Daily flood forecasts with intelligent data analytic models: multivariate empirical mode decomposition-based modeling methods
Edited book (chapter)
Chapter Title | Daily flood forecasts with intelligent data analytic models: multivariate empirical mode decomposition-based modeling methods |
---|---|
Book Chapter Category | Edited book (chapter) |
ERA Publisher ID | 3337 |
Book Title | Intelligent data analytics for decision-support systems in hazard mitigation: theory and practice of hazard mitigation |
Authors | Prasad, Ramendra (Author), Charan, Dhrishna (Author), Joseph, Lionel (Author), Nguyen-Huy, Thong (Author), Deo, Ravinesh C. (Author) and Singh, Sanjay (Author) |
Editors | Deo, Ravinesh C., Samui, Pijush, Kisi, Ozgur and Yaseen, Zaher Mundher |
Page Range | 359-381 |
Series | Springer Transactions in Civil and Environmental Engineering |
Chapter Number | 17 |
Number of Pages | 23 |
Year | 2021 |
Publisher | Springer |
Place of Publication | Singapore |
ISBN | 9789811557712 |
9789811557729 | |
ISSN | 2363-7633 |
2363-7641 | |
Digital Object Identifier (DOI) | https://doi.org/10.1007/978-981-15-5772-9_17 |
Web Address (URL) | https://link.springer.com/chapter/10.1007/978-981-15-5772-9_17 |
Abstract | Flood causes massive damages to infrastructure, agriculture, livelihood and leads to loss of life. This chapter designs M5 tree-based machine learning model integrated with advanced multivariate empirical mode decomposition (i.e., MEMD-M5 Tree) for daily flood index (FI) forecasting for Lockyer Valley in southeast Queensland, Australia, using data from January 01, 1950, to December 31, 2012. The MEMD-M5 tree is evaluated against MEMD-RF, standalone M5 tree, and RF models via statistical metrics, diagnostic plots with error distributions between simulated and observed daily flood index. The results indicate that MEMD-M5 tree outperforms the comparative models by attaining maximum values of r = 0.990, WI = 0.992, ENS = 0.979, and L = 0.920. The MEMD-M5 tree outperforms other models by registering the least value of RMSE and MAE and can precisely emulate 97.94% of daily FI value. Graphical diagnostic analysis and forecast error histograms further reveal that the MEMD-M5 tree has a greater resemblance to that of the observed data supporting the outcomes of statistical evaluation. Such advancements in flood prediction models, attained through data intelligent analytical methods, are very vital and effective in ensuring better mitigation and civil protection in emergency providing an early warning system, disaster risk reduction, disaster policy suggestions, and reduction of the property damage. |
Keywords | MEMD; M5 tree; flood index forecasting; disaster risk reduction; machine learning |
ANZSRC Field of Research 2020 | 410402. Environmental assessment and monitoring |
460207. Modelling and simulation | |
Public Notes | Files associated with this item cannot be displayed due to copyright restrictions. |
Byline Affiliations | University of Fiji, Fiji |
Centre for Applied Climate Sciences | |
School of Sciences | |
Institution of Origin | University of Southern Queensland |
https://research.usq.edu.au/item/q5wqz/daily-flood-forecasts-with-intelligent-data-analytic-models-multivariate-empirical-mode-decomposition-based-modeling-methods
283
total views9
total downloads0
views this month0
downloads this month
Export as
Related outputs
Multi‑step solar ultraviolet index prediction: integrating convolutional neural networks with long short‑term memory for a representative case study in Queensland, Australia
AL-Musaylh, Mohan, Al‑Dafaie, Kadhem, Downs, Nathan, Ghimire, Sujan, Ali, Mumtaz, Yaseen, Zaher Mundher, Igoe, Damien P., Deo, Ravinesh C., Paris, Alfo V. and A. Jebar, Mustapha A.. 2025. "Multi‑step solar ultraviolet index prediction: integrating convolutional neural networks with long short‑term memory for a representative case study in Queensland, Australia." Modeling Earth Systems and Environment. 11. https://doi.org/https://link.springer.com/article/10.1007/s40808-024-02282-yExplainable deeply-fused nets electricity demand prediction model: Factoring climate predictors for accuracy and deeper insights with probabilistic confidence interval and point-based forecasts
Ghimire, Sujan, AL-Musaylh, Mohanad S., Nguyen-Huy, Thong, Deo, Ravinesh C., Acharya, Rajendra, Casillas-Perez, David, Yaseen, Zaher Mundher and Salcedo-sanz, Sancho. 2025. "Explainable deeply-fused nets electricity demand prediction model: Factoring climate predictors for accuracy and deeper insights with probabilistic confidence interval and point-based forecasts." Applied Energy. 378 (Part A). https://doi.org/10.1016/j.apenergy.2024.124763The mitigating effect of street trees, urban flora, and the suburban environment on seasonal peak UV indices: A case study from Brisbane, Australia
Downs, Nathan James, Amar, Abdurazaq, Dearnaley, John, Butler, Harry, Dekeyser, Stijn, Igoe, Damien, Parisi, Alfio V., Raj, Nawin, Deo, Ravinesh and Turner, Joanna. 2025. "The mitigating effect of street trees, urban flora, and the suburban environment on seasonal peak UV indices: A case study from Brisbane, Australia." Photochemistry and Photobiology. 101 (1), pp. 251-266. https://doi.org/10.1111/php.13988A Comprehensive Review of UAV-UGV Collaboration: Advancements and Challenges
Munasinghe, Isuru, Perera, Asanka and Deo, Ravinesh C.. 2024. "A Comprehensive Review of UAV-UGV Collaboration: Advancements and Challenges." Journal of Sensor and Actuator Networks. 13 (6). https://doi.org/10.3390/jsan13060081Retinal Health Screening Using Artificial Intelligence with Digital Fundus Images: A Review of the Last Decade (2012-2023)
Islam, Saad, Deo, Ravinesh C., Barua, Prabal Datta, Soar, Jeffrey, Yu, Ping and Acharya, U. Rajendra. 2024. "Retinal Health Screening Using Artificial Intelligence with Digital Fundus Images: A Review of the Last Decade (2012-2023)." IEEE Access. 12, pp. 176630-176685. https://doi.org/10.1109/ACCESS.2024.3477420Forecasting River Water Temperature Using Explainable Artificial Intelligence and Hybrid Machine Learning: Case Studies in Menindee Region in Australia
Briceno Medina, Leyde, Joehnk, Klaus, Deo, Ravinesh C., Ali, Mumtaz, Prasad, Salvin S. and Downs, Nathan. 2024. "Forecasting River Water Temperature Using Explainable Artificial Intelligence and Hybrid Machine Learning: Case Studies in Menindee Region in Australia." Water. 16 (24). https://doi.org/10.3390/w16243720Explainable artificial intelligence-machine learning models to estimate overall scores in tertiary preparatory general science course
Ghimire, Sujan, Abdulla, Shahab, Joseph, Lionel P., Prasad, Salvin, Murphy, Angela, Devi, Aruna, Barua, Prabal Datta, Deo, Ravinesh C., Acharya, Rajendra and Yaseen, Zaher Mundher. 2024. "Explainable artificial intelligence-machine learning models to estimate overall scores in tertiary preparatory general science course." Computers and Education: Artificial Intelligence. 7. https://doi.org/10.1016/j.caeai.2024.100331Spatiotemporal performance evaluation of high-resolution multiple satellite and reanalysis precipitation products over the semiarid region of India
