Kernel Ridge Regression Hybrid Method for Wheat Yield Prediction with Satellite-Derived Predictors
Article
Article Title | Kernel Ridge Regression Hybrid Method for Wheat Yield Prediction with Satellite-Derived Predictors |
---|---|
ERA Journal ID | 201448 |
Article Category | Article |
Authors | Ahmed, A. A. Masrur (Author), Sharma, Ekta (Author), Jui, S. Janifer Jabin (Author), Deo, Ravinesh C. (Author), Nguyen-Huy, Thong (Author) and Ali, Mumtaz (Author) |
Journal Title | Remote Sensing |
Journal Citation | 14 (5), pp. 1-24 |
Article Number | 1136 |
Number of Pages | 24 |
Year | 2022 |
Publisher | MDPI AG |
Place of Publication | Switzerland |
ISSN | 2072-4292 |
Digital Object Identifier (DOI) | https://doi.org/10.3390/rs14051136 |
Web Address (URL) | https://www.mdpi.com/2072-4292/14/5/1136 |
Abstract | Wheat dominates the Australian grain production market and accounts for 10–15% of the world’s 100 million tonnes annual global wheat trade. Accurate wheat yield prediction is critical to satisfying local consumption and increasing exports regionally and globally to meet human food security. This paper incorporates remote satellite-based information in a wheat-growing region in South Australia to estimate the yield by integrating the kernel ridge regression (KRR) method coupled with complete ensemble empirical mode decomposition with adaptive noise (CEEMDAN) and the grey wolf optimisation (GWO). The hybrid model, ‘GWO-CEEMDAN-KRR,’ employing an initial pool of 23 different satellite-based predictors, is seen to outperform all the benchmark models and all the feature selection (ant colony, atom search, and particle swarm optimisation) methods that are implemented using a set of carefully screened satellite variables and a feature decomposition or CEEMDAN approach. A suite of statistical metrics and infographics comparing the predicted and measured yield shows a model prediction error that can be reduced by ~20% by employing the proposed GWO-CEEMDAN-KRR model. With the metrics verifying the accuracy of simulations, we also show that it is possible to optimise the wheat yield to achieve agricultural profits by quantifying and including the effects of satellite variables on potential yield. With further improvements in the proposed methodology, the GWO-CEEMDAN-KRR model can be adopted in agricultural yield simulation that requires remote sensing data to establish the relationships between crop health, yield, and other productivity features to support precision agriculture. |
Keywords | Kernel ridge regression; Machine learning; Satellite data; South Australia; Wheat yield |
ANZSRC Field of Research 2020 | 460207. Modelling and simulation |
300403. Agronomy | |
Byline Affiliations | School of Mathematics, Physics and Computing |
Torrens University | |
Centre for Applied Climate Sciences | |
Deakin University | |
Institution of Origin | University of Southern Queensland |
https://research.usq.edu.au/item/q71z9/kernel-ridge-regression-hybrid-method-for-wheat-yield-prediction-with-satellite-derived-predictors
Download files
429
total views115
total downloads14
views this month6
downloads this month
Export as
Related outputs
Leveraging Advanced Data-Driven Approaches to Forecast Daily Floods Based on Rainfall for Proactive Prevention Strategies in Saudi Arabia
Aldhafiri, Anwar Ali, Ali, Mumtaz and Labban, Abdulhaleem H.. 2025. "Leveraging Advanced Data-Driven Approaches to Forecast Daily Floods Based on Rainfall for Proactive Prevention Strategies in Saudi Arabia." Water. 17 (11). https://doi.org/10.3390/w17111699The Development of a Novel Quantum Pre-processing Filter to Improve Image Classification Accuracy of Neural Network Models
Riaz, Farina, Abdulla, Shahab, Suzuki, Hajime, Ganguly, Srinjoy, Deo, Ravinesh C. and Hopkins, Susan. 2025. "The Development of a Novel Quantum Pre-processing Filter to Improve Image Classification Accuracy of Neural Network Models ." Journal of Data Science and Intelligent Systems. https://doi.org/10.47852/bonviewJDSIS52024231Predicting near-real-time total water level with an artificial intelligence model based on Australia's tidal wave energy belt dataset
AL-Musaylh, Mohanad S., Gharineiat, Zahra, Al‑Dafaie, Kadhem, Jasim, Khalid Fadhil, Sharma, Ekta and Nahi, Abdullah A.. 2025. "Predicting near-real-time total water level with an artificial intelligence model based on Australia's tidal wave energy belt dataset." Journal of Ocean Engineering and Marine Energy. https://doi.org/10.1007/s40722-025-00394-wSatellite-based data for agricultural index insurance: a systematic quantitative literature review
Nguyen, Thuy, Mushtaq, Shahbaz, Kath, Jarrod, Nguyen-Huy, Thong and Reymondin, Louis. 2025. "Satellite-based data for agricultural index insurance: a systematic quantitative literature review." Natural Hazards and Earth System Sciences. 25 (2), p. 913–927. https://doi.org/10.5194/nhess-25-913-2025Quantifying error in fine-scale crop yield forecasts to guide data and algorithm improvements: case study of mango in Tamil Nadu, India
Kouadio, Louis, Kulanthaivel, Bhuvaneswari, Mai, Thanh, Nguyen-Huy, Thong, Wang, Qingxia (Jenny), Byrareddy, Vivekananda M., Mushtaq, Shahbaz, Senthil, Alagarswamy, Newlands, Nathaniel K. and Geethalakshmi, Vellingiri. 2025. "Quantifying error in fine-scale crop yield forecasts to guide data and algorithm improvements: case study of mango in Tamil Nadu, India." Computers and Electronics in Agriculture. 236. https://doi.org/10.1016/j.compag.2025.110450Hybrid CNN–GRU model for hourly flood forecasting index: case studies from the Fiji islands
Chand, Ravinesh, Deo, Ravinesh C., Ghimire, Sujan, Nguyen-Huy, Thong and Ali, Mumtaz. 2025. "Hybrid CNN–GRU model for hourly flood forecasting index: case studies from the Fiji islands." Stochastic Environmental Research and Risk Assessment. 39 (5), pp. 2203-2229. https://doi.org/10.1007/s00477-025-02964-8Defining potential pathways for improving the resilience and sustainable development of rangeland grazing systems: Insights from northern Australia
Mai, Thanh, Reardon-Smith, Kathryn, Cobon, David H., Nguyen-Huy, Thong and Mushtaq, Shahbaz. 2025. "Defining potential pathways for improving the resilience and sustainable development of rangeland grazing systems: Insights from northern Australia." Science of the Total Environment. 978. https://doi.org/10.1016/j.scitotenv.2025.179488Assessing Pan-Canada wildfire susceptibility by integrating satellite data with novel hybrid deep learning and black widow optimizer algorithms
Khosravi, Khabat, Mosallanejad, Ashkan, Bateni, Sayed M., Kim, Dongkyun, Jun, Changhyun, Shahvaran, Ali Reza, Farooque, Aitazaz A., Karbasi, Massoud and Ali, Mumtaz. 2025. "Assessing Pan-Canada wildfire susceptibility by integrating satellite data with novel hybrid deep learning and black widow optimizer algorithms." Science of the Total Environment. 977. https://doi.org/https://doi.org/10.1016/j.scitotenv.2025.179369Deep learning models for drought susceptibility mapping in Southeast Queensland, Australia
Rezaie, Fatemeh, Panahi, Mahdi P, Jun, Changhyun, Dayal, Kavina, Kim, Dongkyun, Darabi, Hamid, Kalantari, Zahra, Seifollahi-Aghmiuni, Samaneh, Deo, Ravinesh C. and Bateni, Sayed M.. 2025. "Deep learning models for drought susceptibility mapping in Southeast Queensland, Australia." Stochastic Environmental Research and Risk Assessment. https://doi.org/10.1007/s00477-024-02879-wIntelligent modeling and analysis of hybrid organic Rankine plants: Data-driven insights into thermodynamic efficiency and economic viability
Tao, Hai, Aldlemy, Mohammed Suleman, Saad, Mohammed Ayad, Yeap, Swee Pin, Oudah, Atheer Y., Alawi, Omer A., Goliatt, Leonardo, Ahmad, Shamsad, Yaseen, Zaher Mundher and Deo, Ravinesh C.. 2025. "Intelligent modeling and analysis of hybrid organic Rankine plants: Data-driven insights into thermodynamic efficiency and economic viability." Engineering Applications of Artificial Intelligence. 143. https://doi.org/10.1016/j.engappai.2024.109946EEGConvNeXt: A novel convolutional neural network model for automated detection of Alzheimer's Disease and Frontotemporal Dementia using EEG signals
Acharya, Madhav, Deo, Ravinesh C, Barua, Prabal Datta, Devi, Aruna and Tao, Xiaohui. 2025. "EEGConvNeXt: A novel convolutional neural network model for automated detection of Alzheimer's Disease and Frontotemporal Dementia using EEG signals." Computer Methods and Programs in Biomedicine. 262. https://doi.org/10.1016/j.cmpb.2025.108652Electricity demand uncertainty modeling with Temporal Convolution Neural Network models
Ghimire, Sujan, Deo, Ravinesh C., Casillas-Perez, David, Salcedo-Sanz, Sancho, Acharya, Rajendra and Dinh, Toan. 2025. "Electricity demand uncertainty modeling with Temporal Convolution Neural Network models." Renewable and Sustainable Energy Reviews. 209. https://doi.org/10.1016/j.rser.2024.115097Half-hourly electricity price prediction model with explainable-decomposition hybrid deep learning approach
Ghimire, Sujan, Deo, Ravinesh C., Hopf, Konstantin, Liu, Hangyue, Casillas-Perez, David, Helwig, Andreas, Prasad, Salvin S., Perez-Aracil, Jorge, Barua, Prabal Datta and Salcedo-Sanz, Sancho. 2025. "Half-hourly electricity price prediction model with explainable-decomposition hybrid deep learning approach." Energy and AI. 20. https://doi.org/10.1016/j.egyai.2025.100492Explainable deep learning hybrid modeling framework for total suspended particles concentrations prediction
Ghimire, Sujan, Deo, Ravinesh C., Jiang, Ningbo, Ahmed, A. A. Masrur, Prasad, Salvin S., Casillas-Perez, David, Salcedo-Sanz, Sancho and Yaseen, Zaher Mundher. 2025. "Explainable deep learning hybrid modeling framework for total suspended particles concentrations prediction." Atmospheric Environment. 347. https://doi.org/10.1016/j.atmosenv.2025.121079Generating High Spatial and Temporal Surface Albedo with Multispectral-Wavemix and Temporal-Shift Heatmaps
Karalasingham, Sagthitharan, Deo, Ravinesh C., Raj, Nawin, Casillas-Perez, David and Salcedo-Sanz, Sancho. 2025. "Generating High Spatial and Temporal Surface Albedo with Multispectral-Wavemix and Temporal-Shift Heatmaps." Remote Sensing. 17 (3). https://doi.org/10.3390/rs17030461Explainable hybrid deep learning framework for enhancing multi-step solar ultraviolet-B radiation predictions
Prasad, Salvin S., Joseph, Lionel P., Ghimire, Sujan, Deo, Ravinesh C., Downs, Nathan J., Acharya, Rajendra and Yaseen, Zaher M.. 2025. "Explainable hybrid deep learning framework for enhancing multi-step solar ultraviolet-B radiation predictions." Atmospheric Environment. 343. https://doi.org/10.1016/j.atmosenv.2024.120951Enhanced water quality prediction model using advanced hybridized resampling alternating tree-based and deep learning algorithms
Khosravi, Khabat, Farooque, Aitazaz Ahsan, Karbasi, Masoud, Ali, Mumtaz, Heddam, Salim, Faghfouri, Ali and Abolfathi, Soroush. 2025. "Enhanced water quality prediction model using advanced hybridized resampling alternating tree-based and deep learning algorithms." Environmental Science and Pollution Research. 32 (11), pp. 6405-6424. https://doi.org/10.1007/s11356-025-36062-7A hybrid framework: singular value decomposition and kernel ridge regression optimized using mathematical-based fine-tuning for enhancing river water level forecasting
