Machine vision tools for Smarter Irrigation of dairy pasture and cotton
Presentation
Paper/Presentation Title | Machine vision tools for Smarter Irrigation of dairy pasture and cotton |
---|---|
Presentation Type | Presentation |
Authors | McCarthy, Alison, Foley, Joseph, Jamali, Hizbullah, Raedts, Pieter and Hills, James |
Number of Pages | 19 |
Year | 2022 |
Place of Publication | Australia |
Web Address (URL) of Conference Proceedings | https://www.irrigationaustralia.com.au/Web/Shared_Content/Events/Event_Display.aspx?EventKey=IACE |
Conference/Event | 2022 Irrigation Australia Conference and Exhibition |
Event Details | 2022 Irrigation Australia Conference and Exhibition Delivery In person Event Date 05 to end of 07 Oct 2022 Event Location Adelaide, Australia Event Venue Adelaide Convention Centre Event Description IAL Conference Theme: IRRIGATION FOR THE FUTURE – Challenges, Innovations and Opportunities The Irrigation Australia Conference aims to provide a platform for the irrigation industry and the broad range of other stakeholders to address the key future challenges and opportunities facing the irrigation industry and to share their knowledge and experience to provide a sustainable future focused on the efficient use of water and adoption of new technology. The organising committee for the Irrigation Australia Conference have selected a conference theme and topics that specifically identify with irrigation issues in Australia. |
Abstract | Site-specific irrigation enables application of irrigation when and where it is needed using variable-rate hardware on centre pivot and lateral move irrigation machines. Commercial and research tools have been developed to automate the labour-intensive process of developing and uploading irrigation prescription maps to commercial variable-rate hardware. These software tools have different data and computational requirements, and potentially perform in different ways depending on irrigation availability and field variability scenarios. Common approaches include filling the soil-water deficits based on evapotranspiration, or using soil-water sensors and/or canopy temperature sensors to control irrigation. However, these approaches may not optimise the irrigation, if there is limited water, nor will they adapt to the different crop water requirements during different growth stages. Data analytics approaches (e.g. ‘Model Predictive Control’) involve automated analyses of predictive soil-plant-atmosphere models using in-field weather, soil and plant data, to consider water availability. These potentially enable improvements over existing approaches. Software has been developed to implement and simulate these irrigation control strategies for cotton (McCarthy et al. 2010, 2014). These strategies have been transferred to the Australian dairy industry using a pasture growth model, and consider grazing management differences across a field. This enables implementation of data analytics ‘Model Predictive Control’ irrigation optimisation in dairy pasture fields. A field trial has been conducted to compare the performance of four automated irrigation strategies at the TIA Dairy Research Farm (TDRF) in the 2019/20 summer season. The four irrigation strategies include uniform irrigation application, variable-rate irrigation based on a fixed historical map, soil-water deficit irrigation based on real-time data, and Model Predictive Control optimisation using available weather, soil and plant data with predictive modelling. The trial: (i) compared irrigation applied, energy use and productivity for five replicates these four alternative irrigation strategies needing different data requirements; (ii) conducted a cost benefit analysis for each irrigation strategy evaluated; and (iii) evaluated the ability to automate irrigation prescription map development. A plot-based comparison was conducted with five replicates of the four strategies following the approach of reported variable-rate trials (e.g. Hedley et al. 2011; Burdette Barker et al. 2018). A flow meter and energy sensor were installed on the centre pivot irrigation machine, soil-water sensors were installed in each soil-water deficit plot, and infield cameras were installed in each Model Predictive Control plot for assessing grazing status and pasture growth as required to calibrate the predictive model. The mean average error from the image analysis system for detecting pasture height as an indicator of yield, was 2.3 ± 1.9 cm. Periods of grazing were also detected as the pasture height reduced. This presentation will report the comparative performance of the irrigation strategies, and provide recommendations for use of commercial and research software tools for variable-rate irrigation in the Australian dairy industry. |
ANZSRC Field of Research 2020 | 3002. Agriculture, land and farm management |
460304. Computer vision | |
400705. Control engineering | |
Public Notes | Files associated with this item cannot be displayed due to copyright restrictions. |
Byline Affiliations | No affiliation |
Commonwealth Scientific and Industrial Research Organisation (CSIRO), Australia |
https://research.usq.edu.au/item/z365x/machine-vision-tools-for-smarter-irrigation-of-dairy-pasture-and-cotton
127
total views1
total downloads0
views this month0
downloads this month
Export as
Related outputs
Digital strategies for nitrogen management in grain production systems: lessons from multi-method assessment using on-farm experimentation
Colaco, A.F., Whelan, B.M., Bramley, R.G.V., Richetti, J., Fajardo, M., McCarthy, A.C., Perry, E.M., Bender, A., Leo, S., Fitzgerald, G.J. and Lawes, R.A.. 2024. "Digital strategies for nitrogen management in grain production systems: lessons from multi-method assessment using on-farm experimentation ." Precision Agriculture. 25 (2), pp. 983-1013. https://doi.org/10.1007/s11119-023-10102-zArtificial Intelligence: what is it and what does it mean for cotton?