Devadarshini, Elangovan, Bhuvaneswari, Kulanthaivelu, Kumar, Shanmugam Mohan, Geethalakshmi, Vellingiri, Dhasarathan, Manickam, Senthil, Alagarsamy, Senthilraja, Kandasamy, Mushtaq, Shahbaz, Nguyen-Huy, Thong, Mai, Thanh and Kouadio, Louis. 2024. "Spatiotemporal performance evaluation of high-resolution multiple satellite and reanalysis precipitation products over the semiarid region of India." Environmental Monitoring and Assessment. 196. https://doi.org/10.1007/s10661-024-13152-6Forecasting Multi-Step Soil Moisture with Three-Phase Hybrid Wavelet-Least Absolute Shrinkage Selection Operator-Long Short-Term Memory Network (moDWT-Lasso-LSTM) Model
Jayasinghe, W. J. M. Lakmini Prarthana, Deo, Ravinesh C., Raj, Nawin, Ghimire, Sujan, Yaseen, Zaher Mundher, Nguyen-Huy, Thong and Ghahramani, Afshin. 2024. "Forecasting Multi-Step Soil Moisture with Three-Phase Hybrid Wavelet-Least Absolute Shrinkage Selection Operator-Long Short-Term Memory Network (moDWT-Lasso-LSTM) Model." Water. 16 (21), p. 3133. https://doi.org/10.3390/w16213133Harnessing a systems approach for sustainable adaptation in vulnerable mega-deltas: A case study of the dyke heightening program in the Vietnamese Mekong Delta's floodplains
Mai, Thanh, Mushtaq, Shahbaz, Tong, Yen Dan, Nguyen-Huy, Thong, Richards, Russell and Marcussen, Torben. 2024. "Harnessing a systems approach for sustainable adaptation in vulnerable mega-deltas: A case study of the dyke heightening program in the Vietnamese Mekong Delta's floodplains." Science of the Total Environment. 954. https://doi.org/10.1016/j.scitotenv.2024.176501Real-time prediction of the week-ahead flood index using hybrid deep learning algorithms with synoptic climate mode indices
Ahmed, A.A. Masrur, Akther, Shahida, Nguyen-Huy, Thong, Raj, Nawin, Jui (Student), S Janifer and S.Z., Farzana. 2024. "Real-time prediction of the week-ahead flood index using hybrid deep learning algorithms with synoptic climate mode indices." Journal of Hydro-Environment Research. 57, pp. 12-26. https://doi.org/10.1016/j.jher.2024.09.001Artificial Intelligence-Empowered Doppler Weather Profile for Low-Earth-Orbit Satellites
Sharma, Ekta, Deo, Ravinesh C., Davey, Christopher P and Carter, Brad D.. 2024. "Artificial Intelligence-Empowered Doppler Weather Profile for Low-Earth-Orbit Satellites." Sensors. 24 (16). https://doi.org/10.3390/s24165271Pyrolytic Pathway of Wheat Straw Pellet by the Thermogravimetric Analyzer
Nath, Bidhan, Bowtell, Les, Chen, Guangnan, Graham, Elizabeth and Nguyen-Huy, Thong. 2024. "Pyrolytic Pathway of Wheat Straw Pellet by the Thermogravimetric Analyzer." Energies. 17 (15). https://doi.org/10.3390/en17153693Wavelet-fusion image super-resolution model with deep learning for downscaling remotely-sensed, multi-band spectral albedo imagery
Karalasingham, Sagthitharan, Deo, Ravinesh C., Casillas-Perez, David, Raj, Nawin and Salcedo-sanz, Sancho. 2024. "Wavelet-fusion image super-resolution model with deep learning for downscaling remotely-sensed, multi-band spectral albedo imagery." Remote Sensing Applications: Society and Environment. 36. https://doi.org/10.1016/j.rsase.2024.101333Half-hourly electricity price prediction with a hybrid convolution neural network-random vector functional link deep learning approach
Ghimire, Sujan, Deo, Ravinesh C., Casillas-Perez, David, Sharma, Ekta, Salcedo-sanz, Sancho, Barua, Prabal and Acharya, U. Rajendra. 2024. "Half-hourly electricity price prediction with a hybrid convolution neural network-random vector functional link deep learning approach." Applied Energy. 374. https://doi.org/10.1016/j.apenergy.2024.123920Towards next-generation federated learning: A case study on privacy attacks in artificial intelligence systems
Sharma, Ekta, Deo, Ravinesh C, Davey, Christopher P., Carter, Brad D. and Salcedo-sanz, Sancho. 2024. "Towards next-generation federated learning: A case study on privacy attacks in artificial intelligence systems." 2024 IEEE Conference on Artificial Intelligence (CAI 2024). Singapore 25 - 27 Jun 2024 United States. IEEE (Institute of Electrical and Electronics Engineers). https://doi.org/10.1109/CAI59869.2024.00259Poster: Cloud Computing with AI-empowered Trends in Software-Defined Radios: Challenges and Opportunities
Sharma, Ekta, Deo, Ravinesh C., Davey, Christopher P., Carter, Brad D. and Salcedo-sanz, Sancho. 2024. "Poster: Cloud Computing with AI-empowered Trends in Software-Defined Radios: Challenges and Opportunities." 2024 IEEE 25th International Symposium on a World of Wireless, Mobile and Multimedia Networks (WoWMoM 2024). Perth, Australia 04 - 07 Jun 2024 United States. IEEE (Institute of Electrical and Electronics Engineers). https://doi.org/10.1109/WoWMoM60985.2024.00054Atmospheric Visibility and Cloud Ceiling Predictions With Hybrid IIS-LSTM Integrated Model: Case Studies for Fiji's Aviation Industry
Raj, Shiveel, Deo, Ravinesh C., Sharma, Ekta, Prasad, Ramendra, Dinh, Toan and Salcedo-sanz, Sancho. 2024. "Atmospheric Visibility and Cloud Ceiling Predictions With Hybrid IIS-LSTM Integrated Model: Case Studies for Fiji's Aviation Industry." IEEE Access. 12, pp. 72530-72543. https://doi.org/10.1109/ACCESS.2024.3401091Point-based and probabilistic electricity demand prediction with a Neural Facebook Prophet and Kernel Density Estimation model
Ghimire, Sujan, Deo, Ravinesh C., Pourmousavi, S. Ali, Casillas-Perez, David and Salcedo-sanz, Sancho. 2024. "Point-based and probabilistic electricity demand prediction with a Neural Facebook Prophet and Kernel Density Estimation model." Engineering Applications of Artificial Intelligence. 135. https://doi.org/10.1016/j.engappai.2024.108702End-to-end learning of adaptive coded modulation schemes for resilient wireless communications
Davey, Christopher P., Shakeel, Ismail, Deo, Ravinesh C., Sharma, Ekta, Salcedo-sanz, Sancho and Soar, Jeffrey. 2024. "End-to-end learning of adaptive coded modulation schemes for resilient wireless communications." Applied Soft Computing. 159. https://doi.org/10.1016/j.asoc.2024.111672Deep Learning Based Over-the-Air Training of Wireless Communication Systems without Feedback
Davey, Christopher P., Shakeel, Ismail, Deo, Ravinesh C. and Salcedo-sanz, Sancho. 2024. "Deep Learning Based Over-the-Air Training of Wireless Communication Systems without Feedback." Sensors. 24 (10). https://doi.org/10.3390/s24102993Pyrolysis of wheat straw pellets in a pilot-scale reactor: Effect of temperature and residence time
Nath, Bidhan, Chen, Guangnan, Bowtell, Les and Nguyen-Huy, Thong. 2024. "Pyrolysis of wheat straw pellets in a pilot-scale reactor: Effect of temperature and residence time." Energy Science and Engineering. 12 (8), pp. 3524-3539. https://doi.org/10.1002/ese3.1833Integrating rainfall index-based insurance with optimal crop management strategies can reduce financial risks for Australian dryland cotton farmers
Nguyen-Huy, Thong, Kath, Jarrod, Kouadio, Louis, King, Rachel, Mushtaq, Shahbaz and Barratt, Jonathan. 2024. "Integrating rainfall index-based insurance with optimal crop management strategies can reduce financial risks for Australian dryland cotton farmers." Sustainable Futures. 8. https://doi.org/10.1016/j.sftr.2024.100249A playground shade index for standardising ultraviolet protection assessments of open, mixed and protected outdoor recreational spaces
Downs, Nathan, Butler, Harry, Dexter, Benjamin, Raj, Nawin, Downs, Melanie, Turner, Joanna, Dekeyser, Stijn, Deo, Ravinesh, Vanos, Jennifer, Igoe, Damien and Parisi, Alfio V. 2024. "A playground shade index for standardising ultraviolet protection assessments of open, mixed and protected outdoor recreational spaces." 6th International conference UV and Skin Cancer Prevention. Brisbane, Australia 11 - 15 Sep 2024 Australia.Multi-Step-Ahead Wind Speed Forecast System: Hybrid Multivariate Decomposition and Feature Selection-Based Gated Additive Tree Ensemble Model
