Machine learning approaches to predict compressive strength of fly ash-based geopolymer concrete: A comprehensive review
Article
Article Title | Machine learning approaches to predict compressive strength of fly ash-based geopolymer concrete: A comprehensive review |
---|---|
ERA Journal ID | 3470 |
Article Category | Article |
Authors | Rathnayaka, Madushan, Karunasinghe, Dulakshi, Gunasekara, Chamila, Wijesundara, Kushan, Lokuge, Weena and Law, David W. |
Journal Title | Construction and Building Materials |
Journal Citation | 149 |
Article Number | 135519 |
Number of Pages | 15 |
Year | 2024 |
Publisher | Elsevier |
Place of Publication | Netherlands |
ISSN | 0950-0618 |
Digital Object Identifier (DOI) | https://doi.org/10.1016/j.conbuildmat.2024.135519 |
Web Address (URL) | https://www.sciencedirect.com/science/article/pii/S0950061824006603 |
Abstract | Geopolymer concrete is a sustainable replacement to the Ordinary Portland Cement (OPC) concrete as it mitigates some of the associated problems of OPC manufacturing such as greenhouse gas emission and natural resource depletion. There has been significant recent research in the design of fly ash-based geopolymer concrete using advanced machine learning techniques which can address some of the problems with classical mix design approaches. However, practical application of geopolymer concrete is limited due to lack of standard mix design procedure. This comprehensive review summarizes the current literature on machine learning methodologies to predict the compressive strength of fly ash-based geopolymer concrete. Firstly, the input parameters used for the machine learning model development are categorized based on feature selection or feature extraction. Secondly, available machine learning approaches are categorized based on analysis methods namely, nonlinear regression, ensemble learning, and evolutionary programming. The effect of hyperparameters on the individual model performance, and model comparison based on the prediction performance are also discussed to identify potentially more suitable model type and hyper parameter ranges. Further, the paper discusses the input variable’s sensitivity towards the model performance which provides guidance towards future model developments. Overall, this paper will provide an understanding of the current state of machine learning approaches to predict the compressive strength of geopolymer concrete and the gaps in research for the development of models and achieving the required performance. Hence, the summarized knowledge will be highly beneficial to design prospective research towards sustainable cement-free concrete using fly ash. |
Keywords | Fly ash ; Geopolymer concrete ; Machine leaning ; Regression analysis ; Compressive strength |
Contains Sensitive Content | Does not contain sensitive content |
ANZSRC Field of Research 2020 | 400505. Construction materials |
401602. Composite and hybrid materials | |
Byline Affiliations | Royal Melbourne Institute of Technology (RMIT) |
University of Peradeniya, Sri Lanka | |
University of Southern Queensland |
https://research.usq.edu.au/item/z5x95/machine-learning-approaches-to-predict-compressive-strength-of-fly-ash-based-geopolymer-concrete-a-comprehensive-review
Download files
146
total views75
total downloads4
views this month1
downloads this month
Export as
Related outputs
Sustainable mortar with waste glass fine aggregates and pond ash as an alkali-silica reaction suppressor
Fernando, W.C.V., Lokuge, W., Wang, H., Gunasekara, C. and Dhasindrakrishna, K.. 2025. "Sustainable mortar with waste glass fine aggregates and pond ash as an alkali-silica reaction suppressor." Case Studies in Construction Materials. 22. https://doi.org/10.1016/j.cscm.2025.e04269Biochar in cementitious composites: A comprehensive review of properties, compatibility, and prospect of use in sustainable geopolymer concrete
Tayyab, Seemab, Ferdous, Wahid, Lokuge, Weena, Siddique, Rafat and Manalo, Allan. 2025. "Biochar in cementitious composites: A comprehensive review of properties, compatibility, and prospect of use in sustainable geopolymer concrete." Resources, Conservation and Recycling Advances. 25. https://doi.org/10.1016/j.rcradv.2024.200242Examining the Impact of Fly Ash in Glass Fine Aggregate Mortar
Fernando, W. C. V, Lokuge, W., Wang, H. and Gunasekara, C.. 2024. "Examining the Impact of Fly Ash in Glass Fine Aggregate Mortar." 3R International Scientific Conference on Material Cycles and Waste Management (3RINCs 2024). Sydney, Australia 15 - 17 Mar 2024 Australia.Enhancing Fly Ash-Based Geopolymer Concrete Performance through Machine Learning: A Predictive Model for Compressive Strength and Workability Optimization.
