Digital strategies for nitrogen management in grain production systems: lessons from multi-method assessment using on-farm experimentation
Article
Article Title | Digital strategies for nitrogen management in grain production systems: lessons from multi-method assessment using on-farm experimentation |
---|---|
ERA Journal ID | 5325 |
Article Category | Article |
Authors | Colaco, A.F., Whelan, B.M., Bramley, R.G.V., Richetti, J., Fajardo, M., McCarthy, A.C., Perry, E.M., Bender, A., Leo, S., Fitzgerald, G.J. and Lawes, R.A. |
Journal Title | Precision Agriculture |
Journal Citation | 25 (2), pp. 983-1013 |
Number of Pages | 31 |
Year | 2024 |
Publisher | Springer |
Place of Publication | United States |
ISSN | 1385-2256 |
1573-1618 | |
Digital Object Identifier (DOI) | https://doi.org/10.1007/s11119-023-10102-z |
Web Address (URL) | https://link.springer.com/article/10.1007/s11119-023-10102-z |
Abstract | During the past few decades, a range of digital strategies for Nitrogen (N) management using various types of input data and recommendation frameworks have been developed. Despite much research, the benefits accrued from such technology have been equivocal. In this work, thirteen methods for mid-season N recommendations in cereal production systems were evaluated simultaneously, ranging from simple mass balance through to non-mechanistic approaches based on machine learning. To achieve this, an extensive field research program was implemented, comprising twenty-one N strip trials implemented in wheat and barley fields across Australia over four cropping seasons. A moving window regression approach was used to generate crop response functions to applied N and calculate economically optimal N rates along the length of the strips. The N recommendations made using various methods were assessed based on the error against the optimal rate and expected profitability. The root mean squared error of the recommendations ranged from 15 to 57 kg/ha. The best performing method was a data-driven empirical strategy in which a multivariate input to characterise field and season conditions was abundantly available and used to predict optimal N rates using machine learning. This was the only approach with potential to substantially outperform the existing farmer management, reducing the recommendation error from 42 to 15 kg/ha and improving profitability by up to A$47/ha. Despite being reliant on extensive historical databases, such a framework shows a promising pathway to drive production systems closer towards season- and site-specific economically optimum recommendations. Automated on-farm experimentation is a key enabler for building the necessary crop response databases to run empirical data-driven decision tools |
Keywords | Nitrogen decision tools, Crop sensing, Machine learning, Site-specific nutrient management, Digital agriculture |
Contains Sensitive Content | Does not contain sensitive content |
ANZSRC Field of Research 2020 | 3002. Agriculture, land and farm management |
4611. Machine learning | |
300204. Agricultural management of nutrients | |
401304. Photogrammetry and remote sensing | |
Byline Affiliations | Commonwealth Scientific and Industrial Research Organisation (CSIRO), Australia |
University of Sydney | |
Centre for Agricultural Engineering | |
Agriculture Victoria | |
Queensland University of Technology |
https://research.usq.edu.au/item/z3w0x/digital-strategies-for-nitrogen-management-in-grain-production-systems-lessons-from-multi-method-assessment-using-on-farm-experimentation
Download files
109
total views41
total downloads1
views this month5
downloads this month
Export as
Related outputs
Unlocking precision horticulture through machine learning-driven 3D canopy analysis
Banerjee, B.P., Scalisi, A., Valluri, N., Bonzi, L., O'Connell, M.G., Fitzgerald, G.J. and Goodwin, I.. 2024. "Unlocking precision horticulture through machine learning-driven 3D canopy analysis." O'Connell, M. (ed.) II International Symposium on Precision Management of Orchards and Vineyards. Tatura, Australia 03 - 08 Dec 2023 Australia. https://doi.org/10.17660/ActaHortic.2024.1395.13Artificial Intelligence: what is it and what does it mean for cotton?