McCarthy, Alison. 2023. "Artificial Intelligence: what is it and what does it mean for cotton?" 2023 Australian Cotton Research Conference. Toowoomba 05 - 07 Sep 2023 Australia.Variable-rate irrigation: hardware and software tools for improved infield irrigation
McCarthy, Alison and Foley, Joseph. 2023. "Variable-rate irrigation: hardware and software tools for improved infield irrigation." GRDC Grains Research Update: Irrigation efficiency. 30 - 30 May 2023 Australia.Research and industry engagement for Ag tech development
McCarthy, Alison. 2023. "Research and industry engagement for Ag tech development." Scenic Rim Agricultural Workshop. Aratula 22 - 22 Mar 2023 Australia.A Forage Allowance by Forage Type Interaction Impacts the Daily Milk Yield of Early Lactation Dairy Cows
Langworthy, Adam D., Freeman, Mark J., Hills, James L., McLaren, David K., Rawnsley, Richard P. and Pembleton, Keith G.. 2023. "A Forage Allowance by Forage Type Interaction Impacts the Daily Milk Yield of Early Lactation Dairy Cows." Animals. 13, pp. 1-18. https://doi.org/10.3390/ani13081406Field evaluation of automated site-specific irrigation for cotton and perennial ryegrass using soil-water sensors and Model Predictive Control
McCarthy, Alison, Foley, Joseph, Raedts, Pieter and Hills, James. 2023. "Field evaluation of automated site-specific irrigation for cotton and perennial ryegrass using soil-water sensors and Model Predictive Control." Agricultural Water Management. 277, pp. 1-17. https://doi.org/10.1016/j.agwat.2022.108098Potential for machine vision of grain crop features for nitrogen assessment
McCarthy, Alison, Colaco, Andre, Richetti, Jonathan and Baillie, Craig. 2022. "Potential for machine vision of grain crop features for nitrogen assessment." 20th Australian Agronomy Conference (2022). Toowoomba, Australia Toowoomba, Australia.Future farming: Machine vision for in-season nitrogen assessment of grain crops
McCarthy, Alison and Baillie, Craig. 2020. "Future farming: Machine vision for in-season nitrogen assessment of grain crops." 2020 Precision Agriculture Symposium Webinar. Virtual 30 Sep 2020 Sydney, Australia.Future farming: Machine vision for nitrogen assessment in grain crops
McCarthy, Alison and Baillie, Craig. 2020. "Future farming: Machine vision for nitrogen assessment in grain crops." GRDC Grains Research Update: Driving Profit Through Research. Goondiwindi, Australia 03 - 04 Mar 2020 Australia.Smartphone apps under development to aid pest monitoring
McCarthy, Alison, Long, Derek and Grundy, Paul. 2020. "Smartphone apps under development to aid pest monitoring." GRDC Grains Research Update: Driving Profit Through Research. Goondiwindi, Australia 03 - 04 Mar 2020 Australia.Detection of early infestations of Fall armyworm (FAW) in sweet corn and maize
McCarthy, Alison and Humpal, Jacob. 2021. Detection of early infestations of Fall armyworm (FAW) in sweet corn and maize. Toowoomba, Australia. University of Southern Queensland.Smarter Irrigation for Profit - Taggle IrriMATE Irrigation Advance Sensor & SMS notifier
Gillies, Malcolm, Foley, Joseph and Kelderman, Simon. 2018. Smarter Irrigation for Profit - Taggle IrriMATE Irrigation Advance Sensor & SMS notifier. Toowoomba, Australia. University of Southern Queensland.Automated variety trial plot growth and flowering detection for maize and soybean using machine vision
McCarthy, Alison and Raine, Steven. 2022. "Automated variety trial plot growth and flowering detection for maize and soybean using machine vision." Computers and Electronics in Agriculture. 194, pp. 1-21. https://doi.org/10.1016/j.compag.2022.106727Smarter Irrigation for Profit 2 RRDP2002 Precise real-time automated cotton and dairy irrigation for improved water productivity
