). https://doi.org/10.3390/s23249848Deep Image Analysis for Microalgae Identification
Soar, Jeffrey, Lih, Oh Shu, Wen, Loh Hui, Ward, Aleth, Sharma, Ekta, Deo, Ravinesh C., Barua, Prabal Datta, Tan, Ru-San, Rinen, Eliezer and Acharya, Rajendra. 2023. "Deep Image Analysis for Microalgae Identification." Lecture notes in computer science. Switzerland . Springer. pp. 280-292Fostering Enduring Peer Learning Groups for 1st Year Students
Brown, Jason, Kennedy, Joel, Raj, Nawin and Quinton, Matthew. 2023. "Fostering Enduring Peer Learning Groups for 1st Year Students." 34th Annual Conference of the Australasian Association for Engineering Education (AAEE 2023). Gold Coast, Australia 03 - 06 Dec 2023 Australia. Australasian Association for Engineering Education.Short-term wave power forecasting with hybrid multivariate variational mode decomposition model integrated with cascaded feedforward neural networks
Ali, Mumtaz, Prasad, Ramendra, Jamei, Mehdi, Malik, Anurag, Xiang, Yong, Abdulla, Shahab, Deo, Ravinesh C., Farooque, Aitazaz A. and Labban, Abdulhaleem H.. 2023. "Short-term wave power forecasting with hybrid multivariate variational mode decomposition model integrated with cascaded feedforward neural networks." Renewable Energy. 221. https://doi.org/10.1016/j.renene.2023.119773The Academic Numeracy Framework: A tool to embed numeracy in tertiary courses, programs and study-support initiatives
Salmeron, Raquel, Galligan, Linda, Howarth, Debi and Raj, Nawin. 2023. "The Academic Numeracy Framework: A tool to embed numeracy in tertiary courses, programs and study-support initiatives." 8th Students Transitions Achievement Retention & Success Conference (STARS 2023). Brisbane, Australia 03 - 05 Jul 2023 Australia.Climate change and human health in Vietnam: a systematic review and additional analyses on current impacts, future risk, and adaptation
Tran, Nu Quy Linh, Le, Huynh Thi Cam Hong, Pham, Cong Tuan, Nguyen, Xuan Huong, Tran, Ngoc Dang, Tran, Tuyet-Hanh Thi, Nghiem, Son, Luong, Thi Mai Ly, Bui, Vinh, Nguyen-Huy, Thong, Doan, Van Quang, Dang, Kim Anh, Do, Thi Hoai Thuong, Ngo, Hieu Kim Thi, Nguyen, Truong Vien, Nguyen, Ngoc Huy, Do, Manh Cuong, Ton, Tuan Nghia, Dang, Thi Anh Thu, ..., Phung, Dung. 2023. "Climate change and human health in Vietnam: a systematic review and additional analyses on current impacts, future risk, and adaptation." The Lancet Regional Health - Western Pacific. 40. https://doi.org/10.1016/j.lanwpc.2023.100943Enhancing climate resilience by combining practice and insurance strategies: A case study for cotton crop
Nguyen-Huy, Thong and Battersby, Kerry. 2023. "Enhancing climate resilience by combining practice and insurance strategies: A case study for cotton crop." Queensland Disaster Management Research Forum 2023. Brisbane, Australia 07 - 07 Nov 2023An advanced deep learning predictive model for air quality index forecasting with remote satellite-derived hydro-climatological variables
Ahmed, Abul Abrar Masrur, Jui, S. Janifer Jabin, Sharma, Ekta, Ahmed, Mohammad Hafez, Raj, Nawin and Bose, Aditi. 2023. "An advanced deep learning predictive model for air quality index forecasting with remote satellite-derived hydro-climatological variables." Science of the Total Environment. 906. https://doi.org/10.1016/j.scitotenv.2023.167234Cover cropping impacts on soil water and carbon in dryland cropping system
Zhang, Hanlu, Ghahramani, Afshin, Ali, Aram and Erbacher, Andrew. 2023. "Cover cropping impacts on soil water and carbon in dryland cropping system." PLoS One. 18. https://doi.org/10.1371/journal.pone.0286748Statistical and spatial analysis for soil heavy metals over the Murray-Darling river basin in Australia
Tao, Hai, Al-Hilali, Aqeel Ali, Ahmed, Ali M., Mussa, Zainab Haider, Falah, Mayadah W., Abed, Salwan Ali, Deo, Ravinesh, Jawad, Ali H., Maulud, Khairul Nizam Abdul, Latif, Mohd Talib and Yaseen, Zaher Mundher. 2023. "Statistical and spatial analysis for soil heavy metals over the Murray-Darling river basin in Australia." Chemosphere. 317. https://doi.org/10.1016/j.chemosphere.2023.137914Enhanced joint hybrid deep neural network explainable artificial intelligence model for 1-hr ahead solar ultraviolet index prediction
Prasad, Salvin S., Deo, Ravinesh C., Salcedo-sanz, Sancho, Downs, Nathan J., Casillas-Perez, David and Parisi, Alfio V.. 2023. "Enhanced joint hybrid deep neural network explainable artificial intelligence model for 1-hr ahead solar ultraviolet index prediction." Computer Methods and Programs in Biomedicine. 241. https://doi.org/10.1016/j.cmpb.2023.107737Ampelomyces mycoparasites of powdery mildews–a review
Prahl, Rosa E., Khan, Shahjahan and Deo, Ravinesh C.. 2023. "Ampelomyces mycoparasites of powdery mildews–a review." Canadian Journal of Plant Pathology. 45 (4), pp. 391-404. https://doi.org/10.1080/07060661.2023.2206378A fuzzy-based cascade ensemble model for improving extreme wind speeds prediction