McCarthy, Alison. 2023. "Artificial Intelligence: what is it and what does it mean for cotton?" 2023 Australian Cotton Research Conference. Toowoomba 05 - 07 Sep 2023 Australia.Variable-rate irrigation: hardware and software tools for improved infield irrigation
McCarthy, Alison and Foley, Joseph. 2023. "Variable-rate irrigation: hardware and software tools for improved infield irrigation." GRDC Grains Research Update: Irrigation efficiency. 30 - 30 May 2023 Australia.Research and industry engagement for Ag tech development
McCarthy, Alison. 2023. "Research and industry engagement for Ag tech development." Scenic Rim Agricultural Workshop. Aratula 22 - 22 Mar 2023 Australia.Field evaluation of automated site-specific irrigation for cotton and perennial ryegrass using soil-water sensors and Model Predictive Control
McCarthy, Alison, Foley, Joseph, Raedts, Pieter and Hills, James. 2023. "Field evaluation of automated site-specific irrigation for cotton and perennial ryegrass using soil-water sensors and Model Predictive Control." Agricultural Water Management. 277, pp. 1-17. https://doi.org/10.1016/j.agwat.2022.108098Potential for machine vision of grain crop features for nitrogen assessment
McCarthy, Alison, Colaco, Andre, Richetti, Jonathan and Baillie, Craig. 2022. "Potential for machine vision of grain crop features for nitrogen assessment." 20th Australian Agronomy Conference (2022). Toowoomba, Australia Toowoomba, Australia.Future farming: Machine vision for in-season nitrogen assessment of grain crops
McCarthy, Alison and Baillie, Craig. 2020. "Future farming: Machine vision for in-season nitrogen assessment of grain crops." 2020 Precision Agriculture Symposium Webinar. Virtual 30 Sep 2020 Sydney, Australia.Future farming: Machine vision for nitrogen assessment in grain crops
McCarthy, Alison and Baillie, Craig. 2020. "Future farming: Machine vision for nitrogen assessment in grain crops." GRDC Grains Research Update: Driving Profit Through Research. Goondiwindi, Australia 03 - 04 Mar 2020 Australia.Smartphone apps under development to aid pest monitoring
McCarthy, Alison, Long, Derek and Grundy, Paul. 2020. "Smartphone apps under development to aid pest monitoring." GRDC Grains Research Update: Driving Profit Through Research. Goondiwindi, Australia 03 - 04 Mar 2020 Australia.Detection of early infestations of Fall armyworm (FAW) in sweet corn and maize
McCarthy, Alison and Humpal, Jacob. 2021. Detection of early infestations of Fall armyworm (FAW) in sweet corn and maize. Toowoomba, Australia. University of Southern Queensland.Can additional N fertiliser ameliorate the elevated CO2-induced depression in grain and tissue N concentrations of wheat on a high soil N background?
Tausz, M., Norton, R. M., Tausz-Posch, S., Low, M., Seneweera, S., O'Leary, G., Armstrong, R. and Fitzgerald, G. J.. 2017. "Can additional N fertiliser ameliorate the elevated CO2-induced depression in grain and tissue N concentrations of wheat on a high soil N background?" Journal of Agronomy and Crop Science. 203 (6), pp. 574-583. https://doi.org/10.1111/jac.12209Automated variety trial plot growth and flowering detection for maize and soybean using machine vision
McCarthy, Alison and Raine, Steven. 2022. "Automated variety trial plot growth and flowering detection for maize and soybean using machine vision." Computers and Electronics in Agriculture. 194, pp. 1-21. https://doi.org/10.1016/j.compag.2022.106727Machine vision tools for Smarter Irrigation of dairy pasture and cotton
McCarthy, Alison, Foley, Joseph, Jamali, Hizbullah, Raedts, Pieter and Hills, James. 2022. "Machine vision tools for Smarter Irrigation of dairy pasture and cotton." 2022 Irrigation Australia Conference and Exhibition. Adelaide, Australia 05 - 07 Oct 2022 Australia.Estimating early season growth and biomass of field pea for selection of divergent ideotypes using proximal sensing
