Predicting the pyrite oxidation process within coal waste piles using multiple linear regression (MLR) and teaching-learning-based optimization (TLBO) algorithm
Paper
Paper/Presentation Title | Predicting the pyrite oxidation process within coal waste piles using multiple linear regression (MLR) and teaching-learning-based optimization (TLBO) algorithm |
---|---|
Presentation Type | Paper |
Authors | Entezam, Shima (Author), Shokri, Behshad Jodeiri (Author), Ardejani, Shaghayesh Doulati (Author), Mirzaghorbanali, Ali (Author), McDougall, Kevin (Author) and Aziz, Naj (Author) |
Editors | Aziz, Naj and Mirzaghorbanali, Ali |
Journal or Proceedings Title | Proceedings of the 2022 Resource Operators Conference |
Number of Pages | 8 |
Year | 2022 |
Place of Publication | Australia |
ISBN | 9781741283532 |
9781741283556 | |
Web Address (URL) of Paper | https://ro.uow.edu.au/coal/849/ |
Web Address (URL) of Conference Proceedings | https://ro.uow.edu.au/coal2022/ |
Conference/Event | 2022 Resource Operators Conference (ROC2022) |
Event Details | 2022 Resource Operators Conference (ROC2022) Event Date Feb 2022 Event Location Australia Event Venue University of Wollongong University of Southern Queensland |
Abstract | Coal mining often leads to significant environmental hazards and health concerns when sulfide minerals, particularly pyrite, are associated with coal waste. The oxidation of pyrite typically generates acid mine drainage, a significant problem. This paper presents two mathematical relationships using a teaching-learning-based optimization (TLBO) algorithm for predicting pyrite oxidation and pH changes within a coal waste pile from Alborz-Markazi in northern Iran. A dataset was built based on historical data to achieve this goal. Some influential parameters comprising the depths of the various samples, oxygen fraction, and bicarbonate concentrations were considered as input data, while the outputs were pyrite content and pH. Then, the best statistical relationships were suggested between input and output parameters employing curve and surface fitting methods. Afterward, two multiple linear regression (MLR) models were presented for predicting pyrite content and pH. Also, two relationships have been suggested for predicting the same outputs by applying the TLBO algorithm. Comparison of the results of the latter method with the results obtained using the statistical technique, including correlation coefficient and root mean squared error (RMSE), revealed that the TLBO could predict the outcomes better than the MLR. |
Keywords | pyrite oxidation process; coal waste piles |
ANZSRC Field of Research 2020 | 400502. Civil geotechnical engineering |
401902. Geomechanics and resources geotechnical engineering | |
401905. Mining engineering | |
Public Notes | Files associated with this item cannot be displayed due to copyright restrictions. |
Byline Affiliations | School of Civil Engineering and Surveying |
Charles University, Czech Republic | |
Institution of Origin | University of Southern Queensland |
https://research.usq.edu.au/item/q72xz/predicting-the-pyrite-oxidation-process-within-coal-waste-piles-using-multiple-linear-regression-mlr-and-teaching-learning-based-optimization-tlbo-algorithm
111
total views4
total downloads1
views this month0
downloads this month
Export as
Related outputs
A Critical Review on Development of Laboratory Testing Technologies of Tendons Applied to Stabilize Underground Structures
Mottahedi, Adel, Aziz, Naj, Remennikov, Alex, Mirzaghorbanali, Ali and Marston, Kevin. 2025. "A Critical Review on Development of Laboratory Testing Technologies of Tendons Applied to Stabilize Underground Structures ." Rock Mechanics and Rock Engineering. https://doi.org/10.1007/s00603-025-04518-4Impact of Diverse Parameters on CO2 Adsorption in CO2 Sequestration: Utilizing a Novel Triaxial Testing Apparatus
