Novel analytical method for mix design and performance prediction of high calcium fly ash geopolymer concrete
Article
Article Title | Novel analytical method for mix design and performance |
---|---|
ERA Journal ID | 201391 |
Article Category | Article |
Authors | Gunasekara, Chamila (Author), Atzarakis, Peter (Author), Lokuge, Weena (Author), Law, David W. (Author) and Setunge, Sujeeva (Author) |
Journal Title | Polymers |
Journal Citation | 13 (6) |
Article Number | 900 |
Number of Pages | 21 |
Year | 2021 |
Publisher | MDPI AG |
Place of Publication | Basel, Switzerland |
ISSN | 2073-4360 |
Digital Object Identifier (DOI) | https://doi.org/10.3390/polym13060900 |
Web Address (URL) | https://www.mdpi.com/2073-4360/13/6/900 |
Abstract | Despite extensive in‐depth research into high calcium fly ash geopolymer concretes and a number of proposed methods to calculate the mix proportions, no universally applicable method to determine the mix proportions has been developed. This paper uses an artificial neural network (ANN) machine learning toolbox in a MATLAB programming environment together with a Bayes-ian regularization algorithm, the Levenberg‐Marquardt algorithm and a scaled conjugate gradient algorithm to attain a specified target compressive strength at 28 days. The relationship between the four key parameters, namely water/solid ratio, alkaline activator/binder ratio, Na2SiO3/NaOH ratio and NaOH molarity, and the compressive strength of geopolymer concrete is determined. The geo-polymer concrete mix proportions based on the ANN algorithm model and contour plots developed were experimentally validated. Thus, the proposed method can be used to determine mix designs for high calcium fly ash geopolymer concrete in the range 25–45 MPa at 28 days. In addition, the design equations developed using the statistical regression model provide an insight to predict tensile strength and elastic modulus for a given compressive strength. |
Keywords | high calcium fly ash; geopolymer concrete; artificial neural network; mix design; compressive strength; regression analysis |
ANZSRC Field of Research 2020 | 401602. Composite and hybrid materials |
400505. Construction materials | |
Public Notes | Copyright: © 2021 by the authors. Licensee MDPI, Basel, Switzerland. This article is an open access article |
Byline Affiliations | Royal Melbourne Institute of Technology (RMIT) |
School of Civil Engineering and Surveying | |
Institution of Origin | University of Southern Queensland |
https://research.usq.edu.au/item/q64yv/novel-analytical-method-for-mix-design-and-performance-prediction-of-high-calcium-fly-ash-geopolymer-concrete
Download files
133
total views94
total downloads0
views this month0
downloads this month
Export as
Related outputs
Bond performance of fly ash-based geopolymer mortar in simulated concrete sewer substrate
Ariyadasa, Piumika W., Manalo, Allan C., Lokuge, Weena, Aravinthan, Vasantha, Pasupathy, Kiru and Gerdes, Andreas. 2024. "Bond performance of fly ash-based geopolymer mortar in simulated concrete sewer substrate." Construction and Building Materials. 446. https://doi.org/10.1016/j.conbuildmat.2024.137927Concrete self-healing performance using surface roughness parameters: Metrological approach
Sidiq, Amir, Setunge, Sujeeva, Annamalai, Pratheep Kumar, Gravina, Rebecca J. and Giustozzi, Filippo. 2024. "Concrete self-healing performance using surface roughness parameters: Metrological approach." Journal of Building Engineering. 90. https://doi.org/10.1016/j.jobe.2024.109433Mix design determination procedure for geopolymer concrete based on target strength method
Rathnayaka, Madushan, Karunasinghe, Dulakshi, Gunasekara, Chamila, Law, David W., Wijesundara, Kushan and Lokuge, Weena. 2024. "Mix design determination procedure for geopolymer concrete based on target strength method." Archives of Civil and Mechanical Engineering. 24 (3). https://doi.org/10.1007/s43452-024-01002-8Macro and microstructural evolution of low-calcium fly ash-based geopolymer mortar exposed to sulphuric acid corrosion