Tefera, Abeya Temesgen, Banerjee, Bikram Pratap, Pandey, Babu Ram, James, Laura, Puri, Ramesh Raj, Cooray, Onella, Marsh, Jasmine, Richards, Mark, Kant, Surya, Fitzgerald, Glenn J. and Cooray, Onella. 2022. "Estimating early season growth and biomass of field pea for selection of divergent ideotypes using proximal sensing." Field Crops Research. 277. https://doi.org/10.1016/j.fcr.2021.108407Smarter Irrigation for Profit 2 RRDP2002 Precise real-time automated cotton and dairy irrigation for improved water productivity
Foley, J., Gillies, M., McCarthy, A., Kelderman, S., Eberhard, J. and Shippam, R.. 2022. Smarter Irrigation for Profit 2 RRDP2002 Precise real-time automated cotton and dairy irrigation for improved water productivity. Narrabri, Australia.. Cotton Research and Development Corporation.Infield sensing of cotton insects using automated image analysis of crop symptoms: CSP2203
Heimoana, Simone, Blankley, S. and McCarthy, Alison. 2022. Infield sensing of cotton insects using automated image analysis of crop symptoms: CSP2203. Australia. Cotton Research and Development Corporation.Automated infield canopy temperature assessment of early stage cotton using thermal camera and plant segmentation
McCarthy, Alison, Nunn, Christopher, Jamali, Hizbullah and Long, Derek. 2022. Automated infield canopy temperature assessment of early stage cotton using thermal camera and plant segmentation . Australia. Cotton Research and Development Corporation.Cotton health assessment and yield prediction
McCarthy, Alison and Baillie, Craig. 2022. Cotton health assessment and yield prediction. United States. John Deere.Future Farm: Improving farmer confidence in targeted N management through automated sensing and decision support
Bramley, Rob, Whelan, Brett, Colaco, André, McCarthy, Alison, Richetti, Jonathan, Lawes, Roger, Fajardo, Mario, Bender, Asher, Pothula, Anand, Baillie, Craig, Perry, Eileen, Fitzgerald, Glenn, Clancy, Alex, Leo, Stephen and Grace, Peter. 2022. Future Farm: Improving farmer confidence in targeted N management through automated sensing and decision support. Australia. Grains Research and Development Corporation.Identifying sensors for better IPM in cotton: NEC1901
McCarthy, Alison, Long, Derek and Grundy, Paul. 2022. Identifying sensors for better IPM in cotton: NEC1901. Australia. Cotton Research and Development Corporation.Improving pasture growth assessment using machine vision
McCarthy, Alison, Raedts, Pieter, Foley, Joseph and Hills, James. 2022. "Improving pasture growth assessment using machine vision." Australasian Dairy Science Symposium 2022 (ADSS 2022). Twin Waters, Australia 30 Nov - 02 Dec 2022New sensing technologies for citrus gall wasp and Fuller’s rose weevil: CT19009
Long, Derek, McCarthy, Alison and Mo, Jianhua. 2021. New sensing technologies for citrus gall wasp and Fuller’s rose weevil: CT19009. Australia. Hort Innovation Austarlia.Machine vision and automation technologies for the Australian cotton industry
McCarthy, Alison. 2019. "Machine vision and automation technologies for the Australian cotton industry." 78th International Cotton Advisory Committee Plenary Meeting (ICAC 2019). Brisbane, Australia 02 - 07 Dec 2019Machine vision App for automated cotton insect counting: initial development and first results
Long, Derek, Grundy, Paul and McCarthy, Alison. 2019. "Machine vision App for automated cotton insect counting: initial development and first results." Australian Association of Cotton Scientists 2019 Australian Cotton Research Conference (AACS 2019). Armidale, Australia 28 - 30 Oct 2019In-season yield prediction using VARIwise
McCarthy, Alison, O'Keeffe, Kieran and McKay, Andrew. 2019. "In-season yield prediction using VARIwise." Australian Association of Cotton Scientists 2019 Australian Cotton Research Conference (AACS 2019). Armidale, Australia 28 - 30 Oct 2019Site-specific irrigation using automated control and machine vision for horticulture crops in Queensland and New Zealand
McCarthy, Alison, El-Naggar, Ahmed, Roudier, Pierre and Hedley, Carolyn. 2017. "Site-specific irrigation using automated control and machine vision for horticulture crops in Queensland and New Zealand." 18th Australian Agronomy Conference 2017. Ballarat, Victoria 24 - 28 Sep 2017 Australia.Automated cloud-based irrigation for centre pivot irrigated cotton and dairy pasture