Ahmadianfar, Iman, Farooque, Aitazaz Ahsan, Ali, Mumtaz, Jamei, Mehdi, Jamei, Mozhdeh and Yaseen, Zaher Mundher. 2025. "A hybrid framework: singular value decomposition and kernel ridge regression optimized using mathematical-based fine-tuning for enhancing river water level forecasting." Scientific Reports. 15. https://doi.org/10.1038/s41598-025-90628-6Systematic Review of AI and Machine Learning Approaches for Predicting Crop Diseases in the Context of Climate Change and the Food Security
Kaur, Avneet, Randhawa, Gurjit S., Farooque, Aitazaz A., Singh, Rajandeep, Ali, Mumtaz and Zaman, Qamar U.. 2025. "Systematic Review of AI and Machine Learning Approaches for Predicting Crop Diseases in the Context of Climate Change and the Food Security." Filho, Walter Leal, Matandirotya, Newton, Ayal, Desalegn Yayeh, Luetz, Johannes M. and Borsari, Bruno (ed.) Climate Change, Food Security, and Land Management: Strategies for a Sustainable Future. Switzerland. Springer. pp. 1-20Designing empirical fourier decomposition reinforced with multiscale increment entropy and deep learning to forecast dry bulb air temperature
Diykh, Mohammed, Ali, Mumtaz, Labban, Abdulhaleem H., Prasad, Ramendra, Jamei, Mehdi, Abdulla, Shahab and Farooque, Aitazaz Ahsan. 2025. "Designing empirical fourier decomposition reinforced with multiscale increment entropy and deep learning to forecast dry bulb air temperature." Results in Engineering. 26. https://doi.org/10.1016/j.rineng.2025.104597Multi‑step solar ultraviolet index prediction: integrating convolutional neural networks with long short‑term memory for a representative case study in Queensland, Australia
AL-Musaylh, Mohan, Al‑Dafaie, Kadhem, Downs, Nathan, Ghimire, Sujan, Ali, Mumtaz, Yaseen, Zaher Mundher, Igoe, Damien P., Deo, Ravinesh C., Paris, Alfo V. and A. Jebar, Mustapha A.. 2025. "Multi‑step solar ultraviolet index prediction: integrating convolutional neural networks with long short‑term memory for a representative case study in Queensland, Australia." Modeling Earth Systems and Environment. 11. https://doi.org/10.1007/s40808-024-02282-yImproving daily reference evapotranspiration forecasts: Designing AI-enabled recurrent neural networks based long short-term memory
Ali, Mumtaz, Nayahi, Jesu Vedha, Abdi, Erfan, Ghorbani, Mohammad Ali, Mohajeri, Farzan, Farooque, Aitazaz A. and Alamery, Salman. 2025. "Improving daily reference evapotranspiration forecasts: Designing AI-enabled recurrent neural networks based long short-term memory ." Ecological Informatics. 85. https://doi.org/10.1016/j.ecoinf.2025.102995A Review of APHRODITE Performance in Asia: Current Status and Research Concerns
Tan, Mou Leong, Goliatt, Leonardo, Heddam, Salim, Maulud, Khairul Nizam Abdul, Halder, Bijay, Sa’adi, Zulfaqar, Ahmadianfar, Iman, Shafik, Shafik S., Ali, Mumtaz and Yaseen, Zaher Mundher. 2025. "A Review of APHRODITE Performance in Asia: Current Status and Research Concerns." Journal of Hydrology. 651. https://doi.org/10.1016/j.jhydrol.2024.132562An Explainable Predictive Approach for Investigation of Greenhouse Gas Emissions in Maritime Canada's Potato Agriculture
Jamei, Mehdi, Yaqoob, Nauman, Farooque, Aitazaz A., Ali, Mumtaz, Malik, Anurag, Esau, Travis J. and Hu, Yulin. 2025. "An Explainable Predictive Approach for Investigation of Greenhouse Gas Emissions in Maritime Canada's Potato Agriculture." Smart Agricultural Technology . 10. https://doi.org/10.1016/j.atech.2024.100709Explainable deeply-fused nets electricity demand prediction model: Factoring climate predictors for accuracy and deeper insights with probabilistic confidence interval and point-based forecasts
Ghimire, Sujan, AL-Musaylh, Mohanad S., Nguyen-Huy, Thong, Deo, Ravinesh C., Acharya, Rajendra, Casillas-Perez, David, Yaseen, Zaher Mundher and Salcedo-sanz, Sancho. 2025. "Explainable deeply-fused nets electricity demand prediction model: Factoring climate predictors for accuracy and deeper insights with probabilistic confidence interval and point-based forecasts." Applied Energy. 378 (Part A). https://doi.org/10.1016/j.apenergy.2024.124763Near real-time significant wave height prediction along the coastline of Queensland using advanced hybrid machine learning models
Khosravi, K., Ali, M. and Heddam, S.. 2025. "Near real-time significant wave height prediction along the coastline of Queensland using advanced hybrid machine learning models." International Journal of Environmental Science and Technology. 22 (7), pp. 5309-5326. https://doi.org/10.1007/s13762-024-05944-7The mitigating effect of street trees, urban flora, and the suburban environment on seasonal peak UV indices: A case study from Brisbane, Australia
Downs, Nathan James, Amar, Abdurazaq, Dearnaley, John, Butler, Harry, Dekeyser, Stijn, Igoe, Damien, Parisi, Alfio V., Raj, Nawin, Deo, Ravinesh and Turner, Joanna. 2025. "The mitigating effect of street trees, urban flora, and the suburban environment on seasonal peak UV indices: A case study from Brisbane, Australia." Photochemistry and Photobiology. 101 (1), pp. 251-266. https://doi.org/10.1111/php.13988The Application of Quantum Pre-processing Filter for Binary Image Classification with Small Samples
Riaz, Farina, Abdulla, Shahab, Suzuki, Hajime, Ganguly, Srinjoy, Deo, Ravinesh C. and Hopkins, Susan. 2024. "The Application of Quantum Pre-processing Filter for Binary Image Classification with Small Samples ." Journal of Data Science and Intelligent Systems. 3 (2), pp. 109-116. https://doi.org/10.47852/bonviewJDSIS42024229A Comprehensive Review of UAV-UGV Collaboration: Advancements and Challenges
Munasinghe, Isuru, Perera, Asanka and Deo, Ravinesh C.. 2024. "A Comprehensive Review of UAV-UGV Collaboration: Advancements and Challenges." Journal of Sensor and Actuator Networks. 13 (6). https://doi.org/10.3390/jsan13060081Retinal Health Screening Using Artificial Intelligence with Digital Fundus Images: A Review of the Last Decade (2012-2023)
Islam, Saad, Deo, Ravinesh C., Barua, Prabal Datta, Soar, Jeffrey, Yu, Ping and Acharya, U. Rajendra. 2024. "Retinal Health Screening Using Artificial Intelligence with Digital Fundus Images: A Review of the Last Decade (2012-2023)." IEEE Access. 12, pp. 176630-176685. https://doi.org/10.1109/ACCESS.2024.3477420Artificial Intelligence Driven Smart Farming for Accurate Detection of Potato Diseases: A Systematic Review
Kaur, Avneet, Randhawa, Gurjit S., Abbas, Farhat, Ali, Mumtaz, Esau, Travis J., Farooque, Aitazaz A. and Singh, Rajandeep. 2024. "Artificial Intelligence Driven Smart Farming for Accurate Detection of Potato Diseases: A Systematic Review." IEEE Access. 12, pp. 193902-193922. https://doi.org/10.1109/ACCESS.2024.3510456Forecasting River Water Temperature Using Explainable Artificial Intelligence and Hybrid Machine Learning: Case Studies in Menindee Region in Australia
Briceno Medina, Leyde, Joehnk, Klaus, Deo, Ravinesh C., Ali, Mumtaz, Prasad, Salvin S. and Downs, Nathan. 2024. "Forecasting River Water Temperature Using Explainable Artificial Intelligence and Hybrid Machine Learning: Case Studies in Menindee Region in Australia." Water. 16 (24). https://doi.org/10.3390/w16243720A designed predictive modelling strategy based on data decomposition and machine learning to forecast solar radiation
Ali, Mumtaz, Prasad, Ramendra, Alamery, Salman and Farooque, Aitazaz Ahsan. 2024. "A designed predictive modelling strategy based on data decomposition and machine learning to forecast solar radiation." Results in Engineering. 24. https://doi.org/10.1016/j.rineng.2024.103607Explainable artificial intelligence-machine learning models to estimate overall scores in tertiary preparatory general science course
Ghimire, Sujan, Abdulla, Shahab, Joseph, Lionel P., Prasad, Salvin, Murphy, Angela, Devi, Aruna, Barua, Prabal Datta, Deo, Ravinesh C., Acharya, Rajendra and Yaseen, Zaher Mundher. 2024. "Explainable artificial intelligence-machine learning models to estimate overall scores in tertiary preparatory general science course." Computers and Education: Artificial Intelligence. 7. https://doi.org/10.1016/j.caeai.2024.100331Spatiotemporal performance evaluation of high-resolution multiple satellite and reanalysis precipitation products over the semiarid region of India
Devadarshini, Elangovan, Bhuvaneswari, Kulanthaivelu, Kumar, Shanmugam Mohan, Geethalakshmi, Vellingiri, Dhasarathan, Manickam, Senthil, Alagarsamy, Senthilraja, Kandasamy, Mushtaq, Shahbaz, Nguyen-Huy, Thong, Mai, Thanh and Kouadio, Louis. 2024. "Spatiotemporal performance evaluation of high-resolution multiple satellite and reanalysis precipitation products over the semiarid region of India." Environmental Monitoring and Assessment. 196. https://doi.org/10.1007/s10661-024-13152-6Forecasting Multi-Step Soil Moisture with Three-Phase Hybrid Wavelet-Least Absolute Shrinkage Selection Operator-Long Short-Term Memory Network (moDWT-Lasso-LSTM) Model
Jayasinghe, W. J. M. Lakmini Prarthana, Deo, Ravinesh C., Raj, Nawin, Ghimire, Sujan, Yaseen, Zaher Mundher, Nguyen-Huy, Thong and Ghahramani, Afshin. 2024. "Forecasting Multi-Step Soil Moisture with Three-Phase Hybrid Wavelet-Least Absolute Shrinkage Selection Operator-Long Short-Term Memory Network (moDWT-Lasso-LSTM) Model." Water. 16 (21), p. 3133. https://doi.org/10.3390/w16213133Harnessing a systems approach for sustainable adaptation in vulnerable mega-deltas: A case study of the dyke heightening program in the Vietnamese Mekong Delta's floodplains
Mai, Thanh, Mushtaq, Shahbaz, Tong, Yen Dan, Nguyen-Huy, Thong, Richards, Russell and Marcussen, Torben. 2024. "Harnessing a systems approach for sustainable adaptation in vulnerable mega-deltas: A case study of the dyke heightening program in the Vietnamese Mekong Delta's floodplains." Science of the Total Environment. 954. https://doi.org/10.1016/j.scitotenv.2024.176501Monitoring of Greenhouse Gas Emission Drivers in Atlantic Canadian Potato Production: A Robust Explainable Intelligent Glass-Box
Jamei, Mehdi, Hassan, Muhammad, Farooque, Aitazaz A., Ali, Mumtaz, Karbasi, Masoud, Randhawa, Gurjit, Yaseen, Zaher Mundher and Dwyer, Ross. 2024. "Monitoring of Greenhouse Gas Emission Drivers in Atlantic Canadian Potato Production: A Robust Explainable Intelligent Glass-Box." Results in Engineering. 24. https://doi.org/10.1016/j.rineng.2024.103297Innovative Multi-Temporal Evapotranspiration Forecasting Using Empirical Fourier Decomposition and Bidirectional Long Short-Term Memory
Karbasi, Masoud, Ali, Mumtaz, Randhawa, Gurjit S., Jamei, Mehdi, Malik, Anurag, Hussain, Syed Hamid Hussain, Bos, Melanie, Zaman, Qamar and Farooque, Aitazaz Ahsan. 2024. "Innovative Multi-Temporal Evapotranspiration Forecasting Using Empirical Fourier Decomposition and Bidirectional Long Short-Term Memory." Smart Agricultural Technology . 9. https://doi.org/10.1016/j.atech.2024.100619Real-time prediction of the week-ahead flood index using hybrid deep learning algorithms with synoptic climate mode indices
