Enhancing Fly Ash-Based Geopolymer Concrete Performance through Machine Learning: A Predictive Model for Compressive Strength and Workability Optimization.
Paper
Paper/Presentation Title | Enhancing Fly Ash-Based Geopolymer Concrete Performance through Machine Learning: A Predictive Model for Compressive Strength and Workability Optimization. |
---|---|
Presentation Type | Paper |
Authors | Rathnayaka, Madushan, Wijesundara, Kushan, Gunasekara, Chamila, Law, David W, Karunasinghe, Dulakshi and Lokuge, Weena |
Journal or Proceedings Title | Proceedings of the Annual Conference of Japan Society of Material Cycles and Waste Management |
Number of Pages | 2 |
Year | 2024 |
Place of Publication | Australia |
Web Address (URL) of Paper | https://www.jstage.jst.go.jp/article/jsmcwm/t3rincs2024/0/t3rincs2024_43/_article/-char/ja/ |
Conference/Event | 3R International Scientific Conference on Material Cycles and Waste Management (3RINCs 2024) |
Event Details | 3R International Scientific Conference on Material Cycles and Waste Management (3RINCs 2024) Delivery In person Event Date 15 to end of 17 Mar 2024 Event Location Sydney, Australia Event Venue Hilton Hotel, Sydney Event Web Address (URL) |
Abstract | Geopolymer concrete (GPC) offers a promising solution to the problems associated with OPC-based concrete, such as greenhouse gas emissions and natural resource depletion, by utilizing waste materials, like fly ash, to replace the cement in concrete (Ahmed et al., 2020). However, the heterogeneous nature of the fly ash has complicated the development of mixed design and the implementation of GPC in industrial applications (Luan et al., 2021). This study focuses on developing a mix design procedure to achieve a target compressive strength and suitable workability using advanced computational modeling tools, namely machine learning algorithms, data clustering, and optimization. This approach capture the complexities of the aluminum silicate material and develop standard mix proportions, irrespective of the source of fly ash. |
Keywords | geopolymer cocnrete; fly ash; machine learning; compressive strength; workability |
Contains Sensitive Content | Does not contain sensitive content |
ANZSRC Field of Research 2020 | 400505. Construction materials |
Public Notes | Files associated with this item cannot be displayed due to copyright restrictions, but may be accessed online. Please see the link in the URL field. |
Byline Affiliations | Royal Melbourne Institute of Technology (RMIT) |
University of Peradeniya, Sri Lanka | |
School of Engineering |
https://research.usq.edu.au/item/zqxx3/enhancing-fly-ash-based-geopolymer-concrete-performance-through-machine-learning-a-predictive-model-for-compressive-strength-and-workability-optimization
79
total views1
total downloads6
views this month0
downloads this month
Export as
Related outputs
Systematic Analysis of the Impact of Data Preprocessing Techniques on Machine-Learning Model Performance: A Case Study of a Compressive Strength Prediction Model for Geopolymer Concrete
Rathnayaka, Madushan, Karunasinghe, Dulakshi, Gunasekara, Chamila, Wijesundara, Kushan, Law, David W. and Lokuge, Weena. 2025. "Systematic Analysis of the Impact of Data Preprocessing Techniques on Machine-Learning Model Performance: A Case Study of a Compressive Strength Prediction Model for Geopolymer Concrete." Journal of Computing in Civil Engineering. 39 (4). https://doi.org/10.1061/JCCEE5.CPENG-6547Performance of the corrugated metal pipe sliplining method using FRP pipes
Premasuriya, Aluth, Lokuge, Weena, Karunasena, Warna and Manalo, Allan C.. 2025. "Performance of the corrugated metal pipe sliplining method using FRP pipes ." Tunnelling and Underground Space Technology. 162. https://doi.org/10.1016/j.tust.2025.106603Degradation mechanisms of low-calcium fly ash-based geopolymer mortar in simulated aggressive sewer conditions