Rathnayaka, Madushan, Wijesundara, Kushan, Gunasekara, Chamila, Law, David W, Karunasinghe, Dulakshi and Lokuge, Weena. 2024. "Enhancing Fly Ash-Based Geopolymer Concrete Performance through Machine Learning: A Predictive Model for Compressive Strength and Workability Optimization." 3R International Scientific Conference on Material Cycles and Waste Management (3RINCs 2024). Sydney, Australia 15 - 17 Mar 2024 Australia.Bond performance of fly ash-based geopolymer mortar in simulated concrete sewer substrate
Ariyadasa, Piumika W., Manalo, Allan C., Lokuge, Weena, Aravinthan, Vasantha, Pasupathy, Kiru and Gerdes, Andreas. 2024. "Bond performance of fly ash-based geopolymer mortar in simulated concrete sewer substrate." Construction and Building Materials. 446. https://doi.org/10.1016/j.conbuildmat.2024.137927Mix design determination procedure for geopolymer concrete based on target strength method
Rathnayaka, Madushan, Karunasinghe, Dulakshi, Gunasekara, Chamila, Law, David W., Wijesundara, Kushan and Lokuge, Weena. 2024. "Mix design determination procedure for geopolymer concrete based on target strength method." Archives of Civil and Mechanical Engineering. 24 (3). https://doi.org/10.1007/s43452-024-01002-8Macro and microstructural evolution of low-calcium fly ash-based geopolymer mortar exposed to sulphuric acid corrosion
Ariyadasa, Piumika W., Manalo, Allan C., Lokuge, Weena, Aravinthan, Vasantha, Gerdes, Andreas, Kaltenbach, Jonas and Galvan, Beatriz Arevalo. 2024. "Macro and microstructural evolution of low-calcium fly ash-based geopolymer mortar exposed to sulphuric acid corrosion." Cement and Concrete Research. 178. https://doi.org/10.1016/j.cemconres.2024.107436Qualitative investigations into floodways under extreme flood loading
Greene, Isaac Samuel, Gunasekara, Chamila, Lokuge, Weena and Karunasena, Warna. 2024. "Qualitative investigations into floodways under extreme flood loading." Journal of Flood Risk Management. 17 (2). https://doi.org/10.1111/jfr3.12967Opportunities in Australia to promote the beneficial use of coal combustion products in construction
Rex, Adrian, Kitnasamy, Dhasindrakrishna, Lokuge, Weena and Wang, Hao. 2023. "Opportunities in Australia to promote the beneficial use of coal combustion products in construction." Concrete Institute of Australia's Biennial National Conference 2023. Perth, Australia 10 - 13 Sep 2023 Australia.A Reliability-Based Framework for Damage Accumulation Due to Multiple Earthquakes: A Case Study on Bridges
Herath, Nilupa, Zhang, Lihai, Mendis, Priyan, Navaratnam, Satheeskumar, Lokuge, Weena and Setunge, Sujeeva. 2023. "A Reliability-Based Framework for Damage Accumulation Due to Multiple Earthquakes: A Case Study on Bridges ." Infrastructures. 8 (6). https://doi.org/10.3390/infrastructures8060106Development of sustainable concrete using recycled polyethylene terephthalate (PET) granules as fine aggregate
Eyni Kangavar, Mohammad, Lokuge, Weena, Manalo, Allan, Karunasena, Warna and Ozbakkaloglu, Togay. 2023. "Development of sustainable concrete using recycled polyethylene terephthalate (PET) granules as fine aggregate ." Developments in the Built Environment. 15. https://doi.org/10.1016/j.dibe.2023.100192Using an inventory cluster approach for assessing bushfire preparedness and information needs in vulnerable communities