Ahmed, A.A. Masrur, Akther, Shahida, Nguyen-Huy, Thong, Raj, Nawin, Jui (Student), S Janifer and S.Z., Farzana. 2024. "Real-time prediction of the week-ahead flood index using hybrid deep learning algorithms with synoptic climate mode indices." Journal of Hydro-Environment Research. 57, pp. 12-26. https://doi.org/10.1016/j.jher.2024.09.001Artificial Intelligence-Empowered Doppler Weather Profile for Low-Earth-Orbit Satellites
Sharma, Ekta, Deo, Ravinesh C., Davey, Christopher P and Carter, Brad D.. 2024. "Artificial Intelligence-Empowered Doppler Weather Profile for Low-Earth-Orbit Satellites." Sensors. 24 (16). https://doi.org/10.3390/s24165271Pyrolytic Pathway of Wheat Straw Pellet by the Thermogravimetric Analyzer
Nath, Bidhan, Bowtell, Les, Chen, Guangnan, Graham, Elizabeth and Nguyen-Huy, Thong. 2024. "Pyrolytic Pathway of Wheat Straw Pellet by the Thermogravimetric Analyzer." Energies. 17 (15). https://doi.org/10.3390/en17153693Multi-step ahead forecasting of electrical conductivity in rivers by using a hybrid Convolutional Neural Network-Long Short-Term Memory (CNN-LSTM) model enhanced by Boruta-XGBoost feature selection algorithm
Karbasi, Masoud, Ali, Mumtaz, Bateni, Sayed M., Jun, Changhyun, Jamei, Mehdi, Farooque, Aitazaz Ahsan and Yaseen, Zaher Mundher. 2024. "Multi-step ahead forecasting of electrical conductivity in rivers by using a hybrid Convolutional Neural Network-Long Short-Term Memory (CNN-LSTM) model enhanced by Boruta-XGBoost feature selection algorithm." Scientific Reports. 14 (1). https://doi.org/10.1038/s41598-024-69309-3Wavelet-fusion image super-resolution model with deep learning for downscaling remotely-sensed, multi-band spectral albedo imagery
Karalasingham, Sagthitharan, Deo, Ravinesh C., Casillas-Perez, David, Raj, Nawin and Salcedo-sanz, Sancho. 2024. "Wavelet-fusion image super-resolution model with deep learning for downscaling remotely-sensed, multi-band spectral albedo imagery." Remote Sensing Applications: Society and Environment. 36. https://doi.org/10.1016/j.rsase.2024.101333Half-hourly electricity price prediction with a hybrid convolution neural network-random vector functional link deep learning approach
Ghimire, Sujan, Deo, Ravinesh C., Casillas-Perez, David, Sharma, Ekta, Salcedo-sanz, Sancho, Barua, Prabal and Acharya, U. Rajendra. 2024. "Half-hourly electricity price prediction with a hybrid convolution neural network-random vector functional link deep learning approach." Applied Energy. 374. https://doi.org/10.1016/j.apenergy.2024.123920Towards next-generation federated learning: A case study on privacy attacks in artificial intelligence systems
Sharma, Ekta, Deo, Ravinesh C, Davey, Christopher P., Carter, Brad D. and Salcedo-sanz, Sancho. 2024. "Towards next-generation federated learning: A case study on privacy attacks in artificial intelligence systems." 2024 IEEE Conference on Artificial Intelligence (CAI 2024). Singapore 25 - 27 Jun 2024 United States. IEEE (Institute of Electrical and Electronics Engineers). https://doi.org/10.1109/CAI59869.2024.00259Poster: Cloud Computing with AI-empowered Trends in Software-Defined Radios: Challenges and Opportunities
Sharma, Ekta, Deo, Ravinesh C., Davey, Christopher P., Carter, Brad D. and Salcedo-sanz, Sancho. 2024. "Poster: Cloud Computing with AI-empowered Trends in Software-Defined Radios: Challenges and Opportunities." 2024 IEEE 25th International Symposium on a World of Wireless, Mobile and Multimedia Networks (WoWMoM 2024). Perth, Australia 04 - 07 Jun 2024 United States. IEEE (Institute of Electrical and Electronics Engineers). https://doi.org/10.1109/WoWMoM60985.2024.00054Robust drought forecasting in Eastern Canada: Leveraging EMD-TVF and ensemble deep RVFL for SPEI index forecasting
Karbasi, Masoud, Ali, Mumtaz, Farooque, Aitazaz Ahsan, Jamei, Mehdi, Khosravi, Khabat, Cheema, Saad Javed and Yaseen, Zaher Mundher. 2024. "Robust drought forecasting in Eastern Canada: Leveraging EMD-TVF and ensemble deep RVFL for SPEI index forecasting." Expert Systems with Applications. 256. https://doi.org/10.1016/j.eswa.2024.124900Atmospheric Visibility and Cloud Ceiling Predictions With Hybrid IIS-LSTM Integrated Model: Case Studies for Fiji's Aviation Industry
Raj, Shiveel, Deo, Ravinesh C., Sharma, Ekta, Prasad, Ramendra, Dinh, Toan and Salcedo-sanz, Sancho. 2024. "Atmospheric Visibility and Cloud Ceiling Predictions With Hybrid IIS-LSTM Integrated Model: Case Studies for Fiji's Aviation Industry." IEEE Access. 12, pp. 72530-72543. https://doi.org/10.1109/ACCESS.2024.3401091Point-based and probabilistic electricity demand prediction with a Neural Facebook Prophet and Kernel Density Estimation model
Ghimire, Sujan, Deo, Ravinesh C., Pourmousavi, S. Ali, Casillas-Perez, David and Salcedo-sanz, Sancho. 2024. "Point-based and probabilistic electricity demand prediction with a Neural Facebook Prophet and Kernel Density Estimation model." Engineering Applications of Artificial Intelligence. 135. https://doi.org/10.1016/j.engappai.2024.108702End-to-end learning of adaptive coded modulation schemes for resilient wireless communications
Davey, Christopher P., Shakeel, Ismail, Deo, Ravinesh C., Sharma, Ekta, Salcedo-sanz, Sancho and Soar, Jeffrey. 2024. "End-to-end learning of adaptive coded modulation schemes for resilient wireless communications." Applied Soft Computing. 159. https://doi.org/10.1016/j.asoc.2024.111672Deep Learning Based Over-the-Air Training of Wireless Communication Systems without Feedback
Davey, Christopher P., Shakeel, Ismail, Deo, Ravinesh C. and Salcedo-sanz, Sancho. 2024. "Deep Learning Based Over-the-Air Training of Wireless Communication Systems without Feedback." Sensors. 24 (10). https://doi.org/10.3390/s24102993Pyrolysis of wheat straw pellets in a pilot-scale reactor: Effect of temperature and residence time
Nath, Bidhan, Chen, Guangnan, Bowtell, Les and Nguyen-Huy, Thong. 2024. "Pyrolysis of wheat straw pellets in a pilot-scale reactor: Effect of temperature and residence time." Energy Science and Engineering. 12 (8), pp. 3524-3539. https://doi.org/10.1002/ese3.1833Integrating rainfall index-based insurance with optimal crop management strategies can reduce financial risks for Australian dryland cotton farmers
Nguyen-Huy, Thong, Kath, Jarrod, Kouadio, Louis, King, Rachel, Mushtaq, Shahbaz and Barratt, Jonathan. 2024. "Integrating rainfall index-based insurance with optimal crop management strategies can reduce financial risks for Australian dryland cotton farmers." Sustainable Futures. 8. https://doi.org/10.1016/j.sftr.2024.100249Multi-step ahead forecasting of electrical conductivity in rivers by using a hybrid Convolutional Neural Network-Long Short-Term Memory (CNN-LSTM) model enhanced by Boruta-XGBoost feature selection algorithm
Karbasi, Masoud, Ali, Mumtaz, Bateni, Sayed M., Jun, Changhyun, Jamei, Mehdi, Farooque, Aitazaz Ahsan and Yaseen, Zaher Mundher. 2024. "Multi-step ahead forecasting of electrical conductivity in rivers by using a hybrid Convolutional Neural Network-Long Short-Term Memory (CNN-LSTM) model enhanced by Boruta-XGBoost feature selection algorithm." Scientific Reports. 14. https://doi.org/10.1038/s41598-024-65837-0A playground shade index for standardising ultraviolet protection assessments of open, mixed and protected outdoor recreational spaces
Downs, Nathan, Butler, Harry, Dexter, Benjamin, Raj, Nawin, Downs, Melanie, Turner, Joanna, Dekeyser, Stijn, Deo, Ravinesh, Vanos, Jennifer, Igoe, Damien and Parisi, Alfio V. 2024. "A playground shade index for standardising ultraviolet protection assessments of open, mixed and protected outdoor recreational spaces." 6th International conference UV and Skin Cancer Prevention. Brisbane, Australia 11 - 15 Sep 2024 Australia.Multi-Step-Ahead Wind Speed Forecast System: Hybrid Multivariate Decomposition and Feature Selection-Based Gated Additive Tree Ensemble Model
Joseph, Lionel P., Deo, Ravinesh C., Casillas-Perez, David, Prasad, Ramendra, Raj, Nawin and Salcedo-sanz, Sancho. 2024. "Multi-Step-Ahead Wind Speed Forecast System: Hybrid Multivariate Decomposition and Feature Selection-Based Gated Additive Tree Ensemble Model." IEEE Access. 12, pp. 58750-58777. https://doi.org/10.1109/ACCESS.2024.3392899Enhancing groundwater level prediction accuracy using interpolation techniques in deep learning models
Abdi, Erfan, Ali, Mumtaz, Guimarães Santos, Celso Augusto, Olusola, Adeyemi and Ghorbani, Mohammad Ali. 2024. "Enhancing groundwater level prediction accuracy using interpolation techniques in deep learning models." Groundwater for Sustainable Development. 26. https://doi.org/10.1016/j.gsd.2024.101213Deep Learning-Assisted Sensitive 3C-SiC Sensor for Long-Term Monitoring of Physical Respiration
Tran, Thi Lap, Nguyen, Duy Van, Nguyen, Hung, Nguyen, Thi Phuoc Van, Song, Pingan, Deo, Ravinesh C, Moloney, Clint, Dao, Viet Dung, Nguyen, Nam-Trung and Dinh, Toan. 2024. "Deep Learning-Assisted Sensitive 3C-SiC Sensor for Long-Term Monitoring of Physical Respiration." Advanced Sensor Research. 3 (8). https://doi.org/10.1002/adsr.202300159Copula-Probabilistic Flood Risk Analysis with an Hourly Flood Monitoring Index
Chand, Ravinesh, Nguyen-Huy, Thong, Deo, Ravinesh C., Ghimire, Sujan, Ali, Mumtaz and Ghahramani, Afshin. 2024. "Copula-Probabilistic Flood Risk Analysis with an Hourly Flood Monitoring Index." Water. 16 (11). https://doi.org/10.3390/w16111560Empirical curvelet transform based deep DenseNet model to predict NDVI using RGB drone imagery data
Diykh, Mohammed, Ali, Mumtaz, Jamei, Mehdi, Abdulla, Shahab, Uddin, Md Palash, Farooque, Aitazaz Ahsan, Labban, Abdulhaleem H. and Alabdally, Hussein. 2024. "Empirical curvelet transform based deep DenseNet model to predict NDVI using RGB drone imagery data." Computers and Electronics in Agriculture. 221. https://doi.org/10.1016/j.compag.2024.108964Short-term wind speed forecasting using an optimized three-phase convolutional neural network fused with bidirectional long short-term memory network model
Joseph, Lionel P., Deo, Ravinesh C., Casillas-Perez, David, Prasad, Ramendra, Raj, Nawin and Salcedo-sanz, Sancho. 2024. "Short-term wind speed forecasting using an optimized three-phase convolutional neural network fused with bidirectional long short-term memory network model." Applied Energy. 359. https://doi.org/10.1016/j.apenergy.2024.122624Probabilistic-based electricity demand forecasting with hybrid convolutional neural network-extreme learning machine model
Ghimire, Sujan, Deo, Ravinesh C., Casillas-Perez, David, Salcedo-sanz, Sancho, Pourmousavi, S. Ali and Acharya, U. Rajendra. 2024. "Probabilistic-based electricity demand forecasting with hybrid convolutional neural network-extreme learning machine model." Engineering Applications of Artificial Intelligence. 132. https://doi.org/10.1016/j.engappai.2024.107918Electricity demand error corrections with attention bi-directional neural networks
Ghimire, Sujan, Deo, Ravinesh C., Casillas-Perez, David and Salcedo-sanz, Sancho. 2024. "Electricity demand error corrections with attention bi-directional neural networks." Energy. 291. https://doi.org/10.1016/j.energy.2023.129938Micromachined Mechanical Resonant Sensors: From Materials, Structural Designs to Applications
