Identifying sensors for better IPM in cotton: NEC1901
Technical report
Title | Identifying sensors for better IPM in cotton: NEC1901 |
---|---|
Report Type | Technical report |
Research Report Category | Industry |
Authors | McCarthy, Alison, Long, Derek and Grundy, Paul |
Institution of Origin | University of Southern Queensland |
Number of Pages | 57 |
Year | 2022 |
Publisher | Cotton Research and Development Corporation |
Place of Publication | Australia |
Abstract | Insect pests in cotton can cause significant economic loss if undetected or managed incorrectly. Pest populations and plant symptoms are typically monitored manually by agronomists. Accurate pest detection can be difficult with many pests such as Silverleaf Whitefly (SLW) and aphids located on the undersides of leaves and can have irregular distribution throughout a field area. Accurate detection and spatial/temporal tracking of pests is critical to an effective cotton integrated pest management (IPM) strategy that relies on timely decision making. Machine vision has potential to detect cotton pests and symptoms using handheld cameras and software through automatic image analysis. This project developed an automated SLW and aphid counting App for agronomists and growers to improve cotton IPM. This involved working closely with agronomists in workshops to identify required functions of the App. Images were collected using smartphones over four cotton seasons and glasshouse trials and machine vision algorithms developed that counted healthy and parasitised SLW and aphids with 75% accuracy. The algorithms were implemented in UniSQ-developed Apps, as an alpha version for image collection by QDAF, and then as a beta version (25+ users) for image collection and visualisation with the CRDC/CSIRO/QDAF’s Decision Support Tool (DST) for spray decisions. Clevvi Marketing were identified as a commercial partner for App development through CRDC’s Expression of Interest process and developed a version of the App for public release. The project also investigated using imagery and automated analysis to detect honeydew on cotton plants. This involved conducting glasshouse and commercial field trials with known SLW levels and assessing collected imagery. |
Keywords | Machine vision, insects, image analysis |
ANZSRC Field of Research 2020 | 300202. Agricultural land management |
460304. Computer vision | |
Public Notes | Files associated with this item cannot be displayed due to copyright restrictions. |
Byline Affiliations | University of Southern Queensland |
Department of Agriculture and Fisheries, Queensland |
https://research.usq.edu.au/item/z22q1/identifying-sensors-for-better-ipm-in-cotton-nec1901
27
total views3
total downloads7
views this month0
downloads this month
Export as
Related outputs
Digital strategies for nitrogen management in grain production systems: lessons from multi-method assessment using on-farm experimentation
Colaco, A.F., Whelan, B.M., Bramley, R.G.V., Richetti, J., Fajardo, M., McCarthy, A.C., Perry, E.M., Bender, A., Leo, S., Fitzgerald, G.J. and Lawes, R.A.. 2024. "Digital strategies for nitrogen management in grain production systems: lessons from multi-method assessment using on-farm experimentation ." Precision Agriculture. https://doi.org/10.1007/s11119-023-10102-zTactile sensing for tissue discrimination in robotic meat cutting: A feasibility study.
Aly, Basem Adel, Low, Tobias, Long, Derek, Brett, Peter and Baillie, Craig. 2024. "Tactile sensing for tissue discrimination in robotic meat cutting: A feasibility study. " Journal of Food Engineering. 363. https://doi.org/10.1016/j.jfoodeng.2023.111754Artificial Intelligence: what is it and what does it mean for cotton?