Ardehjani, Emad Ansari, Ataei, Mohammad, Sereshki, Farhang, Mirzaghorbanali, Ali and Aziz, Naj. 2025. "Impact of Diverse Parameters on CO2 Adsorption in CO2 Sequestration: Utilizing a Novel Triaxial Testing Apparatus ." Greenhouse Gases: science and technology. 15 (1), pp. 53-67. https://doi.org/10.1002/ghg.2322Unveiling Axial Load Transfer Mechanism in Fully Encapsulated Rock Bolts
Nourizadeh, Hadi, Mirzaghorbanali, Ali, McDougall, Kevin, Mitri, Hani, Craig, Peter, Rastegarmanesh, Ashakan and Aziz, Naj. 2025. "Unveiling Axial Load Transfer Mechanism in Fully Encapsulated Rock Bolts ." Rock Mechanics and Rock Engineering. https://doi.org/10.1007/s00603-024-04347-xPerformance of Cable Bolts in Small- and Large-Scale Laboratory Pullout Tests
Rastegarmanesh, Ashkan, Mirzaghorbanali, Ali, McDougall, Kevin, Aziz, Naj, Anzanpour, Sina and Nourizadeh, Hadi. 2025. "Performance of Cable Bolts in Small- and Large-Scale Laboratory Pullout Tests." Geotechnical and Geological Engineering: an international journal. 43 (67). https://doi.org/10.1007/s10706-024-03019-5Exploring the axial performance of protective sheathed rock bolts through large-scale testing
Nourizadeh, Hadi, Mirzaghorbanali, Ali, McDougall, Kevin and Aziz, Naj. 2025. "Exploring the axial performance of protective sheathed rock bolts through large-scale testing." Tunnelling and Underground Space Technology. 155 (1). https://doi.org/10.1016/j.tust.2024.106157Impact Loading of Tendon Reinforcement Systems Used for Ground Control: A Critical Literature Review
Mottahedi, Adel, Aziz, Naj, Remennikov, A;ex, Mirzaghorbanali, Ali and Marston, Kevin. 2024. "Impact Loading of Tendon Reinforcement Systems Used for Ground Control: A Critical Literature Review." Archive of Mining Science. 69 (4), pp. 711-743. https://doi.org/10.24425/ams.2024.1525Digital Twin Technology in Built Environment: A Review of Applications, Capabilities and Challenges
Mousavi, Yalda, Gharineiat, Zahra, Agha Karimi, Armin Agha, McDougall, Kevin, Rossi, Adriana and Barsanti, Sara Gonizzi. 2024. "Digital Twin Technology in Built Environment: A Review of Applications, Capabilities and Challenges." Smart Cities. 7 (5), pp. 2594-2615. https://doi.org/10.3390/smartcities7050101Granular Soils and Contaminant Modeling in Tailing Dams
Farhadian, Hadi, Jodeiri Shokri, Behshad and Mirzaghorbanali, Ali. 2024. "Granular Soils and Contaminant Modeling in Tailing Dams." Minerals. 14 (11).Data-Driven Optimised XGBoost for Predicting the Performance of Axial Load Bearing Capacity of Fully Cementitious Grouted Rock Bolting Systems
Jodeiri Shokri, Behshad, Mirzaghorbanali, Ali, McDougall, Kevin, Karunasena, Warna, Nourizadeh, Hadi, Entezam, Shima, Hosseini, Shahab and Aziz, Naj. 2024. "Data-Driven Optimised XGBoost for Predicting the Performance of Axial Load Bearing Capacity of Fully Cementitious Grouted Rock Bolting Systems ." Applied Sciences. 14. https://doi.org/10.3390/app14219925Examining the Impact of Coal Gas Emissions on the Stability Analysis of Coal Pillars: A Critical Literature Review
Ardehjani,Emad Ansari, Ataei, Mohammad, Sereshki, Farhang, Mirzaghorbanali, Ali and Aziz, Naj. 2024. "Examining the Impact of Coal Gas Emissions on the Stability Analysis of Coal Pillars: A Critical Literature Review." Mining-Geology-Petroleum Engineering Bulletin. 39 (3), pp. 77-94. https://doi.org/10.17794/rgn.2024.3.7Small scale laboratory monotonic and cyclic pull out testing on grout and resin encapsulated cable bolts
Rastegarmanesh, Ashkan, Mirzaghorbanali, Ali, McDougall, Kevin, Aziz, Naj, Anzanpour, Sina, Nourizadeh, Hadi and Moosavi, Mahdi. 2024. "Small scale laboratory monotonic and cyclic pull out testing on grout and resin encapsulated cable bolts ." International Journal of Rock Mechanics and Mining Sciences. 183. https://doi.org/10.1016/j.ijrmms.2024.105914Analysing Double Shearing Mechanism in Fiberglass Rock Bolting Systems: a Comprehensive Analytical Model and Numerical Simulation Approach