Dinh, Toan, Rais-Zadeh, Mina, Nguyen, Thanh, Phan, Hoang-Phuong, Song, Pingan, Deo, Ravinesh, Dao, Dzung, Nguyen, Nam-Trung and Bell, John. 2024. "Micromachined Mechanical Resonant Sensors: From Materials, Structural Designs to Applications." Advanced Materials Technologies. 9 (2). https://doi.org/10.1002/admt.202300913Machine learning for expediting next-generation of fire-retardant polymer composites
Jafari, Pooya, Zhang, Ruoran, Huo, Siqi, Wang, Qingsheng, Yong, Jianming, Hong, Min, Deo, Ravinesh, Wang, Hao and Song, Pingan. 2024. "Machine learning for expediting next-generation of fire-retardant polymer composites." Composites Communications. 45. https://doi.org/10.1016/j.coco.2023.101806Quantitative improvement of streamflow forecasting accuracy in the Atlantic zones of Canada based on hydro-meteorological signals: A multi-level advanced intelligent expert framework
Jamei, Mozhdeh, Jamei, Mehdi, Ali, Mozhdeh, Karbasi, Masoud, Farooque, Aitazaz A., Malik, Anurag, Cheema, Saad Javed, Esau, Travis J. and Yaseen, Zaher Mundher. 2024. "Quantitative improvement of streamflow forecasting accuracy in the Atlantic zones of Canada based on hydro-meteorological signals: A multi-level advanced intelligent expert framework." Ecological Informatics. 80. https://doi.org/10.1016/j.ecoinf.2023.102455Short-term drought Index forecasting for hot and semi-humid climate Regions: A novel empirical Fourier decomposition-based ensemble Deep-Random vector functional link strategy
Jamei, Mehdi, Ali, Mumtaz, Bateni, Sayed M., Jun, Changhyun, Karbasi, Masoud, Malik, Anurag, Jamei, Mozhdeh and Yaseen, Zaher Mundher. 2024. "Short-term drought Index forecasting for hot and semi-humid climate Regions: A novel empirical Fourier decomposition-based ensemble Deep-Random vector functional link strategy ." Computers and Electronics in Agriculture. 217. https://doi.org/10.1016/j.compag.2023.108609Very short-term solar ultraviolet-A radiation forecasting system with cloud cover images and a Bayesian optimized interpretable artificial intelligence model
Prasad, Salvin Sanjesh, Deo, Ravinesh Chand, Downs, Nathan James, Casillas-Perez, David, Salcedo-sanz, Sancho and Parisi, Alfio Venerando. 2024. "Very short-term solar ultraviolet-A radiation forecasting system with cloud cover images and a Bayesian optimized interpretable artificial intelligence model ." Expert Systems with Applications. 236. https://doi.org/10.1016/j.eswa.2023.121273Short-term wave power forecasting with hybrid multivariate variational mode decomposition model integrated with cascaded feedforward neural networks
Ali, Mumtaz, Prasad, Ramendra, Jamei, Mehdi, Malik, Anurag, Xiang, Yong, Abdulla, Shahab, Deo, Ravinesh C., Farooque, Aitazaz A. and Labban, Abdulhaleem H.. 2024. "Short-term wave power forecasting with hybrid multivariate variational mode decomposition model integrated with cascaded feedforward neural networks." Renewable Energy. 221. https://doi.org/10.1016/j.renene.2023.119773Accurate monitoring of micronutrients in tilled potato soils of eastern Canada: Application of an eXplainable inspired-adaptive boosting framework coupled with SelectKbest
Jamei, Mehdi, Ali, Mumtaz, Afzaal, Hassan, Karbasi, Masoud, Malik, Anurag, Farooque, Aitazaz Ahsan, Haydar, Zeeshan and Zaman, Qamar Uz. 2024. "Accurate monitoring of micronutrients in tilled potato soils of eastern Canada: Application of an eXplainable inspired-adaptive boosting framework coupled with SelectKbest." Computers and Electronics in Agriculture. 216. https://doi.org/10.1016/j.compag.2023.108479Application of an explainable glass-box machine learning approach for prognostic analysis of a biogas-powered small agriculture engine
Jamei, Mehdi, Sharma, Prabhakar, Ali, Mumtaz, Bora, Bhaskor J., Malik, Anurag, Paramasivam, Prabhu, Farooque, Aitazaz A. and Abdulla, Shahab. 2024. "Application of an explainable glass-box machine learning approach for prognostic analysis of a biogas-powered small agriculture engine." Energy. 288. https://doi.org/https://doi.org/10.1016/j.energy.2023.129862Boruta extra tree-bidirectional long short-term memory model development for Pan evaporation forecasting: Investigation of arid climate condition
Karbasi, Masoud, Ali, Mumtaz, Bateni, Sayed M., Jun, Changhyun, Jamei, Mehdi and Yaseen, Zaher Mundher. 2024. "Boruta extra tree-bidirectional long short-term memory model development for Pan evaporation forecasting: Investigation of arid climate condition." Alexandria Engineering Journal. 86, pp. 425-442. https://doi.org/10.1016/j.aej.2023.11.061Hybridized artificial intelligence models with nature-inspired algorithms for river flow modeling: A comprehensive review, assessment, and possible future research directions
Tao, Hai, Abba, Sani I., Al-Areeq, Ahmed M., Tangang, Fredolin, Samantaray, Sandeep, Sahoo, Abinash, Siqueira, Hugo Valadares, Maroufpoor, Saman, Demir, Vahdettin, Bokde, Neeraj Dhanraj, Goliatt, Leonardo, Jamei, Mehdi, Ahmadianfar, Iman, Bhagat, Suraj Kumar, Halder, Bijay, Guo, Tianli, Helman, Daniel S., Ali, Mumtaz, Sattar, Sabaa, ..., Yaseen, Zaher Mundher. 2024. "Hybridized artificial intelligence models with nature-inspired algorithms for river flow modeling: A comprehensive review, assessment, and possible future research directions ." Engineering Applications of Artificial Intelligence. 129. https://doi.org/10.1016/j.engappai.2023.107559Two-step deep learning framework with error compensation technique for short-term, half-hourly electricity price forecasting
Ghimire, Sujan, Deo, Ravinesh C., Casillas-Perez, David and Salcedo-sanz, Sancho. 2024. "Two-step deep learning framework with error compensation technique for short-term, half-hourly electricity price forecasting." Applied Energy. 353 (Part A). https://doi.org/10.1016/j.apenergy.2023.122059Adaptive Regularization and Resilient Estimation in Federated Learning
Uddin, Md Palash, Xiang, Yong, Zhao, Yao, Ali, Mumtaz, Zhang, Yushu and Gao, Longxiang. 2024. "Adaptive Regularization and Resilient Estimation in Federated Learning." IEEE Transactions on Services Computing. 17 (4), pp. 1369-1381. https://doi.org/10.1109/TSC.2023.3332703An advanced deep learning predictive model for air quality index forecasting with remote satellite-derived hydro-climatological variables
Ahmed, Abul Abrar Masrur, Jui, S. Janifer Jabin, Sharma, Ekta, Ahmed, Mohammad Hafez, Raj, Nawin and Bose, Aditi. 2024. "An advanced deep learning predictive model for air quality index forecasting with remote satellite-derived hydro-climatological variables." Science of the Total Environment. 906. https://doi.org/10.1016/j.scitotenv.2023.167234Hybrid Deep Learning Model for Wave Height Prediction in Australia's Wave Energy Region
Ahmed, Abul Abrar Masrur, Jui, S Janifer Jabin, AL-Musaylh, Mohanad S., Raj, Nawin, Saha, Reepa, Deo, Ravinesh C and Saha, Sanjoy Kumar. 2024. "Hybrid Deep Learning Model for Wave Height Prediction in Australia's Wave Energy Region." Applied Soft Computing. 150. https://doi.org/10.1016/j.asoc.2023.111003Gully erosion mapping susceptibility in a Mediterranean environment: A hybrid decision-making model
Hitouri, Sliman, Meriame, Mohajane, Ajim, Ali Sk, Pacheco, Quevedo Renata, Nguyen-Huy, Thong, Pham, Quoc Bao, ElKhrachy, Ismail and Varasano, Antonietta. 2024. "Gully erosion mapping susceptibility in a Mediterranean environment: A hybrid decision-making model." International Soil and Water Conservation Research. 12 (2), pp. 279-297. https://doi.org/10.1016/j.iswcr.2023.09.008Monthly sodium adsorption ratio forecasting in rivers using a dual interpretable glass-box complementary intelligent system: Hybridization of ensemble TVF-EMD-VMD, Boruta-SHAP, and eXplainable GPR
Jamei, Mehdi, Ali, Mumtaz, Karbasi, Masoud, Karimi, Bakhtiar, Jahannemaei, Neshat, Farooque, Aitazaz Ahsan and Yaseen, Zaher Mundher. 2024. "Monthly sodium adsorption ratio forecasting in rivers using a dual interpretable glass-box complementary intelligent system: Hybridization of ensemble TVF-EMD-VMD, Boruta-SHAP, and eXplainable GPR." Expert Systems with Applications. 237 (Part B). https://doi.org/10.1016/j.eswa.2023.121512Modelling future spatial distribution of peanut crops in Australia under climate change scenarios
Haerani, Haerani, Apan, Armando, Nguyen-Huy, Thong and Basnet, Badri. 2024. "Modelling future spatial distribution of peanut crops in Australia under climate change scenarios." Geo-spatial Information Science. 27 (5), pp. 1585-1604. https://doi.org/10.1080/10095020.2022.2155255Evaluation and Prediction of Groundwater Quality for Irrigation Using an Integrated Water Quality Indices, Machine Learning Models and GIS Approaches: A Representative Case Study
Ibrahim, Hekmat, Yaseen, Zaher Mundher, Scholz, Miklas, Ali, Mumtaz, Gad, Mohamed, Elsayed, Salah, Khadr, Mosaad, Hussein, Hend, Ibrahim, Hazem H., Eid, Mohamed Hamdy, Kovács, Attila, Péter, Szűcs and Khalifa, Moataz M.. 2023. "Evaluation and Prediction of Groundwater Quality for Irrigation Using an Integrated Water Quality Indices, Machine Learning Models and GIS Approaches: A Representative Case Study." Water. 15 (4). https://doi.org/10.3390/w15040694A high dimensional features-based cascaded forward neural network coupled with MVMD and Boruta-GBDT for multi-step ahead forecasting of surface soil moisture
Jamei, Mehdi, Ali, Mumtaz, Karbasi, Masoud, Sharma, Ekta, Jamei, Mozhdeh, Chu, Xuefeng and Yaseen, Zaher Mundher. 2023. "A high dimensional features-based cascaded forward neural network coupled with MVMD and Boruta-GBDT for multi-step ahead forecasting of surface soil moisture." Engineering Applications of Artificial Intelligence. 120. https://doi.org/10.1016/j.engappai.2023.105895Author Correction: Explainable AI approach with original vegetation data classifies spatio‑temporal nitrogen in flows from ungauged catchments to the Great Barrier Reef
O’Sullivan, Cherie M., Deo, Ravinesh C. and Ghahramani, Afshin. 2023. "Author Correction: Explainable AI approach with original vegetation data classifies spatio‑temporal nitrogen in flows from ungauged catchments to the Great Barrier Reef." Scientific Reports. 13 (1). https://doi.org/10.1038/s41598-023-48938-0Channel-Agnostic Training of Transmitter and Receiver for Wireless Communications
Davey, Christopher P., Shakeel, Ismail, Deo, Ravinesh C. and Salcedo-sanz, Sancho. 2023. "Channel-Agnostic Training of Transmitter and Receiver for Wireless Communications ." Sensors. 23 (24). https://doi.org/10.3390/s23249848Deep Image Analysis for Microalgae Identification
Soar, Jeffrey, Lih, Oh Shu, Wen, Loh Hui, Ward, Aleth, Sharma, Ekta, Deo, Ravinesh C., Barua, Prabal Datta, Tan, Ru-San, Rinen, Eliezer and Acharya, Rajendra. 2023. "Deep Image Analysis for Microalgae Identification." Lecture notes in computer science. Switzerland . Springer. pp. 280-292Development of an enhanced bidirectional recurrent neural network combined with time-varying filter-based empirical mode decomposition to forecast weekly reference evapotranspiration
Karbasi, Masoud, Jamei, Mehdi, Ali, Mumtaz, Malik, Anurag, Chu, Xuefeng, Farooque, Aitazaz Ahsan and Yaseen, Zaher Mundher. 2023. "Development of an enhanced bidirectional recurrent neural network combined with time-varying filter-based empirical mode decomposition to forecast weekly reference evapotranspiration." Agricultural Water Management. 290. https://doi.org/10.1016/j.agwat.2023.108604Climate change and human health in Vietnam: a systematic review and additional analyses on current impacts, future risk, and adaptation