Ryan, Barbara, King, Rachel, Lokuge, Weena, Karunasena, Warna and Anderson, Esther. 2023. "Using an inventory cluster approach for assessing bushfire preparedness and information needs in vulnerable communities." Natural Hazards. 115, p. 1697–1714. https://doi.org/10.1007/s11069-022-05614-2Markov-based deterioration prediction and asset management of floodway structures
Tran, Huu, Lokuge, Weena, Karunasena, Warna and Setunge, Sujeeva. 2022. "Markov-based deterioration prediction and asset management of floodway structures." Sustainable and Resilient Infrastructure. 7 (6), pp. 789-802. https://doi.org/10.1080/23789689.2022.2067950Investigation on the properties of concrete with recycled polyethylene terephthalate (PET) granules as fine aggregate replacement
Eyni Kangavar, Mohammad, Lokuge, Weena, Manalo, Allan, Karunasena, Warna and Frigione, Mariaenrica. 2022. "Investigation on the properties of concrete with recycled polyethylene terephthalate (PET) granules as fine aggregate replacement." Case Studies in Construction Materials. 16, pp. 1-14. https://doi.org/10.1016/j.cscm.2022.e00934Network Deterioration Prediction for Reinforced Concrete Pipe and Box Culverts Using Markov Model: Case Study
Tran, Huu, Lokuge, Weena, Setunge, Sujeeva and Karunasena, Warna. 2022. "Network Deterioration Prediction for Reinforced Concrete Pipe and Box Culverts Using Markov Model: Case Study." Journal of Performance of Constructed Facilities. 36 (6), pp. 1-12. https://doi.org/10.1061/(ASCE)CF.1943-5509.0001766Flexural behaviour of circular reinforced concrete columns strengthened by glass fibre reinforced polymer wrapping system
Otoom, Omar F., Lokuge, Weena, Karunasena, Warna, Manalo, Allan C., Ozbakkaloglu, Togay and Ehsani, Mohammad R.. 2022. "Flexural behaviour of circular reinforced concrete columns strengthened by glass fibre reinforced polymer wrapping system." Structures. 38, pp. 1326-1348. https://doi.org/10.1016/j.istruc.2022.02.071Flexural behaviour of circular timber columns strengthened by glass fibre reinforced polymer wrapping system
Otoom, Omar F., Lokuge, Weena, Karunasena, Warna, Manalo, Allan C., Ozbakkaloglu, Togay and Ehsani, Mohammad R.. 2022. "Flexural behaviour of circular timber columns strengthened by glass fibre reinforced polymer wrapping system." Structures. 38, pp. 1349-1367. https://doi.org/10.1016/j.istruc.2022.02.070Mix design of fly ash based alkali activated concrete
Gunasekara, Chamila, Lokuge, Weena, Law, David W. and Setunge, Sujeeva. 2021. "Mix design of fly ash based alkali activated concrete." Pacheco-Torgal, Fernando, Chindaprasirt, Prinya and Ozbakkaloglu, Togay (ed.) Handbook of Advances in Alkali-activated Concrete. United Kingdom. Woodhead Publishing Limited. pp. 41-65Mechanical Properties of Macro Polypropylene Fibre-Reinforced Concrete
Abousnina, Rajab, Premasiri, Sachindra, Anise, Vilive, Lokuge, Weena, Vimonsatit, Vanissorn, Ferdous, Wahid and Alajarmeh, Omar. 2021. "Mechanical Properties of Macro Polypropylene Fibre-Reinforced Concrete." Polymers. 13 (23), pp. 1-26.Influence of Filler Properties on the Axial Behaviour of Pultruded FRP Tubes
Al-Saadi, Ali Umran, Aravinthan, Thiru and Lokuge, Weena. 2022. "Influence of Filler Properties on the Axial Behaviour of Pultruded FRP Tubes ." 10th International Conference on FRP Composites in Civil Engineering (CICE 2020/2021). Istanbul, Turkey 08 - 10 Dec 2021 Switzerland . Springer. https://doi.org/10.1007/978-3-030-88166-5_43Investigating snipe depth in girders and corbels in timber bridges