Pelaez-Rodriguez, C., Perez-Aracil, J., Prieto-Godino, L., Ghimire, S., Deo, R. and Salcedo-sanz, S.. 2023. "A fuzzy-based cascade ensemble model for improving extreme wind speeds prediction." Journal of Wind Engineering and Industrial Aerodynamics. 240. https://doi.org/10.1016/j.jweia.2023.105507Explainable AI approach with original vegetation data classifies spatio-temporal nitrogen in flows from ungauged catchments to the Great Barrier Reef
O’Sullivan, Cherie M., Deo, Ravinesh C. and Ghahramani, Afshin. 2023. "Explainable AI approach with original vegetation data classifies spatio-temporal nitrogen in flows from ungauged catchments to the Great Barrier Reef." Scientific Reports. 13 (1). https://doi.org/10.1038/s41598-023-45259-0Ranking Sub-Watersheds for Flood Hazard Mapping: A Multi-Criteria Decision-Making Approach
Nguyen, Nguyet-Minh, Bahramloo, Reza, Sadeghian, Jalal, Sepehri, Mehdi, Nazaripouya, Hadi, Dinh, Vuong Nguyen, Ghahramani, Afshin, Talebi, Ali, ElKhrachy, Ismail, Pande, Chaitanya B. and Meshram, Sarita Gajbhiye. 2023. "Ranking Sub-Watersheds for Flood Hazard Mapping: A Multi-Criteria Decision-Making Approach." Water. 15 (11). https://doi.org/10.3390/w15112128A Novel Attention-Based Model for Semantic Segmentation of Prostate Glands Using Histopathological Images
Inamdar, Mahesh Anil, Raghavendra, U., Gudigar, Anjan, Bhandary, Sarvesh, Salvi, Massimo, Deo, Ravinesh C., Barua, Prabal Datta, Ciaccio, Edward J., Molinari, Filippo and Acharya, RU. Rajendra. 2023. "A Novel Attention-Based Model for Semantic Segmentation of Prostate Glands Using Histopathological Images." IEEE Access. 11, pp. 108982-108994. https://doi.org/10.1109/ACCESS.2023.3321273Efficient daily electricity demand prediction with hybrid deep-learning multi-algorithm approach
Ghimire, Sujan, Deo, Ravinesh C., Casillas-Perez, David and Salcedo-sanz, Sancho. 2023. "Efficient daily electricity demand prediction with hybrid deep-learning multi-algorithm approach." Energy Conversion and Management. 297. https://doi.org/10.1016/j.enconman.2023.117707A drought monitor for Australia
Guillory, Laura, Pudmenzky, Christa, Nguyen-Huy, Thong, Cobon, David and Stone, Roger. 2023. "A drought monitor for Australia." Environmental Modelling and Software. 170. https://doi.org/10.1016/j.envsoft.2023.105852Automated detection of airflow obstructive diseases: A systematic review of the last decade (2013-2022)
Xu, Shuting, Deo, Ravinesh C, Soar, Jeffrey, Barua, Prabal Datta, Faust, Oliver, Homaira, Nusrat, Jaffe, Adam, Kabir, Arm Luthful and Acharya, U. Rajendra. 2023. "Automated detection of airflow obstructive diseases: A systematic review of the last decade (2013-2022)." Computer Methods and Programs in Biomedicine. 241. https://doi.org/10.1016/j.cmpb.2023.107746Brain tumor detection and screening using artificial intelligence techniques: Current trends and future perspectives
Raghavendra, U., Gudigar, Anjan, Paul, Aritra, Goutham, T.S., Inamdar, Mahesh Anil, Hegde, Ajay, Dev, Aruna, Ooi, Chui Ping, Deo, Ravinesh C., Barua, Prabal Datta, Molinari, Filippo, Ciaccio, Edward J. and Acharya, U. Rajendra. 2023. "Brain tumor detection and screening using artificial intelligence techniques: Current trends and future perspectives." Computers in Biology and Medicine. 163. https://doi.org/10.1016/j.compbiomed.2023.107063Application of Entropy for Automated Detection of Neurological Disorders With Electroencephalogram Signals: A Review of the Last Decade (2012-2022)
Jui, S. Janifer Jabin, Deo, Ravinesh C. Deo, Barua, Prabal Datta, Devi, Aruna, Soar, Jeffrey and Acharya, U. Rajendra. 2023. "Application of Entropy for Automated Detection of Neurological Disorders With Electroencephalogram Signals: A Review of the Last Decade (2012-2022)." IEEE Access. 11, pp. 71905-71924. https://doi.org/10.1109/ACCESS.2023.3294473Integrated Multi-Head Self-Attention Transformer model for electricity demand prediction incorporating local climate variables
Ghimire, Sujan, Nguyen-Huy, Thong, AL-Musaylh, Mohanad S., Deo, Ravinesh C., Casillas-Perez, David and Salcedo-sanz, Sancho. 2023. "Integrated Multi-Head Self-Attention Transformer model for electricity demand prediction incorporating local climate variables." Energy and AI. 14. https://doi.org/10.1016/j.egyai.2023.100302A systematic review of emerging environmental markets: Potential pathways to creating shared value for communities
Mittahalli Byrareddy, Vivekananda M, Islam, Md Aminul, Nguyen-Huy, Thong and Slaughter, Geoff. 2023. "A systematic review of emerging environmental markets: Potential pathways to creating shared value for communities ." Heliyon. 9 (9). https://doi.org/10.1016/j.heliyon.2023.e19754Integrating management decisions and insurance to drive climate adaptation in the agricultural industry