Tran, Nu Quy Linh, Le, Huynh Thi Cam Hong, Pham, Cong Tuan, Nguyen, Xuan Huong, Tran, Ngoc Dang, Tran, Tuyet-Hanh Thi, Nghiem, Son, Luong, Thi Mai Ly, Bui, Vinh, Nguyen-Huy, Thong, Doan, Van Quang, Dang, Kim Anh, Do, Thi Hoai Thuong, Ngo, Hieu Kim Thi, Nguyen, Truong Vien, Nguyen, Ngoc Huy, Do, Manh Cuong, Ton, Tuan Nghia, Dang, Thi Anh Thu, ..., Phung, Dung. 2023. "Climate change and human health in Vietnam: a systematic review and additional analyses on current impacts, future risk, and adaptation." The Lancet Regional Health - Western Pacific. 40. https://doi.org/10.1016/j.lanwpc.2023.100943Enhancing climate resilience by combining practice and insurance strategies: A case study for cotton crop
Nguyen-Huy, Thong and Battersby, Kerry. 2023. "Enhancing climate resilience by combining practice and insurance strategies: A case study for cotton crop." Queensland Disaster Management Research Forum 2023. Brisbane, Australia 07 - 07 Nov 2023An ensemble machine learning-based intelligent system for human activity recognition using sensory data
Abdulla, Shahab, Diykh, Mohammed, Siuly, Siuly and Ali, Mumtaz. 2023. "An ensemble machine learning-based intelligent system for human activity recognition using sensory data." Sinha, G.R., Subudhi, Bidyadhar, Fan, Chih-Peng and Nisar, Humaira (ed.) Cognitive Sensing Technologies and Applications. Institution of Engineering and Technology (IET). pp. 119-130Statistical and spatial analysis for soil heavy metals over the Murray-Darling river basin in Australia
Tao, Hai, Al-Hilali, Aqeel Ali, Ahmed, Ali M., Mussa, Zainab Haider, Falah, Mayadah W., Abed, Salwan Ali, Deo, Ravinesh, Jawad, Ali H., Maulud, Khairul Nizam Abdul, Latif, Mohd Talib and Yaseen, Zaher Mundher. 2023. "Statistical and spatial analysis for soil heavy metals over the Murray-Darling river basin in Australia." Chemosphere. 317. https://doi.org/10.1016/j.chemosphere.2023.137914Enhanced joint hybrid deep neural network explainable artificial intelligence model for 1-hr ahead solar ultraviolet index prediction
Prasad, Salvin S., Deo, Ravinesh C., Salcedo-sanz, Sancho, Downs, Nathan J., Casillas-Perez, David and Parisi, Alfio V.. 2023. "Enhanced joint hybrid deep neural network explainable artificial intelligence model for 1-hr ahead solar ultraviolet index prediction." Computer Methods and Programs in Biomedicine. 241. https://doi.org/10.1016/j.cmpb.2023.107737Ampelomyces mycoparasites of powdery mildews–a review
Prahl, Rosa E., Khan, Shahjahan and Deo, Ravinesh C.. 2023. "Ampelomyces mycoparasites of powdery mildews–a review." Canadian Journal of Plant Pathology. 45 (4), pp. 391-404. https://doi.org/10.1080/07060661.2023.2206378A fuzzy-based cascade ensemble model for improving extreme wind speeds prediction
Pelaez-Rodriguez, C., Perez-Aracil, J., Prieto-Godino, L., Ghimire, S., Deo, R. and Salcedo-sanz, S.. 2023. "A fuzzy-based cascade ensemble model for improving extreme wind speeds prediction." Journal of Wind Engineering and Industrial Aerodynamics. 240. https://doi.org/10.1016/j.jweia.2023.105507Explainable AI approach with original vegetation data classifies spatio-temporal nitrogen in flows from ungauged catchments to the Great Barrier Reef
O’Sullivan, Cherie M., Deo, Ravinesh C. and Ghahramani, Afshin. 2023. "Explainable AI approach with original vegetation data classifies spatio-temporal nitrogen in flows from ungauged catchments to the Great Barrier Reef." Scientific Reports. 13 (1). https://doi.org/10.1038/s41598-023-45259-0A Novel Attention-Based Model for Semantic Segmentation of Prostate Glands Using Histopathological Images
Inamdar, Mahesh Anil, Raghavendra, U., Gudigar, Anjan, Bhandary, Sarvesh, Salvi, Massimo, Deo, Ravinesh C., Barua, Prabal Datta, Ciaccio, Edward J., Molinari, Filippo and Acharya, RU. Rajendra. 2023. "A Novel Attention-Based Model for Semantic Segmentation of Prostate Glands Using Histopathological Images." IEEE Access. 11, pp. 108982-108994. https://doi.org/10.1109/ACCESS.2023.3321273Efficient daily electricity demand prediction with hybrid deep-learning multi-algorithm approach
Ghimire, Sujan, Deo, Ravinesh C., Casillas-Perez, David and Salcedo-sanz, Sancho. 2023. "Efficient daily electricity demand prediction with hybrid deep-learning multi-algorithm approach." Energy Conversion and Management. 297. https://doi.org/10.1016/j.enconman.2023.117707The development of dissolved oxygen forecast model using hybrid machine learning algorithm with hydro-meteorological variables
Ahmed, Abul Abrar Masrur, Jui, S. Janifer Jabin, Chowdhury, Mohammad Aktarul Islam, Ahmed, Oli and Sutradhar, Ambica. 2023. "The development of dissolved oxygen forecast model using hybrid machine learning algorithm with hydro-meteorological variables." Environmental Science and Pollution Research. 30 (3), pp. 7851-7873. https://doi.org/10.1007/s11356-022-22601-zA drought monitor for Australia
Guillory, Laura, Pudmenzky, Christa, Nguyen-Huy, Thong, Cobon, David and Stone, Roger. 2023. "A drought monitor for Australia." Environmental Modelling and Software. 170. https://doi.org/10.1016/j.envsoft.2023.105852New achievements on daily reference evapotranspiration forecasting: Potential assessment of multivariate signal decomposition schemes
Ali, Mumtaz, Jamei, Mehdi, Prasad, Ramendra, Karbasi, Masoud, Xiang, Yong, Cai, Borui, Abdulla, Shahab, Farooque, Aitazaz Ahsan and Labban, Abdulhaleem H.. 2023. "New achievements on daily reference evapotranspiration forecasting: Potential assessment of multivariate signal decomposition schemes." Ecological Indicators. 155. https://doi.org/10.1016/j.ecolind.2023.111030Automated detection of airflow obstructive diseases: A systematic review of the last decade (2013-2022)
Xu, Shuting, Deo, Ravinesh C, Soar, Jeffrey, Barua, Prabal Datta, Faust, Oliver, Homaira, Nusrat, Jaffe, Adam, Kabir, Arm Luthful and Acharya, U. Rajendra. 2023. "Automated detection of airflow obstructive diseases: A systematic review of the last decade (2013-2022)." Computer Methods and Programs in Biomedicine. 241. https://doi.org/10.1016/j.cmpb.2023.107746Brain tumor detection and screening using artificial intelligence techniques: Current trends and future perspectives
Raghavendra, U., Gudigar, Anjan, Paul, Aritra, Goutham, T.S., Inamdar, Mahesh Anil, Hegde, Ajay, Dev, Aruna, Ooi, Chui Ping, Deo, Ravinesh C., Barua, Prabal Datta, Molinari, Filippo, Ciaccio, Edward J. and Acharya, U. Rajendra. 2023. "Brain tumor detection and screening using artificial intelligence techniques: Current trends and future perspectives." Computers in Biology and Medicine. 163. https://doi.org/10.1016/j.compbiomed.2023.107063Application of Entropy for Automated Detection of Neurological Disorders With Electroencephalogram Signals: A Review of the Last Decade (2012-2022)
Jui, S. Janifer Jabin, Deo, Ravinesh C. Deo, Barua, Prabal Datta, Devi, Aruna, Soar, Jeffrey and Acharya, U. Rajendra. 2023. "Application of Entropy for Automated Detection of Neurological Disorders With Electroencephalogram Signals: A Review of the Last Decade (2012-2022)." IEEE Access. 11, pp. 71905-71924. https://doi.org/10.1109/ACCESS.2023.3294473Integrated Multi-Head Self-Attention Transformer model for electricity demand prediction incorporating local climate variables
Ghimire, Sujan, Nguyen-Huy, Thong, AL-Musaylh, Mohanad S., Deo, Ravinesh C., Casillas-Perez, David and Salcedo-sanz, Sancho. 2023. "Integrated Multi-Head Self-Attention Transformer model for electricity demand prediction incorporating local climate variables." Energy and AI. 14. https://doi.org/10.1016/j.egyai.2023.100302A systematic review of emerging environmental markets: Potential pathways to creating shared value for communities
Mittahalli Byrareddy, Vivekananda M, Islam, Md Aminul, Nguyen-Huy, Thong and Slaughter, Geoff. 2023. "A systematic review of emerging environmental markets: Potential pathways to creating shared value for communities ." Heliyon. 9 (9). https://doi.org/10.1016/j.heliyon.2023.e19754Integrating management decisions and insurance to drive climate adaptation in the agricultural industry
Nguyen-Huy, Thong. 2023. "Integrating management decisions and insurance to drive climate adaptation in the agricultural industry ." Climate Adaptation 2023. Adelaide, Australia 25 - 27 Jul 2023 Australia.Multivariate data decomposition based deep learning approach to forecast one-day ahead significant wave height for ocean energy generation
Zheng, Zihao, Ali, Mumtaz, Jamei, Mehdi, Xiang, Yong, Abdulla, Shahab, Yaseen, Zaher Mundher and Farooque, Aitazaz A.. 2023. "Multivariate data decomposition based deep learning approach to forecast one-day ahead significant wave height for ocean energy generation." Renewable and Sustainable Energy Reviews. 185. https://doi.org/10.1016/j.rser.2023.113645Flash flood-risk areas zoning using integration of decision-making trial and evaluation laboratory, GIS-based analytic network process and satellite-derived information
Taherizadeh, Mehrnoosh, Niknam, Arman, Nguyen-Huy, Thong, Mezősi, Gábor and Sarli, Reza. 2023. "Flash flood-risk areas zoning using integration of decision-making trial and evaluation laboratory, GIS-based analytic network process and satellite-derived information." Natural Hazards. 118 (3), p. 2309–2335. https://doi.org/10.1007/s11069-023-06089-5Revealing the effect of an industrial flash flood on vegetation area: a case study of Khusheh Mehr in Maragheh-Bonab Plain, Iran
Taherizadeh, Mehrnoosh, Khushemehr, Javid Hojabri, Niknam, Arman, Nguyen-Huy, Thong and Mezősi, Gábor. 2023. "Revealing the effect of an industrial flash flood on vegetation area: a case study of Khusheh Mehr in Maragheh-Bonab Plain, Iran." Remote Sensing Applications: Society and Environment. 32. https://doi.org/10.1016/j.rsase.2023.101016Designing a decomposition-based multi-phase pre-processing strategy coupled with EDBi-LSTM deep learning approach for sediment load forecasting
Jamei, Mehdi, Ali, Mumtaz, Malik, Anurag, Rai, Priya, Karbasi, Masoud, Farooque, Aitazaz A. and Yaseen, Zaher Mundher. 2023. "Designing a decomposition-based multi-phase pre-processing strategy coupled with EDBi-LSTM deep learning approach for sediment load forecasting." Ecological Indicators. 153. https://doi.org/10.1016/j.ecolind.2023.110478Development of high-resolution gridded data for water availability identification through GRACE data downscaling: Development of machine learning models
Tao, Hai, Al-Sulttani, Ahmed H., Salih, Sinan Q., Mohammed, Mustafa K.A., Khan, Mohammad Amir, Beyaztas, Beste Hamiye, Ali, Mumtaz, Elsayed, Salah, Shahid, Shamsuddin and Yaseen, Zaher Mundher. 2023. "Development of high-resolution gridded data for water availability identification through GRACE data downscaling: Development of machine learning models." Atmospheric Research. 291. https://doi.org/10.1016/j.atmosres.2023.106815The Great 2011 Thailand flood disaster revisited: Could it have been mitigated by different dam operations based on better weather forecasts?