Ariyadasa, Piumika W., Manalo, Allan C., Lokuge, Weena, Aravinthan, Vasantha, Gerdes, Andreas, Kaltenbach, Jonas and Galvan, Beatriz Arevalo. 2024. "Macro and microstructural evolution of low-calcium fly ash-based geopolymer mortar exposed to sulphuric acid corrosion." Cement and Concrete Research. 178. https://doi.org/10.1016/j.cemconres.2024.107436Machine learning approaches to predict compressive strength of fly ash-based geopolymer concrete: A comprehensive review
Rathnayaka, Madushan, Karunasinghe, Dulakshi, Gunasekara, Chamila, Wijesundara, Kushan, Lokuge, Weena and Law, David W.. 2024. "Machine learning approaches to predict compressive strength of fly ash-based geopolymer concrete: A comprehensive review." Construction and Building Materials. 149. https://doi.org/10.1016/j.conbuildmat.2024.135519Qualitative investigations into floodways under extreme flood loading
Greene, Isaac Samuel, Gunasekara, Chamila, Lokuge, Weena and Karunasena, Warna. 2024. "Qualitative investigations into floodways under extreme flood loading." Journal of Flood Risk Management. 17 (2). https://doi.org/10.1111/jfr3.12967A Reliability-Based Framework for Damage Accumulation Due to Multiple Earthquakes: A Case Study on Bridges
Herath, Nilupa, Zhang, Lihai, Mendis, Priyan, Navaratnam, Satheeskumar, Lokuge, Weena and Setunge, Sujeeva. 2023. "A Reliability-Based Framework for Damage Accumulation Due to Multiple Earthquakes: A Case Study on Bridges ." Infrastructures. 8 (6). https://doi.org/10.3390/infrastructures8060106Development of sustainable concrete using recycled polyethylene terephthalate (PET) granules as fine aggregate
Eyni Kangavar, Mohammad, Lokuge, Weena, Manalo, Allan, Karunasena, Warna and Ozbakkaloglu, Togay. 2023. "Development of sustainable concrete using recycled polyethylene terephthalate (PET) granules as fine aggregate ." Developments in the Built Environment. 15. https://doi.org/10.1016/j.dibe.2023.100192Using an inventory cluster approach for assessing bushfire preparedness and information needs in vulnerable communities
Ryan, Barbara, King, Rachel, Lokuge, Weena, Karunasena, Warna and Anderson, Esther. 2023. "Using an inventory cluster approach for assessing bushfire preparedness and information needs in vulnerable communities." Natural Hazards. 115, p. 1697–1714. https://doi.org/10.1007/s11069-022-05614-2Markov-based deterioration prediction and asset management of floodway structures
Tran, Huu, Lokuge, Weena, Karunasena, Warna and Setunge, Sujeeva. 2022. "Markov-based deterioration prediction and asset management of floodway structures." Sustainable and Resilient Infrastructure. 7 (6), pp. 789-802. https://doi.org/10.1080/23789689.2022.2067950Investigation on the properties of concrete with recycled polyethylene terephthalate (PET) granules as fine aggregate replacement
Eyni Kangavar, Mohammad, Lokuge, Weena, Manalo, Allan, Karunasena, Warna and Frigione, Mariaenrica. 2022. "Investigation on the properties of concrete with recycled polyethylene terephthalate (PET) granules as fine aggregate replacement." Case Studies in Construction Materials. 16, pp. 1-14. https://doi.org/10.1016/j.cscm.2022.e00934Network Deterioration Prediction for Reinforced Concrete Pipe and Box Culverts Using Markov Model: Case Study
Tran, Huu, Lokuge, Weena, Setunge, Sujeeva and Karunasena, Warna. 2022. "Network Deterioration Prediction for Reinforced Concrete Pipe and Box Culverts Using Markov Model: Case Study." Journal of Performance of Constructed Facilities. 36 (6), pp. 1-12. https://doi.org/10.1061/(ASCE)CF.1943-5509.0001766Flexural behaviour of circular reinforced concrete columns strengthened by glass fibre reinforced polymer wrapping system
Otoom, Omar F., Lokuge, Weena, Karunasena, Warna, Manalo, Allan C., Ozbakkaloglu, Togay and Ehsani, Mohammad R.. 2022. "Flexural behaviour of circular reinforced concrete columns strengthened by glass fibre reinforced polymer wrapping system." Structures. 38, pp. 1326-1348. https://doi.org/10.1016/j.istruc.2022.02.071Flexural behaviour of circular timber columns strengthened by glass fibre reinforced polymer wrapping system