Ariyadasa, Piumika W., Manalo, Allan C., Lokuge, Weena, Aravinthan, Vasantha, Gerdes, Andreas and Kaltenbach, Jonas. 2025. "Degradation mechanisms of low-calcium fly ash-based geopolymer mortar in simulated aggressive sewer conditions." Cement and Concrete Composites. 194. https://doi.org/10.1016/j.cemconres.2025.107882Sustainable mortar with waste glass fine aggregates and pond ash as an alkali-silica reaction suppressor
Fernando, W.C.V., Lokuge, W., Wang, H., Gunasekara, C. and Dhasindrakrishna, K.. 2025. "Sustainable mortar with waste glass fine aggregates and pond ash as an alkali-silica reaction suppressor." Case Studies in Construction Materials. 22. https://doi.org/10.1016/j.cscm.2025.e04269Biochar in cementitious composites: A comprehensive review of properties, compatibility, and prospect of use in sustainable geopolymer concrete
Tayyab, Seemab, Ferdous, Wahid, Lokuge, Weena, Siddique, Rafat and Manalo, Allan. 2025. "Biochar in cementitious composites: A comprehensive review of properties, compatibility, and prospect of use in sustainable geopolymer concrete." Resources, Conservation and Recycling Advances. 25. https://doi.org/10.1016/j.rcradv.2024.200242Examining the Impact of Fly Ash in Glass Fine Aggregate Mortar
Fernando, W. C. V, Lokuge, W., Wang, H. and Gunasekara, C.. 2024. "Examining the Impact of Fly Ash in Glass Fine Aggregate Mortar." 3R International Scientific Conference on Material Cycles and Waste Management (3RINCs 2024). Sydney, Australia 15 - 17 Mar 2024 Australia.Bond performance of fly ash-based geopolymer mortar in simulated concrete sewer substrate
Ariyadasa, Piumika W., Manalo, Allan C., Lokuge, Weena, Aravinthan, Vasantha, Pasupathy, Kiru and Gerdes, Andreas. 2024. "Bond performance of fly ash-based geopolymer mortar in simulated concrete sewer substrate." Construction and Building Materials. 446. https://doi.org/10.1016/j.conbuildmat.2024.137927Mix design determination procedure for geopolymer concrete based on target strength method
Rathnayaka, Madushan, Karunasinghe, Dulakshi, Gunasekara, Chamila, Law, David W., Wijesundara, Kushan and Lokuge, Weena. 2024. "Mix design determination procedure for geopolymer concrete based on target strength method." Archives of Civil and Mechanical Engineering. 24 (3). https://doi.org/10.1007/s43452-024-01002-8Macro and microstructural evolution of low-calcium fly ash-based geopolymer mortar exposed to sulphuric acid corrosion
Ariyadasa, Piumika W., Manalo, Allan C., Lokuge, Weena, Aravinthan, Vasantha, Gerdes, Andreas, Kaltenbach, Jonas and Galvan, Beatriz Arevalo. 2024. "Macro and microstructural evolution of low-calcium fly ash-based geopolymer mortar exposed to sulphuric acid corrosion." Cement and Concrete Research. 178. https://doi.org/10.1016/j.cemconres.2024.107436Machine learning approaches to predict compressive strength of fly ash-based geopolymer concrete: A comprehensive review
Rathnayaka, Madushan, Karunasinghe, Dulakshi, Gunasekara, Chamila, Wijesundara, Kushan, Lokuge, Weena and Law, David W.. 2024. "Machine learning approaches to predict compressive strength of fly ash-based geopolymer concrete: A comprehensive review." Construction and Building Materials. 149. https://doi.org/10.1016/j.conbuildmat.2024.135519Qualitative investigations into floodways under extreme flood loading
Greene, Isaac Samuel, Gunasekara, Chamila, Lokuge, Weena and Karunasena, Warna. 2024. "Qualitative investigations into floodways under extreme flood loading." Journal of Flood Risk Management. 17 (2). https://doi.org/10.1111/jfr3.12967Opportunities in Australia to promote the beneficial use of coal combustion products in construction