Joseph, Lionel P., Deo, Ravinesh C., Casillas-Perez, David, Prasad, Ramendra, Raj, Nawin and Salcedo-sanz, Sancho. 2024. "Multi-Step-Ahead Wind Speed Forecast System: Hybrid Multivariate Decomposition and Feature Selection-Based Gated Additive Tree Ensemble Model." IEEE Access. 12, pp. 58750-58777. https://doi.org/10.1109/ACCESS.2024.3392899Deep Learning-Assisted Sensitive 3C-SiC Sensor for Long-Term Monitoring of Physical Respiration
Tran, Thi Lap, Nguyen, Duy Van, Nguyen, Hung, Nguyen, Thi Phuoc Van, Song, Pingan, Deo, Ravinesh C, Moloney, Clint, Dao, Viet Dung, Nguyen, Nam-Trung and Dinh, Toan. 2024. "Deep Learning-Assisted Sensitive 3C-SiC Sensor for Long-Term Monitoring of Physical Respiration." Advanced Sensor Research. 3 (8). https://doi.org/10.1002/adsr.202300159Copula-Probabilistic Flood Risk Analysis with an Hourly Flood Monitoring Index
Chand, Ravinesh, Nguyen-Huy, Thong, Deo, Ravinesh C., Ghimire, Sujan, Ali, Mumtaz and Ghahramani, Afshin. 2024. "Copula-Probabilistic Flood Risk Analysis with an Hourly Flood Monitoring Index." Water. 16 (11). https://doi.org/10.3390/w16111560Short-term wind speed forecasting using an optimized three-phase convolutional neural network fused with bidirectional long short-term memory network model
Joseph, Lionel P., Deo, Ravinesh C., Casillas-Perez, David, Prasad, Ramendra, Raj, Nawin and Salcedo-sanz, Sancho. 2024. "Short-term wind speed forecasting using an optimized three-phase convolutional neural network fused with bidirectional long short-term memory network model." Applied Energy. 359. https://doi.org/10.1016/j.apenergy.2024.122624Probabilistic-based electricity demand forecasting with hybrid convolutional neural network-extreme learning machine model
Ghimire, Sujan, Deo, Ravinesh C., Casillas-Perez, David, Salcedo-sanz, Sancho, Pourmousavi, S. Ali and Acharya, U. Rajendra. 2024. "Probabilistic-based electricity demand forecasting with hybrid convolutional neural network-extreme learning machine model." Engineering Applications of Artificial Intelligence. 132. https://doi.org/10.1016/j.engappai.2024.107918Electricity demand error corrections with attention bi-directional neural networks
Ghimire, Sujan, Deo, Ravinesh C., Casillas-Perez, David and Salcedo-sanz, Sancho. 2024. "Electricity demand error corrections with attention bi-directional neural networks." Energy. 291. https://doi.org/10.1016/j.energy.2023.129938Micromachined Mechanical Resonant Sensors: From Materials, Structural Designs to Applications
Dinh, Toan, Rais-Zadeh, Mina, Nguyen, Thanh, Phan, Hoang-Phuong, Song, Pingan, Deo, Ravinesh, Dao, Dzung, Nguyen, Nam-Trung and Bell, John. 2024. "Micromachined Mechanical Resonant Sensors: From Materials, Structural Designs to Applications." Advanced Materials Technologies. 9 (2). https://doi.org/10.1002/admt.202300913Machine learning for expediting next-generation of fire-retardant polymer composites
Jafari, Pooya, Zhang, Ruoran, Huo, Siqi, Wang, Qingsheng, Yong, Jianming, Hong, Min, Deo, Ravinesh, Wang, Hao and Song, Pingan. 2024. "Machine learning for expediting next-generation of fire-retardant polymer composites." Composites Communications. 45. https://doi.org/10.1016/j.coco.2023.101806Very short-term solar ultraviolet-A radiation forecasting system with cloud cover images and a Bayesian optimized interpretable artificial intelligence model
Prasad, Salvin Sanjesh, Deo, Ravinesh Chand, Downs, Nathan James, Casillas-Perez, David, Salcedo-sanz, Sancho and Parisi, Alfio Venerando. 2024. "Very short-term solar ultraviolet-A radiation forecasting system with cloud cover images and a Bayesian optimized interpretable artificial intelligence model ." Expert Systems with Applications. 236. https://doi.org/10.1016/j.eswa.2023.121273Short-term wave power forecasting with hybrid multivariate variational mode decomposition model integrated with cascaded feedforward neural networks
Ali, Mumtaz, Prasad, Ramendra, Jamei, Mehdi, Malik, Anurag, Xiang, Yong, Abdulla, Shahab, Deo, Ravinesh C., Farooque, Aitazaz A. and Labban, Abdulhaleem H.. 2024. "Short-term wave power forecasting with hybrid multivariate variational mode decomposition model integrated with cascaded feedforward neural networks." Renewable Energy. 221. https://doi.org/10.1016/j.renene.2023.119773Two-step deep learning framework with error compensation technique for short-term, half-hourly electricity price forecasting
Ghimire, Sujan, Deo, Ravinesh C., Casillas-Perez, David and Salcedo-sanz, Sancho. 2024. "Two-step deep learning framework with error compensation technique for short-term, half-hourly electricity price forecasting." Applied Energy. 353 (Part A). https://doi.org/10.1016/j.apenergy.2023.122059Hybrid Deep Learning Model for Wave Height Prediction in Australia's Wave Energy Region
Ahmed, Abul Abrar Masrur, Jui, S Janifer Jabin, AL-Musaylh, Mohanad S., Raj, Nawin, Saha, Reepa, Deo, Ravinesh C and Saha, Sanjoy Kumar. 2024. "Hybrid Deep Learning Model for Wave Height Prediction in Australia's Wave Energy Region." Applied Soft Computing. 150. https://doi.org/10.1016/j.asoc.2023.111003Gully erosion mapping susceptibility in a Mediterranean environment: A hybrid decision-making model
Hitouri, Sliman, Meriame, Mohajane, Ajim, Ali Sk, Pacheco, Quevedo Renata, Nguyen-Huy, Thong, Pham, Quoc Bao, ElKhrachy, Ismail and Varasano, Antonietta. 2024. "Gully erosion mapping susceptibility in a Mediterranean environment: A hybrid decision-making model." International Soil and Water Conservation Research. 12 (2), pp. 279-297. https://doi.org/10.1016/j.iswcr.2023.09.008Modelling future spatial distribution of peanut crops in Australia under climate change scenarios
Haerani, Haerani, Apan, Armando, Nguyen-Huy, Thong and Basnet, Badri. 2024. "Modelling future spatial distribution of peanut crops in Australia under climate change scenarios." Geo-spatial Information Science. 27 (5), pp. 1585-1604. https://doi.org/10.1080/10095020.2022.2155255Channel-Agnostic Training of Transmitter and Receiver for Wireless Communications
Davey, Christopher P., Shakeel, Ismail, Deo, Ravinesh C. and Salcedo-sanz, Sancho. 2023. "Channel-Agnostic Training of Transmitter and Receiver for Wireless Communications ." Sensors. 23 (24). https://doi.org/10.3390/s23249848Deep Image Analysis for Microalgae Identification
Soar, Jeffrey, Lih, Oh Shu, Wen, Loh Hui, Ward, Aleth, Sharma, Ekta, Deo, Ravinesh C., Barua, Prabal Datta, Tan, Ru-San, Rinen, Eliezer and Acharya, Rajendra. 2023. "Deep Image Analysis for Microalgae Identification." Lecture notes in computer science. Switzerland . Springer. pp. 280-292Climate change and human health in Vietnam: a systematic review and additional analyses on current impacts, future risk, and adaptation
Tran, Nu Quy Linh, Le, Huynh Thi Cam Hong, Pham, Cong Tuan, Nguyen, Xuan Huong, Tran, Ngoc Dang, Tran, Tuyet-Hanh Thi, Nghiem, Son, Luong, Thi Mai Ly, Bui, Vinh, Nguyen-Huy, Thong, Doan, Van Quang, Dang, Kim Anh, Do, Thi Hoai Thuong, Ngo, Hieu Kim Thi, Nguyen, Truong Vien, Nguyen, Ngoc Huy, Do, Manh Cuong, Ton, Tuan Nghia, Dang, Thi Anh Thu, ..., Phung, Dung. 2023. "Climate change and human health in Vietnam: a systematic review and additional analyses on current impacts, future risk, and adaptation." The Lancet Regional Health - Western Pacific. 40. https://doi.org/10.1016/j.lanwpc.2023.100943Enhancing climate resilience by combining practice and insurance strategies: A case study for cotton crop