Loc, Ho Huu, Emadzadeh, Adel, Park, Edward, Nontikansak, Piyanuch and Deo, Ravinesh C.. 2023. "The Great 2011 Thailand flood disaster revisited: Could it have been mitigated by different dam operations based on better weather forecasts? " Environmental Research. 216 (Part 2). https://doi.org/10.1016/j.envres.2022.114493Comparison of machine learning methods emulating process driven crop models
Johnston, David B., Pembleton, Keith G., Huth, Neil I. and Deo, Ravinesh C.. 2023. "Comparison of machine learning methods emulating process driven crop models ." Environmental Modelling and Software. 162. https://doi.org/10.1016/j.envsoft.2023.105634Multi-step ahead hourly forecasting of air quality indices in Australia: Application of an optimal time-varying decomposition-based ensemble deep learning algorithm
Jamei, Mehdi, Ali, Mumtaz, Jun, Changhyun, Bateni, Sayed M., Karbasi, Masoud, Farooque, Aitazaz A. and Yaseen, Zaher Mundher. 2023. "Multi-step ahead hourly forecasting of air quality indices in Australia: Application of an optimal time-varying decomposition-based ensemble deep learning algorithm." Atmospheric Pollution Research. 14 (6). https://doi.org/10.1016/j.apr.2023.101752Machine Learning Algorithms for High-Resolution Prediction of Spatiotemporal Distribution of Air Pollution from Meteorological and Soil Parameters
Tao, Hai, Jawad, Ali H., Shather, A.H., Al-Khafaji, Zainab Al, Rashid, Tarik A., Ali, Mumtaz, Al-Ansari, Nadhir Al, Marhoon, Haydar Abdulameer, Shahid, Shamsuddin and Yaseen, Zaher Mundher. 2023. "Machine Learning Algorithms for High-Resolution Prediction of Spatiotemporal Distribution of Air Pollution from Meteorological and Soil Parameters." Environment International. 175. https://doi.org/10.1016/j.envint.2023.107931A novel approach based on integration of convolutional neural networks and echo state network for daily electricity demand prediction
Ghimire, Sujan, Nguyen-Huy, Thong, AL-Musaylh, Mohanad S., Deo, Ravinesh, Casillas-Perez, David and Salcedo-sanz, Sancho. 2023. "A novel approach based on integration of convolutional neural networks and echo state network for daily electricity demand prediction." Energy. 275, p. 127430. https://doi.org/10.1016/j.energy.2023.127430Climatology and composite evolution of flash drought over Australia and its vegetation impacts
Nguyen, Hanh, Wheeler, Mattew C., Otkin, Jason A., Nguyen-Huy, Thong and Cowan, Timothy. 2023. "Climatology and composite evolution of flash drought over Australia and its vegetation impacts." Journal of Hydrometeorology. 24 (6), p. 1087–1101. https://doi.org/10.1175/JHM-D-22-0033.1Surface water electrical conductivity and bicarbonate ion determination using a smart hybridization of optimal Boruta package with Elman recurrent neural network
Jamei, Mehdi, Ali, Mumtaz, Karimi, Bakhtiar, Karbasi, Masoud, Farooque, Aitataz Ahsan and Yaseen, Zaher Mundher. 2023. "Surface water electrical conductivity and bicarbonate ion determination using a smart hybridization of optimal Boruta package with Elman recurrent neural network." Process Safety and Environmental Protection. 174, pp. 115-134. https://doi.org/10.1016/j.psep.2023.03.062Development of neutrosophic cubic hesitant fuzzy exponential aggregation operators with application in environmental protection problems
Rehman, Ateeq Ur, Gulistan, Muhammad, Ali, Mumtaz, M.Al‑Shamiri, Mohammed M. and Abdulla, Shahab. 2023. "Development of neutrosophic cubic hesitant fuzzy exponential aggregation operators with application in environmental protection problems." Scientific Reports. 13, pp. 1-18. https://doi.org/10.1038/s41598-022-22399-3Digital Farming: An Overview of Its Fundamental Components and Applications
Nguyen-Huy, Thong. 2023. "Digital Farming: An Overview of Its Fundamental Components and Applications." The 4th International Conference of Food Security and Sustainable Agriculture in the Tropics. South Sulawesi, Indonesia 15 - 16 Feb 2023 Indonesia.A comprehensive investigation of wetting distribution pattern on sloping lands under drip irrigation: A new gradient boosting multi-filtering-based deep learning approach
Jamei, Mehdi, Karimi, Bakhtiar, Ali, Mumtaz, Alinazari, Fariba, Karbasi, Masoud, Maroufpoor, Eisa and Chu, Xuefeng. 2023. "A comprehensive investigation of wetting distribution pattern on sloping lands under drip irrigation: A new gradient boosting multi-filtering-based deep learning approach." Journal of Hydrology. 620 (Part A). https://doi.org/10.1016/j.jhydrol.2023.129402Accurate Image Multi-Class Classification Neural Network Model with Quantum Entanglement Approach
Riaz, Farina, Abdulla, Shahab, Suzuki, Hajime, Ganguly, Srinjoy, Deo, Ravinesh C. and Hopkins, Susan. 2023. "Accurate Image Multi-Class Classification Neural Network Model with Quantum Entanglement Approach." Sensors. 23 (5), pp. 1-11. https://doi.org/10.3390/s23052753Near real-time wind speed forecast model with bidirectional LSTM networks
Joseph, Lionel P., Deo, Ravinesh C., Prasad, Ramendra, Salcedo-Sanz, Sancho, Raj, Nawin and Soar, Jeffrey. 2023. "Near real-time wind speed forecast model with bidirectional LSTM networks." Renewable Energy. 204, pp. 39-58. https://doi.org/10.1016/j.renene.2022.12.123A novel global solar exposure forecasting model based on air temperature: Designing a new multi-processing ensemble deep learning paradigm
Jamei, Mehdi, Karbasi, Masoud, Ali, Mumtaz, Malik, Anurag, Chu, Xuefeng and Yaseen, Zaher Mundher. 2023. "A novel global solar exposure forecasting model based on air temperature: Designing a new multi-processing ensemble deep learning paradigm." Expert Systems with Applications. 222. https://doi.org/10.1016/j.eswa.2023.119811Downscaling Surface Albedo to Higher Spatial Resolutions With an Image Super-Resolution Approach and PROBA-V Satellite Images
Deo, Ravinesh C., Karalasingham, Sagthitharan, Casillas-Perez, David, Raj, Narwin and Salcedo-sanz, Sancho. 2023. "Downscaling Surface Albedo to Higher Spatial Resolutions With an Image Super-Resolution Approach and PROBA-V Satellite Images." IEEE Access. 11, pp. 5558-5577. https://doi.org/10.1109/ACCESS.2023.3236253Design data decomposition-based reference evapotranspiration forecasting model: A soft feature filter based deep learning driven approach
Zheng, Zihao, Ali, Mumtaz, Jamei, Mehdi, Xiang, Yong, Karbasi, Masoud, Yaseen, Zaher Mundher and Farooque, Aitazaz Ahsan. 2023. "Design data decomposition-based reference evapotranspiration forecasting model: A soft feature filter based deep learning driven approach." Engineering Applications of Artificial Intelligence. 121. https://doi.org/10.1016/j.engappai.2023.105984Designing pentapartitioned neutrosophic cubic set aggregation operator-based air pollution decision-making model
Li, Yi-ming, Khan, Majid, Khurshid, Adnan, Gulistan, Muhammad, Rehman, Ateeq Ur, Ali, Mumtaz, Abdulla, Shahab and Farooque, Aitazaz A.. 2023. "Designing pentapartitioned neutrosophic cubic set aggregation operator-based air pollution decision-making model ." Complex and Intelligent Systems. https://doi.org/10.1007/s40747-023-00971-2Ensemble robust local mean decomposition integrated with random forest for short-term significant wave height forecasting
Ali, Mumtaz, Prasad, Ramendra, Xiang, Yong, Jamei, Mehdi and Yaseen, Zaher Mundher. 2023. "Ensemble robust local mean decomposition integrated with random forest for short-term significant wave height forecasting." Renewable Energy. 205, pp. 731-746. https://doi.org/10.1016/j.renene.2023.01.108An intelligent model involving multi-channels spectrum patterns based features for automatic sleep stage classification
Abdulla, Shahab, Diykh, Mohammed, Siuly, Siuly and Ali, Mumtaz. 2023. "An intelligent model involving multi-channels spectrum patterns based features for automatic sleep stage classification." International Journal of Medical Informatics. 171. https://doi.org/10.1016/j.ijmedinf.2023.105001Developing a novel hybrid method based on dispersion entropy and adaptive boosting algorithm for human activity recognition
Diykh, Mohammed, Abdulla, Shahab, Deo, Ravinesh C, Siuly, Siuly and Ali, Mumtaz. 2023. "Developing a novel hybrid method based on dispersion entropy and adaptive boosting algorithm for human activity recognition." Computer Methods and Programs in Biomedicine. 229. https://doi.org/10.1016/j.cmpb.2022.107305A study of plithogenic graphs: applications in spreading coronavirus disease (COVID-19) globally
Sultana, Fazeelat, Gulistan, Muhammad, Ali, Mumtaz, Yaqoob, Naveed, Khan, Muhammad, Rashid, Tabasam and Ahmed, Tauseef. 2023. "A study of plithogenic graphs: applications in spreading coronavirus disease (COVID-19) globally ." Journal of Ambient Intelligence and Humanized Computing. 14 (10), p. 13139–13159. https://doi.org/10.1007/s12652-022-03772-6Cloud cover bias correction in numerical weather models for solar energy monitoring and forecasting systems with kernel ridge regression
Deo, Ravinesh C., Ahmed, A.A. Masrur, Casillas-Perez, David, Pourmousavi, S. Ali, Segal, Gary, Yu, Yanshan and Salcedo-sanz, Sancho. 2023. "Cloud cover bias correction in numerical weather models for solar energy monitoring and forecasting systems with kernel ridge regression." Renewable Energy. 203, pp. 113-130. https://doi.org/10.1016/j.renene.2022.12.048Development of a TVF-EMD-based multi-decomposition technique integrated with Encoder-Decoder-Bidirectional-LSTM for monthly rainfall forecasting
Jamei, Mehdi, Ali, Mumtaz, Malik, Anurag, Karbasi, Masoud, Rai, Priya and Yaseen, Zaher Mundher. 2023. "Development of a TVF-EMD-based multi-decomposition technique integrated with Encoder-Decoder-Bidirectional-LSTM for monthly rainfall forecasting." Journal of Hydrology. 617 (Part C). https://doi.org/10.1016/j.jhydrol.2023.129105Deep Multi-Stage Reference Evapotranspiration Forecasting Model: Multivariate Empirical Mode Decomposition Integrated With the Boruta-Random Forest Algorithm
Jayasinghe, W. J. M. Lakmini Prarthana, Deo, Ravinesh C., Ghahramani, Afshin, Ghimire, Sujan and Raj, Nawin. 2021. "Deep Multi-Stage Reference Evapotranspiration Forecasting Model: Multivariate Empirical Mode Decomposition Integrated With the Boruta-Random Forest Algorithm." IEEE Access. 9, pp. 166695-166708. https://doi.org/10.1109/ACCESS.2021.3135362Designing Deep-based Learning Flood Forecast Model with ConvLSTM Hybrid Algorithm
Moishin, Mohammed, Deo, Ravinesh C., Prasad, Ramendra, Raj, Nawin and Abdulla, Shahab. 2021. "Designing Deep-based Learning Flood Forecast Model with ConvLSTM Hybrid Algorithm." IEEE Access. 9, pp. 50982-50993. https://doi.org/10.1109/ACCESS.2021.3065939Pattern recognition describing spatio-temporal drivers of catchment classification for water quality
O'Sullivan, Cherie M., Ghahramani, Afshin, Deo, Ravinesh C. and Pembleton, Keith G.. 2023. "Pattern recognition describing spatio-temporal drivers of catchment classification for water quality." Science of the Total Environment. 861, pp. 1-42. https://doi.org/10.1016/j.scitotenv.2022.160240The Playground Shade Index: A New Design Metric for Measuring Shade and Seasonal Ultraviolet Protection Characteristics of Parks and Playgrounds
Downs, Nathan, Raj, Nawin, Vanos, Jennifer, Parisi, Alfio, Butler, Harry, Deo, Ravinesh, Igoe, Damien, Dexter, Benjamin, Beckman-Downs, Melanie, Turner, Joanna and Dekeyser, Stijn. 2023. "The Playground Shade Index: A New Design Metric for Measuring Shade and Seasonal Ultraviolet Protection Characteristics of Parks and Playgrounds." Photochemistry and Photobiology. 99 (4), pp. 1193-1207. https://doi.org/10.1111/php.13745Using Sequence-to-Sequence Models for Carrier Frequency Offset Estimation of Short Messages and Chaotic Maps
Davey, Christopher P., Shakeel, Ismail, Deo, Ravinesh C., Salcedo-sanz, Sancho and Soar, Jeffrey. 2022. "Using Sequence-to-Sequence Models for Carrier Frequency Offset Estimation of Short Messages and Chaotic Maps." IEEE Access. 10, pp. 119814 - 119825. https://doi.org/10.1109/ACCESS.2022.3221762Developing a novel hybrid Auto Encoder Decoder Bidirectional Gated Recurrent Unit model enhanced with empirical wavelet transform and Boruta-Catboost to forecast significant wave height
Karbasi, Masoud, Jamei, Mehdi, Ali, Mumtaz, Abdulla, Shahab, Chu, Xuefeng and Yaseen, Zaher Mundher. 2022. "Developing a novel hybrid Auto Encoder Decoder Bidirectional Gated Recurrent Unit model enhanced with empirical wavelet transform and Boruta-Catboost to forecast significant wave height." Journal of Cleaner Production. 379 (Part 2). https://doi.org/10.1016/j.jclepro.2022.134820Modelling and Real-time Optimisation of Air Quality Predictions for Australia through Artificial Intelligence Algorithm
Sharma, Ekta, Deo, Ravinesh C., Prasad, Ramendra and Parisi, Alfio V.. 2019. "Modelling and Real-time Optimisation of Air Quality Predictions for Australia through Artificial Intelligence Algorithm." AMSI Optimise 2019. Perth, Australia 17 - 21 Jun 2019 Perth, Australia.Connecting community online and through partnership: A reflective piece