McCarthy, Alison, Foley, Joseph, Hills, James, Shippam, Ralph and McLaren, David. 2018. "Automated cloud-based irrigation for centre pivot irrigated cotton and dairy pasture." 2018 Irrigation Australia International Conference and Exhibition. Sydney, Australia 13 - 15 Jun 2018Advanced data-driven irrigation
Hills, James, McLaren, David, Foley, Joseph and McCarthy, Alison. 2018. "Advanced data-driven irrigation." Where the Waters Meet 2018 - Australian Water Association, Tasmanian State Conference. Hobart, Australia 16 Aug 2018Aerial imagery for yield prediction
McCarthy, Alison and Foley, Joseph. 2018. "Aerial imagery for yield prediction." 2018 Australian Cotton Conference. Gold Coast, Australia 07 - 09 Aug 2018Machine vision for camera-based horticulture crop growth monitoring
McCarthy, Alison, Hedley, Carolyn and El-Naggar, Ahmed. 2017. "Machine vision for camera-based horticulture crop growth monitoring." International Tri-Conference for Precision Agriculture in 2017 (PA17)/7th Asian-Australasian Conference on Precision Agriculture. Hamilton, New Zealand 16 - 18 Oct 2017 New Zealand. https://doi.org/10.5281/zenodo.893702Impacts of elevated atmospheric CO2 on nutrient content of important food crops
Dietterich, Lee H., Zanobetti, Antonella, Kloog, Itai, Huybers, Peter, Leakey, Andrew D. B., Bloom, Arnold J., Carlisle, Eli, Fernando, Nimesha, Fitzgerald, Glenn, Hasegawa, Toshihiro, Holbrook, N. Michele, Nelson, Randall L., Norton, Robert, Ottman, Michael J., Raboy, Victor, Sakai, Hidemitsu, Sartor, Karla A., Schwartz, Joel, Seneweera, Saman, ..., Myers, Samuel S.. 2015. "Impacts of elevated atmospheric CO2 on nutrient content of important food crops." Scientific Data. 2, pp. 1-8. https://doi.org/10.1038/sdata.2015.36Advancing automation in the agricultural working environment
Brett, P, McCarthy, A, Long, D, Gillies, M, Foley, J and Baillie, C. 2019. "Advancing automation in the agricultural working environment." Allsopp, P. (ed.) 41st Annual Conference of the Australian Society of Sugar Cane Technologists (ASSCT 2019). Toowoomba, Australia 30 Apr - 03 May 2019 Australia.Site-specific non-water-stressed and water-stressed baselines to calculate CWSI to schedule irrigation for furrow-irrigated sugarcane: a new approach
Rana, Vishal, Gillies, Malcolm H and McCarthy, Alison C. 2019. "Site-specific non-water-stressed and water-stressed baselines to calculate CWSI to schedule irrigation for furrow-irrigated sugarcane: a new approach." Allsopp, P. (ed.) 41st Annual Conference of the Australian Society of Sugar Cane Technologists (ASSCT 2019). Toowoomba, Australia 30 Apr - 03 May 2019 Australia.Knowledge management, sensing and control tools for irrigated broadacre cropping
McCarthy, Alison, Scobie, Michael, Gillies, Malcolm, Agustina, Lidya and Foley, Joseph. 2016. "Knowledge management, sensing and control tools for irrigated broadacre cropping." 19th Symposium on Precision Agriculture in Australasia. Toowoomba, Australia 12 - 13 Sep 2016Automated camera-based crop monitoring and site-specific irrigation control systems for cotton, horticulture and dairy pasture
McCarthy, Alison. 2016. "Automated camera-based crop monitoring and site-specific irrigation control systems for cotton, horticulture and dairy pasture." The SPAA Expo: Talking precision in the Atherton Tableland (2016). Mareeba, AustraliaAutomated camera-based crop monitoring and site-specific irrigation control systems
McCarthy, Alison. 2016. "Automated camera-based crop monitoring and site-specific irrigation control systems." Precision Agriculture Technologies: Recent applications in the horticultural industry (2016). Gatton, Australia 24 Nov 2016Improving surface irrigation
Gillies, Malcolm H., Foley, Joseph P. and McCarthy, Alison C.. 2018. "Improving surface irrigation." Chen, Guangnan (ed.) Advances in agricultural machinery and technologies. United States. CRC Press. pp. 225-262Tools to aid autonomous irrigation