Foley, J., Gillies, M., McCarthy, A., Kelderman, S., Eberhard, J. and Shippam, R.. 2022. Smarter Irrigation for Profit 2 RRDP2002 Precise real-time automated cotton and dairy irrigation for improved water productivity. Narrabri, Australia.. Cotton Research and Development Corporation.Infield sensing of cotton insects using automated image analysis of crop symptoms: CSP2203
Heimoana, Simone, Blankley, S. and McCarthy, Alison. 2022. Infield sensing of cotton insects using automated image analysis of crop symptoms: CSP2203. Australia. Cotton Research and Development Corporation.Automated infield canopy temperature assessment of early stage cotton using thermal camera and plant segmentation
McCarthy, Alison, Nunn, Christopher, Jamali, Hizbullah and Long, Derek. 2022. Automated infield canopy temperature assessment of early stage cotton using thermal camera and plant segmentation . Australia. Cotton Research and Development Corporation.Cotton health assessment and yield prediction
McCarthy, Alison and Baillie, Craig. 2022. Cotton health assessment and yield prediction. United States. John Deere.Future Farm: Improving farmer confidence in targeted N management through automated sensing and decision support
Bramley, Rob, Whelan, Brett, Colaco, André, McCarthy, Alison, Richetti, Jonathan, Lawes, Roger, Fajardo, Mario, Bender, Asher, Pothula, Anand, Baillie, Craig, Perry, Eileen, Fitzgerald, Glenn, Clancy, Alex, Leo, Stephen and Grace, Peter. 2022. Future Farm: Improving farmer confidence in targeted N management through automated sensing and decision support. Australia. Grains Research and Development Corporation.Identifying sensors for better IPM in cotton: NEC1901
McCarthy, Alison, Long, Derek and Grundy, Paul. 2022. Identifying sensors for better IPM in cotton: NEC1901. Australia. Cotton Research and Development Corporation.Improving pasture growth assessment using machine vision
McCarthy, Alison, Raedts, Pieter, Foley, Joseph and Hills, James. 2022. "Improving pasture growth assessment using machine vision." Australasian Dairy Science Symposium 2022 (ADSS 2022). Twin Waters, Australia 30 Nov - 02 Dec 2022New sensing technologies for citrus gall wasp and Fuller’s rose weevil: CT19009
Long, Derek, McCarthy, Alison and Mo, Jianhua. 2021. New sensing technologies for citrus gall wasp and Fuller’s rose weevil: CT19009. Australia. Hort Innovation Austarlia.Machine vision and automation technologies for the Australian cotton industry
McCarthy, Alison. 2019. "Machine vision and automation technologies for the Australian cotton industry." 78th International Cotton Advisory Committee Plenary Meeting (ICAC 2019). Brisbane, Australia 02 - 07 Dec 2019Machine vision App for automated cotton insect counting: initial development and first results
Long, Derek, Grundy, Paul and McCarthy, Alison. 2019. "Machine vision App for automated cotton insect counting: initial development and first results." Australian Association of Cotton Scientists 2019 Australian Cotton Research Conference (AACS 2019). Armidale, Australia 28 - 30 Oct 2019In-season yield prediction using VARIwise
McCarthy, Alison, O'Keeffe, Kieran and McKay, Andrew. 2019. "In-season yield prediction using VARIwise." Australian Association of Cotton Scientists 2019 Australian Cotton Research Conference (AACS 2019). Armidale, Australia 28 - 30 Oct 2019Smart automation of furrow irrigation in the sugar industry