McCarthy, Alison. 2023. "Artificial Intelligence: what is it and what does it mean for cotton?" 2023 Australian Cotton Research Conference. Toowoomba 05 - 07 Sep 2023 Australia.Variable-rate irrigation: hardware and software tools for improved infield irrigation
McCarthy, Alison and Foley, Joseph. 2023. "Variable-rate irrigation: hardware and software tools for improved infield irrigation." GRDC Grains Research Update: Irrigation efficiency. 30 - 30 May 2023 Australia.Research and industry engagement for Ag tech development
McCarthy, Alison. 2023. "Research and industry engagement for Ag tech development." Scenic Rim Agricultural Workshop. Aratula 22 - 22 Mar 2023 Australia.Robotics and sensing technologies in red meat processing: A review
Aly, Basem Adel, Low, Tobias, Long, Derek, Baillie, Craig and Brett, Peter. 2023. "Robotics and sensing technologies in red meat processing: A review." Trends in Food Science and Technology. 137, pp. 142-155. https://doi.org/10.1016/j.tifs.2023.05.015Field evaluation of automated site-specific irrigation for cotton and perennial ryegrass using soil-water sensors and Model Predictive Control
McCarthy, Alison, Foley, Joseph, Raedts, Pieter and Hills, James. 2023. "Field evaluation of automated site-specific irrigation for cotton and perennial ryegrass using soil-water sensors and Model Predictive Control." Agricultural Water Management. 277, pp. 1-17. https://doi.org/10.1016/j.agwat.2022.108098Potential for machine vision of grain crop features for nitrogen assessment
McCarthy, Alison, Colaco, Andre, Richetti, Jonathan and Baillie, Craig. 2022. "Potential for machine vision of grain crop features for nitrogen assessment." 20th Australian Agronomy Conference (2022). Toowoomba, Australia Toowoomba, Australia.Future farming: Machine vision for in-season nitrogen assessment of grain crops
McCarthy, Alison and Baillie, Craig. 2020. "Future farming: Machine vision for in-season nitrogen assessment of grain crops." 2020 Precision Agriculture Symposium Webinar. Virtual 30 Sep 2020 Sydney, Australia.Future farming: Machine vision for nitrogen assessment in grain crops
McCarthy, Alison and Baillie, Craig. 2020. "Future farming: Machine vision for nitrogen assessment in grain crops." GRDC Grains Research Update: Driving Profit Through Research. Goondiwindi, Australia 03 - 04 Mar 2020 Australia.Smartphone apps under development to aid pest monitoring
McCarthy, Alison, Long, Derek and Grundy, Paul. 2020. "Smartphone apps under development to aid pest monitoring." GRDC Grains Research Update: Driving Profit Through Research. Goondiwindi, Australia 03 - 04 Mar 2020 Australia.Detection of early infestations of Fall armyworm (FAW) in sweet corn and maize
McCarthy, Alison and Humpal, Jacob. 2021. Detection of early infestations of Fall armyworm (FAW) in sweet corn and maize. Toowoomba, Australia. University of Southern Queensland.Review of Technologies, Regulations and Operating Standards for Field Based Autonomous Agricultural Machinery
Baillie, Craig, Torrance, Logan, Long, Derek, Brett, Peter and Humpal, Jacob. 2020. Review of Technologies, Regulations and Operating Standards for Field Based Autonomous Agricultural Machinery. Toowoomba, Australia. University of Southern Queensland.Automated variety trial plot growth and flowering detection for maize and soybean using machine vision
McCarthy, Alison and Raine, Steven. 2022. "Automated variety trial plot growth and flowering detection for maize and soybean using machine vision." Computers and Electronics in Agriculture. 194, pp. 1-21. https://doi.org/10.1016/j.compag.2022.106727Machine vision tools for Smarter Irrigation of dairy pasture and cotton
McCarthy, Alison, Foley, Joseph, Jamali, Hizbullah, Raedts, Pieter and Hills, James. 2022. "Machine vision tools for Smarter Irrigation of dairy pasture and cotton." 2022 Irrigation Australia Conference and Exhibition. Adelaide, Australia 05 - 07 Oct 2022 Australia.Infield sensing of cotton insects using automated image analysis of crop symptoms: CSP2203