Nguyen-Huy, Thong and Battersby, Kerry. 2023. "Enhancing climate resilience by combining practice and insurance strategies: A case study for cotton crop." Queensland Disaster Management Research Forum 2023. Brisbane, Australia 07 - 07 Nov 2023Statistical and spatial analysis for soil heavy metals over the Murray-Darling river basin in Australia
Tao, Hai, Al-Hilali, Aqeel Ali, Ahmed, Ali M., Mussa, Zainab Haider, Falah, Mayadah W., Abed, Salwan Ali, Deo, Ravinesh, Jawad, Ali H., Maulud, Khairul Nizam Abdul, Latif, Mohd Talib and Yaseen, Zaher Mundher. 2023. "Statistical and spatial analysis for soil heavy metals over the Murray-Darling river basin in Australia." Chemosphere. 317. https://doi.org/10.1016/j.chemosphere.2023.137914Enhanced joint hybrid deep neural network explainable artificial intelligence model for 1-hr ahead solar ultraviolet index prediction
Prasad, Salvin S., Deo, Ravinesh C., Salcedo-sanz, Sancho, Downs, Nathan J., Casillas-Perez, David and Parisi, Alfio V.. 2023. "Enhanced joint hybrid deep neural network explainable artificial intelligence model for 1-hr ahead solar ultraviolet index prediction." Computer Methods and Programs in Biomedicine. 241. https://doi.org/10.1016/j.cmpb.2023.107737Ampelomyces mycoparasites of powdery mildews–a review
Prahl, Rosa E., Khan, Shahjahan and Deo, Ravinesh C.. 2023. "Ampelomyces mycoparasites of powdery mildews–a review." Canadian Journal of Plant Pathology. 45 (4), pp. 391-404. https://doi.org/10.1080/07060661.2023.2206378A fuzzy-based cascade ensemble model for improving extreme wind speeds prediction
Pelaez-Rodriguez, C., Perez-Aracil, J., Prieto-Godino, L., Ghimire, S., Deo, R. and Salcedo-sanz, S.. 2023. "A fuzzy-based cascade ensemble model for improving extreme wind speeds prediction." Journal of Wind Engineering and Industrial Aerodynamics. 240. https://doi.org/10.1016/j.jweia.2023.105507Explainable AI approach with original vegetation data classifies spatio-temporal nitrogen in flows from ungauged catchments to the Great Barrier Reef
O’Sullivan, Cherie M., Deo, Ravinesh C. and Ghahramani, Afshin. 2023. "Explainable AI approach with original vegetation data classifies spatio-temporal nitrogen in flows from ungauged catchments to the Great Barrier Reef." Scientific Reports. 13 (1). https://doi.org/10.1038/s41598-023-45259-0A Novel Attention-Based Model for Semantic Segmentation of Prostate Glands Using Histopathological Images
Inamdar, Mahesh Anil, Raghavendra, U., Gudigar, Anjan, Bhandary, Sarvesh, Salvi, Massimo, Deo, Ravinesh C., Barua, Prabal Datta, Ciaccio, Edward J., Molinari, Filippo and Acharya, RU. Rajendra. 2023. "A Novel Attention-Based Model for Semantic Segmentation of Prostate Glands Using Histopathological Images." IEEE Access. 11, pp. 108982-108994. https://doi.org/10.1109/ACCESS.2023.3321273Efficient daily electricity demand prediction with hybrid deep-learning multi-algorithm approach
Ghimire, Sujan, Deo, Ravinesh C., Casillas-Perez, David and Salcedo-sanz, Sancho. 2023. "Efficient daily electricity demand prediction with hybrid deep-learning multi-algorithm approach." Energy Conversion and Management. 297. https://doi.org/10.1016/j.enconman.2023.117707A drought monitor for Australia
Guillory, Laura, Pudmenzky, Christa, Nguyen-Huy, Thong, Cobon, David and Stone, Roger. 2023. "A drought monitor for Australia." Environmental Modelling and Software. 170. https://doi.org/10.1016/j.envsoft.2023.105852Automated detection of airflow obstructive diseases: A systematic review of the last decade (2013-2022)
Xu, Shuting, Deo, Ravinesh C, Soar, Jeffrey, Barua, Prabal Datta, Faust, Oliver, Homaira, Nusrat, Jaffe, Adam, Kabir, Arm Luthful and Acharya, U. Rajendra. 2023. "Automated detection of airflow obstructive diseases: A systematic review of the last decade (2013-2022)." Computer Methods and Programs in Biomedicine. 241. https://doi.org/10.1016/j.cmpb.2023.107746Brain tumor detection and screening using artificial intelligence techniques: Current trends and future perspectives
Raghavendra, U., Gudigar, Anjan, Paul, Aritra, Goutham, T.S., Inamdar, Mahesh Anil, Hegde, Ajay, Dev, Aruna, Ooi, Chui Ping, Deo, Ravinesh C., Barua, Prabal Datta, Molinari, Filippo, Ciaccio, Edward J. and Acharya, U. Rajendra. 2023. "Brain tumor detection and screening using artificial intelligence techniques: Current trends and future perspectives." Computers in Biology and Medicine. 163. https://doi.org/10.1016/j.compbiomed.2023.107063Application of Entropy for Automated Detection of Neurological Disorders With Electroencephalogram Signals: A Review of the Last Decade (2012-2022)
Jui, S. Janifer Jabin, Deo, Ravinesh C. Deo, Barua, Prabal Datta, Devi, Aruna, Soar, Jeffrey and Acharya, U. Rajendra. 2023. "Application of Entropy for Automated Detection of Neurological Disorders With Electroencephalogram Signals: A Review of the Last Decade (2012-2022)." IEEE Access. 11, pp. 71905-71924. https://doi.org/10.1109/ACCESS.2023.3294473Integrated Multi-Head Self-Attention Transformer model for electricity demand prediction incorporating local climate variables
Ghimire, Sujan, Nguyen-Huy, Thong, AL-Musaylh, Mohanad S., Deo, Ravinesh C., Casillas-Perez, David and Salcedo-sanz, Sancho. 2023. "Integrated Multi-Head Self-Attention Transformer model for electricity demand prediction incorporating local climate variables." Energy and AI. 14. https://doi.org/10.1016/j.egyai.2023.100302A systematic review of emerging environmental markets: Potential pathways to creating shared value for communities
Mittahalli Byrareddy, Vivekananda M, Islam, Md Aminul, Nguyen-Huy, Thong and Slaughter, Geoff. 2023. "A systematic review of emerging environmental markets: Potential pathways to creating shared value for communities ." Heliyon. 9 (9). https://doi.org/10.1016/j.heliyon.2023.e19754Integrating management decisions and insurance to drive climate adaptation in the agricultural industry
Nguyen-Huy, Thong. 2023. "Integrating management decisions and insurance to drive climate adaptation in the agricultural industry ." Climate Adaptation 2023. Adelaide, Australia 25 - 27 Jul 2023 Australia.Flash flood-risk areas zoning using integration of decision-making trial and evaluation laboratory, GIS-based analytic network process and satellite-derived information
Taherizadeh, Mehrnoosh, Niknam, Arman, Nguyen-Huy, Thong, Mezősi, Gábor and Sarli, Reza. 2023. "Flash flood-risk areas zoning using integration of decision-making trial and evaluation laboratory, GIS-based analytic network process and satellite-derived information." Natural Hazards. 118 (3), p. 2309–2335. https://doi.org/10.1007/s11069-023-06089-5Revealing the effect of an industrial flash flood on vegetation area: a case study of Khusheh Mehr in Maragheh-Bonab Plain, Iran
Taherizadeh, Mehrnoosh, Khushemehr, Javid Hojabri, Niknam, Arman, Nguyen-Huy, Thong and Mezősi, Gábor. 2023. "Revealing the effect of an industrial flash flood on vegetation area: a case study of Khusheh Mehr in Maragheh-Bonab Plain, Iran." Remote Sensing Applications: Society and Environment. 32. https://doi.org/10.1016/j.rsase.2023.101016The Great 2011 Thailand flood disaster revisited: Could it have been mitigated by different dam operations based on better weather forecasts?
