Forecasting River Water Temperature Using Explainable Artificial Intelligence and Hybrid Machine Learning: Case Studies in Menindee Region in Australia
Article
Briceno Medina (Student), L., Joehnk, K., Deo, R., Ali, M., Prasad, S.S. and Downs, N.. 2024. "Forecasting River Water Temperature Using Explainable Artificial Intelligence and Hybrid Machine Learning: Case Studies in Menindee Region in Australia." Water. 16 (24). https://doi.org/https://doi.org/10.3390/w16243720
Article Title | Forecasting River Water Temperature Using Explainable Artificial Intelligence and Hybrid Machine Learning: Case Studies in Menindee Region in Australia |
---|---|
Article Category | Article |
Authors | Briceno Medina (Student), L., Joehnk, K., Deo, R., Ali, M., Prasad, S.S. and Downs, N. |
Journal Title | Water |
Journal Citation | 16 (24) |
Year | 2024 |
Publisher | MDPI AG |
ISSN | 2073-4441 |
Digital Object Identifier (DOI) | https://doi.org/https://doi.org/10.3390/w16243720 |
Web Address (URL) | https://www.mdpi.com/2073-4441/16/24/3720 |
Contains Sensitive Content | Does not contain sensitive content |
ANZSRC Field of Research 2020 | 460104. Applications in physical sciences |
Permalink -
https://research.usq.edu.au/item/zqxqz/forecasting-river-water-temperature-using-explainable-artificial-intelligence-and-hybrid-machine-learning-case-studies-in-menindee-region-in-australia
1
total views0
total downloads1
views this month0
downloads this month
Export as
Related outputs
An Explainable Predictive Approach for Investigation of Greenhouse Gas Emissions in Maritime Canada's Potato Agriculture
Jamei, Mehdi, Yaqoob, Nauman, Farooque, Aitazaz A., Ali, Mumtaz, Malik, Anurag, Esau, Travis J. and Hu, Yulin. 2025. "An Explainable Predictive Approach for Investigation of Greenhouse Gas Emissions in Maritime Canada's Potato Agriculture." Smart Agricultural Technology . 10. https://doi.org/10.1016/j.atech.2024.100709Explainable deeply-fused nets electricity demand prediction model: Factoring climate predictors for accuracy and deeper insights with probabilistic confidence interval and point-based forecasts
Ghimire, Sujan, AL-Musaylh, Mohanad S., Nguyen-Huy, Thong, Deo, Ravinesh C., Acharya, Rajendra, Casillas-Perez, David, Yaseen, Zaher Mundher and Salcedo-sanz, Sancho. 2025. "Explainable deeply-fused nets electricity demand prediction model: Factoring climate predictors for accuracy and deeper insights with probabilistic confidence interval and point-based forecasts." Applied Energy. 378 (Part A). https://doi.org/10.1016/j.apenergy.2024.124763Tree shade within urban parks and nature strips: standards and perspectives from measurement campaigns conducted in Queensland
Downs, Nathan, Antrobus, Jodie, Dekeyser, Stijn, Butler, Harry, Downs, Melanie, Hickmott, Darren, Madigan, Chris, Brown, Alexandra, Amar, Abdurazaq, Dearnaley, John, Parisi, Alfio V, Vanos, Jennifer, Raj, Nawin, Harrison, Simone and Igoe, Damien. 2024. "Tree shade within urban parks and nature strips: standards and perspectives from measurement campaigns conducted in Queensland ." 6th International conference UV and Skin Cancer Prevention. Brisbane, Australia 11 - 15 Sep 2024 Australia.A designed predictive modelling strategy based on data decomposition and machine learning to forecast solar radiation
Ali, Mumtaz, Prasad, Ramendra, Alamery, Salman and Farooque, Aitazaz Ahsan. 2024. "A designed predictive modelling strategy based on data decomposition and machine learning to forecast solar radiation." Results in Engineering. 24. https://doi.org/10.1016/j.rineng.2024.103607Explainable artificial intelligence-machine learning models to estimate overall scores in tertiary preparatory general science course
Ghimire, Sujan, Abdulla, Shahab, Joseph, Lionel P., Prasad, Salvin, Murphy, Angela, Devi, Aruna, Barua, Prabal Datta, Deo, Ravinesh C., Acharya, Rajendra and Yaseen, Zaher Mundher. 2024. "Explainable artificial intelligence-machine learning models to estimate overall scores in tertiary preparatory general science course." Computers and Education: Artificial Intelligence. 7. https://doi.org/10.1016/j.caeai.2024.100331Forecasting Multi-Step Soil Moisture with Three-Phase Hybrid Wavelet-Least Absolute Shrinkage Selection Operator-Long Short-Term Memory Network (moDWT-Lasso-LSTM) Model
Jayasinghe, W. J. M. Lakmini Prarthana, Deo, Ravinesh C., Raj, Nawin, Ghimire, Sujan, Yaseen, Zaher Mundher, Nguyen-Huy, Thong and Ghahramani, Afshin. 2024. "Forecasting Multi-Step Soil Moisture with Three-Phase Hybrid Wavelet-Least Absolute Shrinkage Selection Operator-Long Short-Term Memory Network (moDWT-Lasso-LSTM) Model." Water. 16 (21), p. 3133. https://doi.org/10.3390/w16213133Monitoring of Greenhouse Gas Emission Drivers in Atlantic Canadian Potato Production: A Robust Explainable Intelligent Glass-Box
Jamei, Mehdi, Hassan, Muhammad, Farooque, Aitazaz A., Ali, Mumtaz, Karbasi, Masoud, Randhawa, Gurjit, Yaseen, Zaher Mundher and Dwyer, Ross. 2024. "Monitoring of Greenhouse Gas Emission Drivers in Atlantic Canadian Potato Production: A Robust Explainable Intelligent Glass-Box." Results in Engineering. 24. https://doi.org/10.1016/j.rineng.2024.103297Innovative Multi-Temporal Evapotranspiration Forecasting Using Empirical Fourier Decomposition and Bidirectional Long Short-Term Memory
Karbasi, Masoud, Ali, Mumtaz, Randhawa, Gurjit S., Jamei, Mehdi, Malik, Anurag, Hussain, Syed Hamid Hussain, Bos, Melanie, Zaman, Qamar and Farooque, Aitazaz Ahsan. 2024. "Innovative Multi-Temporal Evapotranspiration Forecasting Using Empirical Fourier Decomposition and Bidirectional Long Short-Term Memory." Smart Agricultural Technology . 9. https://doi.org/10.1016/j.atech.2024.100619Ultraviolet radiation thin film dosimetry: A review of properties and applications
Parisi, Alfio V., Downs, Nathan J., Schouten, Peter, Igoe, Damien P., Turner, Joanna, Amar, Abdurazaq, Wainwright, Lisa, Dawes, Adrian, Butler, Harry and Dekeyser, Stijn. 2024. "Ultraviolet radiation thin film dosimetry: A review of properties and applications." Photochemistry and Photobiology. https://doi.org/10.1111/php.14022Artificial Intelligence-Empowered Doppler Weather Profile for Low-Earth-Orbit Satellites
Sharma, Ekta, Deo, Ravinesh C., Davey, Christopher P and Carter, Brad D.. 2024. "Artificial Intelligence-Empowered Doppler Weather Profile for Low-Earth-Orbit Satellites." Sensors. 24 (16). https://doi.org/10.3390/s24165271Multi-step ahead forecasting of electrical conductivity in rivers by using a hybrid Convolutional Neural Network-Long Short-Term Memory (CNN-LSTM) model enhanced by Boruta-XGBoost feature selection algorithm
Karbasi, Masoud, Ali, Mumtaz, Bateni, Sayed M., Jun, Changhyun, Jamei, Mehdi, Farooque, Aitazaz Ahsan and Yaseen, Zaher Mundher. 2024. "Multi-step ahead forecasting of electrical conductivity in rivers by using a hybrid Convolutional Neural Network-Long Short-Term Memory (CNN-LSTM) model enhanced by Boruta-XGBoost feature selection algorithm." Scientific Reports. 14 (1). https://doi.org/10.1038/s41598-024-69309-3Wavelet-fusion image super-resolution model with deep learning for downscaling remotely-sensed, multi-band spectral albedo imagery
Karalasingham, Sagthitharan, Deo, Ravinesh C., Casillas-Perez, David, Raj, Nawin and Salcedo-sanz, Sancho. 2024. "Wavelet-fusion image super-resolution model with deep learning for downscaling remotely-sensed, multi-band spectral albedo imagery." Remote Sensing Applications: Society and Environment. 36. https://doi.org/10.1016/j.rsase.2024.101333Half-hourly electricity price prediction with a hybrid convolution neural network-random vector functional link deep learning approach
Ghimire, Sujan, Deo, Ravinesh C., Casillas-Perez, David, Sharma, Ekta, Salcedo-sanz, Sancho, Barua, Prabal and Acharya, U. Rajendra. 2024. "Half-hourly electricity price prediction with a hybrid convolution neural network-random vector functional link deep learning approach." Applied Energy. 374. https://doi.org/10.1016/j.apenergy.2024.123920Towards next-generation federated learning: A case study on privacy attacks in artificial intelligence systems
Sharma, Ekta, Deo, Ravinesh C, Davey, Christopher P., Carter, Brad D. and Salcedo-sanz, Sancho. 2024. "Towards next-generation federated learning: A case study on privacy attacks in artificial intelligence systems." 2024 IEEE Conference on Artificial Intelligence (CAI 2024). Singapore 25 - 27 Jun 2024 United States. IEEE (Institute of Electrical and Electronics Engineers). https://doi.org/10.1109/CAI59869.2024.00259Poster: Cloud Computing with AI-empowered Trends in Software-Defined Radios: Challenges and Opportunities
Sharma, Ekta, Deo, Ravinesh C., Davey, Christopher P., Carter, Brad D. and Salcedo-sanz, Sancho. 2024. "Poster: Cloud Computing with AI-empowered Trends in Software-Defined Radios: Challenges and Opportunities." 2024 IEEE 25th International Symposium on a World of Wireless, Mobile and Multimedia Networks (WoWMoM 2024). Perth, Australia 04 - 07 Jun 2024 United States. IEEE (Institute of Electrical and Electronics Engineers). https://doi.org/10.1109/WoWMoM60985.2024.00054Near real-time significant wave height prediction along the coastline of Queensland using advanced hybrid machine learning models
Khosravi, K., Ali, M. and Heddam, S.. 2024. "Near real-time significant wave height prediction along the coastline of Queensland using advanced hybrid machine learning models." International Journal of Environmental Science and Technology. https://doi.org/10.1007/s13762-024-05944-7Prediction of Sea Level Using Double Data Decomposition and Hybrid Deep Learning Model for Northern Territory, Australia
Raj, Nawin, Murali, Jaishukh, Singh-Peterson, Lila and Downs, Nathan. 2024. "Prediction of Sea Level Using Double Data Decomposition and Hybrid Deep Learning Model for Northern Territory, Australia." Mathematics. 12 (15). https://doi.org/10.3390/math12152376Robust drought forecasting in Eastern Canada: Leveraging EMD-TVF and ensemble deep RVFL for SPEI index forecasting
Karbasi, Masoud, Ali, Mumtaz, Farooque, Aitazaz Ahsan, Jamei, Mehdi, Khosravi, Khabat, Cheema, Saad Javed and Yaseen, Zaher Mundher. 2024. "Robust drought forecasting in Eastern Canada: Leveraging EMD-TVF and ensemble deep RVFL for SPEI index forecasting." Expert Systems with Applications. 256. https://doi.org/10.1016/j.eswa.2024.124900Atmospheric Visibility and Cloud Ceiling Predictions With Hybrid IIS-LSTM Integrated Model: Case Studies for Fiji's Aviation Industry
Raj, Shiveel, Deo, Ravinesh C., Sharma, Ekta, Prasad, Ramendra, Dinh, Toan and Salcedo-sanz, Sancho. 2024. "Atmospheric Visibility and Cloud Ceiling Predictions With Hybrid IIS-LSTM Integrated Model: Case Studies for Fiji's Aviation Industry." IEEE Access. 12, pp. 72530-72543. https://doi.org/10.1109/ACCESS.2024.3401091Point-based and probabilistic electricity demand prediction with a Neural Facebook Prophet and Kernel Density Estimation model
Ghimire, Sujan, Deo, Ravinesh C., Pourmousavi, S. Ali, Casillas-Perez, David and Salcedo-sanz, Sancho. 2024. "Point-based and probabilistic electricity demand prediction with a Neural Facebook Prophet and Kernel Density Estimation model." Engineering Applications of Artificial Intelligence. 135. https://doi.org/10.1016/j.engappai.2024.108702End-to-end learning of adaptive coded modulation schemes for resilient wireless communications
Davey, Christopher P., Shakeel, Ismail, Deo, Ravinesh C., Sharma, Ekta, Salcedo-sanz, Sancho and Soar, Jeffrey. 2024. "End-to-end learning of adaptive coded modulation schemes for resilient wireless communications." Applied Soft Computing. 159. https://doi.org/10.1016/j.asoc.2024.111672Deep Learning Based Over-the-Air Training of Wireless Communication Systems without Feedback
Davey, Christopher P., Shakeel, Ismail, Deo, Ravinesh C. and Salcedo-sanz, Sancho. 2024. "Deep Learning Based Over-the-Air Training of Wireless Communication Systems without Feedback." Sensors. 24 (10). https://doi.org/10.3390/s24102993Multi-step ahead forecasting of electrical conductivity in rivers by using a hybrid Convolutional Neural Network-Long Short-Term Memory (CNN-LSTM) model enhanced by Boruta-XGBoost feature selection algorithm
Karbasi, Masoud, Ali, Mumtaz, Bateni, Sayed M., Jun, Changhyun, Jamei, Mehdi, Farooque, Aitazaz Ahsan and Yaseen, Zaher Mundher. 2024. "Multi-step ahead forecasting of electrical conductivity in rivers by using a hybrid Convolutional Neural Network-Long Short-Term Memory (CNN-LSTM) model enhanced by Boruta-XGBoost feature selection algorithm." Scientific Reports. 14. https://doi.org/https://doi.org/10.1038/s41598-024-65837-0A playground shade index for standardising ultraviolet protection assessments of open, mixed and protected outdoor recreational spaces
Downs, Nathan, Butler, Harry, Dexter, Benjamin, Raj, Nawin, Downs, Melanie, Turner, Joanna, Dekeyser, Stijn, Deo, Ravinesh, Vanos, Jennifer, Igoe, Damien and Parisi, Alfio V. 2024. "A playground shade index for standardising ultraviolet protection assessments of open, mixed and protected outdoor recreational spaces." 6th International conference UV and Skin Cancer Prevention. Brisbane, Australia 11 - 15 Sep 2024 Australia.The mitigating effect of street trees, urban flora, and the suburban environment on seasonal peak UV indices: A case study from Brisbane, Australia
Downs, Nathan James, Amar, Abdurazaq, Dearnaley, John, Butler, Harry, Dekeyser, Stijn, Igoe, Damien, Parisi, Alfio V., Raj, Nawin, Deo, Ravinesh and Turner, Joanna. 2024. "The mitigating effect of street trees, urban flora, and the suburban environment on seasonal peak UV indices: A case study from Brisbane, Australia." Photochemistry and Photobiology. https://doi.org/10.1111/php.13988UV albedo and reflectance: is it possible to predict erythemal reflectance from solar visible reflectance with respect to the type of material?
Turner, Joanna, Parisi, Alfio V, Amar, Abdurazaq and Downs, Nathan. 2024. "UV albedo and reflectance: is it possible to predict erythemal reflectance from solar visible reflectance with respect to the type of material?" 6th International conference UV and Skin Cancer Prevention. Brisbane, Australia 11 - 15 Sep 2024 Australia.A legacy of more than two decades of UV radiation measurement studies at the University of Southern Queensland
Amar, Abdurazaq, Parisi, Alfio, Downs, Nathan, Turner, Joanna, Igoe, Damien, Butler, Harry and Dekeyser, Stijn. 2024. "A legacy of more than two decades of UV radiation measurement studies at the University of Southern Queensland." 6th International conference UV and Skin Cancer Prevention. Brisbane, Australia 11 - 15 Sep 2024 Australia.Multi-Step-Ahead Wind Speed Forecast System: Hybrid Multivariate Decomposition and Feature Selection-Based Gated Additive Tree Ensemble Model
Joseph, Lionel P., Deo, Ravinesh C., Casillas-Perez, David, Prasad, Ramendra, Raj, Nawin and Salcedo-sanz, Sancho. 2024. "Multi-Step-Ahead Wind Speed Forecast System: Hybrid Multivariate Decomposition and Feature Selection-Based Gated Additive Tree Ensemble Model." IEEE Access. 12, pp. 58750-58777. https://doi.org/10.1109/ACCESS.2024.3392899Enhancing groundwater level prediction accuracy using interpolation techniques in deep learning models
Abdi, Erfan, Ali, Mumtaz, Guimarães Santos, Celso Augusto, Olusola, Adeyemi and Ghorbani, Mohammad Ali. 2024. "Enhancing groundwater level prediction accuracy using interpolation techniques in deep learning models." Groundwater for Sustainable Development. 26. https://doi.org/10.1016/j.gsd.2024.101213Deep Learning-Assisted Sensitive 3C-SiC Sensor for Long-Term Monitoring of Physical Respiration
Tran, Thi Lap, Nguyen, Duy Van, Nguyen, Hung, Nguyen, Thi Phuoc Van, Song, Pingan, Deo, Ravinesh C, Moloney, Clint, Dao, Viet Dung, Nguyen, Nam-Trung and Dinh, Toan. 2024. "Deep Learning-Assisted Sensitive 3C-SiC Sensor for Long-Term Monitoring of Physical Respiration." Advanced Sensor Research. 3 (8). https://doi.org/10.1002/adsr.202300159Copula-Probabilistic Flood Risk Analysis with an Hourly Flood Monitoring Index
Chand, Ravinesh, Nguyen-Huy, Thong, Deo, Ravinesh C., Ghimire, Sujan, Ali, Mumtaz and Ghahramani, Afshin. 2024. "Copula-Probabilistic Flood Risk Analysis with an Hourly Flood Monitoring Index." Water. 16 (11). https://doi.org/10.3390/w16111560Electronic sun journal as an evaluation tool for measuring sunflecks while walking through urban forests
Igoe, Damien P., Parisi, Alfio V, Amar, Abdurazaq, Downs, Nathan J. and Butler, Harry. 2024. "Electronic sun journal as an evaluation tool for measuring sunflecks while walking through urban forests." Instrumentation Science and Technology. https://doi.org/10.1080/10739149.2024.2350009Empirical curvelet transform based deep DenseNet model to predict NDVI using RGB drone imagery data
Diykh, Mohammed, Ali, Mumtaz, Jamei, Mehdi, Abdulla, Shahab, Uddin, Md Palash, Farooque, Aitazaz Ahsan, Labban, Abdulhaleem H. and Alabdally, Hussein. 2024. "Empirical curvelet transform based deep DenseNet model to predict NDVI using RGB drone imagery data." Computers and Electronics in Agriculture. 221. https://doi.org/10.1016/j.compag.2024.108964Short-term wind speed forecasting using an optimized three-phase convolutional neural network fused with bidirectional long short-term memory network model
Joseph, Lionel P., Deo, Ravinesh C., Casillas-Perez, David, Prasad, Ramendra, Raj, Nawin and Salcedo-sanz, Sancho. 2024. "Short-term wind speed forecasting using an optimized three-phase convolutional neural network fused with bidirectional long short-term memory network model." Applied Energy. 359. https://doi.org/10.1016/j.apenergy.2024.122624Probabilistic-based electricity demand forecasting with hybrid convolutional neural network-extreme learning machine model
Ghimire, Sujan, Deo, Ravinesh C., Casillas-Perez, David, Salcedo-sanz, Sancho, Pourmousavi, S. Ali and Acharya, U. Rajendra. 2024. "Probabilistic-based electricity demand forecasting with hybrid convolutional neural network-extreme learning machine model." Engineering Applications of Artificial Intelligence. 132. https://doi.org/10.1016/j.engappai.2024.107918Electricity demand error corrections with attention bi-directional neural networks
Ghimire, Sujan, Deo, Ravinesh C., Casillas-Perez, David and Salcedo-sanz, Sancho. 2024. "Electricity demand error corrections with attention bi-directional neural networks." Energy. 291. https://doi.org/10.1016/j.energy.2023.129938Micromachined Mechanical Resonant Sensors: From Materials, Structural Designs to Applications
Dinh, Toan, Rais-Zadeh, Mina, Nguyen, Thanh, Phan, Hoang-Phuong, Song, Pingan, Deo, Ravinesh, Dao, Dzung, Nguyen, Nam-Trung and Bell, John. 2024. "Micromachined Mechanical Resonant Sensors: From Materials, Structural Designs to Applications." Advanced Materials Technologies. 9 (2). https://doi.org/10.1002/admt.202300913Machine learning for expediting next-generation of fire-retardant polymer composites
Jafari, Pooya, Zhang, Ruoran, Huo, Siqi, Wang, Qingsheng, Yong, Jianming, Hong, Min, Deo, Ravinesh, Wang, Hao and Song, Pingan. 2024. "Machine learning for expediting next-generation of fire-retardant polymer composites." Composites Communications. 45. https://doi.org/10.1016/j.coco.2023.101806Quantitative improvement of streamflow forecasting accuracy in the Atlantic zones of Canada based on hydro-meteorological signals: A multi-level advanced intelligent expert framework
Jamei, Mozhdeh, Jamei, Mehdi, Ali, Mozhdeh, Karbasi, Masoud, Farooque, Aitazaz A., Malik, Anurag, Cheema, Saad Javed, Esau, Travis J. and Yaseen, Zaher Mundher. 2024. "Quantitative improvement of streamflow forecasting accuracy in the Atlantic zones of Canada based on hydro-meteorological signals: A multi-level advanced intelligent expert framework." Ecological Informatics. 80. https://doi.org/10.1016/j.ecoinf.2023.102455Short-term drought Index forecasting for hot and semi-humid climate Regions: A novel empirical Fourier decomposition-based ensemble Deep-Random vector functional link strategy
Jamei, Mehdi, Ali, Mumtaz, Bateni, Sayed M., Jun, Changhyun, Karbasi, Masoud, Malik, Anurag, Jamei, Mozhdeh and Yaseen, Zaher Mundher. 2024. "Short-term drought Index forecasting for hot and semi-humid climate Regions: A novel empirical Fourier decomposition-based ensemble Deep-Random vector functional link strategy ." Computers and Electronics in Agriculture. 217. https://doi.org/10.1016/j.compag.2023.108609Very short-term solar ultraviolet-A radiation forecasting system with cloud cover images and a Bayesian optimized interpretable artificial intelligence model
Prasad, Salvin Sanjesh, Deo, Ravinesh Chand, Downs, Nathan James, Casillas-Perez, David, Salcedo-sanz, Sancho and Parisi, Alfio Venerando. 2024. "Very short-term solar ultraviolet-A radiation forecasting system with cloud cover images and a Bayesian optimized interpretable artificial intelligence model ." Expert Systems with Applications. 236. https://doi.org/10.1016/j.eswa.2023.121273Short-term wave power forecasting with hybrid multivariate variational mode decomposition model integrated with cascaded feedforward neural networks
Ali, Mumtaz, Prasad, Ramendra, Jamei, Mehdi, Malik, Anurag, Xiang, Yong, Abdulla, Shahab, Deo, Ravinesh C., Farooque, Aitazaz A. and Labban, Abdulhaleem H.. 2024. "Short-term wave power forecasting with hybrid multivariate variational mode decomposition model integrated with cascaded feedforward neural networks." Renewable Energy. 221. https://doi.org/10.1016/j.renene.2023.119773Accurate monitoring of micronutrients in tilled potato soils of eastern Canada: Application of an eXplainable inspired-adaptive boosting framework coupled with SelectKbest
Jamei, Mehdi, Ali, Mumtaz, Afzaal, Hassan, Karbasi, Masoud, Malik, Anurag, Farooque, Aitazaz Ahsan, Haydar, Zeeshan and Zaman, Qamar Uz. 2024. "Accurate monitoring of micronutrients in tilled potato soils of eastern Canada: Application of an eXplainable inspired-adaptive boosting framework coupled with SelectKbest." Computers and Electronics in Agriculture. 216. https://doi.org/10.1016/j.compag.2023.108479Application of an explainable glass-box machine learning approach for prognostic analysis of a biogas-powered small agriculture engine
Jamei, Mehdi, Sharma, Prabhakar, Ali, Mumtaz, Bora, Bhaskor J., Malik, Anurag, Paramasivam, Prabhu, Farooque, Aitazaz A. and Abdulla, Shahab. 2024. "Application of an explainable glass-box machine learning approach for prognostic analysis of a biogas-powered small agriculture engine." Energy. 288. https://doi.org/https://doi.org/10.1016/j.energy.2023.129862Boruta extra tree-bidirectional long short-term memory model development for Pan evaporation forecasting: Investigation of arid climate condition
Karbasi, Masoud, Ali, Mumtaz, Bateni, Sayed M., Jun, Changhyun, Jamei, Mehdi and Yaseen, Zaher Mundher. 2024. "Boruta extra tree-bidirectional long short-term memory model development for Pan evaporation forecasting: Investigation of arid climate condition." Alexandria Engineering Journal. 86, pp. 425-442. https://doi.org/10.1016/j.aej.2023.11.061Hybridized artificial intelligence models with nature-inspired algorithms for river flow modeling: A comprehensive review, assessment, and possible future research directions
Tao, Hai, Abba, Sani I., Al-Areeq, Ahmed M., Tangang, Fredolin, Samantaray, Sandeep, Sahoo, Abinash, Siqueira, Hugo Valadares, Maroufpoor, Saman, Demir, Vahdettin, Bokde, Neeraj Dhanraj, Goliatt, Leonardo, Jamei, Mehdi, Ahmadianfar, Iman, Bhagat, Suraj Kumar, Halder, Bijay, Guo, Tianli, Helman, Daniel S., Ali, Mumtaz, Sattar, Sabaa, ..., Yaseen, Zaher Mundher. 2024. "Hybridized artificial intelligence models with nature-inspired algorithms for river flow modeling: A comprehensive review, assessment, and possible future research directions ." Engineering Applications of Artificial Intelligence. 129. https://doi.org/10.1016/j.engappai.2023.107559Two-step deep learning framework with error compensation technique for short-term, half-hourly electricity price forecasting