Pickstone, Leigh, Sharma, Ekta, King, Rachel, Galligan, Linda and Langlands, Trevor. 2022. "Connecting community online and through partnership: A reflective piece." International Journal for Students as Partners. 6 (2), pp. 114-120. https://doi.org/10.15173/ijsap.v6i2.4825Experimental and computational assessment of wetting pattern for two-layered soil profiles in pulse drip irrigation: Designing a novel optimized bidirectional deep learning paradigm
Jamei, Mehdi, Karimi, Farahnaz, Ali, Mumtaz, Karimi, Bakhtiar, Karbasi, Masoud and Aminpour, Younes. 2022. "Experimental and computational assessment of wetting pattern for two-layered soil profiles in pulse drip irrigation: Designing a novel optimized bidirectional deep learning paradigm." Journal of Hydrology. 614 (Part A), pp. 1-15. https://doi.org/10.1016/j.jhydrol.2022.128496Hybrid Convolutional Neural Network-Multilayer Perceptron Model for Solar Radiation Prediction
Ghimire, Sujan, Nguyen-Huy, Thong, Prasad, Ramendra, Deo, Ravinesh C., Casillas-Perez, David, Salcedo-sanz, Sancho and Bhandari, Binayak. 2023. "Hybrid Convolutional Neural Network-Multilayer Perceptron Model for Solar Radiation Prediction." Cognitive Computation. 15 (2), pp. 645-671. https://doi.org/10.1007/s12559-022-10070-yVapour pressure deficit determines critical thresholds for global coffee production under climate change
Kath, Jarrod, Craparo, Alessandro, Fong, Youyi, Byrareddy, Vivekananda, Davis, Aaron P., King, Rachel, Nguyen-Huy, Thong, van Asten, Piet J. A., Marcussen, Torben, Mushtaq, Shahbaz, Stone, Roger and Power, Scott. 2022. "Vapour pressure deficit determines critical thresholds for global coffee production under climate change." Nature Food. 3, pp. 871-880. https://doi.org/10.1038/s43016-022-00614-8Student Performance Predictions for Advanced Engineering Mathematics Course With New Multivariate Copula Models
Nguyen-Huy, Thong, Deo, Ravinesh C., Khan, Shahjahan, Devi, Aruna, Adeyinka, Adewuyi Ayodele, Apan, Armando A. and Yaseen, Zaher Mundher. 2022. "Student Performance Predictions for Advanced Engineering Mathematics Course With New Multivariate Copula Models." IEEE Access. 10, pp. 45112 -45136. https://doi.org/10.1109/ACCESS.2022.3168322Rapid assessment of mine rehabilitation areas with airborne LiDAR and deep learning: bauxite strip mining in Queensland, Australia
Murray, Xavier, Apan, Armando, Deo, Ravinesh and Maraseni, Tek. 2022. "Rapid assessment of mine rehabilitation areas with airborne LiDAR and deep learning: bauxite strip mining in Queensland, Australia." Geocarto International. 37 (26), pp. 11223-11252. https://doi.org/10.1080/10106049.2022.2048902Multi-strategy Slime Mould Algorithm for hydropower multi-reservoir systems optimization
Ahmadianfar, Iman, Noori, Ramzia Majeed, Togun, Hussein, Falah, Mayadah W., Homod, Raad Z., Fu, Minglei, Halder, Bijay, Deo, Ravinesh and Yaseen, Zaher Mundher. 2022. "Multi-strategy Slime Mould Algorithm for hydropower multi-reservoir systems optimization." Knowledge-Based Systems. 250, pp. 1-18. https://doi.org/10.1016/j.knosys.2022.109048Suspended sediment load modeling using advanced hybrid rotation forest based elastic network approach
Khosravi, Khabat, Golkarian, Ali, Melesse, Assefa M. and Deo, Ravinesh C.. 2022. "Suspended sediment load modeling using advanced hybrid rotation forest based elastic network approach." Journal of Hydrology. 610, pp. 1-14. https://doi.org/10.1016/j.jhydrol.2022.127963Delineating the Crop-Land Dynamic due to Extreme Environment Using Landsat Datasets: A Case Study
Halder, Bijay, Bandyopadhyay, Jatisankar, Afan, Haitham Abdulmohsin, Naser, Maryam H., Abed, Salwan Ali, Khedher, Khaled Mohamed, Falih, Khaldoon T., Deo, Ravinesh, Scholz, Miklas and Yaseen, Zaher Mundher. 2022. "Delineating the Crop-Land Dynamic due to Extreme Environment Using Landsat Datasets: A Case Study." Agronomy. 12 (6), pp. 1-23. https://doi.org/10.3390/agronomy12061268Coupled online sequential extreme learning machine model with ant colony optimization algorithm for wheat yield prediction
Ali, Mumtaz, Deo, Ravinesh C., Xiang, Yong, Prasad, Ramendra, Li, Jianxin, Farooque, Aitazaz and Yaseen, Zaher Mundher. 2022. "Coupled online sequential extreme learning machine model with ant colony optimization algorithm for wheat yield prediction." Scientific Reports. 12 (1), pp. 1-23. https://doi.org/10.1038/s41598-022-09482-5Forecasting solar photosynthetic photon flux density under cloud cover effects: novel predictive model using convolutional neural network integrated with long short-term memory network
Deo, Ravinesh C., Grant, Richard H., Webb, Ann, Ghimire, Sujan, Igoe, Damien P., Downs, Nathan J., Al-Musaylh, Mohanad S., Parisi, Alfio V. and Soar, Jeffrey. 2022. "Forecasting solar photosynthetic photon flux density under cloud cover effects: novel predictive model using convolutional neural network integrated with long short-term memory network." Stochastic Environmental Research and Risk Assessment. 36, p. 3183–3220. https://doi.org/10.1007/s00477-022-02188-0Improved Complete Ensemble Empirical Mode Decomposition with Adaptive Noise Deep Residual model for short-term multi-step solar radiation prediction
Ghimire, Sujan, Deo, Ravinesh C, Casillas-Perez, David and Salcedo-sanz, Sancho. 2022. "Improved Complete Ensemble Empirical Mode Decomposition with Adaptive Noise Deep Residual model for short-term multi-step solar radiation prediction." Renewable Energy. 190, pp. 408-424. https://doi.org/10.1016/j.renene.2022.03.120Efficient daily solar radiation prediction with deep learning 4-phase convolutional neural network, dual stage stacked regression and support vector machine CNN-REGST hybrid model
Ghimire, Sujan, Nguyen-Huy, Thong, Deo, Ravinesh C., Casillas-Perez, David and Salcedo-sanz, Sancho. 2022. "Efficient daily solar radiation prediction with deep learning 4-phase convolutional neural network, dual stage stacked regression and support vector machine CNN-REGST hybrid model." Sustainable Materials and Technologies. 32, pp. 1-24. https://doi.org/10.1016/j.susmat.2022.e00429Hybrid deep CNN-SVR algorithm for solar radiation prediction problems in Queensland, Australia
Ghimire, Sujan, Bhandari, Binayak, Casillas-Perez, David, Deo, Ravinesh C. and Salcedo-sanz, Sancho. 2022. "Hybrid deep CNN-SVR algorithm for solar radiation prediction problems in Queensland, Australia." Engineering Applications of Artificial Intelligence. 112, pp. 1-26. https://doi.org/10.1016/j.engappai.2022.104860Boosting solar radiation predictions with global climate models, observational predictors and hybrid deep-machine learning algorithms
Ghimire, Sujan, Deo, Ravinesh C., Casillas-Perez, David and Salcedo-sanz, Sancho. 2022. "Boosting solar radiation predictions with global climate models, observational predictors and hybrid deep-machine learning algorithms." Applied Energy. 316, pp. 1-25. https://doi.org/10.1016/j.apenergy.2022.119063Machine learning regression and classification methods for fog events prediction
Castillo-Boton, C., Casillas-Perez, D., Casanova-Mateo, C., Ghimire, S., Cerro-Prada, E., Gutierrez, P. A., Deo, R. C. and Salcedo-sanz, S.. 2022. "Machine learning regression and classification methods for fog events prediction." Atmospheric Research. 272, pp. 1-23. https://doi.org/10.1016/j.atmosres.2022.106157Quantum Artificial Intelligence Predictions Enhancement by Improving Signal Processing
Riaz, Farina, Abdulla, Shahab, Ni, Wei, Radfar, Mohsen, Deo, Ravinesh and Hopkins, Susan. 2022. "Quantum Artificial Intelligence Predictions Enhancement by Improving Signal Processing." Quantum Australia Conference 2022. Online 23 - 25 Feb 2022 Toowoomba, Australia. https://doi.org/10.13140/RG.2.2.34754.66245Forecasting Daily Flood Water Level Using Hybrid Advanced Machine Learning Based Time‑Varying Filtered Empirical Mode Decomposition Approach
Jamei, Mehdi, Ali, Mumtaz, Malik, Anurag, Prasad, Ramendra, Abdulla, Shahab and Yaseen, Zaher Mundher. 2022. "Forecasting Daily Flood Water Level Using Hybrid Advanced Machine Learning Based Time‑Varying Filtered Empirical Mode Decomposition Approach." Water Resources Management. 36 (12), p. 4637–4676. https://doi.org/10.1007/s11269-022-03270-6A satellite-based Standardized Antecedent Precipitation Index (SAPI) for mapping extreme rainfall risk in Myanmar
Nguyen-Huy, Thong, Kath, Jarrod, Nagler, Thomas, Khaung, Ye, Aung, Thee Su Su, Mushtaq, Shahbaz, Marcussen, Torben and Stone, Roger. 2022. "A satellite-based Standardized Antecedent Precipitation Index (SAPI) for mapping extreme rainfall risk in Myanmar." Remote Sensing Applications: Society and Environment. 26, pp. 1-19. https://doi.org/10.1016/j.rsase.2022.100733Deep learning CNN-LSTM-MLP hybrid fusion model for feature optimizations and daily solar radiation prediction
Ghimire, Sujan, Deo, Ravinesh C., Casillas-Perez, David, Salcedo-sanz, Sancho, Sharma, Ekta and Ali, Mumtaz. 2022. "Deep learning CNN-LSTM-MLP hybrid fusion model for feature optimizations and daily solar radiation prediction." Measurement. 202, pp. 1-22. https://doi.org/10.1016/j.measurement.2022.111759Basin management inspiration from impacts of alternating dry and wet conditions on water production and carbon uptake in Murray-Darling Basin
Lu, Zhixiang, Feng, Qi, Wei, Yongping, Zhao, Yan, Deo, Ravinesh C., Xie, Jiali, Zhou, Sha, Zhu, Meng and Xu, Min. 2022. "Basin management inspiration from impacts of alternating dry and wet conditions on water production and carbon uptake in Murray-Darling Basin." Science of the Total Environment. 851 (Part 2), pp. 1-8. https://doi.org/10.1016/j.scitotenv.2022.158359Introductory Engineering Mathematics Students’ Weighted Score Predictions Utilising a Novel Multivariate Adaptive Regression Spline Model
Ahmed, Abul Abrar Masrur, Deo, Ravinesh C., Ghimire, Sujan, Downs, Nathan J., Devi, Aruna, Barua, Prabal D. and Yaseen, Zaher M.. 2022. "Introductory Engineering Mathematics Students’ Weighted Score Predictions Utilising a Novel Multivariate Adaptive Regression Spline Model." Sustainability. 14 (17), pp. 1-27. https://doi.org/10.3390/su141711070Cloud Affected Solar UV Predictions with Three-Phase Wavelet Hybrid Convolutional Long Short-Term Memory Network Multi-Step Forecast System
Prasad, Salvin S., Deo, Ravinesh C., Downs, Nathan, Igoe, Damien, Parisi, Alfio V. and Soar, Jeffrey. 2022. "Cloud Affected Solar UV Predictions with Three-Phase Wavelet Hybrid Convolutional Long Short-Term Memory Network Multi-Step Forecast System." IEEE Access. 10, pp. 24704-24720. https://doi.org/10.1109/ACCESS.2022.3153475Development and evaluation of hybrid deep learning long short-term memory network model for pan evaporation estimation trained with satellite and ground-based data
Jayasinghe, W. J. M. Lakmini Prarthana, Deo, Ravinesh C., Ghahramani, Afshin, Ghimire, Sujan and Raj, Nawin. 2022. "Development and evaluation of hybrid deep learning long short-term memory network model for pan evaporation estimation trained with satellite and ground-based data." Journal of Hydrology. 607, pp. 1-19. https://doi.org/10.1016/j.jhydrol.2022.127534Integrative artificial intelligence models for Australian coastal sediment lead prediction: An investigation of in-situ measurements and meteorological parameters effects
Bhagat, Suraj Kumar, Tiyasha, Tiyasha, Kumar, Adarsh, Malik, Tabarak, Jawad, Ali H., Khedher, Khaled Mohamed, Deo, Ravinesh C. and Yaseen, Zaher Mundher. 2022. "Integrative artificial intelligence models for Australian coastal sediment lead prediction: An investigation of in-situ measurements and meteorological parameters effects." Journal of Environmental Management. 309, pp. 1-16. https://doi.org/10.1016/j.jenvman.2022.114711Assessment and Prediction of Sea Level Trend in the South Pacific Region
Raj, Nawin, Gharineiat, Zahra, Ahmed, Abul Abrar Masrur and Stepanyants, Yury. 2022. "Assessment and Prediction of Sea Level Trend in the South Pacific Region." Remote Sensing. 14 (4), pp. 1-25. https://doi.org/10.3390/rs14040986An Eigenvalues-Based Covariance Matrix Bootstrap Model Integrated With Support Vector Machines for Multichannel EEG Signals Analysis
Al-Hadeethi, Hanan, Abdulla, Shahab, Diykh, Mohammed, Deo, Ravinesh C. and Green, Jonathan H.. 2022. "An Eigenvalues-Based Covariance Matrix Bootstrap Model Integrated With Support Vector Machines for Multichannel EEG Signals Analysis." Frontiers in Neuroinformatics. 15, pp. 1-15. https://doi.org/10.3389/fninf.2021.808339Stacked LSTM Sequence-to-Sequence Autoencoder with Feature Selection for Daily Solar Radiation Prediction: A Review and New Modeling Results