Nguyen-Huy, Thong. 2023. "Integrating management decisions and insurance to drive climate adaptation in the agricultural industry ." Climate Adaptation 2023. Adelaide, Australia 25 - 27 Jul 2023 Australia.Flash flood-risk areas zoning using integration of decision-making trial and evaluation laboratory, GIS-based analytic network process and satellite-derived information
Taherizadeh, Mehrnoosh, Niknam, Arman, Nguyen-Huy, Thong, Mezősi, Gábor and Sarli, Reza. 2023. "Flash flood-risk areas zoning using integration of decision-making trial and evaluation laboratory, GIS-based analytic network process and satellite-derived information." Natural Hazards. 118 (3), p. 2309–2335. https://doi.org/10.1007/s11069-023-06089-5Revealing the effect of an industrial flash flood on vegetation area: a case study of Khusheh Mehr in Maragheh-Bonab Plain, Iran
Taherizadeh, Mehrnoosh, Khushemehr, Javid Hojabri, Niknam, Arman, Nguyen-Huy, Thong and Mezősi, Gábor. 2023. "Revealing the effect of an industrial flash flood on vegetation area: a case study of Khusheh Mehr in Maragheh-Bonab Plain, Iran." Remote Sensing Applications: Society and Environment. 32. https://doi.org/10.1016/j.rsase.2023.101016Prediction of Mean Sea Level with GNSS-VLM Correction Using a Hybrid Deep Learning Model in Australia
Raj, Nawin and Brown, Jason. 2023. "Prediction of Mean Sea Level with GNSS-VLM Correction Using a Hybrid Deep Learning Model in Australia." Remote Sensing. 15 (11).The Great 2011 Thailand flood disaster revisited: Could it have been mitigated by different dam operations based on better weather forecasts?
Loc, Ho Huu, Emadzadeh, Adel, Park, Edward, Nontikansak, Piyanuch and Deo, Ravinesh C.. 2023. "The Great 2011 Thailand flood disaster revisited: Could it have been mitigated by different dam operations based on better weather forecasts? " Environmental Research. 216 (Part 2). https://doi.org/10.1016/j.envres.2022.114493Comparison of machine learning methods emulating process driven crop models
Johnston, David B., Pembleton, Keith G., Huth, Neil I. and Deo, Ravinesh C.. 2023. "Comparison of machine learning methods emulating process driven crop models ." Environmental Modelling and Software. 162. https://doi.org/10.1016/j.envsoft.2023.105634A novel approach based on integration of convolutional neural networks and echo state network for daily electricity demand prediction
Ghimire, Sujan, Nguyen-Huy, Thong, AL-Musaylh, Mohanad S., Deo, Ravinesh, Casillas-Perez, David and Salcedo-sanz, Sancho. 2023. "A novel approach based on integration of convolutional neural networks and echo state network for daily electricity demand prediction." Energy. 275, p. 127430. https://doi.org/10.1016/j.energy.2023.127430Climatology and composite evolution of flash drought over Australia and its vegetation impacts
Nguyen, Hanh, Wheeler, Mattew C., Otkin, Jason A., Nguyen-Huy, Thong and Cowan, Timothy. 2023. "Climatology and composite evolution of flash drought over Australia and its vegetation impacts." Journal of Hydrometeorology. 24 (6), p. 1087–1101. https://doi.org/10.1175/JHM-D-22-0033.1Digital Farming: An Overview of Its Fundamental Components and Applications
Nguyen-Huy, Thong. 2023. "Digital Farming: An Overview of Its Fundamental Components and Applications." The 4th International Conference of Food Security and Sustainable Agriculture in the Tropics. South Sulawesi, Indonesia 15 - 16 Feb 2023 Indonesia.Near real-time wind speed forecast model with bidirectional LSTM networks
Joseph, Lionel P., Deo, Ravinesh C., Prasad, Ramendra, Salcedo-Sanz, Sancho, Raj, Nawin and Soar, Jeffrey. 2023. "Near real-time wind speed forecast model with bidirectional LSTM networks." Renewable Energy. 204, pp. 39-58. https://doi.org/10.1016/j.renene.2022.12.123Downscaling Surface Albedo to Higher Spatial Resolutions With an Image Super-Resolution Approach and PROBA-V Satellite Images
Deo, Ravinesh C., Karalasingham, Sagthitharan, Casillas-Perez, David, Raj, Narwin and Salcedo-sanz, Sancho. 2023. "Downscaling Surface Albedo to Higher Spatial Resolutions With an Image Super-Resolution Approach and PROBA-V Satellite Images." IEEE Access. 11, pp. 5558-5577. https://doi.org/10.1109/ACCESS.2023.3236253Developing a novel hybrid method based on dispersion entropy and adaptive boosting algorithm for human activity recognition
Diykh, Mohammed, Abdulla, Shahab, Deo, Ravinesh C, Siuly, Siuly and Ali, Mumtaz. 2023. "Developing a novel hybrid method based on dispersion entropy and adaptive boosting algorithm for human activity recognition." Computer Methods and Programs in Biomedicine. 229, pp. 1-11. https://doi.org/https://doi.org/10.1016/j.cmpb.2022.107305Cloud cover bias correction in numerical weather models for solar energy monitoring and forecasting systems with kernel ridge regression