Ghimire, Sujan, Deo, Ravinesh C., Casillas-Perez, David and Salcedo-sanz, Sancho. 2024. "Two-step deep learning framework with error compensation technique for short-term, half-hourly electricity price forecasting." Applied Energy. 353 (Part A). https://doi.org/10.1016/j.apenergy.2023.122059Adaptive Regularization and Resilient Estimation in Federated Learning
Uddin, Md Palash, Xiang, Yong, Zhao, Yao, Ali, Mumtaz, Zhang, Yushu and Gao, Longxiang. 2024. "Adaptive Regularization and Resilient Estimation in Federated Learning." IEEE Transactions on Services Computing. 17 (4), pp. 1369-1381. https://doi.org/10.1109/TSC.2023.3332703Hybrid Deep Learning Model for Wave Height Prediction in Australia's Wave Energy Region
Ahmed, Abul Abrar Masrur, Jui, S Janifer Jabin, AL-Musaylh, Mohanad S., Raj, Nawin, Saha, Reepa, Deo, Ravinesh C and Saha, Sanjoy Kumar. 2024. "Hybrid Deep Learning Model for Wave Height Prediction in Australia's Wave Energy Region." Applied Soft Computing. 150. https://doi.org/10.1016/j.asoc.2023.111003Monthly sodium adsorption ratio forecasting in rivers using a dual interpretable glass-box complementary intelligent system: Hybridization of ensemble TVF-EMD-VMD, Boruta-SHAP, and eXplainable GPR
Jamei, Mehdi, Ali, Mumtaz, Karbasi, Masoud, Karimi, Bakhtiar, Jahannemaei, Neshat, Farooque, Aitazaz Ahsan and Yaseen, Zaher Mundher. 2024. "Monthly sodium adsorption ratio forecasting in rivers using a dual interpretable glass-box complementary intelligent system: Hybridization of ensemble TVF-EMD-VMD, Boruta-SHAP, and eXplainable GPR." Expert Systems with Applications. 237 (Part B). https://doi.org/10.1016/j.eswa.2023.121512Evaluation and Prediction of Groundwater Quality for Irrigation Using an Integrated Water Quality Indices, Machine Learning Models and GIS Approaches: A Representative Case Study
Ibrahim, Hekmat, Yaseen, Zaher Mundher, Scholz, Miklas, Ali, Mumtaz, Gad, Mohamed, Elsayed, Salah, Khadr, Mosaad, Hussein, Hend, Ibrahim, Hazem H., Eid, Mohamed Hamdy, Kovács, Attila, Péter, Szűcs and Khalifa, Moataz M.. 2023. "Evaluation and Prediction of Groundwater Quality for Irrigation Using an Integrated Water Quality Indices, Machine Learning Models and GIS Approaches: A Representative Case Study." Water. 15 (4), pp. 1-26. https://doi.org/https://doi.org/10.3390/w15040694Channel-Agnostic Training of Transmitter and Receiver for Wireless Communications
Davey, Christopher P., Shakeel, Ismail, Deo, Ravinesh C. and Salcedo-sanz, Sancho. 2023. "Channel-Agnostic Training of Transmitter and Receiver for Wireless Communications ." Sensors. 23 (24). https://doi.org/10.3390/s23249848Optimizing the Seasonal Shade and Ultraviolet Protection of a Suburban Playground by Utilization of the Playground Shade Index
Downs, Nathan, Butler, Harry, Parisi, Alfio, Beckman-Downs, Melanie and Igoe, Damien. 2023. "Optimizing the Seasonal Shade and Ultraviolet Protection of a Suburban Playground by Utilization of the Playground Shade Index." Photochemistry and Photobiology. 99 (6), pp. 1483-1492. https://doi.org/10.1111/php.13792Deep Image Analysis for Microalgae Identification
Soar, Jeffrey, Lih, Oh Shu, Wen, Loh Hui, Ward, Aleth, Sharma, Ekta, Deo, Ravinesh C., Barua, Prabal Datta, Tan, Ru-San, Rinen, Eliezer and Acharya, Rajendra. 2023. "Deep Image Analysis for Microalgae Identification." Lecture notes in computer science. Switzerland . Springer. pp. 280-292Solar ultraviolet radiation predictions under cloud cover effects with artificial intelligence approaches
Prasad, Salvin Sanjesh. 2023. Solar ultraviolet radiation predictions under cloud cover effects with artificial intelligence approaches. PhD by Publication Doctor of Philosophy. University of Southern Queensland. https://doi.org/10.26192/z3qq0Development of an enhanced bidirectional recurrent neural network combined with time-varying filter-based empirical mode decomposition to forecast weekly reference evapotranspiration
Karbasi, Masoud, Jamei, Mehdi, Ali, Mumtaz, Malik, Anurag, Chu, Xuefeng, Farooque, Aitazaz Ahsan and Yaseen, Zaher Mundher. 2023. "Development of an enhanced bidirectional recurrent neural network combined with time-varying filter-based empirical mode decomposition to forecast weekly reference evapotranspiration." Agricultural Water Management. 290. https://doi.org/https://doi.org/10.1016/j.agwat.2023.108604An ensemble machine learning-based intelligent system for human activity recognition using sensory data
Abdulla, Shahab, Diykh, Mohammed, Siuly, Siuly and Ali, Mumtaz. 2023. "An ensemble machine learning-based intelligent system for human activity recognition using sensory data." Sinha, G.R., Subudhi, Bidyadhar, Fan, Chih-Peng and Nisar, Humaira (ed.) Cognitive Sensing Technologies and Applications. Institution of Engineering and Technology (IET). pp. 119-130Statistical and spatial analysis for soil heavy metals over the Murray-Darling river basin in Australia
Tao, Hai, Al-Hilali, Aqeel Ali, Ahmed, Ali M., Mussa, Zainab Haider, Falah, Mayadah W., Abed, Salwan Ali, Deo, Ravinesh, Jawad, Ali H., Maulud, Khairul Nizam Abdul, Latif, Mohd Talib and Yaseen, Zaher Mundher. 2023. "Statistical and spatial analysis for soil heavy metals over the Murray-Darling river basin in Australia." Chemosphere. 317. https://doi.org/10.1016/j.chemosphere.2023.137914Enhanced joint hybrid deep neural network explainable artificial intelligence model for 1-hr ahead solar ultraviolet index prediction
Prasad, Salvin S., Deo, Ravinesh C., Salcedo-sanz, Sancho, Downs, Nathan J., Casillas-Perez, David and Parisi, Alfio V.. 2023. "Enhanced joint hybrid deep neural network explainable artificial intelligence model for 1-hr ahead solar ultraviolet index prediction." Computer Methods and Programs in Biomedicine. 241. https://doi.org/10.1016/j.cmpb.2023.107737Ampelomyces mycoparasites of powdery mildews–a review
Prahl, Rosa E., Khan, Shahjahan and Deo, Ravinesh C.. 2023. "Ampelomyces mycoparasites of powdery mildews–a review." Canadian Journal of Plant Pathology. 45 (4), pp. 391-404. https://doi.org/10.1080/07060661.2023.2206378A fuzzy-based cascade ensemble model for improving extreme wind speeds prediction
Pelaez-Rodriguez, C., Perez-Aracil, J., Prieto-Godino, L., Ghimire, S., Deo, R. and Salcedo-sanz, S.. 2023. "A fuzzy-based cascade ensemble model for improving extreme wind speeds prediction." Journal of Wind Engineering and Industrial Aerodynamics. 240. https://doi.org/10.1016/j.jweia.2023.105507Explainable AI approach with original vegetation data classifies spatio-temporal nitrogen in flows from ungauged catchments to the Great Barrier Reef
O’Sullivan, Cherie M., Deo, Ravinesh C. and Ghahramani, Afshin. 2023. "Explainable AI approach with original vegetation data classifies spatio-temporal nitrogen in flows from ungauged catchments to the Great Barrier Reef." Scientific Reports. 13 (1). https://doi.org/10.1038/s41598-023-45259-0A Novel Attention-Based Model for Semantic Segmentation of Prostate Glands Using Histopathological Images
Inamdar, Mahesh Anil, Raghavendra, U., Gudigar, Anjan, Bhandary, Sarvesh, Salvi, Massimo, Deo, Ravinesh C., Barua, Prabal Datta, Ciaccio, Edward J., Molinari, Filippo and Acharya, RU. Rajendra. 2023. "A Novel Attention-Based Model for Semantic Segmentation of Prostate Glands Using Histopathological Images." IEEE Access. 11, pp. 108982-108994. https://doi.org/10.1109/ACCESS.2023.3321273Efficient daily electricity demand prediction with hybrid deep-learning multi-algorithm approach
Ghimire, Sujan, Deo, Ravinesh C., Casillas-Perez, David and Salcedo-sanz, Sancho. 2023. "Efficient daily electricity demand prediction with hybrid deep-learning multi-algorithm approach." Energy Conversion and Management. 297. https://doi.org/10.1016/j.enconman.2023.117707New achievements on daily reference evapotranspiration forecasting: Potential assessment of multivariate signal decomposition schemes
Ali, Mumtaz, Jamei, Mehdi, Prasad, Ramendra, Karbasi, Masoud, Xiang, Yong, Cai, Borui, Abdulla, Shahab, Farooque, Aitazaz Ahsan and Labban, Abdulhaleem H.. 2023. "New achievements on daily reference evapotranspiration forecasting: Potential assessment of multivariate signal decomposition schemes." Ecological Indicators. 155. https://doi.org/10.1016/j.ecolind.2023.111030Automated detection of airflow obstructive diseases: A systematic review of the last decade (2013-2022)
Xu, Shuting, Deo, Ravinesh C, Soar, Jeffrey, Barua, Prabal Datta, Faust, Oliver, Homaira, Nusrat, Jaffe, Adam, Kabir, Arm Luthful and Acharya, U. Rajendra. 2023. "Automated detection of airflow obstructive diseases: A systematic review of the last decade (2013-2022)." Computer Methods and Programs in Biomedicine. 241. https://doi.org/10.1016/j.cmpb.2023.107746Brain tumor detection and screening using artificial intelligence techniques: Current trends and future perspectives
Raghavendra, U., Gudigar, Anjan, Paul, Aritra, Goutham, T.S., Inamdar, Mahesh Anil, Hegde, Ajay, Dev, Aruna, Ooi, Chui Ping, Deo, Ravinesh C., Barua, Prabal Datta, Molinari, Filippo, Ciaccio, Edward J. and Acharya, U. Rajendra. 2023. "Brain tumor detection and screening using artificial intelligence techniques: Current trends and future perspectives." Computers in Biology and Medicine. 163. https://doi.org/10.1016/j.compbiomed.2023.107063Application of Entropy for Automated Detection of Neurological Disorders With Electroencephalogram Signals: A Review of the Last Decade (2012-2022)
Jui, S. Janifer Jabin, Deo, Ravinesh C. Deo, Barua, Prabal Datta, Devi, Aruna, Soar, Jeffrey and Acharya, U. Rajendra. 2023. "Application of Entropy for Automated Detection of Neurological Disorders With Electroencephalogram Signals: A Review of the Last Decade (2012-2022)." IEEE Access. 11, pp. 71905-71924. https://doi.org/10.1109/ACCESS.2023.3294473Integrated Multi-Head Self-Attention Transformer model for electricity demand prediction incorporating local climate variables
Ghimire, Sujan, Nguyen-Huy, Thong, AL-Musaylh, Mohanad S., Deo, Ravinesh C., Casillas-Perez, David and Salcedo-sanz, Sancho. 2023. "Integrated Multi-Head Self-Attention Transformer model for electricity demand prediction incorporating local climate variables." Energy and AI. 14. https://doi.org/10.1016/j.egyai.2023.100302Multivariate data decomposition based deep learning approach to forecast one-day ahead significant wave height for ocean energy generation
Zheng, Zihao, Ali, Mumtaz, Jamei, Mehdi, Xiang, Yong, Abdulla, Shahab, Yaseen, Zaher Mundher and Farooque, Aitazaz A.. 2023. "Multivariate data decomposition based deep learning approach to forecast one-day ahead significant wave height for ocean energy generation." Renewable and Sustainable Energy Reviews. 185. https://doi.org/https://doi.org/10.1016/j.rser.2023.113645Designing a decomposition-based multi-phase pre-processing strategy coupled with EDBi-LSTM deep learning approach for sediment load forecasting
Jamei, Mehdi, Ali, Mumtaz, Malik, Anurag, Rai, Priya, Karbasi, Masoud, Farooque, Aitazaz A. and Yaseen, Zaher Mundher. 2023. "Designing a decomposition-based multi-phase pre-processing strategy coupled with EDBi-LSTM deep learning approach for sediment load forecasting." Ecological Indicators. 153. https://doi.org/https://doi.org/10.1016/j.ecolind.2023.110478Development of high-resolution gridded data for water availability identification through GRACE data downscaling: Development of machine learning models
Tao, Hai, Al-Sulttani, Ahmed H., Salih, Sinan Q., Mohammed, Mustafa K.A., Khan, Mohammad Amir, Beyaztas, Beste Hamiye, Ali, Mumtaz, Elsayed, Salah, Shahid, Shamsuddin and Yaseen, Zaher Mundher. 2023. "Development of high-resolution gridded data for water availability identification through GRACE data downscaling: Development of machine learning models." Atmospheric Research. 291, pp. 1-13. https://doi.org/https://doi.org/10.1016/j.atmosres.2023.106815The Great 2011 Thailand flood disaster revisited: Could it have been mitigated by different dam operations based on better weather forecasts?
Loc, Ho Huu, Emadzadeh, Adel, Park, Edward, Nontikansak, Piyanuch and Deo, Ravinesh C.. 2023. "The Great 2011 Thailand flood disaster revisited: Could it have been mitigated by different dam operations based on better weather forecasts? " Environmental Research. 216 (Part 2). https://doi.org/10.1016/j.envres.2022.114493Comparison of machine learning methods emulating process driven crop models
Johnston, David B., Pembleton, Keith G., Huth, Neil I. and Deo, Ravinesh C.. 2023. "Comparison of machine learning methods emulating process driven crop models ." Environmental Modelling and Software. 162. https://doi.org/10.1016/j.envsoft.2023.105634Multi-step ahead hourly forecasting of air quality indices in Australia: Application of an optimal time-varying decomposition-based ensemble deep learning algorithm
Jamei, Mehdi, Ali, Mumtaz, Jun, Changhyun, Bateni, Sayed M., Karbasi, Masoud, Farooque, Aitazaz A. and Yaseen, Zaher Mundher. 2023. "Multi-step ahead hourly forecasting of air quality indices in Australia: Application of an optimal time-varying decomposition-based ensemble deep learning algorithm." Atmospheric Pollution Research. 14 (6). https://doi.org/10.1016/j.apr.2023.101752Machine Learning Algorithms for High-Resolution Prediction of Spatiotemporal Distribution of Air Pollution from Meteorological and Soil Parameters
Tao, Hai, Jawad, Ali H., Shather, A.H., Al-Khafaji, Zainab Al, Rashid, Tarik A., Ali, Mumtaz, Al-Ansari, Nadhir Al, Marhoon, Haydar Abdulameer, Shahid, Shamsuddin and Yaseen, Zaher Mundher. 2023. "Machine Learning Algorithms for High-Resolution Prediction of Spatiotemporal Distribution of Air Pollution from Meteorological and Soil Parameters." Environment International. 175, pp. 1-17. https://doi.org/https://doi.org/10.1016/j.envint.2023.107931A novel approach based on integration of convolutional neural networks and echo state network for daily electricity demand prediction
Ghimire, Sujan, Nguyen-Huy, Thong, AL-Musaylh, Mohanad S., Deo, Ravinesh, Casillas-Perez, David and Salcedo-sanz, Sancho. 2023. "A novel approach based on integration of convolutional neural networks and echo state network for daily electricity demand prediction." Energy. 275, p. 127430. https://doi.org/10.1016/j.energy.2023.127430Surface water electrical conductivity and bicarbonate ion determination using a smart hybridization of optimal Boruta package with Elman recurrent neural network
Jamei, Mehdi, Ali, Mumtaz, Karimi, Bakhtiar, Karbasi, Masoud, Farooque, Aitataz Ahsan and Yaseen, Zaher Mundher. 2023. "Surface water electrical conductivity and bicarbonate ion determination using a smart hybridization of optimal Boruta package with Elman recurrent neural network." Process Safety and Environmental Protection. 174, pp. 115-134. https://doi.org/https://doi.org/10.1016/j.psep.2023.03.062Development of neutrosophic cubic hesitant fuzzy exponential aggregation operators with application in environmental protection problems
Rehman, Ateeq Ur, Gulistan, Muhammad, Ali, Mumtaz, M.Al‑Shamiri, Mohammed M. and Abdulla, Shahab. 2023. "Development of neutrosophic cubic hesitant fuzzy exponential aggregation operators with application in environmental protection problems." Scientific Reports. 13, pp. 1-18. https://doi.org/10.1038/s41598-022-22399-3A comprehensive investigation of wetting distribution pattern on sloping lands under drip irrigation: A new gradient boosting multi-filtering-based deep learning approach
Jamei, Mehdi, Karimi, Bakhtiar, Ali, Mumtaz, Alinazari, Fariba, Karbasi, Masoud, Maroufpoor, Eisa and Chu, Xuefeng. 2023. "A comprehensive investigation of wetting distribution pattern on sloping lands under drip irrigation: A new gradient boosting multi-filtering-based deep learning approach." Journal of Hydrology. 620 (Part A), pp. 1-19. https://doi.org/https://doi.org/10.1016/j.jhydrol.2023.129402Accurate Image Multi-Class Classification Neural Network Model with Quantum Entanglement Approach
Riaz, Farina, Abdulla, Shahab, Suzuki, Hajime, Ganguly, Srinjoy, Deo, Ravinesh C. and Hopkins, Susan. 2023. "Accurate Image Multi-Class Classification Neural Network Model with Quantum Entanglement Approach." Sensors. 23 (5), pp. 1-11. https://doi.org/10.3390/s23052753Near real-time wind speed forecast model with bidirectional LSTM networks
Joseph, Lionel P., Deo, Ravinesh C., Prasad, Ramendra, Salcedo-Sanz, Sancho, Raj, Nawin and Soar, Jeffrey. 2023. "Near real-time wind speed forecast model with bidirectional LSTM networks." Renewable Energy. 204, pp. 39-58. https://doi.org/10.1016/j.renene.2022.12.123A novel global solar exposure forecasting model based on air temperature: Designing a new multi-processing ensemble deep learning paradigm
Jamei, Mehdi, Karbasi, Masoud, Ali, Mumtaz, Malik, Anurag, Chu, Xuefeng and Yaseen, Zaher Mundher. 2023. "A novel global solar exposure forecasting model based on air temperature: Designing a new multi-processing ensemble deep learning paradigm." Expert Systems with Applications. 222. https://doi.org/https://doi.org/10.1016/j.eswa.2023.119811Downscaling Surface Albedo to Higher Spatial Resolutions With an Image Super-Resolution Approach and PROBA-V Satellite Images
Deo, Ravinesh C., Karalasingham, Sagthitharan, Casillas-Perez, David, Raj, Narwin and Salcedo-sanz, Sancho. 2023. "Downscaling Surface Albedo to Higher Spatial Resolutions With an Image Super-Resolution Approach and PROBA-V Satellite Images." IEEE Access. 11, pp. 5558-5577. https://doi.org/10.1109/ACCESS.2023.3236253Design data decomposition-based reference evapotranspiration forecasting model: A soft feature filter based deep learning driven approach
Zheng, Zihao, Ali, Mumtaz, Jamei, Mehdi, Xiang, Yong, Karbasi, Masoud, Yaseen, Zaher Mundher and Farooque, Aitazaz Ahsan. 2023. "Design data decomposition-based reference evapotranspiration forecasting model: A soft feature filter based deep learning driven approach." Engineering Applications of Artificial Intelligence. 121. https://doi.org/10.1016/j.engappai.2023.105984Designing pentapartitioned neutrosophic cubic set aggregation operator-based air pollution decision-making model
Li, Yi-ming, Khan, Majid, Khurshid, Adnan, Gulistan, Muhammad, Rehman, Ateeq Ur, Ali, Mumtaz, Abdulla, Shahab and Farooque, Aitazaz A.. 2023. "Designing pentapartitioned neutrosophic cubic set aggregation operator-based air pollution decision-making model ." Complex and Intelligent Systems. https://doi.org/10.1007/s40747-023-00971-2Ensemble robust local mean decomposition integrated with random forest for short-term significant wave height forecasting
Ali, Mumtaz, Prasad, Ramendra, Xiang, Yong, Jamei, Mehdi and Yaseen, Zaher Mundher. 2023. "Ensemble robust local mean decomposition integrated with random forest for short-term significant wave height forecasting." Renewable Energy. 205, pp. 731-746. https://doi.org/https://doi.org/10.1016/j.renene.2023.01.108An intelligent model involving multi-channels spectrum patterns based features for automatic sleep stage classification
Abdulla, Shahab, Diykh, Mohammed, Siuly, Siuly and Ali, Mumtaz. 2023. "An intelligent model involving multi-channels spectrum patterns based features for automatic sleep stage classification." International Journal of Medical Informatics. 171, pp. 1-10. https://doi.org/10.1016/j.ijmedinf.2023.105001Developing a novel hybrid method based on dispersion entropy and adaptive boosting algorithm for human activity recognition
Diykh, Mohammed, Abdulla, Shahab, Deo, Ravinesh C, Siuly, Siuly and Ali, Mumtaz. 2023. "Developing a novel hybrid method based on dispersion entropy and adaptive boosting algorithm for human activity recognition." Computer Methods and Programs in Biomedicine. 229, pp. 1-11. https://doi.org/https://doi.org/10.1016/j.cmpb.2022.107305A study of plithogenic graphs: applications in spreading coronavirus disease (COVID-19) globally
Sultana, Fazeelat, Gulistan, Muhammad, Ali, Mumtaz, Yaqoob, Naveed, Khan, Muhammad, Rashid, Tabasam and Ahmed, Tauseef. 2023. "A study of plithogenic graphs: applications in spreading coronavirus disease (COVID-19) globally ." Journal of Ambient Intelligence and Humanized Computing. 14 (10), p. 13139–13159. https://doi.org/10.1007/s12652-022-03772-6Cloud cover bias correction in numerical weather models for solar energy monitoring and forecasting systems with kernel ridge regression
Deo, Ravinesh C., Ahmed, A.A. Masrur, Casillas-Perez, David, Pourmousavi, S. Ali, Segal, Gary, Yu, Yanshan and Salcedo-sanz, Sancho. 2023. "Cloud cover bias correction in numerical weather models for solar energy monitoring and forecasting systems with kernel ridge regression." Renewable Energy. 203, pp. 113-130. https://doi.org/10.1016/j.renene.2022.12.048A high dimensional features-based cascaded forward neural network coupled with MVMD and Boruta-GBDT for multi-step ahead forecasting of surface soil moisture
Jamei, Mehdi, Ali, Mumtaz, Karbasi, Masoud, Sharma, Ekta, Jamei, Mozhdeh, Chu, Xuefeng and Yaseen, Zaher Mundher. 2023. "A high dimensional features-based cascaded forward neural network coupled with MVMD and Boruta-GBDT for multi-step ahead forecasting of surface soil moisture." Engineering Applications of Artificial Intelligence. 120. https://doi.org/10.1016/j.engappai.2023.105895Development of a TVF-EMD-based multi-decomposition technique integrated with Encoder-Decoder-Bidirectional-LSTM for monthly rainfall forecasting
Jamei, Mehdi, Ali, Mumtaz, Malik, Anurag, Karbasi, Masoud, Rai, Priya and Yaseen, Zaher Mundher. 2023. "Development of a TVF-EMD-based multi-decomposition technique integrated with Encoder-Decoder-Bidirectional-LSTM for monthly rainfall forecasting." Journal of Hydrology. 617 (Part C), pp. 1-21. https://doi.org/10.1016/j.jhydrol.2023.129105Serial Cross-Sectional Observations of Sun-Protective Behaviors at an Annual Outdoor Motorsport Event in Tropical Queensland, Australia
Dexter, Ben, Smith, Annika, King, Rachel, Downs, Nathan J., Nikles, Catherine Jane, Parisi, Alfio V., Ho, Yik-Hong and Harrison, Simone Lee. 2023. "Serial Cross-Sectional Observations of Sun-Protective Behaviors at an Annual Outdoor Motorsport Event in Tropical Queensland, Australia ." Photochemistry and Photobiology. 99 (5), pp. 1352-1356. https://doi.org/10.1111/php.13773Deep Multi-Stage Reference Evapotranspiration Forecasting Model: Multivariate Empirical Mode Decomposition Integrated With the Boruta-Random Forest Algorithm
Jayasinghe, W. J. M. Lakmini Prarthana, Deo, Ravinesh C., Ghahramani, Afshin, Ghimire, Sujan and Raj, Nawin. 2021. "Deep Multi-Stage Reference Evapotranspiration Forecasting Model: Multivariate Empirical Mode Decomposition Integrated With the Boruta-Random Forest Algorithm." IEEE Access. 9, pp. 166695-166708. https://doi.org/10.1109/ACCESS.2021.3135362Satellite monitoring of environmental solar ultraviolet A (UVA) exposure and irradiance: a review of OMI and GOME-2
Parisi, Alfio V., Igoe, Damien, Downs, Nathan J., Turner, Joanna, Amar, Abdurazaq and A Jebar, Mustapha A.. 2021. "Satellite monitoring of environmental solar ultraviolet A (UVA) exposure and irradiance: a review of OMI and GOME-2." Remote Sensing. 13 (4), pp. 1-19. https://doi.org/10.3390/rs13040752Designing Deep-based Learning Flood Forecast Model with ConvLSTM Hybrid Algorithm
Moishin, Mohammed, Deo, Ravinesh C., Prasad, Ramendra, Raj, Nawin and Abdulla, Shahab. 2021. "Designing Deep-based Learning Flood Forecast Model with ConvLSTM Hybrid Algorithm." IEEE Access. 9, pp. 50982-50993. https://doi.org/10.1109/ACCESS.2021.3065939Pattern recognition describing spatio-temporal drivers of catchment classification for water quality
O'Sullivan, Cherie M., Ghahramani, Afshin, Deo, Ravinesh C. and Pembleton, Keith G.. 2023. "Pattern recognition describing spatio-temporal drivers of catchment classification for water quality." Science of the Total Environment. 861, pp. 1-42. https://doi.org/10.1016/j.scitotenv.2022.160240The Playground Shade Index: A New Design Metric for Measuring Shade and Seasonal Ultraviolet Protection Characteristics of Parks and Playgrounds