Gregor, Peter, Mirzaghorbanali, Ali, McDougall, Kevin, Aziz, Naj, Jodeiri Shokri, Behshad, Nourizadeh, Hadi and Taheri, Abbas. 2024. "Analysing Double Shearing Mechanism in Fiberglass Rock Bolting Systems: a Comprehensive Analytical Model and Numerical Simulation Approach." Geotechnical and Geological Engineering: an international journal. 42 (8), pp. 7339-7370. https://doi.org/10.1007/s10706-024-02929-8Predicting axial-bearing capacity of fully grouted rock bolting systems by applying an ensemble system
Hosseini, Shahab, Jodeiri Shokri, Behshad, Mirzaghorbanali, Ali, Nourizadeh, Hadi, Entezam, Shima, Motallebiyan, Amin, Entezam, Alireza, McDougall, Kevin, Karunasena, Warna and Aziz, Naj. 2024. "Predicting axial-bearing capacity of fully grouted rock bolting systems by applying an ensemble system." Soft Computing. 28, pp. 10491-10518. https://doi.org/10.1007/s00500-024-09828-3Predicting grout’s uniaxial compressive strength (UCS) for fully grouted rock bolting system by applying ensemble machine learning techniques
Hosseini, Shahab, Jodeiri Shokri, Behshad, Entezam, Shima, Mirzaghorbanali, Ali, Nourizadeh, Hadi, Motallebiyan, Amin, Entezam, Alireza, McDougall, Kevin, Karunasena, Warna and Aziz, Naj. 2024. "Predicting grout’s uniaxial compressive strength (UCS) for fully grouted rock bolting system by applying ensemble machine learning techniques." Neural Computing and Applications. 36, pp. 18387-18412. https://doi.org/10.1007/s00521-024-10128-yAxial Load Transfer Mechanism in Fully Grouted Rock Bolting System: A Systematic Review
Jodeiri Shokri, Behshad, Mirzaghorbanali, Ali, Nourizadeh, Hadi, McDougall, Kevin, Karunasena, Warna, Aziz, Naj, Entezam, Shima and Entezam, Alireza. 2024. "Axial Load Transfer Mechanism in Fully Grouted Rock Bolting System: A Systematic Review ." Applied Sciences. 14 (12). https://doi.org/10.3390/app14125232Aspects of testing tendon supports for strength, effective installation, and performance in different ground formation
Mottahedi, Adel, Aziz, Naj, Mirzaghorbanali, Ali, Remmenikov, Alex and Anzanpour, Sina. 2024. "Aspects of testing tendon supports for strength, effective installation, and performance in different ground formation." Aziz, N. and Mirzaghorbanali, A. (ed.) 2024 Resource Operators Conference (ROC2024). Wollongong, Australia 13 - 16 Feb 2024 Australia. University of Wollongong.Application of waste glass powder in cemenitious grouts
Entezam, Alireza, Nourizadeh, Hadi, Mirzaghorbanali, Ali, Burey, Polly, Shelley, Tristan and Aziz, Naj. 2024. "Application of waste glass powder in cemenitious grouts." Aziz, N. and Mirzaghorbanali, A. (ed.) 2024 Resource Operators Conference (ROC2024). Wollongong, Australia 13 - 16 Feb 2024 Australia. University of Wollongong.Finite element modelling of fully encapsulated cable bolts in laboratory large scale pull out test
Mirzaghorbanali, Ashkan, Rastegarmanesh, Ali, McDougall, Kevin, Aziz, Naj, Anzanpour, Sina and Nourizadeh, Hadi. 2024. "Finite element modelling of fully encapsulated cable bolts in laboratory large scale pull out test." Aziz, N. and Mirzaghorbanali, A. (ed.) 2024 Resource Operators Conference (ROC2024). Wollongong, Australia 13 - 16 Feb 2024 Australia. University of Wollongong.Investigating the axial and shear performance of fiberglass rock boltss
Entezam, Shima, Jodeiri Shokri, Behshad, Nourizadeh, Hadi, Gregor, Peter, Entezam, Alireza, Mirzaghorbanali, Ali, McDougall, Kevin, Karunasena, Karu and Aziz, Naj. 2024. "Investigating the axial and shear performance of fiberglass rock boltss." Aziz, N. and Mirzaghorbanali, A. (ed.) 2024 Resource Operators Conference (ROC2024). Wollongong, Australia 13 - 16 Feb 2024 Australia. University of Wollongong.Finite element simulation of fully grouted rock bolts behaviour across varied bore hole diameters