McCarthy, Alison. 2017. "Tools to aid autonomous irrigation." GVIA Grower-led Irrigation Research Field Day (2017). Moree, Australia 08 Feb 2017Fixed camera system for phenology measurements during the 2018 field season
McCarthy, Alison and Raine, Steven. 2018. Fixed camera system for phenology measurements during the 2018 field season. United States. Monsanto.Smart Automated Broad-acre Irrigation (Smarter Irrigation for Profit RRDP1603)
Foley, J.P., Gillies, M.H. and McCarthy, A.C.. 2018. Smart Automated Broad-acre Irrigation (Smarter Irrigation for Profit RRDP1603). Australia. Cotton Research and Development Corporation.Sense-T: Sensor smart irrigation
McCarthy, Alison, Shippam, Ralph and Agustina, Lidya. 2017. Sense-T: Sensor smart irrigation. Australia. Sense-T.Automated camera-based crop and irrigation monitoring
McCarthy, Alison. 2016. "Automated camera-based crop and irrigation monitoring." 18th Australian Cotton Conference (2016). Gold Coast, Australia 02 - 04 Aug 2016Smarter irrigation for profit - increasing farm profit through efficient use of irrigation input to dairy pastures
Hills, James, McCarthy, Alison, Foley, Joseph and McLaren, David. 2016. "Smarter irrigation for profit - increasing farm profit through efficient use of irrigation input to dairy pastures." 7th Australian Dairy Science Symposium (2016). Sydney, Australia 16 - 18 Nov 2016 Australia.Improving horticulture irrigation and fertiliser application using real-time, adaptive control
McCarthy, Alison. 2017. Improving horticulture irrigation and fertiliser application using real-time, adaptive control. Australia. Queensland Government.Optimal irrigation of cotton via real-time, adaptive control
McCarthy, Alison, Smith, Rod and Hancock, Nigel. 2013. Optimal irrigation of cotton via real-time, adaptive control. Narrabri, Australia. Cotton Research and Development Corporation.Advancing VARIwise towards autonomous irrigation and a grower's guide
McCarthy, Alison, Antille, Diogenes, Skowronski, Victor, Zhao, Bo, Kodur, Shreevatsa, Nguyen-Ky, Tai and Raine, Steven. 2016. Advancing VARIwise towards autonomous irrigation and a grower's guide. Narrabri, Australia. Cotton Research and Development Corporation.A preliminary evaluation of using fixed cameras for phenology measurements in a maize and soybean crop 2016/17
McCarthy, Alison and Raine, Steven. 2017. A preliminary evaluation of using fixed cameras for phenology measurements in a maize and soybean crop 2016/17. United States. Monsanto.Remote monitoring and automatic detection of grain crop attributes
McCarthy, Alison, Tscharke, Matthew and Schmidt, Erik. 2014. Remote monitoring and automatic detection of grain crop attributes. Toowoomba, Australia. University of Southern Queensland.Elevated carbon dioxide changes grain protein concentration and composition and compromises baking quality. A FACE study
Panozzo, J. F., Walker, C. K., Partington, D. L., Neumann, N. C., Tausz, M., Seneweera, S. and Fitzgerald, G. J.. 2014. "Elevated carbon dioxide changes grain protein concentration and composition and compromises baking quality. A FACE study." Journal of Cereal Science. 60 (3), pp. 461-470. https://doi.org/10.1016/j.jcs.2014.08.011Automated site-specific irrigation framework and evaluation
McCarthy, Alison. 2016. "Automated site-specific irrigation framework and evaluation." Irrigation Australia International Conference and Exhibition 2016: Irrigation - For Prosperity and Wellbeing. Melbourne, Australia 24 - 26 May 2016Elevated atmospheric [CO2] can dramatically increase wheat yields in semi-arid environments and buffer against heat waves
Fitzgerald, Glenn J., Tausz, Michael, O'Leary, Garry, Mollah, Mahabubur R., Tausz-Posch, Sabine, Seneweera, Saman, Mock, Ivan, Low, Markus, Partington, Debra L., McNeil, David and Norton, Robert M.. 2016. "Elevated atmospheric [CO2] can dramatically increase wheat yields in semi-arid environments and buffer against heat waves." Global Change Biology. 22 (6), pp. 2269-2284. https://doi.org/10.1111/gcb.13263VARIwise: site-specific surface irrigation and fertigation using adaptive control