Deo, Ravinesh C., Ahmed, A.A. Masrur, Casillas-Perez, David, Pourmousavi, S. Ali, Segal, Gary, Yu, Yanshan and Salcedo-sanz, Sancho. 2023. "Cloud cover bias correction in numerical weather models for solar energy monitoring and forecasting systems with kernel ridge regression." Renewable Energy. 203, pp. 113-130. https://doi.org/10.1016/j.renene.2022.12.048CNN Based Image Classification of Malicious UAVs
Brown, Jason, Gharineiat, Zahra and Raj, Nawin. 2023. "CNN Based Image Classification of Malicious UAVs." Applied Sciences. 13 (1), pp. 1-13. https://doi.org/10.3390/app13010240Deep Multi-Stage Reference Evapotranspiration Forecasting Model: Multivariate Empirical Mode Decomposition Integrated With the Boruta-Random Forest Algorithm
Jayasinghe, W. J. M. Lakmini Prarthana, Deo, Ravinesh C., Ghahramani, Afshin, Ghimire, Sujan and Raj, Nawin. 2021. "Deep Multi-Stage Reference Evapotranspiration Forecasting Model: Multivariate Empirical Mode Decomposition Integrated With the Boruta-Random Forest Algorithm." IEEE Access. 9, pp. 166695-166708. https://doi.org/10.1109/ACCESS.2021.3135362Designing Deep-based Learning Flood Forecast Model with ConvLSTM Hybrid Algorithm
Moishin, Mohammed, Deo, Ravinesh C., Prasad, Ramendra, Raj, Nawin and Abdulla, Shahab. 2021. "Designing Deep-based Learning Flood Forecast Model with ConvLSTM Hybrid Algorithm." IEEE Access. 9, pp. 50982-50993. https://doi.org/10.1109/ACCESS.2021.3065939A hierarchical classification/regression algorithm for improving extreme wind speed events prediction
Pelaez-Rodriguez, C., Perez-Aracil, J., Fister, D, Prieto-Godino, L., Deo, R.C. and Salcedo-sanz, S.. 2022. "A hierarchical classification/regression algorithm for improving extreme wind speed events prediction." Renewable Energy. 201 (Part 2), pp. 157-178. https://doi.org/10.1016/j.renene.2022.11.042Pattern recognition describing spatio-temporal drivers of catchment classification for water quality
O'Sullivan, Cherie M., Ghahramani, Afshin, Deo, Ravinesh C. and Pembleton, Keith G.. 2023. "Pattern recognition describing spatio-temporal drivers of catchment classification for water quality." Science of the Total Environment. 861, pp. 1-42. https://doi.org/10.1016/j.scitotenv.2022.160240The Playground Shade Index: A New Design Metric for Measuring Shade and Seasonal Ultraviolet Protection Characteristics of Parks and Playgrounds
Downs, Nathan, Raj, Nawin, Vanos, Jennifer, Parisi, Alfio, Butler, Harry, Deo, Ravinesh, Igoe, Damien, Dexter, Benjamin, Beckman-Downs, Melanie, Turner, Joanna and Dekeyser, Stijn. 2023. "The Playground Shade Index: A New Design Metric for Measuring Shade and Seasonal Ultraviolet Protection Characteristics of Parks and Playgrounds." Photochemistry and Photobiology. 99 (4), pp. 1193-1207. https://doi.org/10.1111/php.13745Using Sequence-to-Sequence Models for Carrier Frequency Offset Estimation of Short Messages and Chaotic Maps
Davey, Christopher P., Shakeel, Ismail, Deo, Ravinesh C., Salcedo-sanz, Sancho and Soar, Jeffrey. 2022. "Using Sequence-to-Sequence Models for Carrier Frequency Offset Estimation of Short Messages and Chaotic Maps." IEEE Access. 10, pp. 119814 - 119825. https://doi.org/10.1109/ACCESS.2022.3221762Modelling and Real-time Optimisation of Air Quality Predictions for Australia through Artificial Intelligence Algorithm
Sharma, Ekta, Deo, Ravinesh C., Prasad, Ramendra and Parisi, Alfio V.. 2019. "Modelling and Real-time Optimisation of Air Quality Predictions for Australia through Artificial Intelligence Algorithm." AMSI Optimise 2019. Perth, Australia 17 - 21 Jun 2019 Perth, Australia.Hybrid Convolutional Neural Network-Multilayer Perceptron Model for Solar Radiation Prediction
Ghimire, Sujan, Nguyen-Huy, Thong, Prasad, Ramendra, Deo, Ravinesh C., Casillas-Perez, David, Salcedo-sanz, Sancho and Bhandari, Binayak. 2022. "Hybrid Convolutional Neural Network-Multilayer Perceptron Model for Solar Radiation Prediction." Cognitive Computation. 15 (2), pp. 645-671. https://doi.org/10.1007/s12559-022-10070-yVapour pressure deficit determines critical thresholds for global coffee production under climate change
Kath, Jarrod, Craparo, Alessandro, Fong, Youyi, Byrareddy, Vivekananda, Davis, Aaron P., King, Rachel, Nguyen-Huy, Thong, van Asten, Piet J. A., Marcussen, Torben, Mushtaq, Shahbaz, Stone, Roger and Power, Scott. 2022. "Vapour pressure deficit determines critical thresholds for global coffee production under climate change." Nature Food. 3, pp. 871-880. https://doi.org/10.1038/s43016-022-00614-8Student Performance Predictions for Advanced Engineering Mathematics Course With New Multivariate Copula Models
Nguyen-Huy, Thong, Deo, Ravinesh C., Khan, Shahjahan, Devi, Aruna, Adeyinka, Adewuyi Ayodele, Apan, Armando A. and Yaseen, Zaher Mundher. 2022. "Student Performance Predictions for Advanced Engineering Mathematics Course With New Multivariate Copula Models." IEEE Access. 10, pp. 45112 -45136. https://doi.org/10.1109/ACCESS.2022.3168322Rapid assessment of mine rehabilitation areas with airborne LiDAR and deep learning: bauxite strip mining in Queensland, Australia