Cohen, Jethro, Lokuge, Weena and Herath, Nilupa. 2018. "Investigating snipe depth in girders and corbels in timber bridges." 9th International Conference on Bridge Maintenance, Safety and Management (IABMAS 2018). Melbourne, Australia 09 - 13 Jul 2018 United Kingdom.Recycling of landfill wastes (tyres, plastics and glass) in construction – A review on global waste generation, performance, application and future opportunities
Ferdous, Wahid, Manalo, Allan, Siddique, Rafat, Mendis, Priyan, Zhuge, Yan, Wong, Hong S., Lokuge, Weena, Aravinthan, Thiru and Schubel, Peter. 2021. "Recycling of landfill wastes (tyres, plastics and glass) in construction – A review on global waste generation, performance, application and future opportunities." Resources, Conservation and Recycling. 173, pp. 1-13. https://doi.org/10.1016/j.resconrec.2021.105745Experimental and numerical evaluation of the compression behaviour of GFRP-wrapped infill materials
Otoom, Omar F., Lokuge, Weena, Karunasena, Warna, Manalo, Allan C., Ozbakkaloglu, Togay and Thambiratnam, David. 2021. "Experimental and numerical evaluation of the compression behaviour of GFRP-wrapped infill materials." Case Studies in Construction Materials. 15. https://doi.org/10.1016/j.cscm.2021.e00654Experimental and numerical analysis on the effectiveness of GFRP wrapping system on timber pile rehabilitation
Lokuge, Weena, Otoom, Omar, Borzou, Rahmin, Navaratnam, Satheeskumar, Herath, Nilupa and Thambiratnam, David. 2021. "Experimental and numerical analysis on the effectiveness of GFRP wrapping system on timber pile rehabilitation." Case Studies in Construction Materials. 15. https://doi.org/10.1016/j.cscm.2021.e00552Bushfire mitigation and preparation on the Toowoomba Region Escarpment: a research report for Toowoomba Regional Council
Ryan, Barbara, King, Rachel, Lokuge, Weena, Karunasena, Karu and Anderson, Esther. 2020. Bushfire mitigation and preparation on the Toowoomba Region Escarpment: a research report for Toowoomba Regional Council. Toowoomba, Australia. University of Southern Queensland.Novel analytical method for mix design and performance prediction of high calcium fly ash geopolymer concrete
Gunasekara, Chamila, Atzarakis, Peter, Lokuge, Weena, Law, David W. and Setunge, Sujeeva. 2021. "Novel analytical method for mix design and performance prediction of high calcium fly ash geopolymer concrete." Polymers. 13 (6). https://doi.org/10.3390/polym13060900Performance of timber girders with end-notch: Experimental and numerical investigation
Navaratnam, Satheeskumar, Herath, Nilupa, Lokuge, Weena, Thamboo, Julian and Poologanathan, Keerthan. 2021. "Performance of timber girders with end-notch: Experimental and numerical investigation." Structures. 29, pp. 730-740. https://doi.org/10.1016/j.istruc.2020.11.075Design of Alkali-Activated Slag-Fly Ash Concrete Mixtures Using Machine Learning
Gunasekara, C., Lokuge, W., Keskic, M., Raj, N., Law, D. W. and Setunge, S.. 2020. "Design of Alkali-Activated Slag-Fly Ash Concrete Mixtures Using Machine Learning." ACI Materials Journal. 117 (5), pp. 263-278. https://doi.org/10.14359/51727019Characteristics, strength development and microstructure of cement mortar containing oil-contaminated sand
Abousnina, Rajab, Manalo, Allan, Ferdous, Wahid, Lokuge, Weena, Benabed, Benchaa and Al-Jabri, Khalifa Saif. 2020. "Characteristics, strength development and microstructure of cement mortar containing oil-contaminated sand." Construction and Building Materials. 252, pp. 1-13. https://doi.org/10.1016/j.conbuildmat.2020.119155Performance of Bridges with Damaged Elements in Extreme Flood Events