Gillies, Malcolm, Attard, Steven, Jaramillo, Andres, Davis, Marian and Foley, Joseph. 2017. "Smart automation of furrow irrigation in the sugar industry." 39th Annual Conference of the Australian Society of Sugar Cane Technologists (ASSCT 2017). Cairns, Australia 03 - 05 May 2017 Mackay, Australia.Site-specific irrigation using automated control and machine vision for horticulture crops in Queensland and New Zealand
McCarthy, Alison, El-Naggar, Ahmed, Roudier, Pierre and Hedley, Carolyn. 2017. "Site-specific irrigation using automated control and machine vision for horticulture crops in Queensland and New Zealand." 18th Australian Agronomy Conference 2017. Ballarat, Victoria 24 - 28 Sep 2017 Australia.Automated cloud-based irrigation for centre pivot irrigated cotton and dairy pasture
McCarthy, Alison, Foley, Joseph, Hills, James, Shippam, Ralph and McLaren, David. 2018. "Automated cloud-based irrigation for centre pivot irrigated cotton and dairy pasture." 2018 Irrigation Australia International Conference and Exhibition. Sydney, Australia 13 - 15 Jun 2018Advanced data-driven irrigation
Hills, James, McLaren, David, Foley, Joseph and McCarthy, Alison. 2018. "Advanced data-driven irrigation." Where the Waters Meet 2018 - Australian Water Association, Tasmanian State Conference. Hobart, Australia 16 Aug 2018Aerial imagery for yield prediction
McCarthy, Alison and Foley, Joseph. 2018. "Aerial imagery for yield prediction." 2018 Australian Cotton Conference. Gold Coast, Australia 07 - 09 Aug 2018The role of forage management in addressing challenges facing Australasian dairy farming
Cranston, Lydia M., Pembleton, Keith G., Burkitt, Lucy L., Curtis, Andrew, Donaghy, Daniel J., Gourley, Cameron J. P., Harrington, Kerry C., Hills, James L., Pembleton, Luke W. and Rawnsley, Richard P.. 2019. "The role of forage management in addressing challenges facing Australasian dairy farming." Animal Production Science. 60 (1), pp. 26-35. https://doi.org/10.1071/AN18570Machine vision for camera-based horticulture crop growth monitoring
McCarthy, Alison, Hedley, Carolyn and El-Naggar, Ahmed. 2017. "Machine vision for camera-based horticulture crop growth monitoring." International Tri-Conference for Precision Agriculture in 2017 (PA17)/7th Asian-Australasian Conference on Precision Agriculture. Hamilton, New Zealand 16 - 18 Oct 2017 New Zealand. https://doi.org/10.5281/zenodo.893702Advancing automation in the agricultural working environment
Brett, P, McCarthy, A, Long, D, Gillies, M, Foley, J and Baillie, C. 2019. "Advancing automation in the agricultural working environment." Allsopp, P. (ed.) 41st Annual Conference of the Australian Society of Sugar Cane Technologists (ASSCT 2019). Toowoomba, Australia 30 Apr - 03 May 2019 Australia.Site-specific non-water-stressed and water-stressed baselines to calculate CWSI to schedule irrigation for furrow-irrigated sugarcane: a new approach
Rana, Vishal, Gillies, Malcolm H and McCarthy, Alison C. 2019. "Site-specific non-water-stressed and water-stressed baselines to calculate CWSI to schedule irrigation for furrow-irrigated sugarcane: a new approach." Allsopp, P. (ed.) 41st Annual Conference of the Australian Society of Sugar Cane Technologists (ASSCT 2019). Toowoomba, Australia 30 Apr - 03 May 2019 Australia.Hydraulic implications of variable-rate irrigation
Msibi, Trevor, Foley, Joseph and Gillies, Malcolm. 2019. "Hydraulic implications of variable-rate irrigation." 41st Annual Conference of the Australian Society of Sugar Cane Technologists (ASSCT 2019). Toowoomba, Australia 30 Apr - 03 May 2019 Australia.Cost-benefit analysis of automated furrow irrigation in the Burdekin