Heimoana, Simone, Blankley, S. and McCarthy, Alison. 2022. Infield sensing of cotton insects using automated image analysis of crop symptoms: CSP2203. Australia. Cotton Research and Development Corporation.Automated infield canopy temperature assessment of early stage cotton using thermal camera and plant segmentation
McCarthy, Alison, Nunn, Christopher, Jamali, Hizbullah and Long, Derek. 2022. Automated infield canopy temperature assessment of early stage cotton using thermal camera and plant segmentation . Australia. Cotton Research and Development Corporation.Cotton health assessment and yield prediction
McCarthy, Alison and Baillie, Craig. 2022. Cotton health assessment and yield prediction. United States. John Deere.Future Farm: Improving farmer confidence in targeted N management through automated sensing and decision support
Bramley, Rob, Whelan, Brett, Colaco, André, McCarthy, Alison, Richetti, Jonathan, Lawes, Roger, Fajardo, Mario, Bender, Asher, Pothula, Anand, Baillie, Craig, Perry, Eileen, Fitzgerald, Glenn, Clancy, Alex, Leo, Stephen and Grace, Peter. 2022. Future Farm: Improving farmer confidence in targeted N management through automated sensing and decision support. Australia. Grains Research and Development Corporation.Improving pasture growth assessment using machine vision
McCarthy, Alison, Raedts, Pieter, Foley, Joseph and Hills, James. 2022. "Improving pasture growth assessment using machine vision." Australasian Dairy Science Symposium 2022. Twin Waters 30 Nov - 02 Dec 2022New sensing technologies for citrus gall wasp and Fuller’s rose weevil: CT19009
Long, Derek, McCarthy, Alison and Mo, Jianhua. 2021. New sensing technologies for citrus gall wasp and Fuller’s rose weevil: CT19009. Australia. Hort Innovation Austarlia.Machine vision and automation technologies for the Australian cotton industry
McCarthy, Alison. 2019. "Machine vision and automation technologies for the Australian cotton industry." 78th International Cotton Advisory Committee Plenary Meeting (ICAC 2019). Brisbane, Australia 02 - 07 Dec 2019Machine vision App for automated cotton insect counting: initial development and first results
Long, Derek, Grundy, Paul and McCarthy, Alison. 2019. "Machine vision App for automated cotton insect counting: initial development and first results." Australian Association of Cotton Scientists 2019 Australian Cotton Research Conference (AACS 2019). Armidale, Australia 28 - 30 Oct 2019In-season yield prediction using VARIwise
McCarthy, Alison, O'Keeffe, Kieran and McKay, Andrew. 2019. "In-season yield prediction using VARIwise." Australian Association of Cotton Scientists 2019 Australian Cotton Research Conference (AACS 2019). Armidale, Australia 28 - 30 Oct 2019Site-specific irrigation using automated control and machine vision for horticulture crops in Queensland and New Zealand
McCarthy, Alison, El-Naggar, Ahmed, Roudier, Pierre and Hedley, Carolyn. 2017. "Site-specific irrigation using automated control and machine vision for horticulture crops in Queensland and New Zealand." 18th Australian Agronomy Conference 2017. Ballarat, Victoria 24 - 28 Sep 2017 Australia.Automated cloud-based irrigation for centre pivot irrigated cotton and dairy pasture
McCarthy, Alison, Foley, Joseph, Hills, James, Shippam, Ralph and McLaren, David. 2018. "Automated cloud-based irrigation for centre pivot irrigated cotton and dairy pasture." 2018 Irrigation Australia International Conference and Exhibition. Sydney, Australia 13 - 15 Jun 2018Advanced data-driven irrigation
Hills, James, McLaren, David, Foley, Joseph and McCarthy, Alison. 2018. "Advanced data-driven irrigation." Where the Waters Meet 2018 - Australian Water Association, Tasmanian State Conference. Hobart, Australia 16 Aug 2018Aerial imagery for yield prediction
McCarthy, Alison and Foley, Joseph. 2018. "Aerial imagery for yield prediction." 2018 Australian Cotton Conference. Gold Coast, Australia 07 - 09 Aug 2018Machine vision for camera-based horticulture crop growth monitoring
McCarthy, Alison, Hedley, Carolyn and El-Naggar, Ahmed. 2017. "Machine vision for camera-based horticulture crop growth monitoring." International Tri-Conference for Precision Agriculture in 2017 (PA17)/7th Asian-Australasian Conference on Precision Agriculture. Hamilton, New Zealand 16 - 18 Oct 2017 New Zealand. https://doi.org/10.5281/zenodo.893702Machine vision tracking of water advance in cotton surface irrigation with an unmanned aerial system