Loc, Ho Huu, Emadzadeh, Adel, Park, Edward, Nontikansak, Piyanuch and Deo, Ravinesh C.. 2023. "The Great 2011 Thailand flood disaster revisited: Could it have been mitigated by different dam operations based on better weather forecasts? " Environmental Research. 216 (Part 2). https://doi.org/10.1016/j.envres.2022.114493Comparison of machine learning methods emulating process driven crop models
Johnston, David B., Pembleton, Keith G., Huth, Neil I. and Deo, Ravinesh C.. 2023. "Comparison of machine learning methods emulating process driven crop models ." Environmental Modelling and Software. 162. https://doi.org/10.1016/j.envsoft.2023.105634A novel approach based on integration of convolutional neural networks and echo state network for daily electricity demand prediction
Ghimire, Sujan, Nguyen-Huy, Thong, AL-Musaylh, Mohanad S., Deo, Ravinesh, Casillas-Perez, David and Salcedo-sanz, Sancho. 2023. "A novel approach based on integration of convolutional neural networks and echo state network for daily electricity demand prediction." Energy. 275, p. 127430. https://doi.org/10.1016/j.energy.2023.127430Climatology and composite evolution of flash drought over Australia and its vegetation impacts
Nguyen, Hanh, Wheeler, Mattew C., Otkin, Jason A., Nguyen-Huy, Thong and Cowan, Timothy. 2023. "Climatology and composite evolution of flash drought over Australia and its vegetation impacts." Journal of Hydrometeorology. 24 (6), p. 1087–1101. https://doi.org/10.1175/JHM-D-22-0033.1Digital Farming: An Overview of Its Fundamental Components and Applications
Nguyen-Huy, Thong. 2023. "Digital Farming: An Overview of Its Fundamental Components and Applications." The 4th International Conference of Food Security and Sustainable Agriculture in the Tropics. South Sulawesi, Indonesia 15 - 16 Feb 2023 Indonesia.Accurate Image Multi-Class Classification Neural Network Model with Quantum Entanglement Approach
Riaz, Farina, Abdulla, Shahab, Suzuki, Hajime, Ganguly, Srinjoy, Deo, Ravinesh C. and Hopkins, Susan. 2023. "Accurate Image Multi-Class Classification Neural Network Model with Quantum Entanglement Approach." Sensors. 23 (5), pp. 1-11. https://doi.org/10.3390/s23052753Near real-time wind speed forecast model with bidirectional LSTM networks
Joseph, Lionel P., Deo, Ravinesh C., Prasad, Ramendra, Salcedo-Sanz, Sancho, Raj, Nawin and Soar, Jeffrey. 2023. "Near real-time wind speed forecast model with bidirectional LSTM networks." Renewable Energy. 204, pp. 39-58. https://doi.org/10.1016/j.renene.2022.12.123Downscaling Surface Albedo to Higher Spatial Resolutions With an Image Super-Resolution Approach and PROBA-V Satellite Images
Deo, Ravinesh C., Karalasingham, Sagthitharan, Casillas-Perez, David, Raj, Narwin and Salcedo-sanz, Sancho. 2023. "Downscaling Surface Albedo to Higher Spatial Resolutions With an Image Super-Resolution Approach and PROBA-V Satellite Images." IEEE Access. 11, pp. 5558-5577. https://doi.org/10.1109/ACCESS.2023.3236253Developing a novel hybrid method based on dispersion entropy and adaptive boosting algorithm for human activity recognition
Diykh, Mohammed, Abdulla, Shahab, Deo, Ravinesh C, Siuly, Siuly and Ali, Mumtaz. 2023. "Developing a novel hybrid method based on dispersion entropy and adaptive boosting algorithm for human activity recognition." Computer Methods and Programs in Biomedicine. 229. https://doi.org/10.1016/j.cmpb.2022.107305Cloud cover bias correction in numerical weather models for solar energy monitoring and forecasting systems with kernel ridge regression
Deo, Ravinesh C., Ahmed, A.A. Masrur, Casillas-Perez, David, Pourmousavi, S. Ali, Segal, Gary, Yu, Yanshan and Salcedo-sanz, Sancho. 2023. "Cloud cover bias correction in numerical weather models for solar energy monitoring and forecasting systems with kernel ridge regression." Renewable Energy. 203, pp. 113-130. https://doi.org/10.1016/j.renene.2022.12.048Deep Multi-Stage Reference Evapotranspiration Forecasting Model: Multivariate Empirical Mode Decomposition Integrated With the Boruta-Random Forest Algorithm
Jayasinghe, W. J. M. Lakmini Prarthana, Deo, Ravinesh C., Ghahramani, Afshin, Ghimire, Sujan and Raj, Nawin. 2021. "Deep Multi-Stage Reference Evapotranspiration Forecasting Model: Multivariate Empirical Mode Decomposition Integrated With the Boruta-Random Forest Algorithm." IEEE Access. 9, pp. 166695-166708. https://doi.org/10.1109/ACCESS.2021.3135362Designing Deep-based Learning Flood Forecast Model with ConvLSTM Hybrid Algorithm
Moishin, Mohammed, Deo, Ravinesh C., Prasad, Ramendra, Raj, Nawin and Abdulla, Shahab. 2021. "Designing Deep-based Learning Flood Forecast Model with ConvLSTM Hybrid Algorithm." IEEE Access. 9, pp. 50982-50993. https://doi.org/10.1109/ACCESS.2021.3065939Pattern recognition describing spatio-temporal drivers of catchment classification for water quality
O'Sullivan, Cherie M., Ghahramani, Afshin, Deo, Ravinesh C. and Pembleton, Keith G.. 2023. "Pattern recognition describing spatio-temporal drivers of catchment classification for water quality." Science of the Total Environment. 861, pp. 1-42. https://doi.org/10.1016/j.scitotenv.2022.160240The Playground Shade Index: A New Design Metric for Measuring Shade and Seasonal Ultraviolet Protection Characteristics of Parks and Playgrounds
Downs, Nathan, Raj, Nawin, Vanos, Jennifer, Parisi, Alfio, Butler, Harry, Deo, Ravinesh, Igoe, Damien, Dexter, Benjamin, Beckman-Downs, Melanie, Turner, Joanna and Dekeyser, Stijn. 2023. "The Playground Shade Index: A New Design Metric for Measuring Shade and Seasonal Ultraviolet Protection Characteristics of Parks and Playgrounds." Photochemistry and Photobiology. 99 (4), pp. 1193-1207. https://doi.org/10.1111/php.13745Using Sequence-to-Sequence Models for Carrier Frequency Offset Estimation of Short Messages and Chaotic Maps
Davey, Christopher P., Shakeel, Ismail, Deo, Ravinesh C., Salcedo-sanz, Sancho and Soar, Jeffrey. 2022. "Using Sequence-to-Sequence Models for Carrier Frequency Offset Estimation of Short Messages and Chaotic Maps." IEEE Access. 10, pp. 119814 - 119825. https://doi.org/10.1109/ACCESS.2022.3221762Modelling and Real-time Optimisation of Air Quality Predictions for Australia through Artificial Intelligence Algorithm
Sharma, Ekta, Deo, Ravinesh C., Prasad, Ramendra and Parisi, Alfio V.. 2019. "Modelling and Real-time Optimisation of Air Quality Predictions for Australia through Artificial Intelligence Algorithm." AMSI Optimise 2019. Perth, Australia 17 - 21 Jun 2019 Perth, Australia.Hybrid Convolutional Neural Network-Multilayer Perceptron Model for Solar Radiation Prediction