Gillies, Malcolm, Attard, Steven, Foley, Joseph and Davis, Marian. 2019. "Cost-benefit analysis of automated furrow irrigation in the Burdekin." Allsopp, P. (ed.) 41st Annual Conference of the Australian Society of Sugar Cane Technologists (ASSCT 2019). Toowoomba, Australia 30 Apr - 03 May 2019 Australia.Knowledge management, sensing and control tools for irrigated broadacre cropping
McCarthy, Alison, Scobie, Michael, Gillies, Malcolm, Agustina, Lidya and Foley, Joseph. 2016. "Knowledge management, sensing and control tools for irrigated broadacre cropping." 19th Symposium on Precision Agriculture in Australasia. Toowoomba, Australia 12 - 13 Sep 2016Automated camera-based crop monitoring and site-specific irrigation control systems for cotton, horticulture and dairy pasture
McCarthy, Alison. 2016. "Automated camera-based crop monitoring and site-specific irrigation control systems for cotton, horticulture and dairy pasture." The SPAA Expo: Talking precision in the Atherton Tableland (2016). Mareeba, AustraliaAutomated camera-based crop monitoring and site-specific irrigation control systems
McCarthy, Alison. 2016. "Automated camera-based crop monitoring and site-specific irrigation control systems." Precision Agriculture Technologies: Recent applications in the horticultural industry (2016). Gatton, Australia 24 Nov 2016Improving surface irrigation
Gillies, Malcolm H., Foley, Joseph P. and McCarthy, Alison C.. 2018. "Improving surface irrigation." Chen, Guangnan (ed.) Advances in agricultural machinery and technologies. United States. CRC Press. pp. 225-262Tools to aid autonomous irrigation
McCarthy, Alison. 2017. "Tools to aid autonomous irrigation." GVIA Grower-led Irrigation Research Field Day (2017). Moree, Australia 08 Feb 2017Modernisation of furrow irrigation in the sugar industry: final report 2014/079
Gillies, M., Attard, S. and Foley, J.. 2017. Modernisation of furrow irrigation in the sugar industry: final report 2014/079. Brisbane, Australia. Sugar Research Australia.Fixed camera system for phenology measurements during the 2018 field season
McCarthy, Alison and Raine, Steven. 2018. Fixed camera system for phenology measurements during the 2018 field season. United States. Monsanto.Smart Automated Broad-acre Irrigation (Smarter Irrigation for Profit RRDP1603)
Foley, J.P., Gillies, M.H. and McCarthy, A.C.. 2018. Smart Automated Broad-acre Irrigation (Smarter Irrigation for Profit RRDP1603). Australia. Cotton Research and Development Corporation.Sense-T: Sensor smart irrigation
McCarthy, Alison, Shippam, Ralph and Agustina, Lidya. 2017. Sense-T: Sensor smart irrigation. Australia. Sense-T.Automated camera-based crop and irrigation monitoring
McCarthy, Alison. 2016. "Automated camera-based crop and irrigation monitoring." 18th Australian Cotton Conference (2016). Gold Coast, Australia 02 - 04 Aug 2016Smarter irrigation for profit - increasing farm profit through efficient use of irrigation input to dairy pastures
Hills, James, McCarthy, Alison, Foley, Joseph and McLaren, David. 2016. "Smarter irrigation for profit - increasing farm profit through efficient use of irrigation input to dairy pastures." 7th Australian Dairy Science Symposium (2016). Sydney, Australia 16 - 18 Nov 2016 Australia.Improving horticulture irrigation and fertiliser application using real-time, adaptive control
McCarthy, Alison. 2017. Improving horticulture irrigation and fertiliser application using real-time, adaptive control. Australia. Queensland Government.Optimal irrigation of cotton via real-time, adaptive control