Jodeiri Shokri, Behshad, Mirzaghorbanali, Ali, Karunasena, Waru, McDougall, Kevin, Aziz, Naj, Entezam, Shima, Nourizadeh, Hadi, Motallebiyan, Amin and Entezam, Alireza. 2024. "Finite element simulation of fully grouted rock bolts behaviour across varied bore hole diameters." Aziz, N. and Mirzaghorbanali, A. (ed.) 2024 Resource Operators Conference (ROC2024). Wollongong, Australia 13 - 16 Feb 2024 Australia. University of Wollongong.Identifying the Impact Factors on the Land Market in Nepal from Land Use Regulation
Subedi, Nab Raj, McDougall, Kevin and Paudyal, Dev Raj. 2024. "Identifying the Impact Factors on the Land Market in Nepal from Land Use Regulation." Urban Science. 8 (2). https://doi.org/10.3390/urbansci8020058Mapping Erosion Hotspots: Coherent Change Detection in the Quilpie Region, Queensland, Australia
Cook, Kyran, Agha Karimi, Armin, Grinham, Alistair and McDougall, Kevin. 2024. "Mapping Erosion Hotspots: Coherent Change Detection in the Quilpie Region, Queensland, Australia." Remote Sensing. 16 (7). https://doi.org/10.3390/rs16071263A look at the performance of barrel and wedge assembly in cable bolts applications
Rastegarmanesh, Ashkan, Mirzaghorbanali, Ali, McDougall, Kevin, Aziz, Naj, Anzanpour, Sina, Nourizadeh, Hadi and Taheri, Abbas. 2024. "A look at the performance of barrel and wedge assembly in cable bolts applications ." Scientific Reports. 14. https://doi.org/10.1038/s41598-024-54999-6Failure characterization of fully grouted rock bolts under triaxial testing
Nourizadeh, Hadi, Mirzaghorbanali, Ali, Serati, Mehdi, Mahmoud, Mutaz El-Amin, McDougall, Kevin and Aziz, Naj. 2024. "Failure characterization of fully grouted rock bolts under triaxial testing." Journal of Rock Mechanics and Geotechnical Engineering. 16 (3), pp. 778-789. https://doi.org/10.1016/j.jrmge.2023.08.013Investigating shear behaviour of fibreglass rock bolts reinforcing infilled discontinuities for various pretension loads
Gregor, Peter, Mirzaghorbanali, Ali, McDougall, Kevin, Aziz, Naj and Jodeiri Shokri, Behshad. 2024. "Investigating shear behaviour of fibreglass rock bolts reinforcing infilled discontinuities for various pretension loads." Canadian Geotechnical Journal. 61 (3). https://doi.org/10.1139/cgj-2022-0619From we ask to iASK: a self-reflection strategy enabling students to connect assessment and employability
Spagnoli, Dino, Kinash, Shelley, Jorre de st Jorre, Trina, Male, Sally, Mouat, Clare M. and McDougall, Kevin. 2023. "From we ask to iASK: a self-reflection strategy enabling students to connect assessment and employability." Journal of Teaching and Learning for Graduate Employability. 14 (1), pp. 71-87. https://doi.org/10.21153/jtlge2023vol14no1art1654Shear Behaviour of Fibreglass Rock Bolts for Various Pretension Loads
Gregor, Peter, Mirzaghorbanali, Ali, McDougall, Kevin, Aziz, Naj and Jodeiri Shokri, Behshad. 2023. "Shear Behaviour of Fibreglass Rock Bolts for Various Pretension Loads." Rock Mechanics and Rock Engineering. 56 (11), pp. 8083-8113. https://doi.org/10.1007/s00603-023-03474-1An Alternative Method for Estimating the Peak Flow for a Regional Catchment Considering the Uncertainty via Continuous Simulation
Brown, Ian, McDougall, Kevin, Chadalavada, Sreeni and Alam, Md Jahangir. 2023. "An Alternative Method for Estimating the Peak Flow for a Regional Catchment Considering the Uncertainty via Continuous Simulation ." Water. 15 (19). https://doi.org/10.3390/w15193355Characterization of mechanical and bonding properties of anchoring resins under elevated temperature
Nourizadeh, Hadi, Mirzaghorbanali, Ali, McDougall, Kevin, Jeewantha, L.H.J., Craig, Peter, Motallebiyan, Amin, Jodeiri Shokri, Behshad Jodeiri, Rastegarmanesh, Ashakan and Aziz, Naj. 2023. "Characterization of mechanical and bonding properties of anchoring resins under elevated temperature." International Journal of Rock Mechanics and Mining Sciences. 170. https://doi.org/10.1016/j.ijrmms.2023.105506Axial Response of Resin Encapsulated Cable Bolts in Monotonic and Cyclic Loading