Ghimire, Sujan, Nguyen-Huy, Thong, Prasad, Ramendra, Deo, Ravinesh C., Casillas-Perez, David, Salcedo-sanz, Sancho and Bhandari, Binayak. 2023. "Hybrid Convolutional Neural Network-Multilayer Perceptron Model for Solar Radiation Prediction." Cognitive Computation. 15 (2), pp. 645-671. https://doi.org/10.1007/s12559-022-10070-yVapour pressure deficit determines critical thresholds for global coffee production under climate change
Kath, Jarrod, Craparo, Alessandro, Fong, Youyi, Byrareddy, Vivekananda, Davis, Aaron P., King, Rachel, Nguyen-Huy, Thong, van Asten, Piet J. A., Marcussen, Torben, Mushtaq, Shahbaz, Stone, Roger and Power, Scott. 2022. "Vapour pressure deficit determines critical thresholds for global coffee production under climate change." Nature Food. 3, pp. 871-880. https://doi.org/10.1038/s43016-022-00614-8Student Performance Predictions for Advanced Engineering Mathematics Course With New Multivariate Copula Models
Nguyen-Huy, Thong, Deo, Ravinesh C., Khan, Shahjahan, Devi, Aruna, Adeyinka, Adewuyi Ayodele, Apan, Armando A. and Yaseen, Zaher Mundher. 2022. "Student Performance Predictions for Advanced Engineering Mathematics Course With New Multivariate Copula Models." IEEE Access. 10, pp. 45112 -45136. https://doi.org/10.1109/ACCESS.2022.3168322Rapid assessment of mine rehabilitation areas with airborne LiDAR and deep learning: bauxite strip mining in Queensland, Australia
Murray, Xavier, Apan, Armando, Deo, Ravinesh and Maraseni, Tek. 2022. "Rapid assessment of mine rehabilitation areas with airborne LiDAR and deep learning: bauxite strip mining in Queensland, Australia." Geocarto International. 37 (26), pp. 11223-11252. https://doi.org/10.1080/10106049.2022.2048902Multi-strategy Slime Mould Algorithm for hydropower multi-reservoir systems optimization
Ahmadianfar, Iman, Noori, Ramzia Majeed, Togun, Hussein, Falah, Mayadah W., Homod, Raad Z., Fu, Minglei, Halder, Bijay, Deo, Ravinesh and Yaseen, Zaher Mundher. 2022. "Multi-strategy Slime Mould Algorithm for hydropower multi-reservoir systems optimization." Knowledge-Based Systems. 250, pp. 1-18. https://doi.org/10.1016/j.knosys.2022.109048Suspended sediment load modeling using advanced hybrid rotation forest based elastic network approach
Khosravi, Khabat, Golkarian, Ali, Melesse, Assefa M. and Deo, Ravinesh C.. 2022. "Suspended sediment load modeling using advanced hybrid rotation forest based elastic network approach." Journal of Hydrology. 610, pp. 1-14. https://doi.org/10.1016/j.jhydrol.2022.127963Delineating the Crop-Land Dynamic due to Extreme Environment Using Landsat Datasets: A Case Study
Halder, Bijay, Bandyopadhyay, Jatisankar, Afan, Haitham Abdulmohsin, Naser, Maryam H., Abed, Salwan Ali, Khedher, Khaled Mohamed, Falih, Khaldoon T., Deo, Ravinesh, Scholz, Miklas and Yaseen, Zaher Mundher. 2022. "Delineating the Crop-Land Dynamic due to Extreme Environment Using Landsat Datasets: A Case Study." Agronomy. 12 (6), pp. 1-23. https://doi.org/10.3390/agronomy12061268Coupled online sequential extreme learning machine model with ant colony optimization algorithm for wheat yield prediction
Ali, Mumtaz, Deo, Ravinesh C., Xiang, Yong, Prasad, Ramendra, Li, Jianxin, Farooque, Aitazaz and Yaseen, Zaher Mundher. 2022. "Coupled online sequential extreme learning machine model with ant colony optimization algorithm for wheat yield prediction." Scientific Reports. 12 (1), pp. 1-23. https://doi.org/10.1038/s41598-022-09482-5Forecasting solar photosynthetic photon flux density under cloud cover effects: novel predictive model using convolutional neural network integrated with long short-term memory network
Deo, Ravinesh C., Grant, Richard H., Webb, Ann, Ghimire, Sujan, Igoe, Damien P., Downs, Nathan J., Al-Musaylh, Mohanad S., Parisi, Alfio V. and Soar, Jeffrey. 2022. "Forecasting solar photosynthetic photon flux density under cloud cover effects: novel predictive model using convolutional neural network integrated with long short-term memory network." Stochastic Environmental Research and Risk Assessment. 36, p. 3183–3220. https://doi.org/10.1007/s00477-022-02188-0Improved Complete Ensemble Empirical Mode Decomposition with Adaptive Noise Deep Residual model for short-term multi-step solar radiation prediction
Ghimire, Sujan, Deo, Ravinesh C, Casillas-Perez, David and Salcedo-sanz, Sancho. 2022. "Improved Complete Ensemble Empirical Mode Decomposition with Adaptive Noise Deep Residual model for short-term multi-step solar radiation prediction." Renewable Energy. 190, pp. 408-424. https://doi.org/10.1016/j.renene.2022.03.120Efficient daily solar radiation prediction with deep learning 4-phase convolutional neural network, dual stage stacked regression and support vector machine CNN-REGST hybrid model
Ghimire, Sujan, Nguyen-Huy, Thong, Deo, Ravinesh C., Casillas-Perez, David and Salcedo-sanz, Sancho. 2022. "Efficient daily solar radiation prediction with deep learning 4-phase convolutional neural network, dual stage stacked regression and support vector machine CNN-REGST hybrid model." Sustainable Materials and Technologies. 32, pp. 1-24. https://doi.org/10.1016/j.susmat.2022.e00429Hybrid deep CNN-SVR algorithm for solar radiation prediction problems in Queensland, Australia
Ghimire, Sujan, Bhandari, Binayak, Casillas-Perez, David, Deo, Ravinesh C. and Salcedo-sanz, Sancho. 2022. "Hybrid deep CNN-SVR algorithm for solar radiation prediction problems in Queensland, Australia." Engineering Applications of Artificial Intelligence. 112, pp. 1-26. https://doi.org/10.1016/j.engappai.2022.104860Boosting solar radiation predictions with global climate models, observational predictors and hybrid deep-machine learning algorithms
Ghimire, Sujan, Deo, Ravinesh C., Casillas-Perez, David and Salcedo-sanz, Sancho. 2022. "Boosting solar radiation predictions with global climate models, observational predictors and hybrid deep-machine learning algorithms." Applied Energy. 316, pp. 1-25. https://doi.org/10.1016/j.apenergy.2022.119063Machine learning regression and classification methods for fog events prediction
Castillo-Boton, C., Casillas-Perez, D., Casanova-Mateo, C., Ghimire, S., Cerro-Prada, E., Gutierrez, P. A., Deo, R. C. and Salcedo-sanz, S.. 2022. "Machine learning regression and classification methods for fog events prediction." Atmospheric Research. 272, pp. 1-23. https://doi.org/10.1016/j.atmosres.2022.106157Quantum Artificial Intelligence Predictions Enhancement by Improving Signal Processing