Downs, Nathan, Raj, Nawin, Vanos, Jennifer, Parisi, Alfio, Butler, Harry, Deo, Ravinesh, Igoe, Damien, Dexter, Benjamin, Beckman-Downs, Melanie, Turner, Joanna and Dekeyser, Stijn. 2023. "The Playground Shade Index: A New Design Metric for Measuring Shade and Seasonal Ultraviolet Protection Characteristics of Parks and Playgrounds." Photochemistry and Photobiology. 99 (4), pp. 1193-1207. https://doi.org/10.1111/php.13745Queensland Guidelines for the provision of tree shade on suburban nature-strips
Downs, Nathan. 2022. Queensland Guidelines for the provision of tree shade on suburban nature-strips. Toowoomba, Australia. University of Southern Queensland.Using Sequence-to-Sequence Models for Carrier Frequency Offset Estimation of Short Messages and Chaotic Maps
Davey, Christopher P., Shakeel, Ismail, Deo, Ravinesh C., Salcedo-sanz, Sancho and Soar, Jeffrey. 2022. "Using Sequence-to-Sequence Models for Carrier Frequency Offset Estimation of Short Messages and Chaotic Maps." IEEE Access. 10, pp. 119814 - 119825. https://doi.org/10.1109/ACCESS.2022.3221762Developing a novel hybrid Auto Encoder Decoder Bidirectional Gated Recurrent Unit model enhanced with empirical wavelet transform and Boruta-Catboost to forecast significant wave height
Karbasi, Masoud, Jamei, Mehdi, Ali, Mumtaz, Abdulla, Shahab, Chu, Xuefeng and Yaseen, Zaher Mundher. 2022. "Developing a novel hybrid Auto Encoder Decoder Bidirectional Gated Recurrent Unit model enhanced with empirical wavelet transform and Boruta-Catboost to forecast significant wave height." Journal of Cleaner Production. 379 (Part 2). https://doi.org/10.1016/j.jclepro.2022.134820Modelling and Real-time Optimisation of Air Quality Predictions for Australia through Artificial Intelligence Algorithm
Sharma, Ekta, Deo, Ravinesh C., Prasad, Ramendra and Parisi, Alfio V.. 2019. "Modelling and Real-time Optimisation of Air Quality Predictions for Australia through Artificial Intelligence Algorithm." AMSI Optimise 2019. Perth, Australia 17 - 21 Jun 2019 Perth, Australia.Experimental and computational assessment of wetting pattern for two-layered soil profiles in pulse drip irrigation: Designing a novel optimized bidirectional deep learning paradigm
Jamei, Mehdi, Karimi, Farahnaz, Ali, Mumtaz, Karimi, Bakhtiar, Karbasi, Masoud and Aminpour, Younes. 2022. "Experimental and computational assessment of wetting pattern for two-layered soil profiles in pulse drip irrigation: Designing a novel optimized bidirectional deep learning paradigm." Journal of Hydrology. 614 (Part A), pp. 1-15. https://doi.org/10.1016/j.jhydrol.2022.128496Hybrid Convolutional Neural Network-Multilayer Perceptron Model for Solar Radiation Prediction
Ghimire, Sujan, Nguyen-Huy, Thong, Prasad, Ramendra, Deo, Ravinesh C., Casillas-Perez, David, Salcedo-sanz, Sancho and Bhandari, Binayak. 2023. "Hybrid Convolutional Neural Network-Multilayer Perceptron Model for Solar Radiation Prediction." Cognitive Computation. 15 (2), pp. 645-671. https://doi.org/10.1007/s12559-022-10070-yStudent Performance Predictions for Advanced Engineering Mathematics Course With New Multivariate Copula Models
Nguyen-Huy, Thong, Deo, Ravinesh C., Khan, Shahjahan, Devi, Aruna, Adeyinka, Adewuyi Ayodele, Apan, Armando A. and Yaseen, Zaher Mundher. 2022. "Student Performance Predictions for Advanced Engineering Mathematics Course With New Multivariate Copula Models." IEEE Access. 10, pp. 45112 -45136. https://doi.org/10.1109/ACCESS.2022.3168322Rapid assessment of mine rehabilitation areas with airborne LiDAR and deep learning: bauxite strip mining in Queensland, Australia
Murray, Xavier, Apan, Armando, Deo, Ravinesh and Maraseni, Tek. 2022. "Rapid assessment of mine rehabilitation areas with airborne LiDAR and deep learning: bauxite strip mining in Queensland, Australia." Geocarto International. 37 (26), pp. 11223-11252. https://doi.org/10.1080/10106049.2022.2048902Multi-strategy Slime Mould Algorithm for hydropower multi-reservoir systems optimization
Ahmadianfar, Iman, Noori, Ramzia Majeed, Togun, Hussein, Falah, Mayadah W., Homod, Raad Z., Fu, Minglei, Halder, Bijay, Deo, Ravinesh and Yaseen, Zaher Mundher. 2022. "Multi-strategy Slime Mould Algorithm for hydropower multi-reservoir systems optimization." Knowledge-Based Systems. 250, pp. 1-18. https://doi.org/10.1016/j.knosys.2022.109048Suspended sediment load modeling using advanced hybrid rotation forest based elastic network approach
Khosravi, Khabat, Golkarian, Ali, Melesse, Assefa M. and Deo, Ravinesh C.. 2022. "Suspended sediment load modeling using advanced hybrid rotation forest based elastic network approach." Journal of Hydrology. 610, pp. 1-14. https://doi.org/10.1016/j.jhydrol.2022.127963Delineating the Crop-Land Dynamic due to Extreme Environment Using Landsat Datasets: A Case Study
Halder, Bijay, Bandyopadhyay, Jatisankar, Afan, Haitham Abdulmohsin, Naser, Maryam H., Abed, Salwan Ali, Khedher, Khaled Mohamed, Falih, Khaldoon T., Deo, Ravinesh, Scholz, Miklas and Yaseen, Zaher Mundher. 2022. "Delineating the Crop-Land Dynamic due to Extreme Environment Using Landsat Datasets: A Case Study." Agronomy. 12 (6), pp. 1-23. https://doi.org/10.3390/agronomy12061268Coupled online sequential extreme learning machine model with ant colony optimization algorithm for wheat yield prediction
Ali, Mumtaz, Deo, Ravinesh C., Xiang, Yong, Prasad, Ramendra, Li, Jianxin, Farooque, Aitazaz and Yaseen, Zaher Mundher. 2022. "Coupled online sequential extreme learning machine model with ant colony optimization algorithm for wheat yield prediction." Scientific Reports. 12 (1), pp. 1-23. https://doi.org/10.1038/s41598-022-09482-5Forecasting solar photosynthetic photon flux density under cloud cover effects: novel predictive model using convolutional neural network integrated with long short-term memory network
Deo, Ravinesh C., Grant, Richard H., Webb, Ann, Ghimire, Sujan, Igoe, Damien P., Downs, Nathan J., Al-Musaylh, Mohanad S., Parisi, Alfio V. and Soar, Jeffrey. 2022. "Forecasting solar photosynthetic photon flux density under cloud cover effects: novel predictive model using convolutional neural network integrated with long short-term memory network." Stochastic Environmental Research and Risk Assessment. 36, p. 3183–3220. https://doi.org/10.1007/s00477-022-02188-0Improved Complete Ensemble Empirical Mode Decomposition with Adaptive Noise Deep Residual model for short-term multi-step solar radiation prediction
Ghimire, Sujan, Deo, Ravinesh C, Casillas-Perez, David and Salcedo-sanz, Sancho. 2022. "Improved Complete Ensemble Empirical Mode Decomposition with Adaptive Noise Deep Residual model for short-term multi-step solar radiation prediction." Renewable Energy. 190, pp. 408-424. https://doi.org/10.1016/j.renene.2022.03.120Efficient daily solar radiation prediction with deep learning 4-phase convolutional neural network, dual stage stacked regression and support vector machine CNN-REGST hybrid model
Ghimire, Sujan, Nguyen-Huy, Thong, Deo, Ravinesh C., Casillas-Perez, David and Salcedo-sanz, Sancho. 2022. "Efficient daily solar radiation prediction with deep learning 4-phase convolutional neural network, dual stage stacked regression and support vector machine CNN-REGST hybrid model." Sustainable Materials and Technologies. 32, pp. 1-24. https://doi.org/10.1016/j.susmat.2022.e00429Hybrid deep CNN-SVR algorithm for solar radiation prediction problems in Queensland, Australia
Ghimire, Sujan, Bhandari, Binayak, Casillas-Perez, David, Deo, Ravinesh C. and Salcedo-sanz, Sancho. 2022. "Hybrid deep CNN-SVR algorithm for solar radiation prediction problems in Queensland, Australia." Engineering Applications of Artificial Intelligence. 112, pp. 1-26. https://doi.org/10.1016/j.engappai.2022.104860Boosting solar radiation predictions with global climate models, observational predictors and hybrid deep-machine learning algorithms
Ghimire, Sujan, Deo, Ravinesh C., Casillas-Perez, David and Salcedo-sanz, Sancho. 2022. "Boosting solar radiation predictions with global climate models, observational predictors and hybrid deep-machine learning algorithms." Applied Energy. 316, pp. 1-25. https://doi.org/10.1016/j.apenergy.2022.119063Machine learning regression and classification methods for fog events prediction
Castillo-Boton, C., Casillas-Perez, D., Casanova-Mateo, C., Ghimire, S., Cerro-Prada, E., Gutierrez, P. A., Deo, R. C. and Salcedo-sanz, S.. 2022. "Machine learning regression and classification methods for fog events prediction." Atmospheric Research. 272, pp. 1-23. https://doi.org/10.1016/j.atmosres.2022.106157Quantum Artificial Intelligence Predictions Enhancement by Improving Signal Processing
Riaz, Farina, Abdulla, Shahab, Ni, Wei, Radfar, Mohsen, Deo, Ravinesh and Hopkins, Susan. 2022. "Quantum Artificial Intelligence Predictions Enhancement by Improving Signal Processing." Quantum Australia Conference 2022. Online 23 - 25 Feb 2022 Toowoomba, Australia. https://doi.org/10.13140/RG.2.2.34754.66245Forecasting Daily Flood Water Level Using Hybrid Advanced Machine Learning Based Time‑Varying Filtered Empirical Mode Decomposition Approach
Jamei, Mehdi, Ali, Mumtaz, Malik, Anurag, Prasad, Ramendra, Abdulla, Shahab and Yaseen, Zaher Mundher. 2022. "Forecasting Daily Flood Water Level Using Hybrid Advanced Machine Learning Based Time‑Varying Filtered Empirical Mode Decomposition Approach." Water Resources Management. 36 (12), p. 4637–4676. https://doi.org/10.1007/s11269-022-03270-6Deep learning CNN-LSTM-MLP hybrid fusion model for feature optimizations and daily solar radiation prediction
Ghimire, Sujan, Deo, Ravinesh C., Casillas-Perez, David, Salcedo-sanz, Sancho, Sharma, Ekta and Ali, Mumtaz. 2022. "Deep learning CNN-LSTM-MLP hybrid fusion model for feature optimizations and daily solar radiation prediction." Measurement. 202, pp. 1-22. https://doi.org/10.1016/j.measurement.2022.111759Basin management inspiration from impacts of alternating dry and wet conditions on water production and carbon uptake in Murray-Darling Basin
Lu, Zhixiang, Feng, Qi, Wei, Yongping, Zhao, Yan, Deo, Ravinesh C., Xie, Jiali, Zhou, Sha, Zhu, Meng and Xu, Min. 2022. "Basin management inspiration from impacts of alternating dry and wet conditions on water production and carbon uptake in Murray-Darling Basin." Science of the Total Environment. 851 (Part 2), pp. 1-8. https://doi.org/10.1016/j.scitotenv.2022.158359Introductory Engineering Mathematics Students’ Weighted Score Predictions Utilising a Novel Multivariate Adaptive Regression Spline Model
Ahmed, Abul Abrar Masrur, Deo, Ravinesh C., Ghimire, Sujan, Downs, Nathan J., Devi, Aruna, Barua, Prabal D. and Yaseen, Zaher M.. 2022. "Introductory Engineering Mathematics Students’ Weighted Score Predictions Utilising a Novel Multivariate Adaptive Regression Spline Model." Sustainability. 14 (17), pp. 1-27. https://doi.org/10.3390/su141711070Kernel Ridge Regression Hybrid Method for Wheat Yield Prediction with Satellite-Derived Predictors
Ahmed, A. A. Masrur, Sharma, Ekta, Jui, S. Janifer Jabin, Deo, Ravinesh C., Nguyen-Huy, Thong and Ali, Mumtaz. 2022. "Kernel Ridge Regression Hybrid Method for Wheat Yield Prediction with Satellite-Derived Predictors." Remote Sensing. 14 (5), pp. 1-24. https://doi.org/10.3390/rs14051136Cloud Affected Solar UV Predictions with Three-Phase Wavelet Hybrid Convolutional Long Short-Term Memory Network Multi-Step Forecast System
Prasad, Salvin S., Deo, Ravinesh C., Downs, Nathan, Igoe, Damien, Parisi, Alfio V. and Soar, Jeffrey. 2022. "Cloud Affected Solar UV Predictions with Three-Phase Wavelet Hybrid Convolutional Long Short-Term Memory Network Multi-Step Forecast System." IEEE Access. 10, pp. 24704-24720. https://doi.org/10.1109/ACCESS.2022.3153475Development and evaluation of hybrid deep learning long short-term memory network model for pan evaporation estimation trained with satellite and ground-based data
Jayasinghe, W. J. M. Lakmini Prarthana, Deo, Ravinesh C., Ghahramani, Afshin, Ghimire, Sujan and Raj, Nawin. 2022. "Development and evaluation of hybrid deep learning long short-term memory network model for pan evaporation estimation trained with satellite and ground-based data." Journal of Hydrology. 607, pp. 1-19. https://doi.org/10.1016/j.jhydrol.2022.127534Integrative artificial intelligence models for Australian coastal sediment lead prediction: An investigation of in-situ measurements and meteorological parameters effects
Bhagat, Suraj Kumar, Tiyasha, Tiyasha, Kumar, Adarsh, Malik, Tabarak, Jawad, Ali H., Khedher, Khaled Mohamed, Deo, Ravinesh C. and Yaseen, Zaher Mundher. 2022. "Integrative artificial intelligence models for Australian coastal sediment lead prediction: An investigation of in-situ measurements and meteorological parameters effects." Journal of Environmental Management. 309, pp. 1-16. https://doi.org/10.1016/j.jenvman.2022.114711An Eigenvalues-Based Covariance Matrix Bootstrap Model Integrated With Support Vector Machines for Multichannel EEG Signals Analysis
Al-Hadeethi, Hanan, Abdulla, Shahab, Diykh, Mohammed, Deo, Ravinesh C. and Green, Jonathan H.. 2022. "An Eigenvalues-Based Covariance Matrix Bootstrap Model Integrated With Support Vector Machines for Multichannel EEG Signals Analysis." Frontiers in Neuroinformatics. 15, pp. 1-15. https://doi.org/10.3389/fninf.2021.808339Stacked LSTM Sequence-to-Sequence Autoencoder with Feature Selection for Daily Solar Radiation Prediction: A Review and New Modeling Results
Ghimire, Sujan, Deo, Ravinesh C., Wang, Hua, Al-Musaylh, Mohanad S., Casillas-Perez, David and Salcedo-sanz, Sancho. 2022. "Stacked LSTM Sequence-to-Sequence Autoencoder with Feature Selection for Daily Solar Radiation Prediction: A Review and New Modeling Results." Energies. 15 (3), pp. 1-39. https://doi.org/10.3390/en15031061Texture analysis based graph approach for automatic detection of neonatal seizure from multi-channel EEG signals
Diykh, Mohammed, Miften, Firas Sabar, Abdulla, Shahab, Deo, Ravinesh C., Siuly, Siuly, Green, Jonathan H. and Oudah, Atheer Y.. 2022. "Texture analysis based graph approach for automatic detection of neonatal seizure from multi-channel EEG signals." Measurement. 190 (110731), pp. 1-13. https://doi.org/10.1016/j.measurement.2022.110731Air quality monitoring based on chemical and meteorological drivers: Application of a novel data filtering-based hybridized deep learning model
Jamei, Mehdi, Ali, Mumtaz, Malik, Anurag, Karbasi, Masoud, Sharma, Ekta and Yaseen, Zaher Mundher. 2022. "Air quality monitoring based on chemical and meteorological drivers: Application of a novel data filtering-based hybridized deep learning model." Journal of Cleaner Production. 374, pp. 1-17. https://doi.org/10.1016/j.jclepro.2022.134011Classification of catchments for nitrogen using Artificial Neural Network Pattern Recognition and spatial data
O'Sullivan, Cherie M., Ghahramani, Afshin, Deo, Ravinesh C., Pembleton, Keith, Khan, Urooj and Tuteja, Narendra. 2022. "Classification of catchments for nitrogen using Artificial Neural Network Pattern Recognition and spatial data." Science of the Total Environment. 809, pp. 1-15. https://doi.org/10.1016/j.scitotenv.2021.151139Study on the Development of Neutrosophic Triplet Ring and Neutrosophic Triplet Field
Ali, Mumtaz, Smarandache, Florentin and Khan, Mohsin. 2018. "Study on the Development of Neutrosophic Triplet Ring and Neutrosophic Triplet Field." Mathematics. 6, pp. 1-11. https://doi.org/10.3390/math6040046Domino effect of climate change over two millennia in ancient China’s Hexi Corridor
Feng, Qi, Yan, Linshan, Deo, Ravinesh C., AghaKouchak, Amir, Adamowski, Jan F., Stone, Roger, Yin, Zhenliang, Liu, Wei, Si, Jianhua, Wen, Xiaohu, Zhu, Meng and Cao, Shixiong. 2019. "Domino effect of climate change over two millennia in ancient China’s Hexi Corridor." Nature Sustainability. 2, pp. 957-961. https://doi.org/10.1038/s41893-019-0397-9Bat algorithm for dam–reservoir operation
Ethteram, Mohammad, Mousavi, Sayed-Farhad, Karami, Hojat, Farzin, Saeed, Deo, Ravinesh, Othman, Faridah Binti, Chau, Kwok-Wing, Sarkamaryan, Saeed, Singh, Vijay P. and El-Shafie, Ahmed. 2018. "Bat algorithm for dam–reservoir operation." Environmental Earth Sciences. 77 (13), pp. 1-15. https://doi.org/10.1007/s12665-018-7662-5Characteristics of ecosystem water use efficiency in a desert riparian forest
Ma, Xiaohong, Feng, Qi, Su, Yonghong, Yu, Tengfei and Deo, Ravinesh C.. 2018. "Characteristics of ecosystem water use efficiency in a desert riparian forest." Environmental Earth Sciences. 77 (358). https://doi.org/10.1007/s12665-018-7518-zThe influence of climatic inputs on stream-flow pattern forecasting: case study of Upper Senegal River
Diop, Lamine, Bodian, Ansoumana, Djaman, Koffi, Yaseen, Zaher Mundher, Deo, Ravinesh C., El-Shafie, Ahmed and Brown, Larry C.. 2018. "The influence of climatic inputs on stream-flow pattern forecasting: case study of Upper Senegal River." Environmental Earth Sciences. 77 (5). https://doi.org/10.1007/s1266Uncertainty assessment of the multilayer perceptron (MLP) neural network model with implementation of the novel hybrid MLP-FFA method for prediction of biochemical oxygen demand and dissolved oxygen: a case study of Langat River
Raheli, Bahare, Aalami, Mohammad Taghi, El-Shafie, Ahmed, Ghorbani, Mohammad Ali and Deo, Ravinesh C.. 2017. "Uncertainty assessment of the multilayer perceptron (MLP) neural network model with implementation of the novel hybrid MLP-FFA method for prediction of biochemical oxygen demand and dissolved oxygen: a case study of Langat River." Environmental Earth Sciences. 76 (14). https://doi.org/10.1007/s12665-017-6842-zNeutrosophic Recommender System for Medical Diagnosis Based on Algebraic Similarity Measure and Clustering
Thanh, Nguyen Dang, Son, Le Hoang and Ali, Mumtaz. 2017. "Neutrosophic Recommender System for Medical Diagnosis Based on Algebraic Similarity Measure and Clustering." 2017 IEEE International Conference on Fuzzy Systems (FUZZ-IEEE). Naples, Italy 09 - 12 Jul 2017 Piscataway, United States. https://doi.org/10.1109/FUZZ-IEEE.2017.8015387An intuitionistic fuzzy clustering algorithm based on a new correlation coefficient with application in medical diagnosis
Thao, Nguyen Xuan, Ali, Mumtaz and Smarandache, Florentin. 2019. "An intuitionistic fuzzy clustering algorithm based on a new correlation coefficient with application in medical diagnosis." Journal of Intelligent and Fuzzy Systems. 36 (1), pp. 189-198. https://doi.org/10.3233/JIFS-181084Linguistic Approaches to Interval Complex Neutrosophic Sets in Decision Making
Dat, Luu Quoc, Thong, Nguyen Tho, Son, Le Hoang, Ali, Mumtaz, Smarandache, Florentin, Abdel-Basset, Mohamed and Long, Hoang Viet. 2019. "Linguistic Approaches to Interval Complex Neutrosophic Sets in Decision Making." IEEE Access. 7, pp. 38902-38917. https://doi.org/10.1109/ACCESS.2019.2902841Dynamic interval valued neutrosophic set: Modeling decision making in dynamic environments
Thong, Nguyen Tho, Dat, Luu Quoc, Son, Le Hoang, Hoa, Nguyen Dinh, Ali, Mumtaz and Smarandache, Florentin. 2019. "Dynamic interval valued neutrosophic set: Modeling decision making in dynamic environments." Computers in Industry. 108, pp. 45-52. https://doi.org/10.1016/j.compind.2019.02.009Fuzzy and neutrosophic modeling for link prediction in social networks
Tuan, Tran Manh, Chuan, Pham Minh, Ali, Mumtaz, Ngan, Tran Thi, Mittal, Mamta and Son, Le Hoang. 2019. "Fuzzy and neutrosophic modeling for link prediction in social networks." Evolving Systems. 10, pp. 629-634. https://doi.org/10.1007/s12530-018-9251-yNovel hybrid deep learning model for satellite based PM10 forecasting in the most polluted Australian hotspots
Sharma, Ekta, Deo, Ravinesh C., Soar, Jeffrey, Prasad, Ramendra, Parisi, Alfio V. and Raj, Nawin. 2022. "Novel hybrid deep learning model for satellite based PM10 forecasting in the most polluted Australian hotspots." Atmospheric Environment. 279, pp. 1-13. https://doi.org/10.1016/j.atmosenv.2022.119111New double decomposition deep learning methods for river water level forecasting
Ahmed, A. A. Masrur, Deo, Ravinesh C., Ghahramani, Afshin, Feng, Qi, Raj, Nawin, Yin, Zhenliang and Yang, Linshan. 2022. "New double decomposition deep learning methods for river water level forecasting." Science of the Total Environment. 831, pp. 1-21. https://doi.org/10.1016/j.scitotenv.2022.154722A Case Study of UV Exposure Risk in Sydney during the 2019/2020 New South Wales Bushfires
Igoe, Damien P., Parisi, Alfio V., Downs, Nathan J. and Butler, Harry. 2022. "A Case Study of UV Exposure Risk in Sydney during the 2019/2020 New South Wales Bushfires." Photochemistry and Photobiology. 98 (5), pp. 1236-1244. https://doi.org/10.1111/php.13603Multi-step daily forecasting of reference evapotranspiration for different climates of India: A modern multivariate complementary technique reinforced with ridge regression feature selection
Malik, Anurag, Jamei, Mehdi, Ali, Mumtaz, Prasad, Ramendra, Karbasi, Masoud and Yaseen, Zaher Mundher. 2022. "Multi-step daily forecasting of reference evapotranspiration for different climates of India: A modern multivariate complementary technique reinforced with ridge regression feature selection." Agricultural Water Management. 272. https://doi.org/https://doi.org/10.1016/j.agwat.2022.107812A hierarchical classification/regression algorithm for improving extreme wind speed events prediction
Pelaez-Rodriguez, C., Perez-Aracil, J., Fister, D, Prieto-Godino, L., Deo, R.C. and Salcedo-sanz, S.. 2022. "A hierarchical classification/regression algorithm for improving extreme wind speed events prediction." Renewable Energy. 201 (Part 2), pp. 157-178. https://doi.org/10.1016/j.renene.2022.11.042Designing a Multi-Stage Expert System for daily ocean wave energy forecasting: A multivariate data decomposition-based approach
Jamei, Mehdi, Ali, Mumtaz, Karbasi, Masoud, Xiang, Yong, Ahmadianfar, Iman and Yaseen, Zaher Mundher. 2022. "Designing a Multi-Stage Expert System for daily ocean wave energy forecasting: A multivariate data decomposition-based approach ." Applied Energy. 326, pp. 1-24. https://doi.org/10.1016/j.apenergy.2022.119925Forecasting weekly reference evapotranspiration using Auto Encoder Decoder Bidirectional LSTM model hybridized with a Boruta-CatBoost input optimizer
Karbasi, Masoud, Jamei, Mehdi, Ali, Mumtaz, Malik, Anurag and Yaseen, Zaher Mundher. 2022. "Forecasting weekly reference evapotranspiration using Auto Encoder Decoder Bidirectional LSTM model hybridized with a Boruta-CatBoost input optimizer." Computers and Electronics in Agriculture. 198, pp. 1-17. https://doi.org/10.1016/j.compag.2022.107121Fuzzy Equivalence on Standard and Rough Neutrosophic Sets and Applications to Clustering Analysis
Thao, Nguyen Xuan, Son, Le Hoang, Cuong, Bui Cong, Ali, Mumtaz and Lan, Luong Hong. 2018. "Fuzzy Equivalence on Standard and Rough Neutrosophic Sets and Applications to Clustering Analysis." Bhateja, Vikrant, Nguyen, Bao Le, Nguyen, Nhu Gia, Satapathy, Suresh Chandra and Le, Dac-Nhuong (ed.) 4th International Conference on Information Systems Design and Intelligent Applications (INDIA 2017). Da Nang, Vietnam 15 - 17 Jun 2017 Singapore. https://doi.org/10.1007/978-981-10-7512-4_82Wearable ultraviolet radiation sensors for research and personal use
Henning, Alyssa, Downs, Nathan J. and Vanos, Jennifer K.. 2022. "Wearable ultraviolet radiation sensors for research and personal use." International Journal of Biometeorology: the description, causes, and implications of climatic change. 66 (3), pp. 627-640. https://doi.org/10.1007/s00484-021-02216-8Wind speed forecasting in Nepal using self-organizing map-based online sequential extreme learning machine
Sharma, Neelesh and Deo, Ravinesh. 2021. "Wind speed forecasting in Nepal using self-organizing map-based online sequential extreme learning machine." Deo, Ravinesh, Samui, Pijush and Roy, Sanjiban Sekhar (ed.) Predictive Modelling for Energy Management and Power Systems Engineering. Netherlands. Elsevier. pp. 437-484Support vector machine model for multistep wind speed forecasting