Otoom, Omar F., Lokuge, Weena, Karunasena, Warna, Manalo, Allan C., Ozbakkaloglu, Togay and Ehsani, Mohammad R.. 2022. "Flexural behaviour of circular timber columns strengthened by glass fibre reinforced polymer wrapping system." Structures. 38, pp. 1349-1367. https://doi.org/10.1016/j.istruc.2022.02.070Mix design of fly ash based alkali activated concrete
Gunasekara, Chamila, Lokuge, Weena, Law, David W. and Setunge, Sujeeva. 2021. "Mix design of fly ash based alkali activated concrete." Pacheco-Torgal, Fernando, Chindaprasirt, Prinya and Ozbakkaloglu, Togay (ed.) Handbook of Advances in Alkali-activated Concrete. United Kingdom. Woodhead Publishing Limited. pp. 41-65Mechanical Properties of Macro Polypropylene Fibre-Reinforced Concrete
Abousnina, Rajab, Premasiri, Sachindra, Anise, Vilive, Lokuge, Weena, Vimonsatit, Vanissorn, Ferdous, Wahid and Alajarmeh, Omar. 2021. "Mechanical Properties of Macro Polypropylene Fibre-Reinforced Concrete." Polymers. 13 (23), pp. 1-26.Influence of Filler Properties on the Axial Behaviour of Pultruded FRP Tubes
Al-Saadi, Ali Umran, Aravinthan, Thiru and Lokuge, Weena. 2022. "Influence of Filler Properties on the Axial Behaviour of Pultruded FRP Tubes ." 10th International Conference on FRP Composites in Civil Engineering (CICE 2020/2021). Istanbul, Turkey 08 - 10 Dec 2021 Switzerland . Springer. https://doi.org/10.1007/978-3-030-88166-5_43Investigating snipe depth in girders and corbels in timber bridges
Cohen, Jethro, Lokuge, Weena and Herath, Nilupa. 2018. "Investigating snipe depth in girders and corbels in timber bridges." 9th International Conference on Bridge Maintenance, Safety and Management (IABMAS 2018). Melbourne, Australia 09 - 13 Jul 2018 United Kingdom.Recycling of landfill wastes (tyres, plastics and glass) in construction – A review on global waste generation, performance, application and future opportunities
Ferdous, Wahid, Manalo, Allan, Siddique, Rafat, Mendis, Priyan, Zhuge, Yan, Wong, Hong S., Lokuge, Weena, Aravinthan, Thiru and Schubel, Peter. 2021. "Recycling of landfill wastes (tyres, plastics and glass) in construction – A review on global waste generation, performance, application and future opportunities." Resources, Conservation and Recycling. 173, pp. 1-13. https://doi.org/10.1016/j.resconrec.2021.105745Experimental and numerical evaluation of the compression behaviour of GFRP-wrapped infill materials
Otoom, Omar F., Lokuge, Weena, Karunasena, Warna, Manalo, Allan C., Ozbakkaloglu, Togay and Thambiratnam, David. 2021. "Experimental and numerical evaluation of the compression behaviour of GFRP-wrapped infill materials." Case Studies in Construction Materials. 15. https://doi.org/10.1016/j.cscm.2021.e00654Experimental and numerical analysis on the effectiveness of GFRP wrapping system on timber pile rehabilitation
Lokuge, Weena, Otoom, Omar, Borzou, Rahmin, Navaratnam, Satheeskumar, Herath, Nilupa and Thambiratnam, David. 2021. "Experimental and numerical analysis on the effectiveness of GFRP wrapping system on timber pile rehabilitation." Case Studies in Construction Materials. 15. https://doi.org/10.1016/j.cscm.2021.e00552Bushfire mitigation and preparation on the Toowoomba Region Escarpment: a research report for Toowoomba Regional Council
Ryan, Barbara, King, Rachel, Lokuge, Weena, Karunasena, Karu and Anderson, Esther. 2020. Bushfire mitigation and preparation on the Toowoomba Region Escarpment: a research report for Toowoomba Regional Council. Toowoomba, Australia. University of Southern Queensland.Performance of timber girders with end-notch: Experimental and numerical investigation
Navaratnam, Satheeskumar, Herath, Nilupa, Lokuge, Weena, Thamboo, Julian and Poologanathan, Keerthan. 2021. "Performance of timber girders with end-notch: Experimental and numerical investigation." Structures. 29, pp. 730-740. https://doi.org/10.1016/j.istruc.2020.11.075Design of Alkali-Activated Slag-Fly Ash Concrete Mixtures Using Machine Learning