Riaz, Farina, Abdulla, Shahab, Ni, Wei, Radfar, Mohsen, Deo, Ravinesh and Hopkins, Susan. 2022. "Quantum Artificial Intelligence Predictions Enhancement by Improving Signal Processing." Quantum Australia Conference 2022. Online 23 - 25 Feb 2022 Toowoomba, Australia. https://doi.org/10.13140/RG.2.2.34754.66245Forecasting Daily Flood Water Level Using Hybrid Advanced Machine Learning Based Time‑Varying Filtered Empirical Mode Decomposition Approach
Jamei, Mehdi, Ali, Mumtaz, Malik, Anurag, Prasad, Ramendra, Abdulla, Shahab and Yaseen, Zaher Mundher. 2022. "Forecasting Daily Flood Water Level Using Hybrid Advanced Machine Learning Based Time‑Varying Filtered Empirical Mode Decomposition Approach." Water Resources Management. 36 (12), p. 4637–4676. https://doi.org/10.1007/s11269-022-03270-6A satellite-based Standardized Antecedent Precipitation Index (SAPI) for mapping extreme rainfall risk in Myanmar
Nguyen-Huy, Thong, Kath, Jarrod, Nagler, Thomas, Khaung, Ye, Aung, Thee Su Su, Mushtaq, Shahbaz, Marcussen, Torben and Stone, Roger. 2022. "A satellite-based Standardized Antecedent Precipitation Index (SAPI) for mapping extreme rainfall risk in Myanmar." Remote Sensing Applications: Society and Environment. 26, pp. 1-19. https://doi.org/10.1016/j.rsase.2022.100733Deep learning CNN-LSTM-MLP hybrid fusion model for feature optimizations and daily solar radiation prediction
Ghimire, Sujan, Deo, Ravinesh C., Casillas-Perez, David, Salcedo-sanz, Sancho, Sharma, Ekta and Ali, Mumtaz. 2022. "Deep learning CNN-LSTM-MLP hybrid fusion model for feature optimizations and daily solar radiation prediction." Measurement. 202, pp. 1-22. https://doi.org/10.1016/j.measurement.2022.111759Basin management inspiration from impacts of alternating dry and wet conditions on water production and carbon uptake in Murray-Darling Basin
Lu, Zhixiang, Feng, Qi, Wei, Yongping, Zhao, Yan, Deo, Ravinesh C., Xie, Jiali, Zhou, Sha, Zhu, Meng and Xu, Min. 2022. "Basin management inspiration from impacts of alternating dry and wet conditions on water production and carbon uptake in Murray-Darling Basin." Science of the Total Environment. 851 (Part 2), pp. 1-8. https://doi.org/10.1016/j.scitotenv.2022.158359Introductory Engineering Mathematics Students’ Weighted Score Predictions Utilising a Novel Multivariate Adaptive Regression Spline Model
Ahmed, Abul Abrar Masrur, Deo, Ravinesh C., Ghimire, Sujan, Downs, Nathan J., Devi, Aruna, Barua, Prabal D. and Yaseen, Zaher M.. 2022. "Introductory Engineering Mathematics Students’ Weighted Score Predictions Utilising a Novel Multivariate Adaptive Regression Spline Model." Sustainability. 14 (17), pp. 1-27. https://doi.org/10.3390/su141711070Kernel Ridge Regression Hybrid Method for Wheat Yield Prediction with Satellite-Derived Predictors
Ahmed, A. A. Masrur, Sharma, Ekta, Jui, S. Janifer Jabin, Deo, Ravinesh C., Nguyen-Huy, Thong and Ali, Mumtaz. 2022. "Kernel Ridge Regression Hybrid Method for Wheat Yield Prediction with Satellite-Derived Predictors." Remote Sensing. 14 (5), pp. 1-24. https://doi.org/10.3390/rs14051136Cloud Affected Solar UV Predictions with Three-Phase Wavelet Hybrid Convolutional Long Short-Term Memory Network Multi-Step Forecast System
Prasad, Salvin S., Deo, Ravinesh C., Downs, Nathan, Igoe, Damien, Parisi, Alfio V. and Soar, Jeffrey. 2022. "Cloud Affected Solar UV Predictions with Three-Phase Wavelet Hybrid Convolutional Long Short-Term Memory Network Multi-Step Forecast System." IEEE Access. 10, pp. 24704-24720. https://doi.org/10.1109/ACCESS.2022.3153475Development and evaluation of hybrid deep learning long short-term memory network model for pan evaporation estimation trained with satellite and ground-based data
Jayasinghe, W. J. M. Lakmini Prarthana, Deo, Ravinesh C., Ghahramani, Afshin, Ghimire, Sujan and Raj, Nawin. 2022. "Development and evaluation of hybrid deep learning long short-term memory network model for pan evaporation estimation trained with satellite and ground-based data." Journal of Hydrology. 607, pp. 1-19. https://doi.org/10.1016/j.jhydrol.2022.127534Integrative artificial intelligence models for Australian coastal sediment lead prediction: An investigation of in-situ measurements and meteorological parameters effects
Bhagat, Suraj Kumar, Tiyasha, Tiyasha, Kumar, Adarsh, Malik, Tabarak, Jawad, Ali H., Khedher, Khaled Mohamed, Deo, Ravinesh C. and Yaseen, Zaher Mundher. 2022. "Integrative artificial intelligence models for Australian coastal sediment lead prediction: An investigation of in-situ measurements and meteorological parameters effects." Journal of Environmental Management. 309, pp. 1-16. https://doi.org/10.1016/j.jenvman.2022.114711An Eigenvalues-Based Covariance Matrix Bootstrap Model Integrated With Support Vector Machines for Multichannel EEG Signals Analysis
Al-Hadeethi, Hanan, Abdulla, Shahab, Diykh, Mohammed, Deo, Ravinesh C. and Green, Jonathan H.. 2022. "An Eigenvalues-Based Covariance Matrix Bootstrap Model Integrated With Support Vector Machines for Multichannel EEG Signals Analysis." Frontiers in Neuroinformatics. 15, pp. 1-15. https://doi.org/10.3389/fninf.2021.808339Stacked LSTM Sequence-to-Sequence Autoencoder with Feature Selection for Daily Solar Radiation Prediction: A Review and New Modeling Results
Ghimire, Sujan, Deo, Ravinesh C., Wang, Hua, Al-Musaylh, Mohanad S., Casillas-Perez, David and Salcedo-sanz, Sancho. 2022. "Stacked LSTM Sequence-to-Sequence Autoencoder with Feature Selection for Daily Solar Radiation Prediction: A Review and New Modeling Results." Energies. 15 (3), pp. 1-39. https://doi.org/10.3390/en15031061Texture analysis based graph approach for automatic detection of neonatal seizure from multi-channel EEG signals
Diykh, Mohammed, Miften, Firas Sabar, Abdulla, Shahab, Deo, Ravinesh C., Siuly, Siuly, Green, Jonathan H. and Oudah, Atheer Y.. 2022. "Texture analysis based graph approach for automatic detection of neonatal seizure from multi-channel EEG signals." Measurement. 190 (110731), pp. 1-13. https://doi.org/10.1016/j.measurement.2022.110731General equilibrium impact evaluation of food top-up induced by households’ renewable power self-supply in 141 regions
Nguyen, Duong Binh, Nong, Duy, Simshauser, Paul and Nguyen-Huy, Thong. 2022. "General equilibrium impact evaluation of food top-up induced by households’ renewable power self-supply in 141 regions." Applied Energy. 306 (Part B), pp. 1-13. https://doi.org/10.1016/j.apenergy.2021.118126Classification of catchments for nitrogen using Artificial Neural Network Pattern Recognition and spatial data