McCarthy, Alison. 2015. "VARIwise: site-specific surface irrigation and fertigation using adaptive control." 2015 Cotton Irrigation Technology Tour. Emerald & Moree & Nevertire, Australia 10 - 12 Feb 2015 Australia.Development of an integrated plant-based image sensing system for soil-water and nitrogen status estimation in cotton
McCarthy, Alison and Nguyen, Tai. 2015. Development of an integrated plant-based image sensing system for soil-water and nitrogen status estimation in cotton. Toowoomba, Australia. University of Southern Queensland.Control and sensing systems for automated site-specific irrigation
McCarthy, Alison. 2015. "Control and sensing systems for automated site-specific irrigation." CCA Cropping Solutions Seminar 2015. Moree, Australia 29 - 30 Jul 2015Automated site-specific control and sensing for irrigating dairy pastures
McCarthy, Alison. 2015. "Automated site-specific control and sensing for irrigating dairy pastures." Tasmanian Institute of Agriculture Dairy Centre Open Day 2015: Tips, Tools and Technologies to Better Manage Climate Variability. Burnie, Australia 02 Dec 2015Image analysis and artificial intelligence based approach for soil-water and nitrogen status estimation
McCarthy, Alison, Nguyen, Tai and Raine, Steven. 2015. "Image analysis and artificial intelligence based approach for soil-water and nitrogen status estimation." 2nd Australian Cotton Research Conference 2015: Science Securing Cotton's Future. Toowoomba, Australia 08 - 10 Sep 2015 Australia.Data requirements for automated model-based control of irrigation and fertiliser application
McCarthy, Alison and Raine, Steven. 2015. "Data requirements for automated model-based control of irrigation and fertiliser application." Acuna, T., Moeller, C., Parsons, D. and Harrison, M. (ed.) 17th Australian Agronomy Conference 2015: Building Productive, Diverse and Sustainable Landscapes (AAC 2015). Hobart, Australia 20 - 24 Sep 2015 Warragul, Australia.Rising CO2 concentration altered wheat grain proteome and flour rheological characteristics
Fernando, Nimesha, Panozzo, Joe, Tausz, Michael, Norton, Robert, Fitzgerald, Glenn, Khan, Alamgir and Seneweera, Saman. 2015. "Rising CO2 concentration altered wheat grain proteome and flour rheological characteristics." Food Chemistry. 170, pp. 448-454. https://doi.org/10.1016/j.foodchem.2014.07.044Intraspecific variation in leaf growth of wheat (Triticum aestivum) under Australian Grain Free Air CO2 Enrichment (AGFACE): Is it regulated through carbon and/or nitrogen supply?
Thilakarathne, Chamindathee L., Tausz-Posch, Sabine, Cane, Karen, Norton, Robert M., Fitzgerald, Glenn J., Tausz, Michael and Seneweera, Saman. 2015. "Intraspecific variation in leaf growth of wheat (Triticum aestivum) under Australian Grain Free Air CO2 Enrichment (AGFACE): Is it regulated through carbon and/or nitrogen supply?" Functional Plant Biology: an international journal of plant function. 42 (3), pp. 299-308. https://doi.org/10.1071/FP14125Automated camera-based height and flower detection for wheat and chickpea
McCarthy, Alison and Tscharke, Matthew. 2014. "Automated camera-based height and flower detection for wheat and chickpea." 5th International Workshop on Applications of Computer Image Analysis and Spectroscopy in Agriculture (ASABE 2014). Montreal, Canada 12 - 13 Jul 2014 St. Joseph, MI. United States.Real-time irrigation decision-making and control for site-specific irrigation of cotton using a centre pivot, 2012/13
McCarthy, Alison, Smith, Rod and Hancock, Nigel. 2014. "Real-time irrigation decision-making and control for site-specific irrigation of cotton using a centre pivot, 2012/13." Irrigation Australia Conference 2014: Water for Life, Future for All. Gold Coast, Australia 02 - 06 Jun 2014 Sydney, Australia.VARIwise: towards autonomous irrigation and a grower's guide
McCarthy, A. C.. 2014. "VARIwise: towards autonomous irrigation and a grower's guide." IRRICOMM – communication of irrigation research in cotton (2014). Narrabri, Australia 11 - 12 Mar 2014 Narrabri, Australia.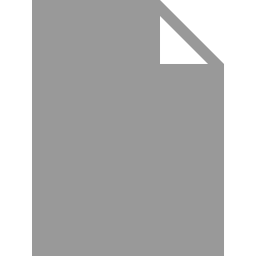