Murray, Xavier, Apan, Armando, Deo, Ravinesh and Maraseni, Tek. 2022. "Rapid assessment of mine rehabilitation areas with airborne LiDAR and deep learning: bauxite strip mining in Queensland, Australia." Geocarto International. 37 (26), pp. 11223-11252. https://doi.org/10.1080/10106049.2022.2048902Multi-strategy Slime Mould Algorithm for hydropower multi-reservoir systems optimization
Ahmadianfar, Iman, Noori, Ramzia Majeed, Togun, Hussein, Falah, Mayadah W., Homod, Raad Z., Fu, Minglei, Halder, Bijay, Deo, Ravinesh and Yaseen, Zaher Mundher. 2022. "Multi-strategy Slime Mould Algorithm for hydropower multi-reservoir systems optimization." Knowledge-Based Systems. 250, pp. 1-18. https://doi.org/10.1016/j.knosys.2022.109048Suspended sediment load modeling using advanced hybrid rotation forest based elastic network approach
Khosravi, Khabat, Golkarian, Ali, Melesse, Assefa M. and Deo, Ravinesh C.. 2022. "Suspended sediment load modeling using advanced hybrid rotation forest based elastic network approach." Journal of Hydrology. 610, pp. 1-14. https://doi.org/10.1016/j.jhydrol.2022.127963Delineating the Crop-Land Dynamic due to Extreme Environment Using Landsat Datasets: A Case Study
Halder, Bijay, Bandyopadhyay, Jatisankar, Afan, Haitham Abdulmohsin, Naser, Maryam H., Abed, Salwan Ali, Khedher, Khaled Mohamed, Falih, Khaldoon T., Deo, Ravinesh, Scholz, Miklas and Yaseen, Zaher Mundher. 2022. "Delineating the Crop-Land Dynamic due to Extreme Environment Using Landsat Datasets: A Case Study." Agronomy. 12 (6), pp. 1-23. https://doi.org/10.3390/agronomy12061268Coupled online sequential extreme learning machine model with ant colony optimization algorithm for wheat yield prediction
Ali, Mumtaz, Deo, Ravinesh C., Xiang, Yong, Prasad, Ramendra, Li, Jianxin, Farooque, Aitazaz and Yaseen, Zaher Mundher. 2022. "Coupled online sequential extreme learning machine model with ant colony optimization algorithm for wheat yield prediction." Scientific Reports. 12 (1), pp. 1-23. https://doi.org/10.1038/s41598-022-09482-5Forecasting solar photosynthetic photon flux density under cloud cover effects: novel predictive model using convolutional neural network integrated with long short-term memory network
Deo, Ravinesh C., Grant, Richard H., Webb, Ann, Ghimire, Sujan, Igoe, Damien P., Downs, Nathan J., Al-Musaylh, Mohanad S., Parisi, Alfio V. and Soar, Jeffrey. 2022. "Forecasting solar photosynthetic photon flux density under cloud cover effects: novel predictive model using convolutional neural network integrated with long short-term memory network." Stochastic Environmental Research and Risk Assessment. 36, p. 3183–3220. https://doi.org/10.1007/s00477-022-02188-0Improved Complete Ensemble Empirical Mode Decomposition with Adaptive Noise Deep Residual model for short-term multi-step solar radiation prediction
Ghimire, Sujan, Deo, Ravinesh C, Casillas-Perez, David and Salcedo-sanz, Sancho. 2022. "Improved Complete Ensemble Empirical Mode Decomposition with Adaptive Noise Deep Residual model for short-term multi-step solar radiation prediction." Renewable Energy. 190, pp. 408-424. https://doi.org/10.1016/j.renene.2022.03.120Efficient daily solar radiation prediction with deep learning 4-phase convolutional neural network, dual stage stacked regression and support vector machine CNN-REGST hybrid model
Ghimire, Sujan, Nguyen-Huy, Thong, Deo, Ravinesh C., Casillas-Perez, David and Salcedo-sanz, Sancho. 2022. "Efficient daily solar radiation prediction with deep learning 4-phase convolutional neural network, dual stage stacked regression and support vector machine CNN-REGST hybrid model." Sustainable Materials and Technologies. 32, pp. 1-24. https://doi.org/10.1016/j.susmat.2022.e00429Hybrid deep CNN-SVR algorithm for solar radiation prediction problems in Queensland, Australia
Ghimire, Sujan, Bhandari, Binayak, Casillas-Perez, David, Deo, Ravinesh C. and Salcedo-sanz, Sancho. 2022. "Hybrid deep CNN-SVR algorithm for solar radiation prediction problems in Queensland, Australia." Engineering Applications of Artificial Intelligence. 112, pp. 1-26. https://doi.org/10.1016/j.engappai.2022.104860Boosting solar radiation predictions with global climate models, observational predictors and hybrid deep-machine learning algorithms
Ghimire, Sujan, Deo, Ravinesh C., Casillas-Perez, David and Salcedo-sanz, Sancho. 2022. "Boosting solar radiation predictions with global climate models, observational predictors and hybrid deep-machine learning algorithms." Applied Energy. 316, pp. 1-25. https://doi.org/10.1016/j.apenergy.2022.119063Machine learning regression and classification methods for fog events prediction