Long, Derek. 2018. Machine vision tracking of water advance in cotton surface irrigation with an unmanned aerial system. PhD Thesis Doctor of Philosophy. University of Southern Queensland. https://doi.org/10.26192/5f643ba5ce22bKnowledge management, sensing and control tools for irrigated broadacre cropping
McCarthy, Alison, Scobie, Michael, Gillies, Malcolm, Agustina, Lidya and Foley, Joseph. 2016. "Knowledge management, sensing and control tools for irrigated broadacre cropping." 19th Symposium on Precision Agriculture in Australasia. Toowoomba, Australia 12 - 13 Sep 2016Automated camera-based crop monitoring and site-specific irrigation control systems for cotton, horticulture and dairy pasture
McCarthy, Alison. 2016. "Automated camera-based crop monitoring and site-specific irrigation control systems for cotton, horticulture and dairy pasture." The SPAA Expo: Talking precision in the Atherton Tableland (2016). Mareeba, AustraliaAutomated camera-based crop monitoring and site-specific irrigation control systems
McCarthy, Alison. 2016. "Automated camera-based crop monitoring and site-specific irrigation control systems." Precision Agriculture Technologies: Recent applications in the horticultural industry (2016). Gatton, Australia 24 Nov 2016Improving surface irrigation
Gillies, Malcolm H., Foley, Joseph P. and McCarthy, Alison C.. 2018. "Improving surface irrigation." Chen, Guangnan (ed.) Advances in agricultural machinery and technologies. United States. CRC Press. pp. 225-262Tools to aid autonomous irrigation
McCarthy, Alison. 2017. "Tools to aid autonomous irrigation." GVIA Grower-led Irrigation Research Field Day (2017). Moree, Australia 08 Feb 2017Fixed camera system for phenology measurements during the 2018 field season
McCarthy, Alison and Raine, Steven. 2018. Fixed camera system for phenology measurements during the 2018 field season. United States. Monsanto.Smart Automated Broad-acre Irrigation (Smarter Irrigation for Profit RRDP1603)
Foley, J.P., Gillies, M.H. and McCarthy, A.C.. 2018. Smart Automated Broad-acre Irrigation (Smarter Irrigation for Profit RRDP1603). Australia. Cotton Research and Development Corporation.Sense-T: Sensor smart irrigation
McCarthy, Alison, Shippam, Ralph and Agustina, Lidya. 2017. Sense-T: Sensor smart irrigation. Australia. Sense-T.Automated camera-based crop and irrigation monitoring
McCarthy, Alison. 2016. "Automated camera-based crop and irrigation monitoring." 18th Australian Cotton Conference (2016). Gold Coast, Australia 02 - 04 Aug 2016Smarter irrigation for profit - increasing farm profit through efficient use of irrigation input to dairy pastures
Hills, James, McCarthy, Alison, Foley, Joseph and McLaren, David. 2016. "Smarter irrigation for profit - increasing farm profit through efficient use of irrigation input to dairy pastures." 7th Australian Dairy Science Symposium (2016). Sydney, Australia 16 - 18 Nov 2016 Australia.Improving horticulture irrigation and fertiliser application using real-time, adaptive control
McCarthy, Alison. 2017. Improving horticulture irrigation and fertiliser application using real-time, adaptive control. Australia. Queensland Government.Row and water front detection from UAV thermal-infrared imagery for furrow irrigation monitoring
Long, Derek, McCarthy, Cheryl and Jensen, Troy. 2016. "Row and water front detection from UAV thermal-infrared imagery for furrow irrigation monitoring." 2016 IEEE/ASME International Conference on Advanced Intelligent Mechatronics (AIM 2016). Banff, Canada 12 - 15 Jul 2016 https://doi.org/10.1109/AIM.2016.7576783Optimal irrigation of cotton via real-time, adaptive control
McCarthy, Alison, Smith, Rod and Hancock, Nigel. 2013. Optimal irrigation of cotton via real-time, adaptive control. Narrabri, Australia. Cotton Research and Development Corporation.Advancing VARIwise towards autonomous irrigation and a grower's guide