McCarthy, Alison, Smith, Rod and Hancock, Nigel. 2013. Optimal irrigation of cotton via real-time, adaptive control. Narrabri, Australia. Cotton Research and Development Corporation.Advancing VARIwise towards autonomous irrigation and a grower's guide
McCarthy, Alison, Antille, Diogenes, Skowronski, Victor, Zhao, Bo, Kodur, Shreevatsa, Nguyen-Ky, Tai and Raine, Steven. 2016. Advancing VARIwise towards autonomous irrigation and a grower's guide. Narrabri, Australia. Cotton Research and Development Corporation.A preliminary evaluation of using fixed cameras for phenology measurements in a maize and soybean crop 2016/17
McCarthy, Alison and Raine, Steven. 2017. A preliminary evaluation of using fixed cameras for phenology measurements in a maize and soybean crop 2016/17. United States. Monsanto.Remote monitoring and automatic detection of grain crop attributes
McCarthy, Alison, Tscharke, Matthew and Schmidt, Erik. 2014. Remote monitoring and automatic detection of grain crop attributes. Toowoomba, Australia. University of Southern Queensland.More milk from forage: Milk production, blood metabolites, and forage intake of dairy cows grazing pasture mixtures and spatially adjacent monocultures
Pembleton, Keith G., Hills, James L., Freeman, Mark J., McLaren, David K., French, Marion and Rawnsley, Richard P.. 2016. "More milk from forage: Milk production, blood metabolites, and forage intake of dairy cows grazing pasture mixtures and spatially adjacent monocultures." Journal of Dairy Science. 99 (5), pp. 3512-3528. https://doi.org/10.3168/jds.2015-10542Automated site-specific irrigation framework and evaluation
McCarthy, Alison. 2016. "Automated site-specific irrigation framework and evaluation." Irrigation Australia International Conference and Exhibition 2016: Irrigation - For Prosperity and Wellbeing. Melbourne, Australia 24 - 26 May 2016VARIwise: site-specific surface irrigation and fertigation using adaptive control
McCarthy, Alison. 2015. "VARIwise: site-specific surface irrigation and fertigation using adaptive control." 2015 Cotton Irrigation Technology Tour. Emerald & Moree & Nevertire, Australia 10 - 12 Feb 2015 Australia.Development of an integrated plant-based image sensing system for soil-water and nitrogen status estimation in cotton
McCarthy, Alison and Nguyen, Tai. 2015. Development of an integrated plant-based image sensing system for soil-water and nitrogen status estimation in cotton. Toowoomba, Australia. University of Southern Queensland.Control and sensing systems for automated site-specific irrigation
McCarthy, Alison. 2015. "Control and sensing systems for automated site-specific irrigation." CCA Cropping Solutions Seminar 2015. Moree, Australia 29 - 30 Jul 2015Automated site-specific control and sensing for irrigating dairy pastures
McCarthy, Alison. 2015. "Automated site-specific control and sensing for irrigating dairy pastures." Tasmanian Institute of Agriculture Dairy Centre Open Day 2015: Tips, Tools and Technologies to Better Manage Climate Variability. Burnie, Australia 02 Dec 2015Image analysis and artificial intelligence based approach for soil-water and nitrogen status estimation
McCarthy, Alison, Nguyen, Tai and Raine, Steven. 2015. "Image analysis and artificial intelligence based approach for soil-water and nitrogen status estimation." 2nd Australian Cotton Research Conference 2015: Science Securing Cotton's Future. Toowoomba, Australia 08 - 10 Sep 2015 Australia.Data requirements for automated model-based control of irrigation and fertiliser application