Castillo-Boton, C., Casillas-Perez, D., Casanova-Mateo, C., Ghimire, S., Cerro-Prada, E., Gutierrez, P. A., Deo, R. C. and Salcedo-sanz, S.. 2022. "Machine learning regression and classification methods for fog events prediction." Atmospheric Research. 272, pp. 1-23. https://doi.org/10.1016/j.atmosres.2022.106157Quantum Artificial Intelligence Predictions Enhancement by Improving Signal Processing
Riaz, Farina, Abdulla, Shahab, Ni, Wei, Radfar, Mohsen, Deo, Ravinesh and Hopkins, Susan. 2022. "Quantum Artificial Intelligence Predictions Enhancement by Improving Signal Processing." Quantum Australia Conference 2022. Online 23 - 25 Feb 2022 Toowoomba, Australia. https://doi.org/10.13140/RG.2.2.34754.66245A satellite-based Standardized Antecedent Precipitation Index (SAPI) for mapping extreme rainfall risk in Myanmar
Nguyen-Huy, Thong, Kath, Jarrod, Nagler, Thomas, Khaung, Ye, Aung, Thee Su Su, Mushtaq, Shahbaz, Marcussen, Torben and Stone, Roger. 2022. "A satellite-based Standardized Antecedent Precipitation Index (SAPI) for mapping extreme rainfall risk in Myanmar." Remote Sensing Applications: Society and Environment. 26, pp. 1-19. https://doi.org/10.1016/j.rsase.2022.100733Deep learning CNN-LSTM-MLP hybrid fusion model for feature optimizations and daily solar radiation prediction
Ghimire, Sujan, Deo, Ravinesh C., Casillas-Perez, David, Salcedo-sanz, Sancho, Sharma, Ekta and Ali, Mumtaz. 2022. "Deep learning CNN-LSTM-MLP hybrid fusion model for feature optimizations and daily solar radiation prediction." Measurement. 202, pp. 1-22. https://doi.org/10.1016/j.measurement.2022.111759Basin management inspiration from impacts of alternating dry and wet conditions on water production and carbon uptake in Murray-Darling Basin
Lu, Zhixiang, Feng, Qi, Wei, Yongping, Zhao, Yan, Deo, Ravinesh C., Xie, Jiali, Zhou, Sha, Zhu, Meng and Xu, Min. 2022. "Basin management inspiration from impacts of alternating dry and wet conditions on water production and carbon uptake in Murray-Darling Basin." Science of the Total Environment. 851 (Part 2), pp. 1-8. https://doi.org/10.1016/j.scitotenv.2022.158359Introductory Engineering Mathematics Students’ Weighted Score Predictions Utilising a Novel Multivariate Adaptive Regression Spline Model
Ahmed, Abul Abrar Masrur, Deo, Ravinesh C., Ghimire, Sujan, Downs, Nathan J., Devi, Aruna, Barua, Prabal D. and Yaseen, Zaher M.. 2022. "Introductory Engineering Mathematics Students’ Weighted Score Predictions Utilising a Novel Multivariate Adaptive Regression Spline Model." Sustainability. 14 (17), pp. 1-27. https://doi.org/10.3390/su141711070Kernel Ridge Regression Hybrid Method for Wheat Yield Prediction with Satellite-Derived Predictors
Ahmed, A. A. Masrur, Sharma, Ekta, Jui, S. Janifer Jabin, Deo, Ravinesh C., Nguyen-Huy, Thong and Ali, Mumtaz. 2022. "Kernel Ridge Regression Hybrid Method for Wheat Yield Prediction with Satellite-Derived Predictors." Remote Sensing. 14 (5), pp. 1-24. https://doi.org/10.3390/rs14051136Cloud Affected Solar UV Predictions with Three-Phase Wavelet Hybrid Convolutional Long Short-Term Memory Network Multi-Step Forecast System
Prasad, Salvin S., Deo, Ravinesh C., Downs, Nathan, Igoe, Damien, Parisi, Alfio V. and Soar, Jeffrey. 2022. "Cloud Affected Solar UV Predictions with Three-Phase Wavelet Hybrid Convolutional Long Short-Term Memory Network Multi-Step Forecast System." IEEE Access. 10, pp. 24704-24720. https://doi.org/10.1109/ACCESS.2022.3153475Development and evaluation of hybrid deep learning long short-term memory network model for pan evaporation estimation trained with satellite and ground-based data
Jayasinghe, W. J. M. Lakmini Prarthana, Deo, Ravinesh C., Ghahramani, Afshin, Ghimire, Sujan and Raj, Nawin. 2022. "Development and evaluation of hybrid deep learning long short-term memory network model for pan evaporation estimation trained with satellite and ground-based data." Journal of Hydrology. 607, pp. 1-19. https://doi.org/10.1016/j.jhydrol.2022.127534Integrative artificial intelligence models for Australian coastal sediment lead prediction: An investigation of in-situ measurements and meteorological parameters effects
Bhagat, Suraj Kumar, Tiyasha, Tiyasha, Kumar, Adarsh, Malik, Tabarak, Jawad, Ali H., Khedher, Khaled Mohamed, Deo, Ravinesh C. and Yaseen, Zaher Mundher. 2022. "Integrative artificial intelligence models for Australian coastal sediment lead prediction: An investigation of in-situ measurements and meteorological parameters effects." Journal of Environmental Management. 309, pp. 1-16. https://doi.org/10.1016/j.jenvman.2022.114711Assessment and Prediction of Sea Level Trend in the South Pacific Region
Raj, Nawin, Gharineiat, Zahra, Ahmed, Abul Abrar Masrur and Stepanyants, Yury. 2022. "Assessment and Prediction of Sea Level Trend in the South Pacific Region." Remote Sensing. 14 (4), pp. 1-25. https://doi.org/10.3390/rs14040986An Eigenvalues-Based Covariance Matrix Bootstrap Model Integrated With Support Vector Machines for Multichannel EEG Signals Analysis