Ghimire, Sujan, Deo, Ravinesh C., Wang, Hua, Al-Musaylh, Mohanad S., Casillas-Perez, David and Salcedo-sanz, Sancho. 2022. "Stacked LSTM Sequence-to-Sequence Autoencoder with Feature Selection for Daily Solar Radiation Prediction: A Review and New Modeling Results." Energies. 15 (3), pp. 1-39. https://doi.org/10.3390/en15031061Texture analysis based graph approach for automatic detection of neonatal seizure from multi-channel EEG signals
Diykh, Mohammed, Miften, Firas Sabar, Abdulla, Shahab, Deo, Ravinesh C., Siuly, Siuly, Green, Jonathan H. and Oudah, Atheer Y.. 2022. "Texture analysis based graph approach for automatic detection of neonatal seizure from multi-channel EEG signals." Measurement. 190 (110731), pp. 1-13. https://doi.org/10.1016/j.measurement.2022.110731Air quality monitoring based on chemical and meteorological drivers: Application of a novel data filtering-based hybridized deep learning model
Jamei, Mehdi, Ali, Mumtaz, Malik, Anurag, Karbasi, Masoud, Sharma, Ekta and Yaseen, Zaher Mundher. 2022. "Air quality monitoring based on chemical and meteorological drivers: Application of a novel data filtering-based hybridized deep learning model." Journal of Cleaner Production. 374, pp. 1-17. https://doi.org/10.1016/j.jclepro.2022.134011General equilibrium impact evaluation of food top-up induced by households’ renewable power self-supply in 141 regions
Nguyen, Duong Binh, Nong, Duy, Simshauser, Paul and Nguyen-Huy, Thong. 2022. "General equilibrium impact evaluation of food top-up induced by households’ renewable power self-supply in 141 regions." Applied Energy. 306 (Part B), pp. 1-13. https://doi.org/10.1016/j.apenergy.2021.118126Classification of catchments for nitrogen using Artificial Neural Network Pattern Recognition and spatial data
O'Sullivan, Cherie M., Ghahramani, Afshin, Deo, Ravinesh C., Pembleton, Keith, Khan, Urooj and Tuteja, Narendra. 2022. "Classification of catchments for nitrogen using Artificial Neural Network Pattern Recognition and spatial data." Science of the Total Environment. 809, pp. 1-15. https://doi.org/10.1016/j.scitotenv.2021.151139Copula-based statistical modelling of synoptic-scale climate indices for quantifying and managing agricultural risks in Australia
Nguyen-Huy, Thong. 2020. "Copula-based statistical modelling of synoptic-scale climate indices for quantifying and managing agricultural risks in Australia." Bulletin of the Australian Mathematical Society. 101 (1), pp. 166-169. https://doi.org/10.1017/S0004972719001217Drought Outlook Products Review
Cobon, David, Nguyen-Huy, Thong and Reardon-Smith, Kate. 2019. Drought Outlook Products Review. Toowoomba, Australia. University of Southern Queensland.Study on the Development of Neutrosophic Triplet Ring and Neutrosophic Triplet Field
Ali, Mumtaz, Smarandache, Florentin and Khan, Mohsin. 2018. "Study on the Development of Neutrosophic Triplet Ring and Neutrosophic Triplet Field." Mathematics. 6, pp. 1-11. https://doi.org/10.3390/math6040046Domino effect of climate change over two millennia in ancient China’s Hexi Corridor
Feng, Qi, Yan, Linshan, Deo, Ravinesh C., AghaKouchak, Amir, Adamowski, Jan F., Stone, Roger, Yin, Zhenliang, Liu, Wei, Si, Jianhua, Wen, Xiaohu, Zhu, Meng and Cao, Shixiong. 2019. "Domino effect of climate change over two millennia in ancient China’s Hexi Corridor." Nature Sustainability. 2, pp. 957-961. https://doi.org/10.1038/s41893-019-0397-9Bat algorithm for dam–reservoir operation
Ethteram, Mohammad, Mousavi, Sayed-Farhad, Karami, Hojat, Farzin, Saeed, Deo, Ravinesh, Othman, Faridah Binti, Chau, Kwok-Wing, Sarkamaryan, Saeed, Singh, Vijay P. and El-Shafie, Ahmed. 2018. "Bat algorithm for dam–reservoir operation." Environmental Earth Sciences. 77 (13), pp. 1-15. https://doi.org/10.1007/s12665-018-7662-5Characteristics of ecosystem water use efficiency in a desert riparian forest
Ma, Xiaohong, Feng, Qi, Su, Yonghong, Yu, Tengfei and Deo, Ravinesh C.. 2018. "Characteristics of ecosystem water use efficiency in a desert riparian forest." Environmental Earth Sciences. 77 (358). https://doi.org/10.1007/s12665-018-7518-zThe influence of climatic inputs on stream-flow pattern forecasting: case study of Upper Senegal River
Diop, Lamine, Bodian, Ansoumana, Djaman, Koffi, Yaseen, Zaher Mundher, Deo, Ravinesh C., El-Shafie, Ahmed and Brown, Larry C.. 2018. "The influence of climatic inputs on stream-flow pattern forecasting: case study of Upper Senegal River." Environmental Earth Sciences. 77 (5). https://doi.org/10.1007/s1266Uncertainty assessment of the multilayer perceptron (MLP) neural network model with implementation of the novel hybrid MLP-FFA method for prediction of biochemical oxygen demand and dissolved oxygen: a case study of Langat River
Raheli, Bahare, Aalami, Mohammad Taghi, El-Shafie, Ahmed, Ghorbani, Mohammad Ali and Deo, Ravinesh C.. 2017. "Uncertainty assessment of the multilayer perceptron (MLP) neural network model with implementation of the novel hybrid MLP-FFA method for prediction of biochemical oxygen demand and dissolved oxygen: a case study of Langat River." Environmental Earth Sciences. 76 (14). https://doi.org/10.1007/s12665-017-6842-zMethodology for producing the Drought Monitor
Pudmenzky, Christa, Guillory, Laura, Cobon, David H. and Nguyen-Huy, Thong. 2020. Methodology for producing the Drought Monitor. Toowoomba, Queensland. University of Southern Queensland.Neutrosophic Recommender System for Medical Diagnosis Based on Algebraic Similarity Measure and Clustering
Thanh, Nguyen Dang, Son, Le Hoang and Ali, Mumtaz. 2017. "Neutrosophic Recommender System for Medical Diagnosis Based on Algebraic Similarity Measure and Clustering." 2017 IEEE International Conference on Fuzzy Systems (FUZZ-IEEE). Naples, Italy 09 - 12 Jul 2017 Piscataway, United States. https://doi.org/10.1109/FUZZ-IEEE.2017.8015387An intuitionistic fuzzy clustering algorithm based on a new correlation coefficient with application in medical diagnosis
Thao, Nguyen Xuan, Ali, Mumtaz and Smarandache, Florentin. 2019. "An intuitionistic fuzzy clustering algorithm based on a new correlation coefficient with application in medical diagnosis." Journal of Intelligent and Fuzzy Systems. 36 (1), pp. 189-198. https://doi.org/10.3233/JIFS-181084Linguistic Approaches to Interval Complex Neutrosophic Sets in Decision Making
Dat, Luu Quoc, Thong, Nguyen Tho, Son, Le Hoang, Ali, Mumtaz, Smarandache, Florentin, Abdel-Basset, Mohamed and Long, Hoang Viet. 2019. "Linguistic Approaches to Interval Complex Neutrosophic Sets in Decision Making." IEEE Access. 7, pp. 38902-38917. https://doi.org/10.1109/ACCESS.2019.2902841Northern Australia Climate Program: supporting adaptation in rangeland grazing systems through more targeted climate forecasts, improved drought information and an innovative extension program
Cobon, David, Jarvis, Chelsea, Reardon-Smith, Kate, Guillory, Laura, Pudmenzky, Christa, Nguyen-Huy, Thong, Mushtaq, Shahbaz and Stone, Roger. 2021. "Northern Australia Climate Program: supporting adaptation in rangeland grazing systems through more targeted climate forecasts, improved drought information and an innovative extension program." The Rangeland Journal. 43 (3), pp. 87-100. https://doi.org/10.1071/RJ20074Dynamic interval valued neutrosophic set: Modeling decision making in dynamic environments
Thong, Nguyen Tho, Dat, Luu Quoc, Son, Le Hoang, Hoa, Nguyen Dinh, Ali, Mumtaz and Smarandache, Florentin. 2019. "Dynamic interval valued neutrosophic set: Modeling decision making in dynamic environments." Computers in Industry. 108, pp. 45-52. https://doi.org/10.1016/j.compind.2019.02.009Fuzzy and neutrosophic modeling for link prediction in social networks
Tuan, Tran Manh, Chuan, Pham Minh, Ali, Mumtaz, Ngan, Tran Thi, Mittal, Mamta and Son, Le Hoang. 2019. "Fuzzy and neutrosophic modeling for link prediction in social networks." Evolving Systems. 10, pp. 629-634. https://doi.org/10.1007/s12530-018-9251-yNovel hybrid deep learning model for satellite based PM10 forecasting in the most polluted Australian hotspots
Sharma, Ekta, Deo, Ravinesh C., Soar, Jeffrey, Prasad, Ramendra, Parisi, Alfio V. and Raj, Nawin. 2022. "Novel hybrid deep learning model for satellite based PM10 forecasting in the most polluted Australian hotspots." Atmospheric Environment. 279, pp. 1-13. https://doi.org/10.1016/j.atmosenv.2022.119111New double decomposition deep learning methods for river water level forecasting
Ahmed, A. A. Masrur, Deo, Ravinesh C., Ghahramani, Afshin, Feng, Qi, Raj, Nawin, Yin, Zhenliang and Yang, Linshan. 2022. "New double decomposition deep learning methods for river water level forecasting." Science of the Total Environment. 831, pp. 1-21. https://doi.org/10.1016/j.scitotenv.2022.154722Global disparities in agricultural climate index-based insurance research
Adeyinka, Adewuyi Ayodele, Kath, Jarrod, Nguyen-Huy, Thong, Mushtaq, Shahbaz, Souvignet, Maxime, Range, Matthias and Barratt, Jonathan. 2022. "Global disparities in agricultural climate index-based insurance research." Climate Risk Management. 35, pp. 1-15. https://doi.org/10.1016/j.crm.2022.100394Spatiotemporal Hybrid Random Forest Model for Tea Yield Prediction Using Satellite-Derived Variables
Jui, S. Janifer Jabin, Ahmed, A. A. Masrur, Bose, Aditi, Raj, Nawin, Sharma, Ekta, Soar, Jeffrey and Chowdhury, Md Wasique Islam. 2022. "Spatiotemporal Hybrid Random Forest Model for Tea Yield Prediction Using Satellite-Derived Variables." Remote Sensing. 14 (3), pp. 1-18. https://doi.org/10.3390/rs14030805Optimization algorithms as training approach with hybrid deep learning methods to develop an ultraviolet index forecasting model
Ahmed, A. A. Masrur, Ahmed, Mohammad Hafez, Saha, Sanjoy Kanti, Ahmed, Oli and Sutradhar, Ambica. 2022. "Optimization algorithms as training approach with hybrid deep learning methods to develop an ultraviolet index forecasting model." Stochastic Environmental Research and Risk Assessment. 36 (10), pp. 3011-3039. https://doi.org/10.1007/s00477-022-02177-3Drought outlook validation using ACCESS-S2 hindcast
Nguyen-Huy, Thong and Cobon, David. 2022. Drought outlook validation using ACCESS-S2 hindcast. Northern Australia Climate Program (NACP).Systemic risk management in farming - geographic distribution and diversity
Nguyen-Huy, Thong. 2022. "Systemic risk management in farming - geographic distribution and diversity." Future Drought Fund’s 2022 Science to Practice Forum. 07 - 08 Jun 2022 Australia.Multi-step daily forecasting of reference evapotranspiration for different climates of India: A modern multivariate complementary technique reinforced with ridge regression feature selection
Malik, Anurag, Jamei, Mehdi, Ali, Mumtaz, Prasad, Ramendra, Karbasi, Masoud and Yaseen, Zaher Mundher. 2022. "Multi-step daily forecasting of reference evapotranspiration for different climates of India: A modern multivariate complementary technique reinforced with ridge regression feature selection." Agricultural Water Management. 272. https://doi.org/10.1016/j.agwat.2022.107812A hierarchical classification/regression algorithm for improving extreme wind speed events prediction
Pelaez-Rodriguez, C., Perez-Aracil, J., Fister, D, Prieto-Godino, L., Deo, R.C. and Salcedo-sanz, S.. 2022. "A hierarchical classification/regression algorithm for improving extreme wind speed events prediction." Renewable Energy. 201 (Part 2), pp. 157-178. https://doi.org/10.1016/j.renene.2022.11.042Designing a Multi-Stage Expert System for daily ocean wave energy forecasting: A multivariate data decomposition-based approach
Jamei, Mehdi, Ali, Mumtaz, Karbasi, Masoud, Xiang, Yong, Ahmadianfar, Iman and Yaseen, Zaher Mundher. 2022. "Designing a Multi-Stage Expert System for daily ocean wave energy forecasting: A multivariate data decomposition-based approach ." Applied Energy. 326, pp. 1-24. https://doi.org/10.1016/j.apenergy.2022.119925Forecasting weekly reference evapotranspiration using Auto Encoder Decoder Bidirectional LSTM model hybridized with a Boruta-CatBoost input optimizer
Karbasi, Masoud, Jamei, Mehdi, Ali, Mumtaz, Malik, Anurag and Yaseen, Zaher Mundher. 2022. "Forecasting weekly reference evapotranspiration using Auto Encoder Decoder Bidirectional LSTM model hybridized with a Boruta-CatBoost input optimizer." Computers and Electronics in Agriculture. 198, pp. 1-17. https://doi.org/10.1016/j.compag.2022.107121Artificial Intelligence and Clean Air: Development of Novel Algorithms with Machine Learning and Deep Learning
Sharma, Ekta. 2022. Artificial Intelligence and Clean Air: Development of Novel Algorithms with Machine Learning and Deep Learning. PhD by Publication Doctor of Philosophy. University of Southern Queensland. https://doi.org/10.26192/q7q7wDevelopment of Deep Learning Hybrid Models for Hydrological Predictions
Ahmed, Abul Abrar Masrur. 2022. Development of Deep Learning Hybrid Models for Hydrological Predictions. PhD by Publication Doctor of Philosophy. University of Southern Queensland. https://doi.org/10.26192/q7q5z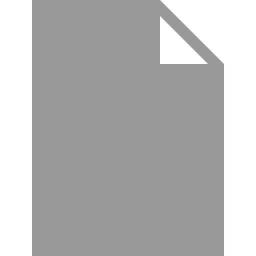