Prasad, Shobna Mohini Mala, Nguyen-Huy, Thong and Deo, Ravinesh. 2021. "Support vector machine model for multistep wind speed forecasting." Deo, Ravinesh, Samui, Pijush and Roy, Sanjiban Sekhar (ed.) Predictive modelling for energy management and power systems engineering. Netherlands. Elsevier. pp. 335-389Development of data-driven models for wind speed forecasting in Australia
Neupane, Ananta, Raj, Nawin, Deo, Ravinesh and Ali, Mumtaz. 2021. "Development of data-driven models for wind speed forecasting in Australia." Deo, R., Samui, Pijush and Roy, Sanjiban Sekhar (ed.) Predictive modelling for energy management and power systems engineering. Netherlands. Elsevier. pp. 143-190Sun exposure and physical activity: the valuable role of UV wearables
Henning, Alyssa, Vanos, Jennifer and Downs, Nathan. 2021. "Sun exposure and physical activity: the valuable role of UV wearables." IEEE Technology and Society Magazine. 40 (3), pp. 16-18. https://doi.org/10.1109/MTS.2021.3101927Application of deep learning models for automated identification of Parkinson’s disease: a review (2011–2021)
Loh, Hui Wen, Hong, Wanrong, Ooi, Chui Ping, Chakraborty, Subrata, Barua, Prabal Datta, Deo, Ravinesh C., Soar, Jeffrey, Palmer, Elizabeth E. and Acharya, U. Rajendra. 2021. "Application of deep learning models for automated identification of Parkinson’s disease: a review (2011–2021)." Sensors. 21, pp. 1-27. https://doi.org/10.3390/s21217034Solar ultraviolet radiation, shade profile and heat index survey report: New South Wales playgrounds (2020 - 2021)
Downs, Nathan J.. 2021. Solar ultraviolet radiation, shade profile and heat index survey report: New South Wales playgrounds (2020 - 2021). Unpublished.Artificial intelligence models for suspended river sediment prediction: state-of-the art, modeling framework appraisal, and proposed future research directions
Tao, Hai, Al-Khafaji, Zainab S., Qi, Chongchong, Zounemat-Kermani, Mohammad, Kisi, Ozgur, Tiyasha, Tiyasha, Chau, Kwok-Wing, Nourani, Vahid, Melesse, Assefa M., Elhakeem, Mohamed, Farooque, Aitazaz Ahsan, Nejadhashemi, A. Pouyan, Khedher, Khaled Mohamed, Alawi, Omer A., Deo, Ravinesh C., Shahid, Shamsuddin, Singh, Vijay P. and Yaseen, Zaher Mundher. 2021. "Artificial intelligence models for suspended river sediment prediction: state-of-the art, modeling framework appraisal, and proposed future research directions." Engineering Applications of Computational Fluid Mechanics. 15 (1), pp. 1585-1612. https://doi.org/10.1080/19942060.2021.1984992Advanced extreme learning machines vs. deep learning models for peak wave energy period forecasting: A case study in Queensland, Australia
Ali, Mumtaz, Prasad, Ramendra, Xiang, Yong, Sankaran, Adarsh, Deo, Ravinesh C., Xiao, Fuyuan and Zhu, Shuyu. 2021. "Advanced extreme learning machines vs. deep learning models for peak wave energy period forecasting: A case study in Queensland, Australia." Renewable Energy. 177, pp. 1033-1044. https://doi.org/10.1016/j.renene.2021.06.052Novel short-term solar radiation hybrid model: long short-term memory network integrated with robust local mean decomposition
Huynh, Anh Ngoc-Lan, Deo, Ravinesh C., Ali, Mumtaz, Abdulla, Shahab and Raj, Nawin. 2021. "Novel short-term solar radiation hybrid model: long short-term memory network integrated with robust local mean decomposition." Applied Energy. 298, pp. 1-19. https://doi.org/10.1016/j.apenergy.2021.117193Evaluating management strategies for sustainable crop production under changing climate conditions: a system dynamics approach
Pham, Yen Hoang, Reardon-Smith, Kathryn and Deo, Ravinesh C.. 2021. "Evaluating management strategies for sustainable crop production under changing climate conditions: a system dynamics approach." Journal of Environmental Management. 292. https://doi.org/10.1016/j.jenvman.2021.112790An interplay of soil salinization and groundwater degradation threatening coexistence of oasis-desert ecosystems
Yin, Xinwei, Feng, Qi, Li, Yan, Deo, Ravinesh C., Liu, Wei, Zhu, Meng, Zheng, Xinjun and Liu, Ran. 2022. "An interplay of soil salinization and groundwater degradation threatening coexistence of oasis-desert ecosystems." Science of the Total Environment. 806 (2), pp. 1-20. https://doi.org/10.1016/j.scitotenv.2021.150599Hybrid deep learning method for a week-ahead evapotranspiration forecasting
Ahmed, A. A. Masrur, Deo, Ravinesh C., Feng, Qi, Ghahramani, Afshin, Raj, Nawin, Yin, Zhenliang and Yang, Linshan. 2022. "Hybrid deep learning method for a week-ahead evapotranspiration forecasting." Stochastic Environmental Research and Risk Assessment. 36 (3), pp. 831-849. https://doi.org/10.1007/s00477-021-02078-xStreamflow prediction using an integrated methodology based on convolutional neural network and long short‑term memory networks
Ghimire, Sujan, Yaseen, Zaher Mundher, Farooque, Aitazaz A., Deo, Ravinesh C., Zhang, Ji and Tao, Xiaohui. 2021. "Streamflow prediction using an integrated methodology based on convolutional neural network and long short‑term memory networks." Scientific Reports. 11, pp. 1-26. https://doi.org/10.1038/s41598-021-96751-4The role of internal transcribed spacer 2 secondary structures in classifying mycoparasitic Ampelomyces
Prahl, Rosa E., Khan, Shahjahan and Deo, Ravinesh C.. 2021. "The role of internal transcribed spacer 2 secondary structures in classifying mycoparasitic Ampelomyces." PLoS One. 16 (6), pp. 1-28. https://doi.org/10.1371/journal.pone.0253772Evaluation of the Long-term Cumulative UVA Facial Exposure of Queensland School Teachers derived for an Extended Period from the OMI Satellite Irradiance
A Jebar, Mustapha A., Downs, Nathan J., Parisi, Alfio V. and Turner, Joanna. 2021. "Evaluation of the Long-term Cumulative UVA Facial Exposure of Queensland School Teachers derived for an Extended Period from the OMI Satellite Irradiance." Photochemistry and Photobiology. 97 (1), pp. 192-197. https://doi.org/10.1111/php.13329Deep Learning Forecasts of Soil Moisture: Convolutional Neural Network and Gated Recurrent Unit Models Coupled with Satellite-Derived MODIS, Observations and Synoptic-Scale Climate Index Data
Ahmed, A. A. Masrur, Deo, Ravinesh C., Raj, Nawin, Ghahramani, Afshin, Feng, Qi, Yin, Zhenliang and Yang, Linshan. 2021. "Deep Learning Forecasts of Soil Moisture: Convolutional Neural Network and Gated Recurrent Unit Models Coupled with Satellite-Derived MODIS, Observations and Synoptic-Scale Climate Index Data." Remote Sensing. 13 (4), pp. 1-30. https://doi.org/10.3390/rs13040554LSTM integrated with Boruta-random forest optimiser for soil moisture estimation under RCP4.5 and RCP8.5 global warming scenarios
Ahmed, A. A. Masrur, Deo, Ravinesh C., Ghahramani, Afshin, Raj, Nawin, Feng, Qi, Yin, Zhenliang and Yang, Linshan. 2021. "LSTM integrated with Boruta-random forest optimiser for soil moisture estimation under RCP4.5 and RCP8.5 global warming scenarios." Stochastic Environmental Research and Risk Assessment. 35, pp. 1851-1881. https://doi.org/10.1007/s00477-021-01969-3A new framework for classification of multi-category hand grasps using EMG signals
Miften, Firas Sabar, Diykh, Mohammed, Abdulla, Shahab, Siuly, Siuly, Green, Jonathan H. and Deo, Ravinesh C.. 2021. "A new framework for classification of multi-category hand grasps using EMG signals." Artificial Intelligence in Medicine. 112, pp. 1-14. https://doi.org/10.1016/j.artmed.2020.102005Deep learning hybrid model with Boruta-Random forest optimiser algorithm for streamflow forecasting with climate mode indices, rainfall, and periodicity
Ahmed, A. A. Masrur, Deo, Ravinesh C., Feng, Qi, Ghahramani, Afshin, Raj, Nawin, Yin, Zhenliang and Yang, Linshan. 2021. "Deep learning hybrid model with Boruta-Random forest optimiser algorithm for streamflow forecasting with climate mode indices, rainfall, and periodicity." Journal of Hydrology. 599, pp. 1-23. https://doi.org/10.1016/j.jhydrol.2021.126350Modeling soil temperature using air temperature features in diverse climatic conditions with complementary machine learning models
Bayatvarkeshi, Maryam, Bhagat, Suraj Kumar, Mohammadi, Kourosh, Kisi, Ozgur, Farahani, M., Hasani, A., Deo, Ravinesh and Yaseen, Zaher Mundher. 2021. "Modeling soil temperature using air temperature features in diverse climatic conditions with complementary machine learning models." Computers and Electronics in Agriculture. 185. https://doi.org/10.1016/j.compag.2021.106158SEQ Ultraviolet and Cloud Measurement Data Collection
Downs, Nathan. SEQ Ultraviolet and Cloud Measurement Data Collection. Toowoomba. https://doi.org/10.26192/9209-3504Electronic Sun Journal Versus Self-report Sun Diary: A Comparison of Recording Personal Sunlight Exposure Methods
Dexter, Benjamin R., King, Rachel, Parisi, Alfio V., Harrison, Simone L., Igoe, Damien P. and Downs, Nathan J.. 2021. "Electronic Sun Journal Versus Self-report Sun Diary: A Comparison of Recording Personal Sunlight Exposure Methods." Photochemistry and Photobiology. 97 (3), pp. 641-649. https://doi.org/10.1111/php.13359Mapping rice area and yield in northeastern asia by incorporating a crop model with dense vegetation index profiles from a geostationary satellite
Yeom, Jong-Min, Jeong, Seungtaek, Deo, Ravinesh C. and Ko, Jonghan. 2021. "Mapping rice area and yield in northeastern asia by incorporating a crop model with dense vegetation index profiles from a geostationary satellite." GIScience and Remote Sensing. https://doi.org/10.1080/15481603.2020.1853352Deep Air Quality Forecasts: Suspended Particulate Matter Modeling With Convolutional Neural and Long Short-Term Memory Networks
Sharma, Ekta, Deo, Ravinesh C., Prasad, Ramendra, Parisi, Alfio and Raj, Nawin. 2020. "Deep Air Quality Forecasts: Suspended Particulate Matter Modeling With Convolutional Neural and Long Short-Term Memory Networks." IEEE Access. 8, pp. 209503-209516. https://doi.org/10.1109/ACCESS.2020.3039002Low cost and centimeter-level global positioning system accuracy using real-time kinematic library and real-time kinematic gpsa
Gianey, Hemant K., Ali, Mumtaz, Vijayakumar, Varadarajan, Sharma, Ashutosh and Kumar, Rajiv. 2021. "Low cost and centimeter-level global positioning system accuracy using real-time kinematic library and real-time kinematic gpsa." Recent Advances in Computer Science and Communications. 14 (2), pp. 360-367. https://doi.org/10.2174/2213275912666190328201322Variational mode decomposition based random forest model for solar radiation forecasting: New emerging machine learning technology
Ali, Mumtaz, Prasad, Ramendra, Xiang, Yong, Khan, Mohsin, Farooque, Aitazaz Ahsan, Zong, Tianrui and Yaseen, Zaher Mundher. 2021. "Variational mode decomposition based random forest model for solar radiation forecasting: New emerging machine learning technology." Energy Reports. 7, pp. 6700-6717. https://doi.org/10.1016/j.egyr.2021.09.113Forecasting standardized precipitation index using data intelligence models: regional investigation of Bangladesh
Yaseen, Zaher Mundher, Ali, Mumtaz, Sharafati, Ahmad, Al-Ansari, Nadhir and Shahid, Shamsuddin. 2021. "Forecasting standardized precipitation index using data intelligence models: regional investigation of Bangladesh." Scientific Reports. 11 (1), pp. 1-25. https://doi.org/10.1038/s41598-021-82977-9Multifractal characterization and cross correlations of reference evapotranspiration time series of India
Adarsh, S., Nityanjaly, L. J., Pham, Quoc Bao, Sarang, R., Ali, Mumtaz and Nandhineekrishna, P.. 2021. "Multifractal characterization and cross correlations of reference evapotranspiration time series of India." European Physical Journal: Special Topics. 230 (21-22), pp. 3845-3859. https://doi.org/10.1140/epjs/s11734-021-00325-4Self-supervised cross-iterative clustering for unlabeled plant disease images
Fang, Uno, Li, J., Lu, X., Gao, Longxiang, Ali, Mumtaz and Xiang, Yong. 2021. "Self-supervised cross-iterative clustering for unlabeled plant disease images." Neurocomputing. 456, pp. 36-48. https://doi.org/10.1016/j.neucom.2021.05.066Spatiotemporal variability of multifractal properties of fineresolution daily gridded rainfall fields over India
Sankaran, Adarsh, Chavan, Sagar Rohidas, Ali, Mumtaz, Devarajan, Archana Devarajan, Dharan, Drisya Sasi and Khan, Muhammad Ismail. 2021. "Spatiotemporal variability of multifractal properties of fineresolution daily gridded rainfall fields over India." Natural Hazards. 106 (3), pp. 1951-1979. https://doi.org/10.1007/s11069-021-04523-0MARS model for prediction of short- and long-term global solar radiation
Balalla, Dilki T., Nguyen-Huy, Thong and Deo, Ravinesh. 2021. "MARS model for prediction of short- and long-term global solar radiation." Deo, Ravinesh, Roy, Sanjiban Sekhar and Samui, Pijush (ed.) Predictive Modelling for Energy Management and Power Systems Engineering. United Kingdom. Elsevier. pp. 391-436Developing reservoir evaporation predictive model for successful dam management
Allawi, Mohammed Falah, Ahmed, Mohammed Lateef, Aidan, Ibraheem Abdallah, Deo, Ravinesh C. and El-Shafie, Ahmed. 2021. "Developing reservoir evaporation predictive model for successful dam management." Stochastic Environmental Research and Risk Assessment. 35 (2), pp. 499-514. https://doi.org/10.1007/s00477-020-01918-6Application of effective drought index for quantification of meteorological drought events: a case study in Australia
Deo, Ravinesh C., Byun, Hi-Ryong, Adamowski, Jan F. and Begum, Khaleda. 2017. "Application of effective drought index for quantification of meteorological drought events: a case study in Australia." Theoretical and Applied Climatology. 128 (1-2), pp. 359-379. https://doi.org/10.1007/s00704-015-1706-5Feedback modelling of the impacts of drought: A case study in coffee production systems in Viet Nam
Pham, Yen, Reardon-Smith, Kathryn, Mushtaq, Shahbaz and Deo, Ravinesh C.. 2020. "Feedback modelling of the impacts of drought: A case study in coffee production systems in Viet Nam." Climate Risk Management. 30, pp. 1-17. https://doi.org/10.1016/j.crm.2020.100255Forecasting long-term precipitation for water resource management: a new multi-step data-intelligent modelling approach
Ali, Mumtaz, Deo, Ravinesh C., Xiang, Yong, Li, Ya and Yaseen, Zaher Mundher. 2020. "Forecasting long-term precipitation for water resource management: a new multi-step data-intelligent modelling approach." Hydrological Sciences Journal. 65 (16), pp. 2693-2708. https://doi.org/10.1080/02626667.2020.1808219Keratinocyte skin cancer risks for working school teachers: scenarios and implications of the timing of scheduled duty periods in Queensland, Australia
Dexter, B. R., King, R., Parisi, A. V., Harrison, S. L., Konovalov, D. A. and Downs, N. J.. 2020. "Keratinocyte skin cancer risks for working school teachers: scenarios and implications of the timing of scheduled duty periods in Queensland, Australia." Journal of Photochemistry and Photobiology, B: Biology. 213, pp. 1-10. https://doi.org/10.1016/j.jphotobiol.2020.112046Development of Flood Monitoring Index for daily flood risk evaluation: case studies in Fiji
Moishin, Mohammed, Deo, Ravinesh C., Prasad, Ramendra, Raj, Nawin and Abdulla, Shahab. 2021. "Development of Flood Monitoring Index for daily flood risk evaluation: case studies in Fiji." Stochastic Environmental Research and Risk Assessment. 35 (7), pp. 1387-1402. https://doi.org/10.1007/s00477-020-01899-6The modeling of human facial pain intensity based on Temporal Convolutional Networks trained with video frames in HSV color space
Bargshady, Ghazal, Zhou, Xujuan, Deo, Ravinesh C., Soar, Jeffrey, Whittaker, Frank and Wang, Hua. 2020. "The modeling of human facial pain intensity based on Temporal Convolutional Networks trained with video frames in HSV color space." Applied Soft Computing. 97 (Part A), pp. 1-14. https://doi.org/10.1016/j.asoc.2020.106805Modeling wheat yield with data-intelligent algorithms: artificial neural network versus genetic programming and minimax probability machine regression
Ali, Mumtaz and Deo, Ravinesh C.. 2020. "Modeling wheat yield with data-intelligent algorithms: artificial neural network versus genetic programming and minimax probability machine regression." Samui, Pijush, Bui, Dieu Tien, Chakraborty, Subrata and Deo, Ravinesh C. (ed.) Handbook of probabilistic models. Oxford, United Kingdom. Elsevier. pp. 37-87MARS model for prediction of short- and long-term global solar radiation
Balalla, Dilki T., Nguyen-Huy, Thong and Deo, Ravinesh. 2021. "MARS model for prediction of short- and long-term global solar radiation." Deo, Ravinesh, Samui, Pijush and Roy, Sanjiban Sekhar (ed.) Predictive modelling for energy management and power systems engineering. Amsterdam, Netherlands. Elsevier. pp. 391-436Short-term electrical energy demand prediction under heat island effects using emotional neural network integrated with genetic algorithm
Karalasingham, Sagthitharan, Deo, Ravinesh and Prasad, Ramendra. 2021. "Short-term electrical energy demand prediction under heat island effects using emotional neural network integrated with genetic algorithm." Deo, Ravinesh, Samui, Pijush and Roy, Sanjiban Sekhar (ed.) Predictive modelling for energy management and power systems engineering. Amsterdam, Netherlands. Elsevier. pp. 271-298Hybrid multilayer perceptron-firefly optimizer algorithm for modelling photosynthetic active solar radiation for biofuel energy exploration
Goundar, Harshna, Yaseen, Zaher Mundher and Deo, Ravinesh. 2021. "Hybrid multilayer perceptron-firefly optimizer algorithm for modelling photosynthetic active solar radiation for biofuel energy exploration." Deo, Ravinesh, Samui, Pijush and Roy, Sanjiban Sekhar (ed.) Predictive modelling for energy management and power systems engineering. Amsterdam, Netherlands. Elsevier. pp. 191-232Design and performance of two decomposition paradigms in forecasting daily solar radiation with evolutionary polynomial regression: wavelet transform versus ensemble empirical mode decomposition
Rezaie-Balf, Mohammad, Kim, Sungwon, Ghaemi, Alireza and Deo, Ravinesh. 2021. "Design and performance of two decomposition paradigms in forecasting daily solar radiation with evolutionary polynomial regression: wavelet transform versus ensemble empirical mode decomposition." Deo, Ravinesh, Samui, Pijush and Roy, Sanjiban Sekhar (ed.) Predictive modelling for energy management and power systems engineering. Amsterdam, Netherlands. Elsevier. pp. 115-142Shade auditing in Queensland
Downs, Nathan, Parisi, Alfio, Baldwin, Louise and Harrison, Simone. 2019. "Shade auditing in Queensland." 6th Annual Brisbane Cancer Conference. Brisbane, Australia 28 - 29 Nov 2019Experimental Study on the Rainfall-Runoff Responses of Typical Urban Surfaces and Two Green Infrastructures Using Scale-Based Models
Liu, Wen, Feng, Qi, Deo, Ravinesh C., Yao, Lei and Wei, Wei. 2020. "Experimental Study on the Rainfall-Runoff Responses of Typical Urban Surfaces and Two Green Infrastructures Using Scale-Based Models ." Environmental Management (New York): an international journal for decision-makers, scientists and environmental auditors. 66 (4), pp. 683-693. https://doi.org/10.1007/s00267-020-01339-9Multifractal Cross Correlation Analysis of Agro-Meteorological Datasets (Including Reference Evapotranspiration) of California, United States
Sankaran, A., Krzyszczak, Jaromir, Baranowski, Piotr, Devarajan Sindhu, Archana, Nandhineekrishna Pradeep, Kumar, Lija Jayaprakash, Nityanjali, Thankamani, Vandana and Ali, Mumtaz. 2020. "Multifractal Cross Correlation Analysis of Agro-Meteorological Datasets (Including Reference Evapotranspiration) of California, United States." Atmosphere. 11 (10), pp. 1-24. https://doi.org/10.3390/atmos11101116Ensemble neural network approach detecting pain intensity from facial expressions
Bargshady, Ghazal, Zhou, Xujuan, Deo, Ravinesh C., Soar, Jeffrey, Whittaker, Frank and Wang, Hua. 2020. "Ensemble neural network approach detecting pain intensity from facial expressions." Artificial Intelligence in Medicine. 109, pp. 1-12. https://doi.org/10.1016/j.artmed.2020.101954Probabilistic and artificial intelligence modelling of drought and agricultural crop yield in Pakistan
Ali, Mumtaz. 2019. Probabilistic and artificial intelligence modelling of drought and agricultural crop yield in Pakistan. PhD Thesis Doctor of Philosophy. University of Southern Queensland. https://doi.org/10.26192/S847-M467Daily flood forecasts with intelligent data analytic models: multivariate empirical mode decomposition-based modeling methods
Prasad, Ramendra, Charan, Dhrishna, Joseph, Lionel, Nguyen-Huy, Thong, Deo, Ravinesh C. and Singh, Sanjay. 2021. "Daily flood forecasts with intelligent data analytic models: multivariate empirical mode decomposition-based modeling methods." Deo, Ravinesh C., Samui, Pijush, Kisi, Ozgur and Yaseen, Zaher Mundher (ed.) Intelligent data analytics for decision-support systems in hazard mitigation: theory and practice of hazard mitigation. Singapore. Springer. pp. 359-381Artificial neural networks for prediction of Steadman Heat Index
Chand, Bhuwan, Nguyen-Huy, Thong and Deo, Ravinesh C.. 2021. "Artificial neural networks for prediction of Steadman Heat Index." Deo, Ravinesh C., Samui, Pijush, Kisi, Ozgur and Yaseen, Zaher Mundher (ed.) Intelligent data analytics for decision-support systems in hazard mitigation: theory and practice of hazard mitigation. Singapore. Springer. pp. 293-357Spatial prediction of landslide susceptibility using random forest algorithm
Rahmati, Omid, Kornejady, Aiding and Deo, Ravinesh C.. 2021. "Spatial prediction of landslide susceptibility using random forest algorithm." Deo, Ravinesh C., Samui, Pijush, Kisi, Ozgur and Yaseen, Zaher Mundher (ed.) Intelligent data analytics for decision-support systems in hazard mitigation: theory and practice of hazard mitigation. Singapore. Springer. pp. 281-292Intelligent data analytics for time series, trend analysis and drought indices comparison
Dayal, Kavina S., Deo, Ravinesh C. and Apan, Armando A.. 2021. "Intelligent data analytics for time series, trend analysis and drought indices comparison." Deo, Ravinesh C., Samui, Pijush, Kisi, Ozgur and Yaseen, Zaher Mundher (ed.) Intelligent data analytics for decision-support systems in hazard mitigation: theory and practice of hazard mitigation. Singapore. Springer. pp. 151-169Modulation of tropical cyclone genesis by Madden–Julian Oscillation in the Southern Hemisphere
Dayal, Kavina S., Wang, Bin and Deo, Ravinesh C.. 2021. "Modulation of tropical cyclone genesis by Madden–Julian Oscillation in the Southern Hemisphere." Deo, Ravinesh C., Samui, Pijush, Kisi, Ozgur and Yaseen, Zaher Mundher (ed.) Intelligent data analytics for decision-support systems in hazard mitigation: theory and practice of hazard mitigation. Singapore. Springer. pp. 127-150Bayesian Markov Chain Monte Carlo-based copulas: factoring the role of large-scale climate indices in monthly flood prediction
Nguyen-Huy, Thong, Deo, Ravinesh C., Yaseen, Zaher Mundher, Mushtaq, Shahbaz and Prasad, Ramendra. 2021. "Bayesian Markov Chain Monte Carlo-based copulas: factoring the role of large-scale climate indices in monthly flood prediction." Deo, Ravinesh C., Samui, Pijush, Kisi, Ozgur and Yaseen, Zaher Mundher (ed.) Intelligent data analytics for decision-support systems in hazard mitigation: theory and practice of hazard mitigation. Singapore. Springer. pp. 29-47Intelligent data analytics for decision-support systems in hazard mitigation: theory and practice of hazard mitigation
Deo, Ravinesh C., Samui, Pijush, Kisi, Ozgur and Yaseen, Zaher Mundher. 2021. Intelligent data analytics for decision-support systems in hazard mitigation: theory and practice of hazard mitigation. Singapore. Springer.Solar blue light radiation enhancement during mid to low solar elevation periods under cloud affected skies