McCarthy, Alison, Antille, Diogenes, Skowronski, Victor, Zhao, Bo, Kodur, Shreevatsa, Nguyen-Ky, Tai and Raine, Steven. 2016. Advancing VARIwise towards autonomous irrigation and a grower's guide. Narrabri, Australia. Cotton Research and Development Corporation.A preliminary evaluation of using fixed cameras for phenology measurements in a maize and soybean crop 2016/17
McCarthy, Alison and Raine, Steven. 2017. A preliminary evaluation of using fixed cameras for phenology measurements in a maize and soybean crop 2016/17. United States. Monsanto.Remote monitoring and automatic detection of grain crop attributes
McCarthy, Alison, Tscharke, Matthew and Schmidt, Erik. 2014. Remote monitoring and automatic detection of grain crop attributes. Toowoomba, Australia. University of Southern Queensland.Automated site-specific irrigation framework and evaluation
McCarthy, Alison. 2016. "Automated site-specific irrigation framework and evaluation." Irrigation Australia International Conference and Exhibition 2016: Irrigation - For Prosperity and Wellbeing. Melbourne, Australia 24 - 26 May 2016VARIwise: site-specific surface irrigation and fertigation using adaptive control
McCarthy, Alison. 2015. "VARIwise: site-specific surface irrigation and fertigation using adaptive control." 2015 Cotton Irrigation Technology Tour. Emerald & Moree & Nevertire, Australia 10 - 12 Feb 2015 Australia.Development of an integrated plant-based image sensing system for soil-water and nitrogen status estimation in cotton
McCarthy, Alison and Nguyen, Tai. 2015. Development of an integrated plant-based image sensing system for soil-water and nitrogen status estimation in cotton. Toowoomba, Australia. University of Southern Queensland.Control and sensing systems for automated site-specific irrigation
McCarthy, Alison. 2015. "Control and sensing systems for automated site-specific irrigation." CCA Cropping Solutions Seminar 2015. Moree, Australia 29 - 30 Jul 2015Automated site-specific control and sensing for irrigating dairy pastures
McCarthy, Alison. 2015. "Automated site-specific control and sensing for irrigating dairy pastures." Tasmanian Institute of Agriculture Dairy Centre Open Day 2015: Tips, Tools and Technologies to Better Manage Climate Variability. Burnie, Australia 02 Dec 2015Image analysis and artificial intelligence based approach for soil-water and nitrogen status estimation
McCarthy, Alison, Nguyen, Tai and Raine, Steven. 2015. "Image analysis and artificial intelligence based approach for soil-water and nitrogen status estimation." 2nd Australian Cotton Research Conference 2015: Science Securing Cotton's Future. Toowoomba, Australia 08 - 10 Sep 2015 Australia.Data requirements for automated model-based control of irrigation and fertiliser application
McCarthy, Alison and Raine, Steven. 2015. "Data requirements for automated model-based control of irrigation and fertiliser application." Acuna, T., Moeller, C., Parsons, D. and Harrison, M. (ed.) 17th Australian Agronomy Conference 2015: Building Productive, Diverse and Sustainable Landscapes (AAC 2015). Hobart, Australia 20 - 24 Sep 2015 Warragul, Australia.Automated camera-based height and flower detection for wheat and chickpea
McCarthy, Alison and Tscharke, Matthew. 2014. "Automated camera-based height and flower detection for wheat and chickpea." 5th International Workshop on Applications of Computer Image Analysis and Spectroscopy in Agriculture (ASABE 2014). Montreal, Canada 12 - 13 Jul 2014 St. Joseph, MI. United States.Real-time irrigation decision-making and control for site-specific irrigation of cotton using a centre pivot, 2012/13
McCarthy, Alison, Smith, Rod and Hancock, Nigel. 2014. "Real-time irrigation decision-making and control for site-specific irrigation of cotton using a centre pivot, 2012/13." Irrigation Australia Conference 2014: Water for Life, Future for All. Gold Coast, Australia 02 - 06 Jun 2014 Sydney, Australia.VARIwise: towards autonomous irrigation and a grower's guide
McCarthy, A. C.. 2014. "VARIwise: towards autonomous irrigation and a grower's guide." IRRICOMM – communication of irrigation research in cotton (2014). Narrabri, Australia 11 - 12 Mar 2014 Narrabri, Australia.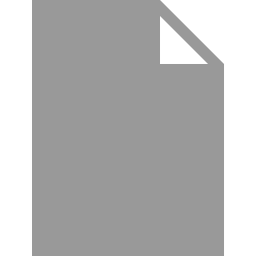