Rastegarmanesh, Ashkan, Mirzaghorbanali, Ali, McDougall, Kevin, Aziz, Naj, Anzanpour, Sina, Nourizadeh, Hadi and Moosavi, Mahdi. 2023. "Axial Response of Resin Encapsulated Cable Bolts in Monotonic and Cyclic Loading ." Canadian Geotechnical Journal. 60 (11). https://doi.org/10.1139/cgj-2022-0379Assessing the relationship between tree dimensions in an urban environment
Scarmana, G. and McDougall, K.. 2023. "Assessing the relationship between tree dimensions in an urban environment ." 12th International Symposium on Mobile Mapping Technology (MMT 2023). Padua, Italy 24 - 26 May 2023 Italy. https://doi.org/10.5194/isprs-archives-XLVIII-1-W1-2023-437-2023Construction and Deconstruction: Materials and substances from 'waste'
Burey, Paulomi, Feldman, Jessica, Seligmann, Hannah, Song, Eric, Flynn, Matthew, Helwig, Andreas, Gharineiat, Zahra, Seneviratne, Dinuki, Whiteside, Eliza, Shelley, Tristan, Priesler, Nils, Manalo, Allan, Mirzaghorbanali, Ali, Nourizadeh, Hadi, Roberts, Michae, Nicol, Rose, Redmond, Petrea, Lynch, Mark, Dearnaley, John, ..., Germon, Geoff. 2023. "Construction and Deconstruction: Materials and substances from 'waste'." Chemistry in Australia. (June-August 2023), pp. 16-21.Shear strength properties of clean and clay infilled rock joints: an analysis of the impast of moisture content under CNL conditions
Downing, Elizabeth, Mirzaghorbanali, Ali and Aziz, Naj. 2023. "Shear strength properties of clean and clay infilled rock joints: an analysis of the impast of moisture content under CNL conditions." Aziz, Naj, Marston, Kevin and Mirzaghorbanali, Ali (ed.) 2023 Resource Operators conference (ROC2023). Wollongong, Australia 09 - 10 Feb 2023 Australia. University of Wollongong.Investigation of the Effect of Using Fly Ash in the Grout Mixture on Performing the Fully Grouted Rock Bolt Systems
Entezam, Shima, Jodeiri Shokri, Behshad, Nourizadeh, Hadi, Motallebiyan, Amin, Mirzaghorbanali, Ali, McDougall, Kevin, Aziz, Naj and Karunasena, Karu. 2023. "Investigation of the Effect of Using Fly Ash in the Grout Mixture on Performing the Fully Grouted Rock Bolt Systems." Aziz, Naj, Marston, Kevin and Mirzaghorbanali, Ali (ed.) 2023 Resource Operators conference (ROC2023). Wollongong, Australia 09 - 10 Feb 2023 Australia. University of Wollongong.Substitution of Recycled Plastic Aggregates (RPA) in concrete and its influence on pullout capacity of mechanical and chemical anchors
Akhtar, Raza, Skellern, Graham and Mirzaghorbanali, Ali. 2023. "Substitution of Recycled Plastic Aggregates (RPA) in concrete and its influence on pullout capacity of mechanical and chemical anchors." Aziz, Naj, Manson, Kevin and Mirzaghorbanali, Ali (ed.) 2023 Resource Operators conference (ROC2023). Wollongong, Australia 09 - 10 Feb 2023 Australia. University of Wollongong.SPT-CPT Correlation in Southeast Queensland, Australia
Faivre, Yohanne, Mirzaghorbanali, Ali, Nourizadeh, Hadi, Jodeiri Shokri, Behshad, McDougall, Kevin and Aziz, Naj. 2023. "SPT-CPT Correlation in Southeast Queensland, Australia." Aziz, Naj, Marston, Kevin and Mirzaghorbanali, Ali (ed.) 2023 Resource Operators conference (ROC2023). Wollongong, Australia 09 - 10 Feb 2023 Australia. University of Wollongong.The effect of changing confinement diameter on axial load transfer mechanisms of fully grouted rock bolts
Jodeiri Shokri, Behshad Jodeiri, Entezam, Shima, Nourizadeh, Hadi, Motallebiyan, Amin, Mirzaghorbanali, Ali, McDougall, Kevin, Aziz, Naj and Karunasena, Karu. 2023. "The effect of changing confinement diameter on axial load transfer mechanisms of fully grouted rock bolts." Aziz, Naj, Marston, Kevin and Mirzaghorbanali, Ali (ed.) 2023 Resource Operators conference (ROC2023). Wollongong, Australia 09 - 10 Feb 2023 University of Wollongong. University of Wollongong.Finite Element Numerical Modelling of Rock Bolt Axial Behaviour Subject to Different Geotechnical Conditions