Parisi, Alfio V., Igoe, Damien P., Amar, Abdurazaq and Downs, Nathan. 2020. "Solar blue light radiation enhancement during mid to low solar elevation periods under cloud affected skies." Sensors. 20 (15), pp. 1-13. https://doi.org/10.3390/s20154105Electronic Sun Journals: integrating calibrated field radiometers and traditional dosimeters for monitoring personal sun exposure behavior
Downs, Nathan. 2018. "Electronic Sun Journals: integrating calibrated field radiometers and traditional dosimeters for monitoring personal sun exposure behavior." 2018 National Cancer Institute Exercise Science and Skin Cancer Prevention Research Meeting. Rockville, United States 26 - 27 Sep 2018Near real-time significant wave height forecasting with hybridized multiple linear regression algorithms
Ali, Mumtaz, Prasad, Ramendra, Xiang, Yong and Deo, Ravinesh C.. 2020. "Near real-time significant wave height forecasting with hybridized multiple linear regression algorithms." Renewable and Sustainable Energy Reviews. 132. https://doi.org/10.1016/j.rser.2020.110003Electrical Energy Demand Forecasting Model Development and Evaluation with Maximum Overlap Discrete Wavelet Transform-Online Sequential Extreme Learning Machines Algorithms
Al-Musaylh, Mohanad S., Deo, Ravinesh C. and Li, Yan. 2020. "Electrical Energy Demand Forecasting Model Development and Evaluation with Maximum Overlap Discrete Wavelet Transform-Online Sequential Extreme Learning Machines Algorithms." Energies. 13 (9). https://doi.org/10.3390/en13092307Arithmetic Operations of Neutrosophic Sets, Interval Neutrosophic Sets and Rough Neutrosophic Sets
Smarandache, Florentin, Ali, Mumtaz and Khan, Mohsin. 2019. "Arithmetic Operations of Neutrosophic Sets, Interval Neutrosophic Sets and Rough Neutrosophic Sets." Kahraman, Cengiz and Otay, Irem (ed.) Fuzzy Multi-criteria Decision-Making Using Neutrosophic Sets. Cham, Switzerland. Springer. pp. 25-42Near real-time global solar radiation forecasting at multiple time-step horizons using the long short-term memory network
Huynh, Anh Ngoc‐Lan, Deo, Ravinesh C., An-Vo, Duc-Anh, Ali, Mumtaz, Raj, Nawin and Abdulla, Shahab. 2020. "Near real-time global solar radiation forecasting at multiple time-step horizons using the long short-term memory network." Energies. 13 (14). https://doi.org/10.3390/en13143517Adaptive boost LS-SVM classification approach for time-series signal classification in epileptic seizure diagnosis applications
Al-Hadeethi, Hanan, Abdulla, Shahab, Diykh, Mohammed, Deo, Ravinesh C. and Green, Jonathan H.. 2020. "Adaptive boost LS-SVM classification approach for time-series signal classification in epileptic seizure diagnosis applications." Expert Systems with Applications. 161. https://doi.org/10.1016/j.eswa.2020.113676Random forest predictive model development with uncertainty analysis capability for the estimation of evapotranspiration in an arid oasis region
Wu, Min, Feng, Qi, Wen, Xiaohu, Deo, Ravinesh C., Yin, Zhenliang, Yang, Linshan and Sheng, Danrui. 2020. "Random forest predictive model development with uncertainty analysis capability for the estimation of evapotranspiration in an arid oasis region." Hydrology Research: an international journal. 51 (4), pp. 648-665. https://doi.org/10.2166/nh.2020.012Measured UV Exposures of Ironman, Sprint and Olympic-Distance Triathlon Competitors
Downs, Nathan J., Axelsen, Taryn, Parisi, Alfio V., Schouten, Peter W. and Dexter, Ben R.. 2020. "Measured UV Exposures of Ironman, Sprint and Olympic-Distance Triathlon Competitors." Atmosphere. 11 (5), pp. 1-15. https://doi.org/10.3390/atmos11050440Evaluation of shade profiles while walking in urban environments: A case study from inner suburban Sydney, Australia
Igoe, Damien P., Downs, Nathan J., Parisi, Alfio V. and Amar, Abdurazaq. 2020. "Evaluation of shade profiles while walking in urban environments: A case study from inner suburban Sydney, Australia." Building and Environment. 177, pp. 1-7. https://doi.org/10.1016/j.buildenv.2020.106873Stormwater runoff and pollution retention performances of permeable pavements and the effects of structural factors
Liu, Wen, Feng, Qi, Chen, Weiping and Deo, Ravinesh C.. 2020. "Stormwater runoff and pollution retention performances of permeable pavements and the effects of structural factors." Environmental Science and Pollution Research. 27 (24), pp. 30831-30843. https://doi.org/10.1007/s11356-020-09220-2Spatial mapping of short-term solar radiation prediction incorporating geostationary satellite images coupled with deep convolutional LSTM networks for South Korea
Yeom, Jong-Min, Deo, Ravinesh C., Adamowski, Jan F., Park, Seonyoung and Lee, Chang-Suk. 2020. "Spatial mapping of short-term solar radiation prediction incorporating geostationary satellite images coupled with deep convolutional LSTM networks for South Korea." Environmental Research Letters. 15 (9), pp. 1-10. https://doi.org/10.1088/1748-9326/ab9467Development of Advanced Computer Aid Model for Shear Strength of Concrete Slender Beam Prediction
Sharafati, Ahmad, Haghbin, Masoud, Aldlemy, Mohammed Suleman, Mussa, Mohamed H., Al Zand, Ahmed W., Ali, Mumtaz, Bhagat, Suraj Kumar, Al-Ansari, Nadhir and Yaseen, Zaher Mundher. 2020. "Development of Advanced Computer Aid Model for Shear Strength of Concrete Slender Beam Prediction." Applied Sciences. 10 (11), pp. 1-25. https://doi.org/10.3390/app10113811A double decomposition-based modelling approach to forecast weekly solar radiation
Prasad, Ramendra, Ali, Mumtaz, Xiang, Yong and Khan, Huma. 2020. "A double decomposition-based modelling approach to forecast weekly solar radiation." Renewable Energy. 152, pp. 9-22. https://doi.org/10.1016/j.renene.2020.01.005Integrative stochastic model standardization with genetic algorithm for rainfall pattern forecasting in tropical and semi-arid environments
Salih, Sinan Q., Sharafati, Ahmad, Ebtehaj, Isa, Sanikhani, Hadi, Siddique, Ridwan, Deo, Ravinesh C., Bonakdari, Hossein, Shahid, Shamsuddin and Yaseen, Zaher Mundher. 2020. "Integrative stochastic model standardization with genetic algorithm for rainfall pattern forecasting in tropical and semi-arid environments." Hydrological Sciences Journal. 65 (7), pp. 1145-1157. https://doi.org/10.1080/02626667.2020.1734813Hybridized neural fuzzy ensembles for dust source modeling and prediction
Rahmati, Omid, Panahi, Mahdi, Ghiasi, Seid Saeid, Deo, Ravinesh C., Tiefenbacher, John P., Pradhan, Biswajeet, Jahani, Ali, Goshtasb, Hamid, Kornejady, Aiding, Shahabi, Himan, Shirzadi, Ataollah, Khosravi, Hassan, Moghaddam, Davoud Davoudi, Mohtashamian, Maryamsadat and Bui, Dieu Tien. 2020. "Hybridized neural fuzzy ensembles for dust source modeling and prediction." Atmospheric Environment. 224, pp. 1-11. https://doi.org/10.1016/j.atmosenv.2020.117320Global solar radiation estimation and climatic variability analysis using extreme learning machine based predictive model
Tao, Hai, Sharafati, Ahmad, Mohammed, Achite, Salih, Sinan Q., Deo, Ravinesh C., Al-Ansari, Nadhir and Yaseen, Zaher Mundher. 2020. "Global solar radiation estimation and climatic variability analysis using extreme learning machine based predictive model." IEEE Access. 8, pp. 12026-12042. https://doi.org/10.1109/ACCESS.2020.2965303Development and evaluation of the cascade correlation neural network and the random forest models for river stage and river flow prediction in Australia
Ghorbani, Mohammad Ali, Deo, Ravinesh C., Kim, Sungwon, Kashani, Mahasa Hasanpour, Karimi, Vahid and Izadkhah, Maryam. 2020. "Development and evaluation of the cascade correlation neural network and the random forest models for river stage and river flow prediction in Australia." Soft Computing. 24, pp. 12079-12090. https://doi.org/10.1007/s00500-019-04648-2Causality of climate, food production and conflict over the last two millennia in the Hexi Corridor, China
Yang, Linshan, Feng, Qi, Adamowski, Jan F., Deo, Ravinesh C., Yin, Zhenlaing, Wen, Xiaohu, Tang, Xia and Wu, Min. 2020. "Causality of climate, food production and conflict over the last two millennia in the Hexi Corridor, China." Science of the Total Environment. 713, pp. 1-11. https://doi.org/10.1016/j.scitotenv.2020.136587Mixing characteristics of a film-exciting flapping jet
Wu, M., Xu, M., Mi, J. and Deo, R. C.. 2020. "Mixing characteristics of a film-exciting flapping jet." International Journal of Heat and Fluid Flow. 82, pp. 1-9. https://doi.org/10.1016/j.ijheatfluidflow.2019.108532Influence of Clouds on OMI Satellite Total Daily UVA Exposure over a 12-year Period at a Southern Hemisphere Site
A Jebar, Mustapha A., Parisi, Alfio V., Downs, Nathan J. and Turner, Joanna. 2020. "Influence of Clouds on OMI Satellite Total Daily UVA Exposure over a 12-year Period at a Southern Hemisphere Site." International Journal of Remote Sensing. 41 (1), pp. 272-283. https://doi.org/10.1080/01431161.2019.1641243Enhanced deep learning algorithm development to detect pain intensity from facial expression images
Bargshady, Ghazal, Zhou, Xujuan, Deo, Ravinesh C., Soar, Jeffery, Whittaker, Frank and Wang, Hua. 2020. "Enhanced deep learning algorithm development to detect pain intensity from facial expression images." Expert Systems with Applications. 149, pp. 1-10. https://doi.org/10.1016/j.eswa.2020.113305A general extensible learning approach for multi-disease recommendations in a telehealth environment
Lafta, Raid, Zhang, Ji, Tao, Xiaohui, Zhu, Xiaodong, Li, Hongzhou, Chang, Liang and Deo, Ravinesh. 2020. "A general extensible learning approach for multi-disease recommendations in a telehealth environment ." Pattern Recognition Letters. 132, pp. 106-114. https://doi.org/10.1016/j.patrec.2018.11.006A hybrid air quality early-warning framework: an hourly forecasting model with online sequential extreme learning machines and empirical mode decomposition algorithms
Sharma, Ekta, Deo, Ravinesh C., Prasad, Ramendra and Parisi, Alfio V.. 2020. "A hybrid air quality early-warning framework: an hourly forecasting model with online sequential extreme learning machines and empirical mode decomposition algorithms." Science of the Total Environment. 709, pp. 1-23. https://doi.org/10.1016/j.scitotenv.2019.135934Machine learning approaches for spatial modeling of agricultural droughts in the south-east region of Queensland Australia
Rahmati, Omid, Falah, Fatemeh, Dayal, Kavina Shaanu, Deo, Ravinesh C., Mohammadi, Farnoush, Biggs, Trent, Moghaddam, Davoud Davoudi, Naghibi, Seyed Amir and Bui, Dieu Tien. 2020. "Machine learning approaches for spatial modeling of agricultural droughts in the south-east region of Queensland Australia." Science of the Total Environment. 699 (134230). https://doi.org/10.1016/j.scitotenv.2019.134230M-CFIS-R: Mamdani Complex Fuzzy Inference System with Rule Reduction Using Complex Fuzzy Measures in Granular Computing
Tuan, Tran Manh, Lan, Luong Thi Hong, Chou, Shuo-Yan, Ngan, Tran Thi, Son, Le Hoang, Giang, Nguyen Long and Ali, Mumtaz. 2020. "M-CFIS-R: Mamdani Complex Fuzzy Inference System with Rule Reduction Using Complex Fuzzy Measures in Granular Computing." Mathematics. 8 (5), pp. 1-24. https://doi.org/10.3390/math8050707Complete ensemble empirical mode decomposition hybridized with random forest and kernel ridge regression model for monthly rainfall forecasts
Ali, Mumtaz, Prasad, Ramendra, Xiang, Yong and Yaseen, Z.. 2020. "Complete ensemble empirical mode decomposition hybridized with random forest and kernel ridge regression model for monthly rainfall forecasts." Journal of Hydrology. 584, pp. 1-15. https://doi.org/10.1016/j.jhydrol.2020.124647The Generalized Neutrosophic Cubic Aggregation Operators and Their Application to Multi-Expert Decision-Making Method
Khan, Majid, Gulistan, Muhammad, Ali, Mumtaz and Chammam, Wathek. 2020. "The Generalized Neutrosophic Cubic Aggregation Operators and Their Application to Multi-Expert Decision-Making Method." Symmetry. 12 (4), pp. 1-15. https://doi.org/10.3390/sym12040496The Simulated Ocular and Whole-Body Distribution of Natural Sunlight to Kiteboarders: A High-Risk Case of UVR Exposure for Athletes Utilizing Water Surfaces in Sport
Downs, Nathan J., Parisi, Alfio V., Schouten, Peter W., Igoe, Damien P. and De Castro-Maqueda, Guillermo. 2020. "The Simulated Ocular and Whole-Body Distribution of Natural Sunlight to Kiteboarders: A High-Risk Case of UVR Exposure for Athletes Utilizing Water Surfaces in Sport." Photochemistry and Photobiology. 96 (4), pp. 926-935. https://doi.org/10.1111/PHP.13200Beyond the current Smartphone Application: Using smartphone hardware to measure UV radiation
Turner, J., Igoe, D. P., Parisi, A. V., Downs, N. J. and Amar, A.. 2018. "Beyond the current Smartphone Application: Using smartphone hardware to measure UV radiation." NIWA UV Workshop 2018 - UV Radiation: Effects on Human Health and the Environment. Wellington, New Zealand 04 - 06 Apr 2018 New Zealand.Projected spatial patterns in precipitation and air temperature for China's northwest region derived from high‐resolution regional climate models
Yin, Zhenliang, Feng, Qi, Yang, Linshan, Deo, Ravinesh C., Adamowski, Jan F., Wen, Xiaohu, Jia, Bing and Si, Jianhua. 2020. "Projected spatial patterns in precipitation and air temperature for China's northwest region derived from high‐resolution regional climate models." International Journal of Climatology. 40 (8), pp. 3922-3941. https://doi.org/10.1002/joc.6435Exploring solar and wind energy resources in North Korea with COMS MI geostationary satellite data coupled with numerical weather prediction reanalysis variables
Yeom, Jong-Min, Deo, Ravinesh, Adamowski, Jan F., Chae, Taebyeong, Kim, Dong-Su, Han, Kyung-Soo and Kim, Do-Yong. 2020. "Exploring solar and wind energy resources in North Korea with COMS MI geostationary satellite data coupled with numerical weather prediction reanalysis variables." Renewable and Sustainable Energy Reviews. 119, pp. 1-14. https://doi.org/10.1016/j.rser.2019.109570Capability and robustness of novel hybridized models used for drought hazard modeling in southeast Queensland, Australia
Rahmati, Omid, Panahi, Mahdi, Kalantari, Zahra, Soltani, Elinaz, Falah, Fatemeh, Dayal, Kavina S., Mohammadi, Farnoush, Deo, Ravinesh C., Tiefenbacher, John and Bui, Dieu Tien. 2020. "Capability and robustness of novel hybridized models used for drought hazard modeling in southeast Queensland, Australia." Science of the Total Environment. 718, pp. 1-17. https://doi.org/10.1016/j.scitotenv.2019.134656Regional hydrology heterogeneity and the response to climate and land surface changes in arid alpine basin, northwest China
Yang, Linshan, Feng, Qi, Yin, Zhenliang, Deo, Ravinesh C., Wen, Xiaohu, Si, Jianhua and Liu, Wen. 2020. "Regional hydrology heterogeneity and the response to climate and land surface changes in arid alpine basin, northwest China." Catena. 187. https://doi.org/10.1016/j.catena.2019.104345A site-specific standard for comparing dynamic solar ultraviolet protection characteristics of established tree canopies
Downs, N. J., Butler, H. J., Baldwin, L., Parisi, A. V., Amar, A., Vanos, J. and Harrison, S.. 2019. "A site-specific standard for comparing dynamic solar ultraviolet protection characteristics of established tree canopies." MethodsX. 6, pp. 1683-1693. https://doi.org/10.1016/j.mex.2019.07.013Seasonal Minimum and Maximum Solar Ultraviolet Exposure Measurements of Classroom Teachers Residing in Tropical North Queensland, Australia
Downs, Nathan J., Igoe, Damien P., Parisi, Alfio V., Taylor, Olivia, Lazzaroni, Shari L., Rawlings, Alex, Garzon-Chavez, Daniel R. and Harrison, Simone L.. 2019. "Seasonal Minimum and Maximum Solar Ultraviolet Exposure Measurements of Classroom Teachers Residing in Tropical North Queensland, Australia." Photochemistry and Photobiology. 95, pp. 1083-1093. https://doi.org/10.1111/php.13081An ensemble tree-based machine learning model for predicting the uniaxial compressive strength of travertine rocks
Barzegar, Rahim, Sattarpour, Masoud, Deo, Ravinesh, Fijani, Elham and Adamowski, Jan. 2020. "An ensemble tree-based machine learning model for predicting the uniaxial compressive strength of travertine rocks." Neural Computing and Applications. 32 (13), pp. 9065-9080. https://doi.org/10.1007/s00521-019-04418-zPrediction of evaporation in arid and semi-arid regions: a comparative study using different machine learning models
Yaseen, Zaher Mundher, Al-Juboori, Al-Juboori, Beyaztasc, Ufuk, Al-Ansari, Nadhir, Chau, Kwok-Wing, Qi, Chongchong, Ali, Mumtaz, Salih, Sinan Q. and Shahid, Shamsuddin. 2020. "Prediction of evaporation in arid and semi-arid regions: a comparative study using different machine learning models." Engineering Applications of Computational Fluid Mechanics. 14 (1), pp. 70-89. https://doi.org/10.1080/19942060.2019.1680576Modern artificial intelligence model development for undergraduate student performance prediction: an investigation on engineering mathematics courses
Deo, Ravinesh C., Yaseen, Zaher Mundher, Al-Ansari, Nadhir, Nguyen-Huy, Thong, Langlands, Trevor and Galligan, Linda. 2020. "Modern artificial intelligence model development for undergraduate student performance prediction: an investigation on engineering mathematics courses." IEEE Access. 8, pp. 136697-136724. https://doi.org/10.1109/ACCESS.2020.3010938Correcting satellite precipitation data and assimilating satellite-derived soil moisture data to generate ensemble hydrological forecasts within the HBV rainfall-runoff model
Ciupak, Maurycy, Ozga-Zielinski, Bogdan, Adamowski, Jan, Deo, Ravinesh C and Kochanek, Krzysztof. 2019. "Correcting satellite precipitation data and assimilating satellite-derived soil moisture data to generate ensemble hydrological forecasts within the HBV rainfall-runoff model." Water: an open access journal. 11 (10), pp. 1-31. https://doi.org/10.3390/w11102138Partitioning groundwater recharge sources in multiple aquifers system within a desert oasis environment: Implications for water resources management in endorheic basins
Guo, Xiaoyan, Feng, Qi, Si, Jianhua, Xi, Haiyang, Zhao, Yan and Deo, Ravinesh C.. 2019. "Partitioning groundwater recharge sources in multiple aquifers system within a desert oasis environment: Implications for water resources management in endorheic basins." Journal of Hydrology. 579, pp. 1-11. https://doi.org/10.1016/j.jhydrol.2019.124212Probabilistic seasonal rainfall forecasts using semiparametric d-vine copula-based quantile regression
Nguyen-Huy, Thong, Deo, Ravinesh C., Mushtaq, Shahbaz and Khan, Shahjahan. 2020. "Probabilistic seasonal rainfall forecasts using semiparametric d-vine copula-based quantile regression." Samui, Pijush, Bui, Dieu Tien, Chakraborty, Subrata and Deo, Ravinesh C. (ed.) Handbook of probabilistic models. Oxford, United Kingdom. Elsevier. pp. 203-227Monthly rainfall forecasting with Markov Chain Monte Carlo simulations integrated with statistical bivariate copulas
Ali, Mumtaz, Deo, Ravinesh C., Downs, Nathan J. and Maraseni, Tek. 2020. "Monthly rainfall forecasting with Markov Chain Monte Carlo simulations integrated with statistical bivariate copulas." Samui, Pijush, Bui, Dieu Tien, Chakraborty, Subrata and Deo, Ravinesh C. (ed.) Handbook of probabilistic models. Oxford, United Kingdom. Elsevier. pp. 89-105Development of copula statistical drought prediction model using the Standardized Precipitation-Evapotranspiration Index
Dayal, Kavina S., Deo, Ravinesh C. and Apan, Armando A.. 2020. "Development of copula statistical drought prediction model using the Standardized Precipitation-Evapotranspiration Index." Samui, Pijush, Bui, Dieu Tien, Chakraborty, Subrata and Deo, Ravinesh C. (ed.) Handbook of probabilistic models. Oxford, United Kingdom. Elsevier. pp. 141-178Soil organic carbon in semiarid alpine regions: the spatial distribution, stock estimation, and environmental controls
Zhu, Meng, Feng, Qi, Zhang, Mengxu, Liu, Wei, Deo, Ravinesh C., Zhang, Chengqi and Yang, Linshan. 2019. "Soil organic carbon in semiarid alpine regions: the spatial distribution, stock estimation, and environmental controls." Journal of Soils and Sediments: protection, risk assessment and remediation. 19 (10), pp. 3427-3441. https://doi.org/10.1007/s11368-019-02295-6Controlling factors of plant community composition with respect to the slope aspect gradient in the Qilian Mountains
Qin, Yanyan, Adamowski, Jan F., Deo, Ravinesh C., Hu, Zeyong, Cao, Jianjun, Zhu, Meng and Feng, Qi. 2019. "Controlling factors of plant community composition with respect to the slope aspect gradient in the Qilian Mountains." Ecosphere. 10 (9), pp. 1-13. https://doi.org/10.1002/ecs2.2851Grassland degradation on the Qinghai-Tibetan Plateau: reevaluation of causative factors
Cao, Jianjun, Adamowski, Jan F., Deo, Ravinesh C., Xu, Xueyun, Gong, Yifan and Feng, Qi. 2019. "Grassland degradation on the Qinghai-Tibetan Plateau: reevaluation of causative factors." Rangeland Ecology and Management. 72 (6), pp. 988-995. https://doi.org/10.1016/j.rama.2019.06.001Fractal dimension undirected correlation graph-based support vector machine model for identification of focal and non-focal electroencephalography signals
Diykh, Mohammed, Abdulla, Shahab, Saleh, Khalid and Deo, Ravinesh C.. 2019. "Fractal dimension undirected correlation graph-based support vector machine model for identification of focal and non-focal electroencephalography signals." Biomedical Signal Processing and Control. 54, pp. 1-10. https://doi.org/10.1016/j.bspc.2019.101611The impacts of substrate and vegetation on stormwater runoff quality from extensive green roofs
Liu, Wen, Wei, Wei, Chen, Weiping, Deo, Ravinesh C., Si, Jianhua, Xi, Haiyang, Li, Baofeng and Feng, Qi. 2019. "The impacts of substrate and vegetation on stormwater runoff quality from extensive green roofs." Journal of Hydrology. 576, pp. 575-582. https://doi.org/10.1016/j.jhydrol.2019.06.061Application of multivariate recursive nesting bias correction, multiscale wavelet entropy and AI-based models to improve future precipitation projection in upstream of the Heihe River, Northwest China
Yang, Linshan, Feng, Qi, Yin, Zhenliang, Wen, Xiaohu, Deo, Ravinesh C., Si, Jianhua and Li, Changbin. 2019. "Application of multivariate recursive nesting bias correction, multiscale wavelet entropy and AI-based models to improve future precipitation projection in upstream of the Heihe River, Northwest China." Theoretical and Applied Climatology. 137 (1-2), pp. 323-339. https://doi.org/10.1007/s00704-018-2598-yWavelet-based 3-phase hybrid SVR model trained with satellite-derived predictors, particle swarm optimization and maximum overlap discrete wavelet transform for solar radiation prediction
Ghimire, Sujan, Deo, Ravinesh C., Raj, Nawin and Mi, Jianchun. 2019. "Wavelet-based 3-phase hybrid SVR model trained with satellite-derived predictors, particle swarm optimization and maximum overlap discrete wavelet transform for solar radiation prediction." Renewable and Sustainable Energy Reviews. 113, pp. 1-19. https://doi.org/10.1016/j.rser.2019.109247Deep solar radiation forecasting with convolutional neural network and long short-term memory network algorithms
Ghimire, Sujan, Deo, Ravinesh C., Raj, Nawin and Mi, Jianchun. 2019. "Deep solar radiation forecasting with convolutional neural network and long short-term memory network algorithms." Applied Energy. 253, pp. 1-20. https://doi.org/10.1016/j.apenergy.2019.113541Sleep EEG signal analysis based on correlation graph similarity coupled with an ensemble extreme machine learning algorithm
Abdulla, Shahab, Diykh, Mohammed, Lafta, Raid Luaibi, Saleh, Khalid and Deo, Ravinesh C.. 2019. "Sleep EEG signal analysis based on correlation graph similarity coupled with an ensemble extreme machine learning algorithm." Expert Systems with Applications. 138, pp. 1-15. https://doi.org/10.1016/j.eswa.2019.07.007Short-term electricity demand forecasting using machine learning methods enriched with ground-based climate and ECMWF Reanalysis atmospheric predictors in southeast Queensland, Australia