O'Sullivan, Cherie M., Ghahramani, Afshin, Deo, Ravinesh C., Pembleton, Keith, Khan, Urooj and Tuteja, Narendra. 2022. "Classification of catchments for nitrogen using Artificial Neural Network Pattern Recognition and spatial data." Science of the Total Environment. 809, pp. 1-15. https://doi.org/10.1016/j.scitotenv.2021.151139Copula-based statistical modelling of synoptic-scale climate indices for quantifying and managing agricultural risks in Australia
Nguyen-Huy, Thong. 2020. "Copula-based statistical modelling of synoptic-scale climate indices for quantifying and managing agricultural risks in Australia." Bulletin of the Australian Mathematical Society. 101 (1), pp. 166-169. https://doi.org/10.1017/S0004972719001217Drought Outlook Products Review
Cobon, David, Nguyen-Huy, Thong and Reardon-Smith, Kate. 2019. Drought Outlook Products Review. Toowoomba, Australia. University of Southern Queensland.Domino effect of climate change over two millennia in ancient China’s Hexi Corridor
Feng, Qi, Yan, Linshan, Deo, Ravinesh C., AghaKouchak, Amir, Adamowski, Jan F., Stone, Roger, Yin, Zhenliang, Liu, Wei, Si, Jianhua, Wen, Xiaohu, Zhu, Meng and Cao, Shixiong. 2019. "Domino effect of climate change over two millennia in ancient China’s Hexi Corridor." Nature Sustainability. 2, pp. 957-961. https://doi.org/10.1038/s41893-019-0397-9Bat algorithm for dam–reservoir operation
Ethteram, Mohammad, Mousavi, Sayed-Farhad, Karami, Hojat, Farzin, Saeed, Deo, Ravinesh, Othman, Faridah Binti, Chau, Kwok-Wing, Sarkamaryan, Saeed, Singh, Vijay P. and El-Shafie, Ahmed. 2018. "Bat algorithm for dam–reservoir operation." Environmental Earth Sciences. 77 (13), pp. 1-15. https://doi.org/10.1007/s12665-018-7662-5Characteristics of ecosystem water use efficiency in a desert riparian forest
Ma, Xiaohong, Feng, Qi, Su, Yonghong, Yu, Tengfei and Deo, Ravinesh C.. 2018. "Characteristics of ecosystem water use efficiency in a desert riparian forest." Environmental Earth Sciences. 77 (358). https://doi.org/10.1007/s12665-018-7518-zThe influence of climatic inputs on stream-flow pattern forecasting: case study of Upper Senegal River
Diop, Lamine, Bodian, Ansoumana, Djaman, Koffi, Yaseen, Zaher Mundher, Deo, Ravinesh C., El-Shafie, Ahmed and Brown, Larry C.. 2018. "The influence of climatic inputs on stream-flow pattern forecasting: case study of Upper Senegal River." Environmental Earth Sciences. 77 (5). https://doi.org/10.1007/s1266Uncertainty assessment of the multilayer perceptron (MLP) neural network model with implementation of the novel hybrid MLP-FFA method for prediction of biochemical oxygen demand and dissolved oxygen: a case study of Langat River
Raheli, Bahare, Aalami, Mohammad Taghi, El-Shafie, Ahmed, Ghorbani, Mohammad Ali and Deo, Ravinesh C.. 2017. "Uncertainty assessment of the multilayer perceptron (MLP) neural network model with implementation of the novel hybrid MLP-FFA method for prediction of biochemical oxygen demand and dissolved oxygen: a case study of Langat River." Environmental Earth Sciences. 76 (14). https://doi.org/10.1007/s12665-017-6842-zMethodology for producing the Drought Monitor
Pudmenzky, Christa, Guillory, Laura, Cobon, David H. and Nguyen-Huy, Thong. 2020. Methodology for producing the Drought Monitor. Toowoomba, Queensland. University of Southern Queensland.Northern Australia Climate Program: supporting adaptation in rangeland grazing systems through more targeted climate forecasts, improved drought information and an innovative extension program
Cobon, David, Jarvis, Chelsea, Reardon-Smith, Kate, Guillory, Laura, Pudmenzky, Christa, Nguyen-Huy, Thong, Mushtaq, Shahbaz and Stone, Roger. 2021. "Northern Australia Climate Program: supporting adaptation in rangeland grazing systems through more targeted climate forecasts, improved drought information and an innovative extension program." The Rangeland Journal. 43 (3), pp. 87-100. https://doi.org/10.1071/RJ20074Novel hybrid deep learning model for satellite based PM10 forecasting in the most polluted Australian hotspots
Sharma, Ekta, Deo, Ravinesh C., Soar, Jeffrey, Prasad, Ramendra, Parisi, Alfio V. and Raj, Nawin. 2022. "Novel hybrid deep learning model for satellite based PM10 forecasting in the most polluted Australian hotspots." Atmospheric Environment. 279, pp. 1-13. https://doi.org/10.1016/j.atmosenv.2022.119111New double decomposition deep learning methods for river water level forecasting
Ahmed, A. A. Masrur, Deo, Ravinesh C., Ghahramani, Afshin, Feng, Qi, Raj, Nawin, Yin, Zhenliang and Yang, Linshan. 2022. "New double decomposition deep learning methods for river water level forecasting." Science of the Total Environment. 831, pp. 1-21. https://doi.org/10.1016/j.scitotenv.2022.154722Global disparities in agricultural climate index-based insurance research
Adeyinka, Adewuyi Ayodele, Kath, Jarrod, Nguyen-Huy, Thong, Mushtaq, Shahbaz, Souvignet, Maxime, Range, Matthias and Barratt, Jonathan. 2022. "Global disparities in agricultural climate index-based insurance research." Climate Risk Management. 35, pp. 1-15. https://doi.org/10.1016/j.crm.2022.100394Explainable diabetes classification using hybrid Bayesian-optimized TabNet architecture
Joseph, Lionel P., Joseph, Erica A. and Prasad, Ramendra. 2022. "Explainable diabetes classification using hybrid Bayesian-optimized TabNet architecture." Computers in Biology and Medicine. 151 (Part A). https://doi.org/10.1016/j.compbiomed.2022.106178Drought outlook validation using ACCESS-S2 hindcast
Nguyen-Huy, Thong and Cobon, David. 2022. Drought outlook validation using ACCESS-S2 hindcast. Northern Australia Climate Program (NACP).Systemic risk management in farming - geographic distribution and diversity
Nguyen-Huy, Thong. 2022. "Systemic risk management in farming - geographic distribution and diversity." Future Drought Fund’s 2022 Science to Practice Forum. 07 - 08 Jun 2022 Australia.A hierarchical classification/regression algorithm for improving extreme wind speed events prediction
Pelaez-Rodriguez, C., Perez-Aracil, J., Fister, D, Prieto-Godino, L., Deo, R.C. and Salcedo-sanz, S.. 2022. "A hierarchical classification/regression algorithm for improving extreme wind speed events prediction." Renewable Energy. 201 (Part 2), pp. 157-178. https://doi.org/10.1016/j.renene.2022.11.042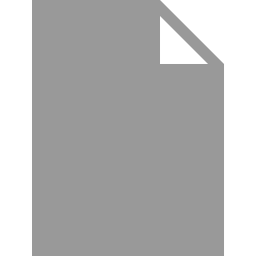