Nourizadeh, Hadi, Mirzaghorbanali, Ali, Aziz, Naj, McDougall, Kevin, Jodeiri Shokri, Behshad, Sahebi, Akbar, Motallebiyan, Amin and Entezam, Shima. 2023. "Finite Element Numerical Modelling of Rock Bolt Axial Behaviour Subject to Different Geotechnical Conditions." Aziz, Naj, Marston, Kevin and Mirzaghorbanali, Ali (ed.) 2023 Resource Operators conference (ROC2023). Wollongong, Australia 09 - 10 Feb 2023 Australia. University of Wollongong.Application of numerical modeling in study of the behaviour of cable bolts under axial loading
Anzanpour, Sina, Aziz, Naj, Topa, Ameen, Nemcik, Jan and Mirzaghorbanali, Ali. 2023. "Application of numerical modeling in study of the behaviour of cable bolts under axial loading." Aziz, Naj, Marston, Kevin and Mirzaghorbanali, Ali (ed.) 2023 Resource Operators conference (ROC2023). Wollongong, Australia 09 - 10 Feb 2023 Australia. University of Wollongong.Effects of rib distances on axial load transfer mechanisms of fully grouted rock bolts
Nourizadeh, Hadi, Motallebiyan, Amin, Jodeiri Shokri, Behshad, Entezam, Shima, Mirzaghorbanali, Ali, Aziz, Naj and McDougall, Kevin. 2023. "Effects of rib distances on axial load transfer mechanisms of fully grouted rock bolts." Aziz, Naj, Marston, Kevin and Mirzaghorbanali, Ali (ed.) 2023 Resource Operators conference (ROC2023). Wollongong, Australia 09 - 10 Feb 2023 Australia. University of Wollongong.Investigating the effect of coal macerals on the recovery rate of the flotation process- a case study
Jodeiri Shokri, Behshad, Entezam, Shima, Entezam, Alireza and Mirzaghorbanali, Ali. 2023. "Investigating the effect of coal macerals on the recovery rate of the flotation process- a case study." Aziz, Naj, Marston, Kevin and Mirzaghorbanali, Ali (ed.) 2023 Resource Operators conference (ROC2023). Wollongong, Australia 09 - 10 Feb 2023 Australia. University of Wollongong.Crowd-Assisted Flood Disaster Management
Koswatte, S., McDougall, K. and Liu, X.. 2023. "Crowd-Assisted Flood Disaster Management." Singh, Vijay P., Yadav, Shalini, Yadac, Krishna Kumar, Corzo, Gerald Augusto, Munoz-Arriola, Francisco and Yadava, Ram Narayan (ed.) Application of Remote Sensing and GIS in Natural Resources and Built Infrastructure Management. Switzerland. Springer. pp. 39-55Laboratory study of the behaviour of grouted cable bolts under static and dynamic axial loading
Anzanpour, S., Aziz, N., Remennikov, A., Nemcik, J., Mirzaghorbanali, A. and Rastegarmanesh, A.. 2022. "Laboratory study of the behaviour of grouted cable bolts under static and dynamic axial loading." Eurock 2022: Rock and Fracture Mechanics in Rock Engineering and Mining. Espoo, Finland Finland.Use of time-lapse 2D and 3D geoelectrical inverse models for monitoring acid mine drainage - a case study
Shokri, Behshad Jodeiri, Shafaei, Foojan, Ardejani, Faramarz Doulati, Mirzaghorbanali, Ali and Entezam, Shima. 2023. "Use of time-lapse 2D and 3D geoelectrical inverse models for monitoring acid mine drainage - a case study." Soil and Sediment Contamination: an international journal. 32 (4), pp. 376-399. https://doi.org/10.1080/15320383.2022.2090895Static and dynamic tendon pull-out test research at the University of Wollongong
Anzanpour, Sina, Aziz, Naj, Nemcik, Naj, Remennikov, Alex, Mirzaghorbanali, Ali and Wallace, Jordan. 2022. "Static and dynamic tendon pull-out test research at the University of Wollongong." Aziz, Naj and Mirzaghorbanali, Ali (ed.) 2022 Resource Operators Conference (ROC2022). Australia Feb 2022 Australia.Crack damage evolution in coal at elevated temperatures