Al-Hadeethi, Hanan, Abdulla, Shahab, Diykh, Mohammed, Deo, Ravinesh C. and Green, Jonathan H.. 2022. "An Eigenvalues-Based Covariance Matrix Bootstrap Model Integrated With Support Vector Machines for Multichannel EEG Signals Analysis." Frontiers in Neuroinformatics. 15, pp. 1-15. https://doi.org/10.3389/fninf.2021.808339Stacked LSTM Sequence-to-Sequence Autoencoder with Feature Selection for Daily Solar Radiation Prediction: A Review and New Modeling Results
Ghimire, Sujan, Deo, Ravinesh C., Wang, Hua, Al-Musaylh, Mohanad S., Casillas-Perez, David and Salcedo-sanz, Sancho. 2022. "Stacked LSTM Sequence-to-Sequence Autoencoder with Feature Selection for Daily Solar Radiation Prediction: A Review and New Modeling Results." Energies. 15 (3), pp. 1-39. https://doi.org/10.3390/en15031061Texture analysis based graph approach for automatic detection of neonatal seizure from multi-channel EEG signals
Diykh, Mohammed, Miften, Firas Sabar, Abdulla, Shahab, Deo, Ravinesh C., Siuly, Siuly, Green, Jonathan H. and Oudah, Atheer Y.. 2022. "Texture analysis based graph approach for automatic detection of neonatal seizure from multi-channel EEG signals." Measurement. 190 (110731), pp. 1-13. https://doi.org/10.1016/j.measurement.2022.110731General equilibrium impact evaluation of food top-up induced by households’ renewable power self-supply in 141 regions
Nguyen, Duong Binh, Nong, Duy, Simshauser, Paul and Nguyen-Huy, Thong. 2022. "General equilibrium impact evaluation of food top-up induced by households’ renewable power self-supply in 141 regions." Applied Energy. 306 (Part B), pp. 1-13. https://doi.org/10.1016/j.apenergy.2021.118126Classification of catchments for nitrogen using Artificial Neural Network Pattern Recognition and spatial data
O'Sullivan, Cherie M., Ghahramani, Afshin, Deo, Ravinesh C., Pembleton, Keith, Khan, Urooj and Tuteja, Narendra. 2022. "Classification of catchments for nitrogen using Artificial Neural Network Pattern Recognition and spatial data." Science of the Total Environment. 809, pp. 1-15. https://doi.org/10.1016/j.scitotenv.2021.151139Copula-based statistical modelling of synoptic-scale climate indices for quantifying and managing agricultural risks in Australia
Nguyen-Huy, Thong. 2020. "Copula-based statistical modelling of synoptic-scale climate indices for quantifying and managing agricultural risks in Australia." Bulletin of the Australian Mathematical Society. 101 (1), pp. 166-169. https://doi.org/10.1017/S0004972719001217Drought Outlook Products Review
Cobon, David, Nguyen-Huy, Thong and Reardon-Smith, Kate. 2019. Drought Outlook Products Review. Toowoomba, Australia. University of Southern Queensland.Domino effect of climate change over two millennia in ancient China’s Hexi Corridor
Feng, Qi, Yan, Linshan, Deo, Ravinesh C., AghaKouchak, Amir, Adamowski, Jan F., Stone, Roger, Yin, Zhenliang, Liu, Wei, Si, Jianhua, Wen, Xiaohu, Zhu, Meng and Cao, Shixiong. 2019. "Domino effect of climate change over two millennia in ancient China’s Hexi Corridor." Nature Sustainability. 2, pp. 957-961. https://doi.org/10.1038/s41893-019-0397-9Bat algorithm for dam–reservoir operation
Ethteram, Mohammad, Mousavi, Sayed-Farhad, Karami, Hojat, Farzin, Saeed, Deo, Ravinesh, Othman, Faridah Binti, Chau, Kwok-Wing, Sarkamaryan, Saeed, Singh, Vijay P. and El-Shafie, Ahmed. 2018. "Bat algorithm for dam–reservoir operation." Environmental Earth Sciences. 77 (13), pp. 1-15. https://doi.org/10.1007/s12665-018-7662-5Characteristics of ecosystem water use efficiency in a desert riparian forest
Ma, Xiaohong, Feng, Qi, Su, Yonghong, Yu, Tengfei and Deo, Ravinesh C.. 2018. "Characteristics of ecosystem water use efficiency in a desert riparian forest." Environmental Earth Sciences. 77 (358). https://doi.org/10.1007/s12665-018-7518-zThe influence of climatic inputs on stream-flow pattern forecasting: case study of Upper Senegal River
Diop, Lamine, Bodian, Ansoumana, Djaman, Koffi, Yaseen, Zaher Mundher, Deo, Ravinesh C., El-Shafie, Ahmed and Brown, Larry C.. 2018. "The influence of climatic inputs on stream-flow pattern forecasting: case study of Upper Senegal River." Environmental Earth Sciences. 77 (5). https://doi.org/10.1007/s1266Uncertainty assessment of the multilayer perceptron (MLP) neural network model with implementation of the novel hybrid MLP-FFA method for prediction of biochemical oxygen demand and dissolved oxygen: a case study of Langat River
Raheli, Bahare, Aalami, Mohammad Taghi, El-Shafie, Ahmed, Ghorbani, Mohammad Ali and Deo, Ravinesh C.. 2017. "Uncertainty assessment of the multilayer perceptron (MLP) neural network model with implementation of the novel hybrid MLP-FFA method for prediction of biochemical oxygen demand and dissolved oxygen: a case study of Langat River." Environmental Earth Sciences. 76 (14). https://doi.org/10.1007/s12665-017-6842-zMethodology for producing the Drought Monitor