Al-Musaylh, Mohanad S., Deo, Ravinesh C., Adamowski, Jan F. and Li, Yan. 2019. "Short-term electricity demand forecasting using machine learning methods enriched with ground-based climate and ECMWF Reanalysis atmospheric predictors in southeast Queensland, Australia." Renewable and Sustainable Energy Reviews. 113. https://doi.org/10.1016/j.rser.2019.109293Comparing the annualised dynamic shade characteristics of twenty-one tree canopies across twenty-six municipalities in a high ambient UV climate, Queensland - Australia
Downs, Nathan J., Baldwin, Louise, Parisi, Alfio V., Butler, Harry J., Vanos, Jennifer, Beckman, Melanie and Harrison, Simone. 2019. "Comparing the annualised dynamic shade characteristics of twenty-one tree canopies across twenty-six municipalities in a high ambient UV climate, Queensland - Australia." Applied Geography. 108, pp. 74-82. https://doi.org/10.1016/j.apgeog.2019.05.006Cloud segmentation property extraction from total sky image repositories using Python
Igoe, Damien P., Parisi, Alfio V. and Downs, Nathan J.. 2019. "Cloud segmentation property extraction from total sky image repositories using Python." Instrumentation Science and Technology. 47 (5), pp. 522-534. https://doi.org/10.1080/10739149.2019.1603996Designing a new data intelligence model for global solar radiation prediction: application of multivariate modeling scheme
Tao, Hai, Ebtehaj, Isa, Bonakdari, Hossein, Heddam, Salim, Voyant, Cyril, Al-Ansari, Nadhir, Deo, Ravinesh and Yaseen, Zaheer Mundher. 2019. "Designing a new data intelligence model for global solar radiation prediction: application of multivariate modeling scheme." Energies. 12 (7), pp. 1-24. https://doi.org/10.3390/en12071365Incorporating synoptic-scale climate signals for streamflow modelling over the Mediterranean region using machine learning models
Kisi, Ozgur, Choubin, Bahram, Deo, Ravinesh C. and Yaseen, Zaheer Mundher. 2019. "Incorporating synoptic-scale climate signals for streamflow modelling over the Mediterranean region using machine learning models." Hydrological Sciences Journal. 64 (10), pp. 1240-1252. https://doi.org/10.1080/02626667.2019.1632460Improving SPI-derived drought forecasts incorporating synoptic-scale climate indices in multi-phase multivariate empirical mode decomposition model hybridized with simulated annealing and kernel ridge regression algorithms
Ali, Mumtaz, Deo, Ravinesh C., Maraseni, Tek and Downs, Nathan J.. 2019. "Improving SPI-derived drought forecasts incorporating synoptic-scale climate indices in multi-phase multivariate empirical mode decomposition model hybridized with simulated annealing and kernel ridge regression algorithms." Journal of Hydrology. 576, pp. 164-184. https://doi.org/10.1016/j.jhydrol.2019.06.032Deep Learning Neural Networks Trained with MODIS Satellite-Derived Predictors for Long-Term Global Solar Radiation Prediction
Ghimire, Sujan, Deo, Ravinesh C., Raj, Nawin and Mi, Jianchun. 2019. "Deep Learning Neural Networks Trained with MODIS Satellite-Derived Predictors for Long-Term Global Solar Radiation Prediction." Energies. 12 (12), pp. 1-42. https://doi.org/10.3390/en12122407Situations, challenges and strategies of urban water management in Beijing under rapid urbanization effect
Liu, Wen, Chen, Weiping, Feng, Qi and Deo, Ravinesh C.. 2019. "Situations, challenges and strategies of urban water management in Beijing under rapid urbanization effect." Water Science and Technology: Water Supply. 19 (1), pp. 115-127. https://doi.org/10.2166/ws.2018.057Significant wave height forecasting via an extreme learning machine model integrated with improved complete ensemble empirical mode decomposition
Ali, Mumtaz and Prasad, Ramendra. 2019. "Significant wave height forecasting via an extreme learning machine model integrated with improved complete ensemble empirical mode decomposition." Renewable and Sustainable Energy Reviews. 104, pp. 281-295. https://doi.org/10.1016/j.rser.2019.01.014Designing a multi-stage multivariate empirical mode decomposition coupled with ant colony optimization and random forest model to forecast monthly solar radiation
Prasad, Ramendra, Ali, Mumtaz, Kwan, Paul and Khan, Huma. 2019. "Designing a multi-stage multivariate empirical mode decomposition coupled with ant colony optimization and random forest model to forecast monthly solar radiation." Applied Energy. 236, pp. 778-792. https://doi.org/10.1016/j.apenergy.2018.12.034Biologically effective solar ultraviolet exposures and the potential skin cancer risk for individual gold medalists of the 2020 Tokyo Summer Olympic Games
Downs, Nathan J., Axelsen, Taryn, Schouten, Peter, Igoe, Damien P., Parisi, Alfio V. and Vanos, Jennifer. 2019. "Biologically effective solar ultraviolet exposures and the potential skin cancer risk for individual gold medalists of the 2020 Tokyo Summer Olympic Games." Temperature. https://doi.org/10.1080/23328940.2019.1581427Suitable exclosure duration for the restoration of degraded alpine grasslands on the Qinghai-Tibetan Plateau
Cao, Jianjun, Li, Guangdong, Adamowski, Jan F., Holden, Nicolas M., Deo, Ravinesh C., Hu, Zeyong, Zhu, Guofeng, Xu, Xueyun and Feng, Qi. 2019. "Suitable exclosure duration for the restoration of degraded alpine grasslands on the Qinghai-Tibetan Plateau." Land Use Policy: the international journal covering all aspects of land use. 86, pp. 261-267. https://doi.org/10.1016/j.landusepol.2019.05.008Environmental and economic impacts and trade-offs from simultaneous management of soil constraints, nitrogen and water
Kodur, Shreevatsa, Shrestha, Uttam Babu, Maraseni, Tek Narayan and Deo, Ravinesh C.. 2019. "Environmental and economic impacts and trade-offs from simultaneous management of soil constraints, nitrogen and water." Journal of Cleaner Production. 222, pp. 960-970. https://doi.org/10.1016/j.jclepro.2019.03.079Land subsidence modelling using tree-based machine learning algorithms
Rahmati, Omid, Falah, Fatemeh, Naghibi, Seyed Amir, Biggs, Trent, Soltani, Milad, Deo, Ravinesh C., Cerda, Artemi, Mohammadi, Farnoush and Bui, Dieu Tien. 2019. "Land subsidence modelling using tree-based machine learning algorithms." Science of the Total Environment. 672, pp. 239-252. https://doi.org/10.1016/j.scitotenv.2019.03.496Weekly soil moisture forecasting with multivariate sequential, ensemble empirical mode decomposition and Boruta-random forest hybridizer algorithm approach
Prasad, Ramendra, Deo, Ravinesh C., Li, Yan and Maraseni, Tek. 2019. "Weekly soil moisture forecasting with multivariate sequential, ensemble empirical mode decomposition and Boruta-random forest hybridizer algorithm approach." Catena. 177, pp. 149-166. https://doi.org/10.1016/j.catena.2019.02.012Copula statistical models for analyzing stochastic dependencies of systemic drought risk and potential adaptation strategies
Nguyen-Huy, Thong, Deo, Ravinesh C., Mushtaq, Shahbaz, Kath, Jarrod and Khan, Shahjahan. 2019. "Copula statistical models for analyzing stochastic dependencies of systemic drought risk and potential adaptation strategies." Stochastic Environmental Research and Risk Assessment. 33 (3), pp. 779-799. https://doi.org/10.1007/s00477-019-01662-6Effects of stand age on carbon storage in dragon spruce forest ecosystems in the upper reaches of the Bailongjiang River basin, China
Cao, Jianjun, Gong, Yifan, Adamowski, Jan F., Deo, Ravinesh C., Zhu, Guofeng, Dong, Xiaogang, Zhang, Xiaofang, Liu, Haibo and Xin, Cunlin. 2019. "Effects of stand age on carbon storage in dragon spruce forest ecosystems in the upper reaches of the Bailongjiang River basin, China." Scientific Reports. 9 (1), pp. 1-11. https://doi.org/10.1038/s41598-019-39626-zThe role of topography in shaping the spatial patterns of soil organic carbon
Zhu, Meng, Feng, Qi, Qin, Yanyan, Cao, Jiajun, Zhang, Mengxu, Liu, Wei, Deo, Ravinesh C., Zhang, Chengqi, Li, Ruolin and Li, Baofeng. 2019. "The role of topography in shaping the spatial patterns of soil organic carbon." Catena. 176, pp. 296-305. https://doi.org/10.1016/j.catena.2019.01.029PMT: new analytical framework for automated evaluation of geo-environmental modelling approaches
Rahmati, Omid, Kornejady, Aiding, Samadi, Mahmood, Deo, Ravinesh C., Conoscenti, Christian, Lombardo, Luigi, Dayal, Kavina, Taghizadeh-Mehrjardi, Ruhollah, Pourghasemi, Hamid Reza, Kumar, Sandeep and Bui, Dieu Tien. 2019. "PMT: new analytical framework for automated evaluation of geo-environmental modelling approaches." Science of the Total Environment. 664, pp. 296-311. https://doi.org/10.1016/j.scitotenv.2019.02.017Universally deployable extreme learning machines integrated with remotely sensed MODIS satellite predictors over Australia to forecast global solar radiation: a new approach
Deo, Ravinesh C., Sahin, Mehmet, Adamowski, Jan F. and Mi, Jianchun. 2019. "Universally deployable extreme learning machines integrated with remotely sensed MODIS satellite predictors over Australia to forecast global solar radiation: a new approach." Renewable and Sustainable Energy Reviews. 104, pp. 235-261. https://doi.org/10.1016/j.rser.2019.01.009Two-phase extreme learning machines integrated with the complete ensemble empirical mode decomposition with adaptive noise algorithm for multi-scale runoff prediction problems
Wen, Xiaohu, Feng, Qi, Deo, Ravinesh C., Wu, Min, Yin, Zhenliang, Yang, Linshan and Singh, Vijay P.. 2019. "Two-phase extreme learning machines integrated with the complete ensemble empirical mode decomposition with adaptive noise algorithm for multi-scale runoff prediction problems." Journal of Hydrology. 570, pp. 167-184. https://doi.org/10.1016/j.jhydrol.2018.12.060New approach for sediment yield forecasting with a two-phase feed forward neuron network-particle swarm optimization model integrated with the gravitational search algorithm
Meshram, Sarita Gajbhiye, Ghorbani, M. A., Deo, Ravinesh C., Kashani, Mahsa Hasanpour, Meshram, Chandrashekhar and Karimi, Vahid. 2019. "New approach for sediment yield forecasting with a two-phase feed forward neuron network-particle swarm optimization model integrated with the gravitational search algorithm." Water Resources Management. 33 (7), pp. 2335-2356. https://doi.org/10.1007/s11269-019-02265-0Long-term modelling of wind speeds using six different heuristic artificial intelligence approaches
Maroufpoor, Saman, Sanikhani, Hadi, Kisi, Ozgur, Deo, Ravinesh C. and Yaseen, Zaher Mundher. 2019. "Long-term modelling of wind speeds using six different heuristic artificial intelligence approaches." International Journal of Climatology. 39 (8), pp. 3543-3557. https://doi.org/10.1002/joc.6037Development and evaluation of hybrid artificial neural network architectures for modeling spatio-temporal groundwater fluctuations in a complex aquifer system
Roshni, Thendiyath, Jha, Madan K., Deo, Ravinesh C. and Vandana, A.. 2019. "Development and evaluation of hybrid artificial neural network architectures for modeling spatio-temporal groundwater fluctuations in a complex aquifer system." Water Resources Management. 33 (7), pp. 2381-2397. https://doi.org/10.1007/s11269-019-02253-4An enhanced extreme learning machine model for river flow forecasting: state-of-the-art, practical applications in water resource engineering area and future research direction
Yaseen, Zaher Mundher, Sulaiman, Sadeq Oleiwi, Deo, Ravinesh C. and Chau, Kwok-Wing. 2019. "An enhanced extreme learning machine model for river flow forecasting: state-of-the-art, practical applications in water resource engineering area and future research direction." Journal of Hydrology. 569, pp. 387-408. https://doi.org/10.1016/j.jhydrol.2018.11.069The influence of structural factors on stormwater runoff retention of extensive green roofs: new evidence from scale-based models and real experiments
Liu, Wen, Feng, Qi, Chen, Weiping, Wei, Wei and Deo, Ravinesh C.. 2019. "The influence of structural factors on stormwater runoff retention of extensive green roofs: new evidence from scale-based models and real experiments." Journal of Hydrology. 569, pp. 230-238. https://doi.org/10.1016/j.jhydrol.2018.11.066Development of a model for calculating the solar ultraviolet protection factor of small to medium sized built shade structure
Parisi, Alfio V., Amar, Abdurazaq, Downs, Nathan J., Igoe, Damien P., Harrison, Simone L. and Turner, Joanna. 2019. "Development of a model for calculating the solar ultraviolet protection factor of small to medium sized built shade structure." Building and Environment. 147, pp. 415-421. https://doi.org/10.1016/j.buildenv.2018.10.010Direct and indirect impacts of ionic components of saline water on irrigated soil chemical and microbial processes
Chen, Lijuan, Li, Changsheng, Feng, Qi, Wei, Yongping, Zhao, Yan, Zhu, Meng and Deo, Ravinesh C.. 2019. "Direct and indirect impacts of ionic components of saline water on irrigated soil chemical and microbial processes." Catena. 172, pp. 581-589. https://doi.org/10.1016/j.catena.2018.09.030Global solar radiation prediction by ANN integrated with European Centre for medium range weather forecast fields in solar rich cities of Queensland Australia
Ghimire, Sujan, Deo, Ravinesh C., Downs, Nathan J. and Raj, Nawin. 2019. "Global solar radiation prediction by ANN integrated with European Centre for medium range weather forecast fields in solar rich cities of Queensland Australia." Journal of Cleaner Production. 216, pp. 288-310. https://doi.org/10.1016/j.jclepro.2019.01.158Future projection with an extreme-learning machine and support vector regression of reference evapotranspiration in a mountainous inland watershed in north-west China
Yin, Zhenliang, Feng, Qi, Yang, Linshan, Deo, Ravinesh C., Wen, Xiaohu, Si, Jianhua and Xiao, Shengchun. 2017. "Future projection with an extreme-learning machine and support vector regression of reference evapotranspiration in a mountainous inland watershed in north-west China." Water: an open access journal. 9 (11), pp. 880-902. https://doi.org/10.3390/w9110880The spatial and temporal contribution of glacier runoff to watershed discharge in the Yarkant River Basin, Northwest China
Yin, Zhenliang, Feng, Qi, Liu, Shiyin, Zhou, Songbing, Li, Jing, Yang, Linshan and Deo, Ravinesh C.. 2017. "The spatial and temporal contribution of glacier runoff to watershed discharge in the Yarkant River Basin, Northwest China." Water: an open access journal. 9 (3), pp. 159-178. https://doi.org/10.3390/w9030159Logic connectives of complex fuzzy sets
Ngan, Tran Thi, Lan, Luong Thi Hong, Ali, Mumtaz, Tamir, Dan, Son, Le Hoang, Tuan, Tran Manh, Rishe, Naphtali and Kandel, Abe. 2018. "Logic connectives of complex fuzzy sets." Romanian Journal of Information Science and Technology. 21 (4), pp. 344-357.A novel approach for fuzzy clustering based on neutrosophic association matrix
Long, Hoang Viet, Ali, Mumtaz, Son, Le Hoang, Khan, Mohsin and Tu, Doan Ngoc. 2019. "A novel approach for fuzzy clustering based on neutrosophic association matrix." Computers and Industrial Engineering. 127, pp. 687-697. https://doi.org/10.1016/j.cie.2018.11.007A pilot observational study of environmental summertime health risk behaviour in central Brisbane, Queensland: opportunities to raise sun protection awareness in Australia’s Sunshine State
Dexter, Ben, King, Rachel, Harrison, Simone L., Parisi, Alfio V. and Downs, Nathan J.. 2019. "A pilot observational study of environmental summertime health risk behaviour in central Brisbane, Queensland: opportunities to raise sun protection awareness in Australia’s Sunshine State." Photochemistry and Photobiology. 95 (2), pp. 650-655. https://doi.org/10.1111/php.13011Systematic review of decision making algorithms in extended neutrosophic sets
Khan, Mohsin, Son, Le Hoang, Ali, Mumtaz, Chau, Hoang Thi Minh, Na, Nguyen Thi Nhu and Smarandache, Florentin. 2018. "Systematic review of decision making algorithms in extended neutrosophic sets." Symmetry. 10 (8), pp. 1-28. https://doi.org/10.3390/sym10080314Global Solar Radiation Prediction Using Hybrid Online Sequential Extreme Learning Machine Model
Hou, Muzhou, Zhang, Tianle, Weng, Futian, Ali, Mumtaz, Al-Ansari, Nadhir and Yaseen, Zaher Mundher. 2018. "Global Solar Radiation Prediction Using Hybrid Online Sequential Extreme Learning Machine Model." Energies. 11 (12), pp. 1-19. https://doi.org/10.3390/en11123415New Soft Set Based Class of Linear Algebraic Codes
Ali, Mumtaz, Khan, Huma, Son, Le Hoang, Smarandache, Florentin and Kandasamy, W. B. Vasantha. 2018. "New Soft Set Based Class of Linear Algebraic Codes." Symmetry. 10 (10), pp. 1-10. https://doi.org/10.3390/sym10100510H-max distance measure of intuitionistic fuzzy sets in decision making
Ngan, Roan Thi, Son, Le Hoang, Cuong, Bui Cong and Ali, Mumtaz. 2018. "H-max distance measure of intuitionistic fuzzy sets in decision making." Applied Soft Computing. 69, pp. 393-425. https://doi.org/10.1016/j.asoc.2018.04.036Evaluated UVA irradiances over a twelve year period at a sub-tropical site from ozone monitoring instrument data including the influence of cloud
A Jebar, Mustapha A., Parisi, Alfio V., Downs, Nathan J. and Turner, Joanna F.. 2018. "Evaluated UVA irradiances over a twelve year period at a sub-tropical site from ozone monitoring instrument data including the influence of cloud." Photochemistry and Photobiology. 94 (6), pp. 1281-1288. https://doi.org/10.1111/php.12948Particle swarm optimized–support vector regression hybrid model for daily horizon electricity demand forecasting using climate dataset
Al-Musaylh, Mohanad S., Deo, Ravinesh C. and Li, Yan. 2018. "Particle swarm optimized–support vector regression hybrid model for daily horizon electricity demand forecasting using climate dataset." Huang, Q. and Kolhe, M. (ed.) 3rd International Conference on Power and Renewable Energy (ICPRE 2018). Berlin, Germany 21 - 24 Sep 2018 Germany. https://doi.org/10.1051/e3sconf/20186408001Effects of topography on soil organic carbon stocks in grasslands of a semiarid alpine region, northwestern China
Zhu, Meng, Feng, Qi, Zhang, Mengxu, Liu, Wei, Qin, Yanyan, Deo, Ravinesh C. and Zhang, Chengqi. 2019. "Effects of topography on soil organic carbon stocks in grasslands of a semiarid alpine region, northwestern China." Journal of Soils and Sediments: protection, risk assessment and remediation. 19 (4), pp. 1640-1650. https://doi.org/10.1007/s11368-018-2203-0A joint deep neural network model for pain recognition from face
Bargshady, Ghazal, Soar, Jeffrey, Zhou, Xujuan, Deo, Ravinesh, Whittaker, Frank and Wang, Hua. 2019. "A joint deep neural network model for pain recognition from face." 4th IEEE International Conference on Computer and Communication Systems (ICCCS 2019). Singapore 23 - 25 Feb 2019 Singapore. pp. 52-56 https://doi.org/10.1109/CCOMS.2019.8821779Effects of Afforestation on Soil Bulk Density and pH in the Loess Plateau, China
Zhang, Xiaofang, Adamowski, Jan F., Deo, Ravinesh C., Xu, Xueyun, Zhu, Guofeng and Cao, Jianjun. 2018. "Effects of Afforestation on Soil Bulk Density and pH in the Loess Plateau, China." Water: an open access journal. 10 (12), pp. 1-15. https://doi.org/10.3390/w10121710Assessment of soil salinisation in the Ejina Oasis located in the lower reaches of Heihe River, Northwestern China
Zhao, Yu, Feng, Qi, Lu, Aigang and Deo, Ravinesh C.. 2019. "Assessment of soil salinisation in the Ejina Oasis located in the lower reaches of Heihe River, Northwestern China." Chemistry and Ecology. 35 (4), pp. 330-343. https://doi.org/10.1080/02757540.2018.1554060Design and implementation of a hybrid MLP-GSA model with multilayer perceptron-gravitational search algorithm for monthly lake water level forecasting
Ghorbani, Mohammad Ali, Deo, Ravinesh C., Karimi, Vahid, Kashani, Mahsa H. and Ghorbani, Shahryar. 2018. "Design and implementation of a hybrid MLP-GSA model with multilayer perceptron-gravitational search algorithm for monthly lake water level forecasting." Stochastic Environmental Research and Risk Assessment. 33 (1), pp. 125-147. https://doi.org/10.1007/s00477-018-1630-1Monitoring paddy productivity in North Korea employing geostationary satellite images integrated with GRAMI-rice model
Yeom, Jong-min, Jeong, Seungtaek, Jeong, Gwanyong, Ng, Chi Tim, Deo, Ravinesh C. and Ko, Jonghan. 2018. "Monitoring paddy productivity in North Korea employing geostationary satellite images integrated with GRAMI-rice model." Scientific Reports. 8, pp. 1-15. https://doi.org/10.1038/s41598-018-34550-0Artificial intelligence approach for the prediction of Robusta coffee yield using soil fertility properties
Kouadio, Louis, Deo, Ravinesh C., Byrareddy, Vivekananda, Adamowski, Jan F., Mushtaq, Shahbaz and Nguyen, Van Phuong. 2018. "Artificial intelligence approach for the prediction of Robusta coffee yield using soil fertility properties." Computers and Electronics in Agriculture. 155, pp. 324-338. https://doi.org/10.1016/j.compag.2018.10.014Artificial intelligence-based fast and efficient hybrid approach for spatial modelling of soil electrical conductivity
Ghorbani, Mohammad Ali, Deo, Ravinesh C., Kashani, Mahsa H., Shahabi, Mahmoud and Ghorbani, Shahryar. 2019. "Artificial intelligence-based fast and efficient hybrid approach for spatial modelling of soil electrical conductivity." Soil and Tillage Research. 186, pp. 152-164. https://doi.org/10.1016/j.still.2018.09.012Quantifying flood events in Bangladesh with a daily-step flood monitoring index based on the concept of daily effective precipitation
Deo, Ravinesh C., Adamowski, Jan F., Begum, Khaleda, Salcedo-sanz, Sancho, Kim, Do-Woo, Dayal, Kavina S. and Byun, Hi-Ryong. 2019. "Quantifying flood events in Bangladesh with a daily-step flood monitoring index based on the concept of daily effective precipitation." Theoretical and Applied Climatology. 137, pp. 1201-1215. https://doi.org/10.1007/s00704-018-2657-4Shear strength prediction of steel fiber reinforced concrete beam using hybrid intelligence models: a new approach
Yaseen, Zaheer Munder, Tran, Minh Tung, Kim, Sungwon, Bakhshpoori, Taha and Deo, Ravinesh C.. 2018. "Shear strength prediction of steel fiber reinforced concrete beam using hybrid intelligence models: a new approach." Engineering Structures. 177, pp. 244-255. https://doi.org/10.1016/j.engstruct.2018.09.074Adaptive Neuro-Fuzzy Inference System integrated with solar zenith angle for forecasting sub-tropical photosynthetically active radiation
Deo, Ravinesh C., Downs, Nathan J., Adamowski, Jan F. and Parisi, Alfio V.. 2018. "Adaptive Neuro-Fuzzy Inference System integrated with solar zenith angle for forecasting sub-tropical photosynthetically active radiation." Food and Energy Security. 8 (1). https://doi.org/10.1002/fes3.151Cotton yield prediction with Markov Chain Monte Carlo-based simulation model integrated with genetic programing algorithm: a new hybrid copula-driven approach
Ali, Mumtaz, Deo, Ravinesh C., Downs, Nathan J. and Maraseni, Tek. 2018. "Cotton yield prediction with Markov Chain Monte Carlo-based simulation model integrated with genetic programing algorithm: a new hybrid copula-driven approach." Agricultural and Forest Meteorology. 263, pp. 428-448. https://doi.org/10.1016/j.agrformet.2018.09.002Weekly heat wave death prediction model using zero-inflated regression approach
Kim, Do-Woo, Deo, Ravinesh C., Park, Sang-Jin, Lee, Jong-Seol and Lee, Woo-Seop. 2019. "Weekly heat wave death prediction model using zero-inflated regression approach." Theoretical and Applied Climatology. 137 (1-2), pp. 823-838. https://doi.org/10.1007/s00704-018-2636-9A comparative study of temperature and precipitation‑based aridity indices and their trends in Mongolia
Nyamtseren, Mandakh, Feng, Qi and Deo, Ravinesh C.. 2018. "A comparative study of temperature and precipitation‑based aridity indices and their trends in Mongolia." International Journal of Environmental Research. 12, pp. 887-899. https://doi.org/10.1007/s41742-018-0143-6Can individual land ownership reduce grassland degradation and favor socioeconomic sustainability on the Qinghai-Tibetan Plateau?