Serati, Mehdi, Kwan, King Ho Benny, Gordon, Nick, Moravej, Sasan, Bahaaddini, Mojtaba and Mirzaghorbanali, Ali. 2022. "Crack damage evolution in coal at elevated temperatures." Aziz, Naj and Mirzaghorbanali, Ali (ed.) 2022 Resource Operators Conference (ROC2022). Australia Feb 2022 Wollongong, Australia.Development of a wireless system to measure the strain/deformation of rock bolts
Nourizadeh, Hadi, Mirzaghorbanali, Ali, Aziz, Naj, McDougall, Kevin and Sahebi, Ali Akbar. 2022. "Development of a wireless system to measure the strain/deformation of rock bolts." Aziz, Naj and Mirzaghorbanali, Ali (ed.) 2022 Resource Operators Conference (ROC2022). Australia Feb 2022 Australia.Application of a geographic information system (GIS) for selecting the location of a coal wastes dump - a case study
Shokri, Behshad Jodeiri, Ardejani, Faramarz Doulati, Bagheri, Mehdi, Entezam, Shima, Mirzaghorbanali, Ali, McDougall, Kevin and Aziz, Naj. 2022. "Application of a geographic information system (GIS) for selecting the location of a coal wastes dump - a case study." Aziz, Naj and Mirzaghorbanali, Ali (ed.) 2022 Resource Operators Conference (ROC2022). Australia Feb 2022 Australia.Shear behavior of clayey infilled rock joints having triangular and sinusoidal asperities
Mirzaghorbanali, Ali, Ghimire, Basanta, Rastegarmanesh, Ashkan, Nourizadeh, Hadi, McDougall, Kevin and Aziz, Naj. 2022. "Shear behavior of clayey infilled rock joints having triangular and sinusoidal asperities." Aziz, Naj and Mirzaghorbanali, Ali (ed.) 2022 Resource Operators Conference (ROC2022). Australia Feb 2022 Australia.Probabilistic risk assessment of acid mine drainage generation resulted from chalcopyrite oxidation process within Sarcheshmeh copper mine tailings
Entezam, Shima, Shokri, Behshad Jodeiri, Ardejani, Faramarz Doulati, Mirzaghorbanali, Ali, McDougall, Kevin and Aziz, Naj. 2022. "Probabilistic risk assessment of acid mine drainage generation resulted from chalcopyrite oxidation process within Sarcheshmeh copper mine tailings." Aziz, Naj and Mirzaghorbanali, Ali (ed.) 2022 Resource Operators Conference (ROC2022). Australia Feb 2022 Australia.Angle Shear Testing of 15.2 mm Seven Wire Cable Bolt
Aziz, Naj, Mirzaghorbanali, Ali, Anzanpour, Sina, Rastegarmanesh, Ashkan, Khaleghparast, Saman, Nemcik, Jan, Oh, Joung and Si, Guangyao. 2022. "Angle Shear Testing of 15.2 mm Seven Wire Cable Bolt." Rock Mechanics and Rock Engineering. 55 (7), pp. 3919-3937. https://doi.org/10.1007/s00603-022-02847-2Effect of pretension on the performance of cable bolts and its optimisation in underground coal mines with various geological conditions
Li, Xu, Si, Guangyao, Oh, Joung, Corbett, Peter, O'Sullivan, Terri, Xiang, Zizhuo, Aziz, Naj and Mirzaghorbanali, Ali. 2022. "Effect of pretension on the performance of cable bolts and its optimisation in underground coal mines with various geological conditions." International Journal of Rock Mechanics and Mining Sciences. 152, pp. 1-21. https://doi.org/10.1016/j.ijrmms.2022.105076Calibration of a continuous hydrologic simulation model in the urban Gowrie Creek catchment in Toowoomba, Australia
Brown, I. W., McDougall, K., Alam, Md. Jahangir, Chowdhury, R. and Chadalavada, S.. 2022. "Calibration of a continuous hydrologic simulation model in the urban Gowrie Creek catchment in Toowoomba, Australia." Journal of Hydrology: Regional Studies. 40, pp. 1-16. https://doi.org/10.1016/j.ejrh.2022.101021Fracture propagation mode of coal under indirect tensile stresses
Serati, Mehdi, Roshan, Hamid, Mirzaghorbanali, Ali, Mahmoud, Mutaz El-Amin and Thejaswee, Valluru. 2021. "Fracture propagation mode of coal under indirect tensile stresses." Aziz, Naj and Mirzaghorbanali, Ali (ed.) 2021 Resource Operators Conference (ROC2021). Springfield, Australia 10 - 12 Feb 2021 Australia.Strength properties of grout for strata reinforcement