Gunasekara, C., Lokuge, W., Keskic, M., Raj, N., Law, D. W. and Setunge, S.. 2020. "Design of Alkali-Activated Slag-Fly Ash Concrete Mixtures Using Machine Learning." ACI Materials Journal. 117 (5), pp. 263-278. https://doi.org/10.14359/51727019Characteristics, strength development and microstructure of cement mortar containing oil-contaminated sand
Abousnina, Rajab, Manalo, Allan, Ferdous, Wahid, Lokuge, Weena, Benabed, Benchaa and Al-Jabri, Khalifa Saif. 2020. "Characteristics, strength development and microstructure of cement mortar containing oil-contaminated sand." Construction and Building Materials. 252, pp. 1-13. https://doi.org/10.1016/j.conbuildmat.2020.119155Performance of Bridges with Damaged Elements in Extreme Flood Events
Lokuge, W., Fraser, C. and Karunasena, W.. 2020. "Performance of Bridges with Damaged Elements in Extreme Flood Events." Wang, Chien Ming, Ho, Johnny C.M. and Kitipornchai, Sritawat (ed.) 25th Australasian Conference on Mechanics of Structures and Materials (ACMSM25). Brisbane, Australia 04 - 07 Dec 2018 Singapore. https://doi.org/10.1007/978-981-13-7603-0_40Numerical Investigation on Hollow Pultruded Fibre Reinforced Polymer Tube Columns
Al-Saadi, A.U., Aravinthan, T. and Lokuge, W.. 2020. "Numerical Investigation on Hollow Pultruded Fibre Reinforced Polymer Tube Columns." Wang, Chien Ming, Ho, Johnny C.M. and Kitipornchai, Sritawat (ed.) 25th Australasian Conference on Mechanics of Structures and Materials (ACMSM25). Brisbane, Australia 04 - 07 Dec 2018 Singapore. https://doi.org/10.1007/978-981-13-7603-0_45Floodway Design Process Revisted
Greene, I., Lokuge, W. and Karunasena, W.. 2020. "Floodway Design Process Revisted." Wang, Chien Ming, Ho, Johnny C.M. and Kitipornchai, Sritawat (ed.) 25th Australasian Conference on Mechanics of Structures and Materials (ACMSM25). Brisbane, Australia 04 - 07 Dec 2018 Singapore. https://doi.org/10.1007/978-981-13-7603-0_94Flexural Behavior of Functionally Graded-Graphene Reinforced Composite Plates
Rasappagari, M.R.R., Karunasena, W. and Lokuge, W.. 2020. "Flexural Behavior of Functionally Graded-Graphene Reinforced Composite Plates." Wang, Chen Ming, Ho, Johnny C.M. and Kitipornchai, Sritawat (ed.) 25th Australasian Conference on Mechanics of Structures and Materials (ACMSM25). Brisbane, Australia 04 - 07 Dec 2018 Singapore. https://doi.org/10.1007/978-981-13-7603-0_22Rehabilitation of timber bridge piles using a wrapping system
Borzou, R. and Lokuge, W.. 2018. "Rehabilitation of timber bridge piles using a wrapping system." Powers, Nigel, Frangopol, Dan M., Al-Mahaidi, Riadh and Caprani, Colin (ed.) 9th International Conference on Bridge Maintenance, Safety and Management (IABMAS 2018). Melbourne, Australia 09 - 13 Jul 2018 London, United Kingdom. https://doi.org/10.1201/9781315189390-281Structural design of floodways under extreme flood loading
Greene, Isaac, Lokuge, Weena and Karunasena, Warna. 2020. "Structural design of floodways under extreme flood loading." International Journal of Disaster Resilience in the Built Environment. 11 (4), pp. 535-555. https://doi.org/10.1108/IJDRBE-10-2019-0072Vulnerability assessment of bridges subjected to extreme cyclonic events
Pathiranage, Thilini and Lokuge, Weena. 2020. "Vulnerability assessment of bridges subjected to extreme cyclonic events." Natural Hazards. 102 (1), pp. 401-417. https://doi.org/10.1007/s11069-020-03931-yFlexural behaviour of concrete slabs reinforced with GFRP bars and hollow composite reinforcing systems
Al-Rubaye, Mohammed, Manalo, Allan, Alajarmeh, Omar, Ferdous, Wahid, Lokuge, Weena, Benmokrane, Brahim and Edoo, Azam. 2020. "Flexural behaviour of concrete slabs reinforced with GFRP bars and hollow composite reinforcing systems." Composite Structures. 236, pp. 1-12. https://doi.org/10.1016/j.compstruct.2019.111836Floodways and Flood-Related Experiences: A Survey of Industry Experts and Asset Owners
Greene, I., Lokuge, W. and Karunasena, K.. 2020. "Floodways and Flood-Related Experiences: A Survey of Industry Experts and Asset Owners." Engineering for Public Works. 19, pp. 69-75.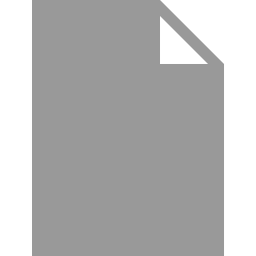