Cao, J. J., Holden, N. M., Adamowski, J. F., Deo, R. C., Xu, X. Y. and Feng, Q.. 2018. "Can individual land ownership reduce grassland degradation and favor socioeconomic sustainability on the Qinghai-Tibetan Plateau?" Environmental Science and Policy. 89, pp. 192-197. https://doi.org/10.1016/j.envsci.2018.08.003Copula-based agricultural conditional value-at-risk modelling for geographical diversifications in wheat farming portfolio management
Nguyen-Huy, Thong, Deo, Ravinesh C., Mushtaq, Shahbaz, Kath, Jarrod and Khan, Shahjahan. 2018. "Copula-based agricultural conditional value-at-risk modelling for geographical diversifications in wheat farming portfolio management." Weather and Climate Extremes. 21, pp. 76-89. https://doi.org/10.1016/j.wace.2018.07.002Design and evaluation of SVR, MARS and M5Tree models for 1, 2 and 3-day lead time forecasting of river flow data in a semiarid mountainous catchment
Yin, Zhenliang, Feng, Qi, Wen, Xiaohu, Deo, Ravinesh C., Yang, Linshan, Si, Jianhua and He, Zhibin. 2018. "Design and evaluation of SVR, MARS and M5Tree models for 1, 2 and 3-day lead time forecasting of river flow data in a semiarid mountainous catchment." Stochastic Environmental Research and Risk Assessment. 32 (9), pp. 2457-2476. https://doi.org/10.1007/s00477-018-1585-2Mapping hypothermia death vulnerability in Korea
Park, Sang-Jin, Kim, Do-Woo, Deo, Ravinesh C. and Lee, Jong-Seol. 2018. "Mapping hypothermia death vulnerability in Korea." International Journal of Disaster Risk Reduction. 31, pp. 668-678. https://doi.org/10.1016/j.ijdrr.2018.06.016Multi-stage committee based extreme learning machine model incorporating the influence of climate parameters and seasonality on drought forecasting
Ali, Mumtaz, Deo, Ravinesh C., Downs, Nathan J. and Maraseni, Tek. 2018. "Multi-stage committee based extreme learning machine model incorporating the influence of climate parameters and seasonality on drought forecasting." Computers and Electronics in Agriculture. 152, pp. 149-165. https://doi.org/10.1016/j.compag.2018.07.013A real-time hourly water index for flood risk monitoring: pilot studies in Brisbane, Australia, and Dobong Observatory, South Korea
Deo, Ravinesh C., Byun, Hi-Ryong, Kim, Ga-Byn and Adamowski, Jan F.. 2018. "A real-time hourly water index for flood risk monitoring: pilot studies in Brisbane, Australia, and Dobong Observatory, South Korea." Environmental Monitoring and Assessment. 190 (450). https://doi.org/10.1007/s10661-018-6806-0Multi-stage hybridized online sequential extreme learning machine integrated with Markov Chain Monte Carlo copula-Bat algorithm for rainfall forecasting
Ali, Mumtaz, Deo, Ravinesh C., Downs, Nathan J. and Maraseni, Tek. 2018. "Multi-stage hybridized online sequential extreme learning machine integrated with Markov Chain Monte Carlo copula-Bat algorithm for rainfall forecasting." Atmospheric Research. 213, pp. 450-464. https://doi.org/10.1016/j.atmosres.2018.07.005Influence of stand type and stand age on soil carbon storage in China’s arid and semi-arid regions
Cao, Jianjun, Zhang, Xiaofang, Deo, Ravinesh, Gong, Yifan and Feng, Qi. 2018. "Influence of stand type and stand age on soil carbon storage in China’s arid and semi-arid regions." Land Use Policy: the international journal covering all aspects of land use. 78, pp. 258-265. https://doi.org/10.1016/j.landusepol.2018.07.002The geospatial relationship of pterygium and senile cataract to ambient solar ultraviolet in tropical Ecuador
Garzon-Chavez, Daniel R., Quentin, Emmanuelle, Harrison, Simone L., Parisi, Alfio V., Butler, Harry J. and Downs, Nathan J.. 2018. "The geospatial relationship of pterygium and senile cataract to ambient solar ultraviolet in tropical Ecuador." Photochemical and Photobiological Sciences. 17 (8), pp. 1075-1083. https://doi.org/10.1039/C8PP00023ADesign and implementation of a hybrid model based on two-layer decomposition method coupled with extreme learning machines to support real-time environmental monitoring of water quality parameters
Fijani, Elham, Barzegar, Rahim, Deo, Ravinesh, Tziritis, Evangelos and Konstantinos, Skordas. 2019. "Design and implementation of a hybrid model based on two-layer decomposition method coupled with extreme learning machines to support real-time environmental monitoring of water quality parameters." Science of the Total Environment. 648, pp. 839-853. https://doi.org/10.1016/j.scitotenv.2018.08.221Triathlon and the potential for solar ultraviolet exposure risk assessed during the swim, bicycle and running legs of competitive Australian Ironman events
Axelsen, Taryn, Parisi, Alfio V. and Downs, Nathan J.. 2018. "Triathlon and the potential for solar ultraviolet exposure risk assessed during the swim, bicycle and running legs of competitive Australian Ironman events." 4th International Conference on UV & Skin Cancer Prevention 2018. Toronto, Canada 01 - 04 May 2018Reflecting on fraction addition: using the mirror formula to visualise unit fraction addition
Igoe, Damien, Boucher, Nicholas, Clark, Iain, Parisi, Alfio and Downs, Nathan. 2018. "Reflecting on fraction addition: using the mirror formula to visualise unit fraction addition." Australian Mathematics Teacher. 74 (2), pp. 3-9.Optimization of windspeed prediction using an artificial neural network compared with a genetic programming model
Deo, Ravinesh C., Ghimire, Sujan, Downs, Nathan J. and Raj, Nawin. 2018. "Optimization of windspeed prediction using an artificial neural network compared with a genetic programming model." Kim, Dookie, Roy, Sanjiban Sekhar, Lansivaara, Tim, Deo, Ravinesh C. and Samui, Pijush (ed.) Handbook of research on predictive modeling and optimization methods in science and engineering. Hershey, United States. IGI Global. pp. 328-359Hybrid data intelligent models and applications for water level prediction
Yaseen, Zaher Mundher, Deo, Ravinesh C., Ebtehaj, Isa and Bonakdari, Hossein. 2018. "Hybrid data intelligent models and applications for water level prediction." Kim, Dookie, Roy, Sanjiban Sekhar, Länsivaara, Tim, Deo, Ravinesh C. and Samui, Pijush (ed.) Handbook of research on predictive modeling and optimization methods in science and engineering. Hershey, United States. IGI Global. pp. 121-139Selection of representative feature training sets with self-organized maps for optimized time series modeling and prediction: application to forecasting daily drought conditions with ARIMA and neural network models
McCarthy, Elizabeth, Deo, Ravinesh C., Li, Yan and Maraseni, Tek. 2018. "Selection of representative feature training sets with self-organized maps for optimized time series modeling and prediction: application to forecasting daily drought conditions with ARIMA and neural network models." Kim, Dookie, Roy, Sanjiban Sekhar, Lansivaara, Tim, Deo, Ravinesh C. and Samui, Pijush (ed.) Handbook of research on predictive modeling and optimization methods in science and engineering. Hershey, United States. IGI Global. pp. 446-464Survey of different data-intelligent modeling strategies for forecasting air temperature using geographic information as model predictors
Sanikhani, Hadi, Deo, Ravinesh C., Samui, Pijush, Kisi, Ozgur, Mert, Chian, Mirabbasi, Rasoul, Gavili, Siavash and Yaseen, Zaher Mundher. 2018. "Survey of different data-intelligent modeling strategies for forecasting air temperature using geographic information as model predictors." Computers and Electronics in Agriculture. 152, pp. 242-260. https://doi.org/10.1016/j.compag.2018.07.008Soil moisture forecasting by a hybrid machine learning technique: ELM integrated with ensemble empirical mode decomposition
Prasad, Ramendra, Deo, Ravinesh C., Li, Yan and Maraseni, Tek. 2018. "Soil moisture forecasting by a hybrid machine learning technique: ELM integrated with ensemble empirical mode decomposition." Geoderma. 330, pp. 136-161. https://doi.org/10.1016/j.geoderma.2018.05.035Modeling the joint influence of multiple synoptic-scale, climate mode indices on Australian wheat yield using a vine copula-based approach
Nguyen-Huy, Thong, Deo, Ravinesh C., Mushtaq, Shahbaz, An-Vo, Duc-Anh and Khan, Shahjahan. 2018. "Modeling the joint influence of multiple synoptic-scale, climate mode indices on Australian wheat yield using a vine copula-based approach." European Journal of Agronomy. 98, pp. 65-81. https://doi.org/10.1016/j.eja.2018.05.006Non-tuned data intelligent model for soil temperature estimation: a new approach
Sanikhani, Hadi, Deo, Ravinesh C., Yaseen, Zaher Mundheer, Eray, Okan and Kisi, Ozgur. 2018. "Non-tuned data intelligent model for soil temperature estimation: a new approach." Geoderma. 330, pp. 52-64. https://doi.org/10.1016/j.geoderma.2018.05.030Drought prediction with standardized precipitation and evapotranspiration index and support vector regression models
Deo, Ravinesh C., Salcedo-sanz, Sancho, Carro-Calvo, Leopoldo and Saavedra-Moreno, Beatriz. 2018. "Drought prediction with standardized precipitation and evapotranspiration index and support vector regression models." Samui, Pijush, Kim, Dookie and Ghosh, Chandan (ed.) Integrating disaster science and management. Amsterdam, Netherlands. Elsevier. pp. 151-174Drought modelling based on artificial intelligence and neural network algorithms: a case study in Queensland, Australia
Dayal, Kavina, Deo, Ravinesh and Apan, Armando A.. 2017. "Drought modelling based on artificial intelligence and neural network algorithms: a case study in Queensland, Australia." Filho, Walter Leal (ed.) Climate change adaptation in Pacific countries:fostering resilience and improving the quality of life. Springer. pp. 177-198The inverse relationship between the incidence of solar induced eye disease and reported skin cancer rates at high elevation in the tropical Andes, Ecuador, South America
Downs, Nathan J., Garzon Chavez, Daniel R., Quentin, Emmanuelle, Harrison, Simone L., Parisi, Alfio V. and Butler, Harry. 2018. "The inverse relationship between the incidence of solar induced eye disease and reported skin cancer rates at high elevation in the tropical Andes, Ecuador, South America." 4th International Conference on UV & Skin Cancer Prevention 2018. Toronto, Canada 01 - 04 May 2018 Canada.The Garment Protection Factor (GPF): an index for sun-protective clothing that combines garment coverage and UVR transmittance
Harrison, Simone L., Downs, Nathan J., Rawlings, Alex T. and Parisi, Alfio V.. 2018. "The Garment Protection Factor (GPF): an index for sun-protective clothing that combines garment coverage and UVR transmittance." 4th International Conference on UV & Skin Cancer Prevention 2018. Toronto, Canada 01 - 04 May 2018 Canada.Input selection and data-driven model performance optimization to predict the Standardized Precipitation and Evaporation Index in a drought-prone region
Mouatadid, Soukayna, Raj, Nawin, Deo, Ravinesh C. and Adamowski, Jan F.. 2018. "Input selection and data-driven model performance optimization to predict the Standardized Precipitation and Evaporation Index in a drought-prone region." Atmospheric Research. 212, pp. 130-149. https://doi.org/10.1016/j.atmosres.2018.05.012Re-imagining standard timescales in forecasting precipitation events for Queensland’s grazing enterprises
McCarthy, E., Deo, R. C., Li, Y. and Maraseni, T.. 2017. "Re-imagining standard timescales in forecasting precipitation events for Queensland’s grazing enterprises." Syme, G., Hatton MacDonald, D., Fulton, B. and Piantadosi, J. (ed.) 22nd International Congress on Modelling and Simulation (MODSIM2017). Hobart, Australia 03 - 08 Dec 2017 Australia. Modelling and Simulation Society of Australia and New Zealand . https://doi.org/10.36334/modsim.2017.L1.mccarthySelf-adaptive differential evolutionary extreme learning machines for long-term solar radiation prediction with remotely-sensed MODIS satellite and Reanalysis atmospheric products in solar-rich cities
Ghimire, Sujan, Deo, Ravinesh C., Downs, Nathan J. and Raj, Nawin. 2018. "Self-adaptive differential evolutionary extreme learning machines for long-term solar radiation prediction with remotely-sensed MODIS satellite and Reanalysis atmospheric products in solar-rich cities." Remote Sensing of Environment: an interdisciplinary journal. 212, pp. 176-198. https://doi.org/10.1016/j.rse.2018.05.003An ensemble-ANFIS based uncertainty assessment model for forecasting multi-scalar standardized precipitation index
Ali, Mumtaz, Deo, Ravinesh C., Downs, Nathan J. and Maraseni, Tek. 2018. "An ensemble-ANFIS based uncertainty assessment model for forecasting multi-scalar standardized precipitation index." Atmospheric Research. 207, pp. 155-180. https://doi.org/10.1016/j.atmosres.2018.02.024Wavelet analysis–artificial neural network conjunction models for multi-scale monthly groundwater level predicting in an arid inland river basin, northwestern China
Wen, Xiaohu, Feng, Qi, Deo, Ravinesh C., Wu, Min and Si, Jianhua. 2017. "Wavelet analysis–artificial neural network conjunction models for multi-scale monthly groundwater level predicting in an arid inland river basin, northwestern China." Hydrology Research: an international journal. 48 (6), pp. 1710-1729. https://doi.org/10.2166/nh.2016.396Ensemble committee-based data intelligent approach for generating soil moisture forecasts with multivariate hydro-meteorological predictors
Prasad, Ramendra, Deo, Ravinesh C., Li, Yan and Maraseni, Tek. 2018. "Ensemble committee-based data intelligent approach for generating soil moisture forecasts with multivariate hydro-meteorological predictors." Soil and Tillage Research. 181, pp. 63-81. https://doi.org/10.1016/j.still.2018.03.021Comparison of social-ecological resilience between two grassland management patterns driven by grassland land contract policy in the Maqu, Qinghai-Tibetan Plateau
Cao, Jianjun, Li, Mengtian, Deo, Ravinesh C., Adamowski, Jan F, Cerda, Artemi, Feng, Qi, Liu, Minxia, Zhang, Jian, Zhu, Guofeng, Zhang, Xuebin, Xu, Xueyun, Yang, Shurong and Gong, Yifan. 2018. "Comparison of social-ecological resilience between two grassland management patterns driven by grassland land contract policy in the Maqu, Qinghai-Tibetan Plateau." Land Use Policy: the international journal covering all aspects of land use. 74, pp. 88-96. https://doi.org/10.1016/j.landusepol.2017.07.027Spatio-temporal drought risk mapping approach and its application in the drought-prone region of south-east Queensland, Australia
Dayal, Kavina S., Deo, Ravinesh C. and Apan, Armando A.. 2018. "Spatio-temporal drought risk mapping approach and its application in the drought-prone region of south-east Queensland, Australia." Natural Hazards. 93 (2), pp. 823-847. https://doi.org/10.1007/s11069-018-3326-8A new approach to predict daily pH in rivers based on the 'a trous' redundant wavelet transform algorithm
Rajaee, Taher, Ravansalar, Masoud, Adamowski, Jan F. and Deo, Ravinesh C.. 2018. "A new approach to predict daily pH in rivers based on the 'a trous' redundant wavelet transform algorithm." Water, Air and Soil Pollution: an international journal of environmental pollution. 229 (3). https://doi.org/10.1007/s11270-018-3715-3Computational intelligence approaches for energy load forecasting in smart energy management grids: state of the art, future challenges, and research directions and Research Directions
Fallah, Seyedeh Narjes, Deo, Ravinesh Chand, Shojafar, Mohammad, Conti, Mauro and Shamshirband, Shahaboddin. 2018. "Computational intelligence approaches for energy load forecasting in smart energy management grids: state of the art, future challenges, and research directions and Research Directions." Energies. 11 (3). https://doi.org/10.3390/en11030596Two-phase particle swarm optimized-support vector regression hybrid model integrated with improved empirical mode decomposition with adaptive noise for multiple-horizon electricity demand forecasting
Al-Musaylh, Mohanad S., Deo, Ravinesh C., Li, Yan and Adamowski, Jan F.. 2018. "Two-phase particle swarm optimized-support vector regression hybrid model integrated with improved empirical mode decomposition with adaptive noise for multiple-horizon electricity demand forecasting." Applied Energy. 217, pp. 422-439. https://doi.org/10.1016/j.apenergy.2018.02.140Comparative signal to noise ratio as a determinant to select smartphone image sensor colour channels for analysis in the UVB
Igoe, D. P., Parisi, A. V., Downs, N. J., Amar, A. and Turner, J.. 2018. "Comparative signal to noise ratio as a determinant to select smartphone image sensor colour channels for analysis in the UVB." Sensors and Actuators A: Physical. 272, pp. 125-133. https://doi.org/10.1016/j.sna.2018.01.057Atmospheric total ozone column evaluation with a smartphone image sensor
Igoe, Damien P., Parisi, Alfio V., Amar, Abdurazaq, Downs, Nathan J. and Turner, Joanna. 2018. "Atmospheric total ozone column evaluation with a smartphone image sensor." International Journal of Remote Sensing. 39 (9), pp. 2766-2783. https://doi.org/10.1080/01431161.2018.1433895Application of the hybrid artificial neural network coupled with rolling mechanism and grey model algorithms for streamflow forecasting over multiple time horizons
Yaseen, Zaher Mundher, Fu, Minglei, Wang, Chen, Mohtar, Wan Hanna Melini Wan, Deo, Ravinesh C. and El-Shafie, Ahmed. 2018. "Application of the hybrid artificial neural network coupled with rolling mechanism and grey model algorithms for streamflow forecasting over multiple time horizons." Water Resources Management. 32 (5), pp. 1883-1899. https://doi.org/10.1007/s11269-018-1909-5Multi-household grazing management pattern maintains better soil fertility
Cao, Jianjun, Xu, Xueyun, Deo, Ravinesh C., Holden, Nicholas M., Adamowski, Jan F., Gong, Yifan, Feng, Qi, Yang, Shurong, Li, Mengtian, Zhou, Junju, Zhang, Jian and Liu, Minxia. 2018. "Multi-household grazing management pattern maintains better soil fertility." Agronomy for Sustainable Development: sciences des productions vegetales et de l'environnement. 38 (1). https://doi.org/10.1007/s13593-017-0482-2Short-term electricity demand forecasting with MARS, SVR and ARIMA models using aggregated demand data in Queensland, Australia
Al-Musaylh, Mohanad S., Deo, Ravinesh C., Adamowski, Jan F. and Li, Yan. 2018. "Short-term electricity demand forecasting with MARS, SVR and ARIMA models using aggregated demand data in Queensland, Australia." Advanced Engineering Informatics: the science of supporting knowledge-intensive activities. 35 (C), pp. 1-16. https://doi.org/10.1016/j.aei.2017.11.002Mapping groundwater contamination risk of multiple aquifers using multi-model ensemble of machine learning algorithms
Barzegar, Rahim, Moghaddam, Asghar Asghari, Deo, Ravinesh, Fijani, Elham and Tziritis, Evangelos. 2018. "Mapping groundwater contamination risk of multiple aquifers using multi-model ensemble of machine learning algorithms." Science of the Total Environment. 621, pp. 697-712. https://doi.org/10.1016/j.scitotenv.2017.11.185Investigating drought duration-severity-intensity characteristics using the Standardized Precipitation-Evapotranspiration Index: case studies in drought-prone Southeast Queensland
Dayal, Kavina S., Deo, Ravinesh C. and Apan, Armando A.. 2018. "Investigating drought duration-severity-intensity characteristics using the Standardized Precipitation-Evapotranspiration Index: case studies in drought-prone Southeast Queensland." Journal of Hydrologic Engineering. 23 (1), p. 05017029. https://doi.org/10.1061/(ASCE)HE.1943-5584.0001593An efficient neuro-evolutionary hybrid modelling mechanism for the estimation of daily global solar radiation in the Sunshine State of Australia
Salcedo-sanz, Sancho, Deo, Ravinesh C., Cornejo-Bueno, Laura, Camacho-Gomez, Carlos and Ghimire, Sujan. 2018. "An efficient neuro-evolutionary hybrid modelling mechanism for the estimation of daily global solar radiation in the Sunshine State of Australia." Applied Energy. 209, pp. 79-94. https://doi.org/10.1016/j.apenergy.2017.10.076Multi-layer perceptron hybrid model integrated with the firefly optimizer algorithm for windspeed prediction of target site using a limited set of neighboring reference station data
Deo, Ravinesh C., Ghorbani, Mohammad Ali, Samadianfard, Saeed, Maraseni, Tek, Bilgili, Mehmet and Biazar, Mustafa. 2018. "Multi-layer perceptron hybrid model integrated with the firefly optimizer algorithm for windspeed prediction of target site using a limited set of neighboring reference station data." Renewable Energy. 116 (Part A), pp. 309-323. https://doi.org/10.1016/j.renene.2017.09.078A comprehensive approach to evaluating and classifying sun-protective clothing
Downs, N. J. and Harrison, S. L.. 2018. "A comprehensive approach to evaluating and classifying sun-protective clothing." British Journal of Dermatology. 178 (4), pp. 958-964. https://doi.org/10.1111/bjd.15938Identifying separate impacts of climate and land use/cover change on hydrological processes in upper stream of Heihe River, northwest China