Rex, Adrian, Kitnasamy, Dhasindrakrishna, Lokuge, Weena and Wang, Hao. 2023. "Opportunities in Australia to promote the beneficial use of coal combustion products in construction." Concrete Institute of Australia's Biennial National Conference 2023. Perth, Australia 10 - 13 Sep 2023 Australia.A Reliability-Based Framework for Damage Accumulation Due to Multiple Earthquakes: A Case Study on Bridges
Herath, Nilupa, Zhang, Lihai, Mendis, Priyan, Navaratnam, Satheeskumar, Lokuge, Weena and Setunge, Sujeeva. 2023. "A Reliability-Based Framework for Damage Accumulation Due to Multiple Earthquakes: A Case Study on Bridges ." Infrastructures. 8 (6). https://doi.org/10.3390/infrastructures8060106Development of sustainable concrete using recycled polyethylene terephthalate (PET) granules as fine aggregate
Eyni Kangavar, Mohammad, Lokuge, Weena, Manalo, Allan, Karunasena, Warna and Ozbakkaloglu, Togay. 2023. "Development of sustainable concrete using recycled polyethylene terephthalate (PET) granules as fine aggregate ." Developments in the Built Environment. 15. https://doi.org/10.1016/j.dibe.2023.100192Using an inventory cluster approach for assessing bushfire preparedness and information needs in vulnerable communities
Ryan, Barbara, King, Rachel, Lokuge, Weena, Karunasena, Warna and Anderson, Esther. 2023. "Using an inventory cluster approach for assessing bushfire preparedness and information needs in vulnerable communities." Natural Hazards. 115, p. 1697–1714. https://doi.org/10.1007/s11069-022-05614-2Markov-based deterioration prediction and asset management of floodway structures
Tran, Huu, Lokuge, Weena, Karunasena, Warna and Setunge, Sujeeva. 2022. "Markov-based deterioration prediction and asset management of floodway structures." Sustainable and Resilient Infrastructure. 7 (6), pp. 789-802. https://doi.org/10.1080/23789689.2022.2067950Flexural behaviour of circular timber columns strengthened by glass fibre reinforced polymer wrapping system
Otoom, Omar F., Lokuge, Weena, Karunasena, Warna, Manalo, Allan C., Ozbakkaloglu, Togay and Ehsani, Mohammad R.. 2022. "Flexural behaviour of circular timber columns strengthened by glass fibre reinforced polymer wrapping system." Structures. 38, pp. 1349-1367. https://doi.org/10.1016/j.istruc.2022.02.070Investigation on the properties of concrete with recycled polyethylene terephthalate (PET) granules as fine aggregate replacement
Eyni Kangavar, Mohammad, Lokuge, Weena, Manalo, Allan, Karunasena, Warna and Frigione, Mariaenrica. 2022. "Investigation on the properties of concrete with recycled polyethylene terephthalate (PET) granules as fine aggregate replacement." Case Studies in Construction Materials. 16, pp. 1-14. https://doi.org/10.1016/j.cscm.2022.e00934Network Deterioration Prediction for Reinforced Concrete Pipe and Box Culverts Using Markov Model: Case Study
Tran, Huu, Lokuge, Weena, Setunge, Sujeeva and Karunasena, Warna. 2022. "Network Deterioration Prediction for Reinforced Concrete Pipe and Box Culverts Using Markov Model: Case Study." Journal of Performance of Constructed Facilities. 36 (6), pp. 1-12. https://doi.org/10.1061/(ASCE)CF.1943-5509.0001766Flexural behaviour of circular reinforced concrete columns strengthened by glass fibre reinforced polymer wrapping system
Otoom, Omar F., Lokuge, Weena, Karunasena, Warna, Manalo, Allan C., Ozbakkaloglu, Togay and Ehsani, Mohammad R.. 2022. "Flexural behaviour of circular reinforced concrete columns strengthened by glass fibre reinforced polymer wrapping system." Structures. 38, pp. 1326-1348. https://doi.org/10.1016/j.istruc.2022.02.071Mix design of fly ash based alkali activated concrete
Gunasekara, Chamila, Lokuge, Weena, Law, David W. and Setunge, Sujeeva. 2021. "Mix design of fly ash based alkali activated concrete." Pacheco-Torgal, Fernando, Chindaprasirt, Prinya and Ozbakkaloglu, Togay (ed.) Handbook of Advances in Alkali-activated Concrete. United Kingdom. Woodhead Publishing Limited. pp. 41-65Mechanical Properties of Macro Polypropylene Fibre-Reinforced Concrete