Pudmenzky, Christa, Guillory, Laura, Cobon, David H. and Nguyen-Huy, Thong. 2020. Methodology for producing the Drought Monitor. Toowoomba, Queensland. University of Southern Queensland.Northern Australia Climate Program: supporting adaptation in rangeland grazing systems through more targeted climate forecasts, improved drought information and an innovative extension program
Cobon, David, Jarvis, Chelsea, Reardon-Smith, Kate, Guillory, Laura, Pudmenzky, Christa, Nguyen-Huy, Thong, Mushtaq, Shahbaz and Stone, Roger. 2021. "Northern Australia Climate Program: supporting adaptation in rangeland grazing systems through more targeted climate forecasts, improved drought information and an innovative extension program." The Rangeland Journal. 43 (3), pp. 87-100. https://doi.org/10.1071/RJ20074Novel hybrid deep learning model for satellite based PM10 forecasting in the most polluted Australian hotspots
Sharma, Ekta, Deo, Ravinesh C., Soar, Jeffrey, Prasad, Ramendra, Parisi, Alfio V. and Raj, Nawin. 2022. "Novel hybrid deep learning model for satellite based PM10 forecasting in the most polluted Australian hotspots." Atmospheric Environment. 279, pp. 1-13. https://doi.org/10.1016/j.atmosenv.2022.119111New double decomposition deep learning methods for river water level forecasting
Ahmed, A. A. Masrur, Deo, Ravinesh C., Ghahramani, Afshin, Feng, Qi, Raj, Nawin, Yin, Zhenliang and Yang, Linshan. 2022. "New double decomposition deep learning methods for river water level forecasting." Science of the Total Environment. 831, pp. 1-21. https://doi.org/10.1016/j.scitotenv.2022.154722On the Systematic of Anomalous Absorption of Partial Waves by the Nuclear Optical Potential
Dahanayaka, S. D. and Jayasinghe, W. J. M. L. P.. 2012. "On the Systematic of Anomalous Absorption of Partial Waves by the Nuclear Optical Potential." 4th Symposium on Applied Science, Business & Industrial Research (ASBIRES 2012). Sri Lanka 10 May 2012 Sri Lanka.Elastic scattering based on integral equation theory for potentials including the Coulomb potential
Jayasinghe, W. J. M. L. P.. 2015. "Elastic scattering based on integral equation theory for potentials including the Coulomb potential." 7th Applied Science, Business & Industrial Research Symposium (ASBIRES 2015). Sri Lanka 05 Aug 2015 Sri Lanka.Global disparities in agricultural climate index-based insurance research
Adeyinka, Adewuyi Ayodele, Kath, Jarrod, Nguyen-Huy, Thong, Mushtaq, Shahbaz, Souvignet, Maxime, Range, Matthias and Barratt, Jonathan. 2022. "Global disparities in agricultural climate index-based insurance research." Climate Risk Management. 35, pp. 1-15. https://doi.org/10.1016/j.crm.2022.100394Spatiotemporal Hybrid Random Forest Model for Tea Yield Prediction Using Satellite-Derived Variables
Jui, S. Janifer Jabin, Ahmed, A. A. Masrur, Bose, Aditi, Raj, Nawin, Sharma, Ekta, Soar, Jeffrey and Chowdhury, Md Wasique Islam. 2022. "Spatiotemporal Hybrid Random Forest Model for Tea Yield Prediction Using Satellite-Derived Variables." Remote Sensing. 14 (3), pp. 1-18. https://doi.org/10.3390/rs14030805Creating streamtubes based on mass conservative streamlines
Raj, Nawin and Li, Zhenquan. 2008. "Creating streamtubes based on mass conservative streamlines." International Journal of Mathematical, Physical and Engineering Sciences. 2 (1), pp. 41-45. https://doi.org/10.5281/zenodo.1081061Do regenerative grazing management practices improve vegetation and soil health in grazed rangelands? Preliminary insights from a space-for-time study in the Great Barrier Reef catchments, Australia
Bartley, Rebecca, Abbott, Brett N., Ghahramani, Afshin, Ali, Aram, Kerr, Rod, Roth, Christian H. and Kinsey-Henderson, Anne. 2022. "Do regenerative grazing management practices improve vegetation and soil health in grazed rangelands? Preliminary insights from a space-for-time study in the Great Barrier Reef catchments, Australia." The Rangeland Journal. 44 (4), pp. 221-246. https://doi.org/10.1071/RJ22047Prediction of Sea Level with Vertical Land Movement Correction Using Deep Learning
Raj, Nawin. 2022. "Prediction of Sea Level with Vertical Land Movement Correction Using Deep Learning." Mathematics. 10 (23). https://doi.org/10.3390/math10234533Drought outlook validation using ACCESS-S2 hindcast
Nguyen-Huy, Thong and Cobon, David. 2022. Drought outlook validation using ACCESS-S2 hindcast. Northern Australia Climate Program (NACP).Systemic risk management in farming - geographic distribution and diversity
Nguyen-Huy, Thong. 2022. "Systemic risk management in farming - geographic distribution and diversity." Future Drought Fund’s 2022 Science to Practice Forum. 07 - 08 Jun 2022 Australia.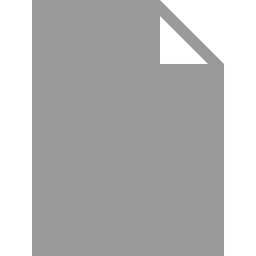