Yang, Linshan, Feng, Qi, Yin, Zhenliang, Wen, Xiaohu, Si, Jianhua, Li, Changbin and Deo, Ravinesh C.. 2017. "Identifying separate impacts of climate and land use/cover change on hydrological processes in upper stream of Heihe River, northwest China." Hydrological Processes. 31 (5), pp. 1100-1112. https://doi.org/10.1002/hyp.11098Reservoir inflow forecasting with a modified coactive neuro-fuzzy inference system: a case study for a semi-arid region
Allawi, Mohammed Falah, Jaafar, Othman, Hamzah, Firdaus Mohamad, Mohd, Nuruol Syuhadaa, Deo, Ravinesh C. and El-Shafie, Ahmed. 2018. "Reservoir inflow forecasting with a modified coactive neuro-fuzzy inference system: a case study for a semi-arid region." Theoretical and Applied Climatology. 134 (1-2), pp. 545-563. https://doi.org/10.1007/s00704-017-2292-5Implementation of a hybrid MLP-FFA model for water level prediction of Lake Egirdir, Turkey
Ghorbani, Mohammad Ali, Deo, Ravinesh C., Karimi, Vahid, Yaseen, Zaher Mundher and Terz, Ozlem. 2018. "Implementation of a hybrid MLP-FFA model for water level prediction of Lake Egirdir, Turkey." Stochastic Environmental Research and Risk Assessment. 32 (6), pp. 1683-1697. https://doi.org/10.1007/s00477-017-1474-0Pan evaporation prediction using a hybrid multilayer perceptron-firefly algorithm (MLP-FFA) model: case study in North Iran
Ghorbani, M. A., Deo, Ravinesh C., Yaseen, Zaher Mundher, Kashani, Mahsa H. and Mohammadi, Babak. 2018. "Pan evaporation prediction using a hybrid multilayer perceptron-firefly algorithm (MLP-FFA) model: case study in North Iran." Theoretical and Applied Climatology. 133 (3-4), pp. 1119-1131. https://doi.org/10.1007/s00704-017-2244-0Impact of grassland contract policy on soil organic carbon losses from alpine grassland on the Qinghai–Tibetan Plateau
Cao, J., Gong, Y., Yeh, E. T., Holden, N. M., Adamowski, J. F., Deo, R. C., Liu, M., Zhou, J., Zhang, J., Zhang, S., Sheng, D., Yang, S., Xu, X., Li, M. and Feng, Q.. 2017. "Impact of grassland contract policy on soil organic carbon losses from alpine grassland on the Qinghai–Tibetan Plateau." Soil Use and Management. 33 (4), pp. 633-671. https://doi.org/10.1111/sum.12387Effects of ecological water transport on photosynthesis and chlorophyll fluorescence of Populus euphratica
Zhao, Chun Yan, Si, Jian Hua, Feng, Qi, Yu, Teng Fei, Deo, Ravinesh C. and Luo, Huan. 2018. "Effects of ecological water transport on photosynthesis and chlorophyll fluorescence of Populus euphratica." Water Science and Technology: Water Supply. 18 (5), pp. 1747-1756. https://doi.org/10.2166/ws.2017.236Trend analysis of Water Poverty Index for assessment of water stress and water management polices: a case study in the Hexi Corridor, China
Huang, Shan, Feng, Qi, Lu, Zhixiang, Wen, Xiaohu and Deo, Ravinesh C.. 2017. "Trend analysis of Water Poverty Index for assessment of water stress and water management polices: a case study in the Hexi Corridor, China." Sustainability. 9 (5), pp. 756-772. https://doi.org/10.3390/su9050756Forecasting evaporative loss by least-square support-vector regression and evaluation with genetic programming, Gaussian process, and minimax probability machine regression: case study of Brisbane City
Deo, Ravinesh C. and Samui, Pijush. 2017. "Forecasting evaporative loss by least-square support-vector regression and evaluation with genetic programming, Gaussian process, and minimax probability machine regression: case study of Brisbane City." Journal of Hydrologic Engineering. 22 (6), pp. 1-15. https://doi.org/10.1061/(ASCE)HE.1943-5584.0001506Statistical evaluation of rainfall time series in concurrence with agriculture and water resources of Ken River basin, Central India (1901–2010)
Meshram, Sarita Gajbhiye, Singh, Sudhir Kumar, Meshram, Chandrashekhar, Deo, Ravinesh C. and Ambade, Balram. 2018. "Statistical evaluation of rainfall time series in concurrence with agriculture and water resources of Ken River basin, Central India (1901–2010)." Theoretical and Applied Climatology. 134, pp. 1231-1243. https://doi.org/10.1007/s00704-017-2335-yAn international comparison of rice consumption behaviours and greenhouse gas emissions from rice production
Maraseni, Tek Narayan, Deo, Ravinesh C., Qu, Jiansheng, Gentle, Popular and Neupane, Prem Raj. 2018. "An international comparison of rice consumption behaviours and greenhouse gas emissions from rice production." Journal of Cleaner Production. 172, pp. 2288-2300. https://doi.org/10.1016/j.jclepro.2017.11.182An Inexpensive High-Temporal Resolution Electronic Sun Journal for Monitoring Personal Day to Day Sun Exposure Patterns
Downs, Nathan J., Parisi, Alfio V., Butler, Harry, Rawlings, Alex and Elrahoumi, Raja Salem. 2017. "An Inexpensive High-Temporal Resolution Electronic Sun Journal for Monitoring Personal Day to Day Sun Exposure Patterns." Frontiers in Public Health. 5, pp. 1-9. https://doi.org/10.3389/fpubh.2017.00310Physiological response to salinity stress and tolerance mechanics of Populus euphratica
Zhao, Chun Yan, Si, Jian Hua, Feng, Qi, Deo, Ravinesh C., Yu, Teng Fei and Li, Pei Du. 2017. "Physiological response to salinity stress and tolerance mechanics of Populus euphratica." Environmental Monitoring and Assessment. 189 (11), pp. 533-543. https://doi.org/10.1007/s10661-017-6257-zCarbon dioxide fluxes and their environmental controls in a riparian forest within the hyper-arid region of Northwest China
Ma, Xiaohong, Feng, Qi, Yu, Tengfei, Su, Yonghong and Deo, Ravinesh C.. 2017. "Carbon dioxide fluxes and their environmental controls in a riparian forest within the hyper-arid region of Northwest China." Forests. 8 (10), pp. 1-17. https://doi.org/10.3390/f8100379Comparative study of hybrid-wavelet artificial intelligence models for monthly groundwater depth forecasting in extreme arid regions, Northwest China
Yu, Haijiao, Wen, Xiaohu, Feng, Qi, Deo, Ravinesh C., Si, Jianhua and Wu, Min. 2018. "Comparative study of hybrid-wavelet artificial intelligence models for monthly groundwater depth forecasting in extreme arid regions, Northwest China." Water Resources Management. 32 (1), pp. 301-323. https://doi.org/10.1007/s11269-017-1811-6Predicting compressive strength of lightweight foamed concrete using extreme learning machine model
Yaseen, Zaher Mundher, Deo, Ravinesh C., Hilal, Ameer, Abd, Abbas M., Bueno, Laura Cornejo, Salcedo-sanz, Sancho and Nehdi, Moncef L.. 2018. "Predicting compressive strength of lightweight foamed concrete using extreme learning machine model." Advances in Engineering Software. 115, pp. 112-125. https://doi.org/10.1016/j.advengsoft.2017.09.004Novel approach for streamflow forecasting using a hybrid ANFIS-FFA model
Yaseen, Zaher Mundher, Ebtehaj, Isa, Bonakdari, Hossein, Deo, Ravinesh C., Mehr, Ali Danandeh, Mohtar, Wan Hanna Melini Wan, Diop, Lamine, El-Shafie, Ahmed and Singh, Vijay P.. 2017. "Novel approach for streamflow forecasting using a hybrid ANFIS-FFA model." Journal of Hydrology. 554, pp. 263-276. https://doi.org/10.1016/j.jhydrol.2017.09.007Rainfall Pattern Forecasting Using Novel Hybrid Intelligent Model Based ANFIS-FFA
Yaseen, Zaher Mundher, Ghareb, Mazen Ismaeel, Ebtehaj, Isa, Bonakdari, Hossein, Siddique, Ridwan, Heddam, Sali, Yusif, Ali A. and Deo, Ravinesh. 2018. "Rainfall Pattern Forecasting Using Novel Hybrid Intelligent Model Based ANFIS-FFA." Water Resources Management. 32 (1), pp. 105-122. https://doi.org/10.1007/s11269-017-1797-0Synthetic retrieval of hourly net ecosystem exchange using the neural network model with combined MI and GOCI geostationary sensor datasets and ground-based measurements
Yeom, Jong-Min, Deo, Ravinesh, Chun, Junghwa, Hong, Jinkyu, Kim, Dong-Su, Han, Kyung-Soo and Cho, Jaeil. 2017. "Synthetic retrieval of hourly net ecosystem exchange using the neural network model with combined MI and GOCI geostationary sensor datasets and ground-based measurements." International Journal of Remote Sensing. 38 (23), pp. 7441-7456. https://doi.org/10.1080/01431161.2017.1375573Separation of the Climatic and Land Cover Impacts on the Flow Regime Changes in Two Watersheds of Northeastern Tibetan Plateau
Yang, Linshan, Feng, Qi, Yin, Zhenliang, Deo, Ravinesh C., Wen, Xiaohu, Si, Jianhua and Li, Changbin. 2017. "Separation of the Climatic and Land Cover Impacts on the Flow Regime Changes in Two Watersheds of Northeastern Tibetan Plateau." Advances in Meteorology. 2017, pp. 1-15. https://doi.org/10.1155/2017/6310401Changes in climatic elements in the Pan-Hexi region during 1960–2014 and responses to global climatic changes
Wei, Liu, Feng, Qi and Deo, Ravinesh C.. 2018. "Changes in climatic elements in the Pan-Hexi region during 1960–2014 and responses to global climatic changes." Theoretical and Applied Climatology. 133 (1-2), pp. 405-420. https://doi.org/10.1007/s00704-017-2194-6Mapping heatwave vulnerability in Korea
Kim, Do-Woo, Deo, Ravinesh C., Lee, Jong-Seol and Yeom, Jong-Min. 2017. "Mapping heatwave vulnerability in Korea." Natural Hazards. 89 (1), pp. 35-55. https://doi.org/10.1007/s11069-017-2951-yCopula-statistical precipitation forecasting model in Australia’s agro-ecological zones
Nguyen-Huy, Thong, Deo, Ravinesh C., An-Vo, Duc-Anh, Mushtaq, Shahbaz and Khan, Shahjahan. 2017. "Copula-statistical precipitation forecasting model in Australia’s agro-ecological zones." Agricultural Water Management. 191, pp. 153-172. https://doi.org/10.1016/j.agwat.2017.06.010Input selection and performance optimization of ANN-based streamflow forecasts in the drought-prone Murray Darling Basin region using IIS and MODWT algorithm
Prasad, Ramendra, Deo, Ravinesh C., Li, Yan and Maraseni, Tek. 2017. "Input selection and performance optimization of ANN-based streamflow forecasts in the drought-prone Murray Darling Basin region using IIS and MODWT algorithm." Atmospheric Research. 197, pp. 42-63. https://doi.org/10.1016/j.atmosres.2017.06.014Very short-term reactive forecasting of the solar ultraviolet index using an extreme learning machine integrated with the solar zenith angle
Deo, Ravinesh C., Downs, Nathan, Parisi, Alfio V., Adamowski, Jan F. and Quilty, John M.. 2017. "Very short-term reactive forecasting of the solar ultraviolet index using an extreme learning machine integrated with the solar zenith angle." Environmental Research. 155, pp. 141-166. https://doi.org/10.1016/j.envres.2017.01.035Association between plant species diversity and edaphic factors in the lower reaches of the Heihe River, northwestern China
Zhao, Yu, Feng, Qi, Xi, Haiyang, Li, Huiya, Yang, Huaide and Deo, Ravinesh C.. 2017. "Association between plant species diversity and edaphic factors in the lower reaches of the Heihe River, northwestern China." Chemistry and Ecology. 33 (3), pp. 181-195. https://doi.org/10.1080/02757540.2017.1287904Bipolar neutrosophic soft sets and applications in decision making
Ali, Mumtaz, Son, Le Hoang, Deli, Irfan and Tien, Nguyen Dang. 2017. "Bipolar neutrosophic soft sets and applications in decision making." Journal of Intelligent and Fuzzy Systems. 33 (6), pp. 4077-4087. https://doi.org/10.3233/JIFS-17999Forecasting long-term global solar radiation with an ANN algorithm coupled with satellite-derived (MODIS) land surface temperature (LST) for regional locations in Queensland
Deo, Ravinesh C. and Sahin, Mehmet. 2017. "Forecasting long-term global solar radiation with an ANN algorithm coupled with satellite-derived (MODIS) land surface temperature (LST) for regional locations in Queensland." Renewable and Sustainable Energy Reviews. 72, pp. 828-848. https://doi.org/10.1016/j.rser.2017.01.114Computational intelligence approach for modeling hydrogen production: a review
Faizollahzadeh, Sina, Najafi, Bahman, Shamshirband, Shahaboddin, Bidgoli, Behrouz Minaei, Deo, Ravinesh Chand and Chau, Kwok-wing. 2018. "Computational intelligence approach for modeling hydrogen production: a review." Engineering Applications of Computational Fluid Mechanics. 12 (1), pp. 438-458. https://doi.org/10.1080/19942060.2018.1452296Link prediction in co-authorship networks based on hybrid content similarity metric
Chuan, Pham Minh, Son, Le Hoang, Ali, Mumtaz, Khang, Tran Dinh, Huong, Le Thanh and Dey, Nilanjan. 2018. "Link prediction in co-authorship networks based on hybrid content similarity metric." Applied Intelligence. 48 (8), pp. 2470-2486. https://doi.org/10.1007/s10489-017-1086-xA neutrosophic recommender system for medical diagnosis based on algebraic neutrosophic measures
Ali, Mumtaz, Son, Le Hoang, Thanh, Nguyen Dang and Minh, Nguyen Van. 2018. "A neutrosophic recommender system for medical diagnosis based on algebraic neutrosophic measures." Applied Soft Computing. 71, pp. 1054-1071. https://doi.org/10.1016/j.asoc.2017.10.012Segmentation of dental X-ray images in medical imaging using neutrosophic orthogonal matrices
Ali, Mumtaz, Son, Le Hoang, Khan, Mohsin and Tung, Nguyen Thanh. 2017. "Segmentation of dental X-ray images in medical imaging using neutrosophic orthogonal matrices." Expert Systems with Applications. 91, pp. 434-441. https://doi.org/10.1016/j.eswa.2017.09.027Stream-flow forecasting using extreme learning machines: a case study in a semi-arid region in Iraq
Yaseen, Zaher Mundher, Jaafar, Othman, Deo, Ravinesh C., Kisi, Ozgur, Adamowski, Jan, Quilty, John and El-Shafie, Ahmed. 2016. "Stream-flow forecasting using extreme learning machines: a case study in a semi-arid region in Iraq." Journal of Hydrology. 542, pp. 603-614. https://doi.org/10.1016/j.jhydrol.2016.09.035Quantitative definition and spatiotemporal distribution of little water season (LIWAS) in Korea
Kim, Su-Jeong, Byun, Hi-Ryong and Deo, Ravinesh C.. 2016. "Quantitative definition and spatiotemporal distribution of little water season (LIWAS) in Korea." Asia-Pacific Journal of Atmospheric Sciences. 52 (4), pp. 379-393. https://doi.org/10.1007/s13143-016-0012-1Interval Complex Neutrosophic Set: Formulation and Applications in Decision-Making
Ali, Mumtaz, Dat, Luu Quoc, Son, Le Hoang and Smarandache, Florentin. 2018. "Interval Complex Neutrosophic Set: Formulation and Applications in Decision-Making." International Journal of Fuzzy Systems. 20 (3), pp. 986-999. https://doi.org/10.1007/s40815-017-0380-4Validation of ozone monitoring instrument UV satellite data using spectral and broadband surface based measurements at a Queensland site
A Jebar, Mustapha A., Parisi, Alfio V., Downs, Nathan J. and Turner, Joanna F.. 2017. "Validation of ozone monitoring instrument UV satellite data using spectral and broadband surface based measurements at a Queensland site." Photochemistry and Photobiology. 93 (5), pp. 1289-1293. https://doi.org/10.1111/php.12784Solar ultraviolet and the occupational radiant exposure of Queensland school teachers: a comparative study between teaching classifications and behavior patterns
Downs, Nathan J., Harrison, Simone L., Garzon Chavez, Daniel R. and Parisi, Alfio V.. 2016. "Solar ultraviolet and the occupational radiant exposure of Queensland school teachers: a comparative study between teaching classifications and behavior patterns." Journal of Photochemistry and Photobiology, B: Biology. 158, pp. 105-112. https://doi.org/10.1016/j.jphotobiol.2016.02.018Dual calibrated dosimeter for simultaneous measurements of erythemal and vitamin D effective solar ultraviolet radiation
Wainwright, L., Parisi, A. V. and Downs, N.. 2016. "Dual calibrated dosimeter for simultaneous measurements of erythemal and vitamin D effective solar ultraviolet radiation." Journal of Photochemistry and Photobiology, B: Biology. 157, pp. 15-21. https://doi.org/10.1016/j.jphotobiol.2016.02.003An extreme learning machine model for the simulation of monthly mean streamflow water level in eastern Queensland
Deo, Ravinesh C. and Sahin, Mehmet. 2016. "An extreme learning machine model for the simulation of monthly mean streamflow water level in eastern Queensland." Environmental Monitoring and Assessment. 188 (90). https://doi.org/10.1007/s10661-016-5094-9Forecasting effective drought index using a wavelet extreme learning machine (W-ELM) model
Deo, Ravinesh C., Tiwari, Mukesh K., Adamowski, Jan F. and Quilty, John M.. 2017. "Forecasting effective drought index using a wavelet extreme learning machine (W-ELM) model." Stochastic Environmental Research and Risk Assessment. 31 (5), pp. 1211-1240. https://doi.org/10.1007/s00477-016-1265-zδ-equality of intuitionistic fuzzy sets: a new proximity measure and applications in medical diagnosis
Ngan, Roan Thi, Ali, Mumtaz and Son, Le Hoang. 2018. "δ-equality of intuitionistic fuzzy sets: a new proximity measure and applications in medical diagnosis." Applied Intelligence. 48 (2), pp. 499-525. https://doi.org/10.1007/s10489-017-0986-0A novel clustering algorithm in a neutrosophic recommender system for medical diagnosis
Thanh, Nguyen Dang, Ali, Mumtaz and Son, Le Hoang. 2017. "A novel clustering algorithm in a neutrosophic recommender system for medical diagnosis." Cognitive Computation. 9 (4), pp. 526-544. https://doi.org/10.1007/s12559-017-9462-8Drought forecasting in eastern Australia using multivariate adaptive regression spline, least square support vector machine and M5Tree model
Deo, Ravinesh C., Kisi, Ogzur and Singh, Vijay P.. 2017. "Drought forecasting in eastern Australia using multivariate adaptive regression spline, least square support vector machine and M5Tree model." Atmospheric Research. 184, pp. 149-175. https://doi.org/10.1016/j.atmosres.2016.10.004A wavelet-coupled support vector machine model for forecasting global incident solar radiation using limited meteorological dataset
Deo, Ravinesh C., Wen, Xiaohu and Feng, Qi. 2016. "A wavelet-coupled support vector machine model for forecasting global incident solar radiation using limited meteorological dataset." Applied Energy. 168, pp. 568-593. https://doi.org/10.1016/j.apenergy.2016.01.130Concurrent evaluation of personal damaging and beneficial UV exposures over an extended period
Wainwright, L. K., Parisi, A. V. and Downs, N. J.. 2017. "Concurrent evaluation of personal damaging and beneficial UV exposures over an extended period." Journal of Photochemistry and Photobiology, B: Biology. 170, pp. 188-196. https://doi.org/10.1016/j.jphotobiol.2017.04.013Comparison of GOME-2 UVA satellite data to ground-based spectroradiometer measurements at a subtropical site
Parisi, Alfio V., Downs, Nathan, Turner, Joanna and King, Rachel. 2017. "Comparison of GOME-2 UVA satellite data to ground-based spectroradiometer measurements at a subtropical site." IEEE Transactions on Geoscience and Remote Sensing. 55 (6), pp. 3145-3149. https://doi.org/10.1109/TGRS.2017.2662714Educating the younger generation (and the public) in UV effects with a simple experiment
Turner, Joanna, Parisi, Alfio V. and Downs, Nathan. 2013. "Educating the younger generation (and the public) in UV effects with a simple experiment." Byrne, Scott and Chen, Min (ed.) 6th Asia & Oceania Conference on Photobiology (AOCP 2013): Life on a Sun-Drenched Planet. Sydney, Australia 10 - 13 Nov 2013In-situ measurements of solar exposure distribution on a coral reef: preliminary findings relating to reef aspect, water turbidity and dosimeter design
Downs, N. J., Schouten, P. W. and Parisi, A. V.. 2016. "In-situ measurements of solar exposure distribution on a coral reef: preliminary findings relating to reef aspect, water turbidity and dosimeter design." 13th International Coral Reef Symposium. Honolulu, Hawaii 19 - 24 Jun 2016Application of hybrid artificial neural network algorithm for the prediction of Standardized Precipitation Index
Dayal, Kavina S., Deo, Ravinesh C. and Apan, Armando A.. 2016. "Application of hybrid artificial neural network algorithm for the prediction of Standardized Precipitation Index." 2016 IEEE Region 10 International Conference: Technologies for Smart Nation (TENCON 2016). Singapore 22 - 25 Nov 2016 Singapore. https://doi.org/10.1109/TENCON.2016.7848588Solar ultraviolet attenuation during the Australian (Red Dawn) dust event of 23 September 2009
Downs, Nathan, Butler, Harry and Parisi, Alfio. 2016. "Solar ultraviolet attenuation during the Australian (Red Dawn) dust event of 23 September 2009." Bulletin of the American Meteorological Society. 97 (11), pp. 2039-2050. https://doi.org/10.1175/BAMS-D-15-00053.1Characterization of cloud cover with a smartphone camera
Parisi, A. V., Downs, N., Igoe, D. and Turner, J.. 2016. "Characterization of cloud cover with a smartphone camera." Instrumentation Science and Technology. 44 (1), pp. 23-34. https://doi.org/10.1080/10739149.2015.1055577Solar radiation and the UV index: an application of numerical integration, trigonometric functions, online education and the modelling process
Downs, Nathan, Parisi, Alfio, Galligan, Linda, Turner, Joanna, Amar, Abdurazaq, King, Rachel, Ultra, Filipina and Butler, Harry. 2016. "Solar radiation and the UV index: an application of numerical integration, trigonometric functions, online education and the modelling process." International Journal of Research in Education and Science. 2 (1), pp. 179-189.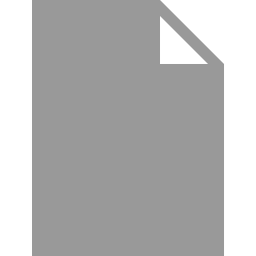