Abousnina, Rajab, Premasiri, Sachindra, Anise, Vilive, Lokuge, Weena, Vimonsatit, Vanissorn, Ferdous, Wahid and Alajarmeh, Omar. 2021. "Mechanical Properties of Macro Polypropylene Fibre-Reinforced Concrete." Polymers. 13 (23), pp. 1-26.Influence of Filler Properties on the Axial Behaviour of Pultruded FRP Tubes
Al-Saadi, Ali Umran, Aravinthan, Thiru and Lokuge, Weena. 2022. "Influence of Filler Properties on the Axial Behaviour of Pultruded FRP Tubes ." 10th International Conference on FRP Composites in Civil Engineering (CICE 2020/2021). Istanbul, Turkey 08 - 10 Dec 2021 Switzerland . Springer. https://doi.org/10.1007/978-3-030-88166-5_43Investigating snipe depth in girders and corbels in timber bridges
Cohen, Jethro, Lokuge, Weena and Herath, Nilupa. 2018. "Investigating snipe depth in girders and corbels in timber bridges." 9th International Conference on Bridge Maintenance, Safety and Management (IABMAS 2018). Melbourne, Australia 09 - 13 Jul 2018 United Kingdom.Recycling of landfill wastes (tyres, plastics and glass) in construction – A review on global waste generation, performance, application and future opportunities
Ferdous, Wahid, Manalo, Allan, Siddique, Rafat, Mendis, Priyan, Zhuge, Yan, Wong, Hong S., Lokuge, Weena, Aravinthan, Thiru and Schubel, Peter. 2021. "Recycling of landfill wastes (tyres, plastics and glass) in construction – A review on global waste generation, performance, application and future opportunities." Resources, Conservation and Recycling. 173, pp. 1-13. https://doi.org/10.1016/j.resconrec.2021.105745Experimental and numerical evaluation of the compression behaviour of GFRP-wrapped infill materials
Otoom, Omar F., Lokuge, Weena, Karunasena, Warna, Manalo, Allan C., Ozbakkaloglu, Togay and Thambiratnam, David. 2021. "Experimental and numerical evaluation of the compression behaviour of GFRP-wrapped infill materials." Case Studies in Construction Materials. 15. https://doi.org/10.1016/j.cscm.2021.e00654Experimental and numerical analysis on the effectiveness of GFRP wrapping system on timber pile rehabilitation
Lokuge, Weena, Otoom, Omar, Borzou, Rahmin, Navaratnam, Satheeskumar, Herath, Nilupa and Thambiratnam, David. 2021. "Experimental and numerical analysis on the effectiveness of GFRP wrapping system on timber pile rehabilitation." Case Studies in Construction Materials. 15. https://doi.org/10.1016/j.cscm.2021.e00552Performance of timber girders with end-notch: Experimental and numerical investigation
Navaratnam, Satheeskumar, Herath, Nilupa, Lokuge, Weena, Thamboo, Julian and Poologanathan, Keerthan. 2021. "Performance of timber girders with end-notch: Experimental and numerical investigation." Structures. 29, pp. 730-740. https://doi.org/10.1016/j.istruc.2020.11.075Bushfire mitigation and preparation on the Toowoomba Region Escarpment: a research report for Toowoomba Regional Council
Ryan, Barbara, King, Rachel, Lokuge, Weena, Karunasena, Karu and Anderson, Esther. 2020. Bushfire mitigation and preparation on the Toowoomba Region Escarpment: a research report for Toowoomba Regional Council. Toowoomba, Australia. University of Southern Queensland.Novel analytical method for mix design and performance prediction of high calcium fly ash geopolymer concrete
Gunasekara, Chamila, Atzarakis, Peter, Lokuge, Weena, Law, David W. and Setunge, Sujeeva. 2021. "Novel analytical method for mix design and performance prediction of high calcium fly ash geopolymer concrete." Polymers. 13 (6). https://doi.org/10.3390/polym13060900Design of Alkali-Activated Slag-Fly Ash Concrete Mixtures Using Machine Learning
Gunasekara, C., Lokuge, W., Keskic, M., Raj, N., Law, D. W. and Setunge, S.. 2020. "Design of Alkali-Activated Slag-Fly Ash Concrete Mixtures Using Machine Learning." ACI Materials Journal. 117 (5), pp. 263-278. https://doi.org/10.14359/51727019Characteristics, strength development and microstructure of cement mortar containing oil-contaminated sand