McCarthy, Alison and Raine, Steven. 2015. "Data requirements for automated model-based control of irrigation and fertiliser application." Acuna, T., Moeller, C., Parsons, D. and Harrison, M. (ed.) 17th Australian Agronomy Conference 2015: Building Productive, Diverse and Sustainable Landscapes (AAC 2015). Hobart, Australia 20 - 24 Sep 2015 Warragul, Australia.Estimation of wet canopy bulk stomatal resistance from energy flux measurements during sprinkler irrigation
Uddin, Jasim M., Smith, Rod J., Hancock, Nigel H. and Foley, Joseph P.. 2016. "Estimation of wet canopy bulk stomatal resistance from energy flux measurements during sprinkler irrigation." Biosystems Engineering. 143, pp. 61-67. https://doi.org/10.1016/j.biosystemseng.2015.12.017A new approach to estimate canopy evaporation and canopy interception capacity from evapotranspiration and sap flow measurements during and following wetting
Uddin, J., Foley, J. P., Smith, R. J. and Hancock, N. H.. 2016. "A new approach to estimate canopy evaporation and canopy interception capacity from evapotranspiration and sap flow measurements during and following wetting." Hydrological Processes. 30 (11), pp. 1757-1767. https://doi.org/10.1002/hyp.10739Micrometeorology of sprinkler irrigation
Hancock, N. H., Uddin, J. M., Smith, R. J. and Foley, J. P.. 2015. "Micrometeorology of sprinkler irrigation." Agricultural and Forest Meteorology. 200, pp. 293-301. https://doi.org/10.1016/j.agrformet.2014.10.010Are evaporation losses in sprinkler irrigation high?
Uddin, Jasim, Smith, Rod, Hancock, Nigel and Foley, Joseph. 2014. "Are evaporation losses in sprinkler irrigation high?" 17th Australian Cotton Conference (ACC 2014): Our Fibre, Our Focus, Our Future. Gold Coast, Australia 05 - 07 Aug 2014 Narrabri, Australia.Can advected energy affect the water use efficiency in sprinkler irrigation?
Uddin, Jasim, Smith, Rod, Hancock, Nigel and Foley, Joseph. 2014. "Can advected energy affect the water use efficiency in sprinkler irrigation? " Irrigation Australia Conference 2014: Water for Life, Future for All. Gold Coast, Australia 02 - 06 Jun 2014 Sydney, Australia.Estimation of canopy interception under sprinkler irrigation: a new approach
Uddin, Jasim, Smith, Rod, Hancock, Nigel and Foley, Joseph. 2014. "Estimation of canopy interception under sprinkler irrigation: a new approach." Irrigation Australia Conference 2014: Water for Life, Future for All. Gold Coast, Australia 02 - 06 Jun 2014 Sydney, Australia.Automated camera-based height and flower detection for wheat and chickpea
McCarthy, Alison and Tscharke, Matthew. 2014. "Automated camera-based height and flower detection for wheat and chickpea." 5th International Workshop on Applications of Computer Image Analysis and Spectroscopy in Agriculture (ASABE 2014). Montreal, Canada 12 - 13 Jul 2014 St. Joseph, MI. United States.Real-time irrigation decision-making and control for site-specific irrigation of cotton using a centre pivot, 2012/13
McCarthy, Alison, Smith, Rod and Hancock, Nigel. 2014. "Real-time irrigation decision-making and control for site-specific irrigation of cotton using a centre pivot, 2012/13." Irrigation Australia Conference 2014: Water for Life, Future for All. Gold Coast, Australia 02 - 06 Jun 2014 Sydney, Australia.VARIwise: towards autonomous irrigation and a grower's guide
McCarthy, A. C.. 2014. "VARIwise: towards autonomous irrigation and a grower's guide." IRRICOMM – communication of irrigation research in cotton (2014). Narrabri, Australia 11 - 12 Mar 2014 Narrabri, Australia.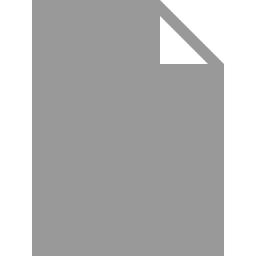