Lokuge, W., Fraser, C. and Karunasena, W.. 2020. "Performance of Bridges with Damaged Elements in Extreme Flood Events." Wang, Chien Ming, Ho, Johnny C.M. and Kitipornchai, Sritawat (ed.) 25th Australasian Conference on Mechanics of Structures and Materials (ACMSM25). Brisbane, Australia 04 - 07 Dec 2018 Singapore. https://doi.org/10.1007/978-981-13-7603-0_40Numerical Investigation on Hollow Pultruded Fibre Reinforced Polymer Tube Columns
Al-Saadi, A.U., Aravinthan, T. and Lokuge, W.. 2020. "Numerical Investigation on Hollow Pultruded Fibre Reinforced Polymer Tube Columns." Wang, Chien Ming, Ho, Johnny C.M. and Kitipornchai, Sritawat (ed.) 25th Australasian Conference on Mechanics of Structures and Materials (ACMSM25). Brisbane, Australia 04 - 07 Dec 2018 Singapore. https://doi.org/10.1007/978-981-13-7603-0_45Floodway Design Process Revisted
Greene, I., Lokuge, W. and Karunasena, W.. 2020. "Floodway Design Process Revisted." Wang, Chien Ming, Ho, Johnny C.M. and Kitipornchai, Sritawat (ed.) 25th Australasian Conference on Mechanics of Structures and Materials (ACMSM25). Brisbane, Australia 04 - 07 Dec 2018 Singapore. https://doi.org/10.1007/978-981-13-7603-0_94Flexural Behavior of Functionally Graded-Graphene Reinforced Composite Plates
Rasappagari, M.R.R., Karunasena, W. and Lokuge, W.. 2020. "Flexural Behavior of Functionally Graded-Graphene Reinforced Composite Plates." Wang, Chen Ming, Ho, Johnny C.M. and Kitipornchai, Sritawat (ed.) 25th Australasian Conference on Mechanics of Structures and Materials (ACMSM25). Brisbane, Australia 04 - 07 Dec 2018 Singapore. https://doi.org/10.1007/978-981-13-7603-0_22Rehabilitation of timber bridge piles using a wrapping system
Borzou, R. and Lokuge, W.. 2018. "Rehabilitation of timber bridge piles using a wrapping system." Powers, Nigel, Frangopol, Dan M., Al-Mahaidi, Riadh and Caprani, Colin (ed.) 9th International Conference on Bridge Maintenance, Safety and Management (IABMAS 2018). Melbourne, Australia 09 - 13 Jul 2018 London, United Kingdom. https://doi.org/10.1201/9781315189390-281Structural design of floodways under extreme flood loading
Greene, Isaac, Lokuge, Weena and Karunasena, Warna. 2020. "Structural design of floodways under extreme flood loading." International Journal of Disaster Resilience in the Built Environment. 11 (4), pp. 535-555. https://doi.org/10.1108/IJDRBE-10-2019-0072Vulnerability assessment of bridges subjected to extreme cyclonic events
Pathiranage, Thilini and Lokuge, Weena. 2020. "Vulnerability assessment of bridges subjected to extreme cyclonic events." Natural Hazards. 102 (1), pp. 401-417. https://doi.org/10.1007/s11069-020-03931-yFlexural behaviour of concrete slabs reinforced with GFRP bars and hollow composite reinforcing systems
Al-Rubaye, Mohammed, Manalo, Allan, Alajarmeh, Omar, Ferdous, Wahid, Lokuge, Weena, Benmokrane, Brahim and Edoo, Azam. 2020. "Flexural behaviour of concrete slabs reinforced with GFRP bars and hollow composite reinforcing systems." Composite Structures. 236, pp. 1-12. https://doi.org/10.1016/j.compstruct.2019.111836Floodways and Flood-Related Experiences: A Survey of Industry Experts and Asset Owners
Greene, I., Lokuge, W. and Karunasena, K.. 2020. "Floodways and Flood-Related Experiences: A Survey of Industry Experts and Asset Owners." Engineering for Public Works. 19, pp. 69-75.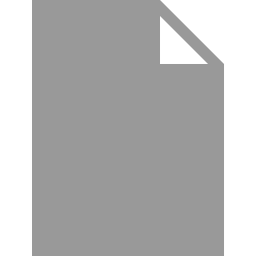