Abousnina, Rajab, Manalo, Allan, Ferdous, Wahid, Lokuge, Weena, Benabed, Benchaa and Al-Jabri, Khalifa Saif. 2020. "Characteristics, strength development and microstructure of cement mortar containing oil-contaminated sand." Construction and Building Materials. 252, pp. 1-13. https://doi.org/10.1016/j.conbuildmat.2020.119155Performance of Bridges with Damaged Elements in Extreme Flood Events
Lokuge, W., Fraser, C. and Karunasena, W.. 2020. "Performance of Bridges with Damaged Elements in Extreme Flood Events." Wang, Chien Ming, Ho, Johnny C.M. and Kitipornchai, Sritawat (ed.) 25th Australasian Conference on Mechanics of Structures and Materials (ACMSM25). Brisbane, Australia 04 - 07 Dec 2018 Singapore. https://doi.org/10.1007/978-981-13-7603-0_40Numerical Investigation on Hollow Pultruded Fibre Reinforced Polymer Tube Columns
Al-Saadi, A.U., Aravinthan, T. and Lokuge, W.. 2020. "Numerical Investigation on Hollow Pultruded Fibre Reinforced Polymer Tube Columns." Wang, Chien Ming, Ho, Johnny C.M. and Kitipornchai, Sritawat (ed.) 25th Australasian Conference on Mechanics of Structures and Materials (ACMSM25). Brisbane, Australia 04 - 07 Dec 2018 Singapore. https://doi.org/10.1007/978-981-13-7603-0_45Floodway Design Process Revisted
Greene, I., Lokuge, W. and Karunasena, W.. 2020. "Floodway Design Process Revisted." Wang, Chien Ming, Ho, Johnny C.M. and Kitipornchai, Sritawat (ed.) 25th Australasian Conference on Mechanics of Structures and Materials (ACMSM25). Brisbane, Australia 04 - 07 Dec 2018 Singapore. https://doi.org/10.1007/978-981-13-7603-0_94Flexural Behavior of Functionally Graded-Graphene Reinforced Composite Plates
Rasappagari, M.R.R., Karunasena, W. and Lokuge, W.. 2020. "Flexural Behavior of Functionally Graded-Graphene Reinforced Composite Plates." Wang, Chen Ming, Ho, Johnny C.M. and Kitipornchai, Sritawat (ed.) 25th Australasian Conference on Mechanics of Structures and Materials (ACMSM25). Brisbane, Australia 04 - 07 Dec 2018 Singapore. https://doi.org/10.1007/978-981-13-7603-0_22Rehabilitation of timber bridge piles using a wrapping system
Borzou, R. and Lokuge, W.. 2018. "Rehabilitation of timber bridge piles using a wrapping system." Powers, Nigel, Frangopol, Dan M., Al-Mahaidi, Riadh and Caprani, Colin (ed.) 9th International Conference on Bridge Maintenance, Safety and Management (IABMAS 2018). Melbourne, Australia 09 - 13 Jul 2018 London, United Kingdom. https://doi.org/10.1201/9781315189390-281Structural design of floodways under extreme flood loading
Greene, Isaac, Lokuge, Weena and Karunasena, Warna. 2020. "Structural design of floodways under extreme flood loading." International Journal of Disaster Resilience in the Built Environment. 11 (4), pp. 535-555. https://doi.org/10.1108/IJDRBE-10-2019-0072Vulnerability assessment of bridges subjected to extreme cyclonic events
Pathiranage, Thilini and Lokuge, Weena. 2020. "Vulnerability assessment of bridges subjected to extreme cyclonic events." Natural Hazards. 102 (1), pp. 401-417. https://doi.org/10.1007/s11069-020-03931-yFlexural behaviour of concrete slabs reinforced with GFRP bars and hollow composite reinforcing systems
Al-Rubaye, Mohammed, Manalo, Allan, Alajarmeh, Omar, Ferdous, Wahid, Lokuge, Weena, Benmokrane, Brahim and Edoo, Azam. 2020. "Flexural behaviour of concrete slabs reinforced with GFRP bars and hollow composite reinforcing systems." Composite Structures. 236, pp. 1-12. https://doi.org/10.1016/j.compstruct.2019.111836Floodways and Flood-Related Experiences: A Survey of Industry Experts and Asset Owners
Greene, I., Lokuge, W. and Karunasena, K.. 2020. "Floodways and Flood-Related Experiences: A Survey of Industry Experts and Asset Owners." Engineering for Public Works. 19, pp. 69-75.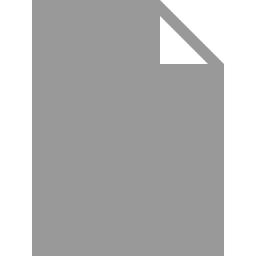