Majoor, Dean, Mirzaghorbanali, Ali and Aziz, Naj. 2017. "Strength properties of grout for strata reinforcement." Aziz, Naj and Kinnemonth, Bob (ed.) 2017 Coal Operators' Conference. Wollongong, Australia 08 - 10 Feb 2017 Wollongong, Australia.The extent of shearing and the integrity of protective sleeve coating of cable bolts
Aziz, Naj, Mirzaghorbanali, Ali and Holden, Matthew. 2017. "The extent of shearing and the integrity of protective sleeve coating of cable bolts." Aziz, Naj and Kinnemonth, Bob (ed.) 2017 Coal Operators' Conference. Wollongong, Australia 08 - 10 Feb 2017 Wollongong, Australia.Axial Performance of Cementitious Grouted Cable Bolts Under Rotation Constraint Scenarios
Rastegarmanesh, Ashkan, Mirzaghorbanali, Ali, McDougall, Kevin, Aziz, Naj, Anzanpour, Sina, Nourizadeh, Hadi and Moosavi, Mahdi. 2022. "Axial Performance of Cementitious Grouted Cable Bolts Under Rotation Constraint Scenarios." Rock Mechanics and Rock Engineering. 55 (9), pp. 5773-5788. https://doi.org/10.1007/s00603-022-02950-4A case of Longwall coal mining productivity & safety optimisation
Ardehali, Sahar, Alehossein, Habib, Aziz, Naj, Bowerman, Matthew and Robbins, Matt. 2021. "A case of Longwall coal mining productivity & safety optimisation." Aziz, Naj and Mirzaghorbanali, Ali (ed.) 2021 Resource Operators Conference (ROC2021). Springfield, Australia 10 - 12 Feb 2021 Australia.Proceedings of the 2022 Resource Operators Conference
Aziz, Naj and Mirzaghorbanali, Ali. Aziz, Naj and Mirzaghorbanali, Ali (ed.) 2022. Proceedings of the 2022 Resource Operators Conference. Wollongong, Australia. University of Wollongong.Prediction of Fly-rock using Gene Expression Programming and Teaching– learning-based Optimization Algorithm
Shamsi, R., Amin, Mohammad Saeed, Dehghani, Hesam, Bascompta, Marc, Jodeiri Shokri, Behshad and Entezam, Shima. 2022. "Prediction of Fly-rock using Gene Expression Programming and Teaching– learning-based Optimization Algorithm." Journal of Mining and Environment. 13 (2), pp. 391-406. https://doi.org/10.22044/jme.2022.11825.2171Determination of ozone concentration using gene expression programming algorithm (GEP)- Zrenjanin, Serbia
Dehghani, Hesam, Velicković, Milica, Jodeiri Shokri, Behshad, Mihajlovic, Ivan, Nikolic, Djordje and Panic, Marija. 2022. "Determination of ozone concentration using gene expression programming algorithm (GEP)- Zrenjanin, Serbia." International Journal of Mining and Geo-Engineering. 56 (1), pp. 1-9. https://doi.org/10.22059/IJMGE.2021.313278.594874Developing a Conceptual Framework of Green Mining Strategy in Coal Mines: Integrating Socio-economic, Health, and Environmental Factors
Ardejani, Faramarz Doulati, Maghsoudy, Soroush, Shahhosseini, Majid, Jodeiri Shokri, Behshad, Ardejani, Shaghayegh Doulati, Shafaei, Foojan, Shiraz, Farzin Amirkhani and Rajaee, Ali. 2022. "Developing a Conceptual Framework of Green Mining Strategy in Coal Mines: Integrating Socio-economic, Health, and Environmental Factors." Journal of Mining and Environment. 13 (1), pp. 101-115. https://doi.org/10.22044/jme.2022.11704.2161Next Generation “Living” Laboratory for Engineering Education and Engagement
Nguyen, Andy, Le, Ngoc Thach, Landers, Richard, Byrne, Terry, McDougall, Kevin, Gharehbaghi, Vahid Reza, Brown, Jason, Nguyen, Canh Long and Karunasena, Warna. 2022. "Next Generation “Living” Laboratory for Engineering Education and Engagement." 33rd Annual Conference of the Australasian Association of Engineering Education (AAEE 2022). Sydney, Australia 04 - 07 Dec 2022 Australia.A convolution-deconvolution method for improved storage and communication of remotely-sensed image data
Scarmana, Gabriel and McDougall, Kevin. 2018. "A convolution-deconvolution method for improved storage and communication of remotely-sensed image data." Larar, Allen M., Suzuki, Makoto and Wang, Jianyu (ed.) 11th SPIE Asia-Pacific Remote Sensing Symposium 2018: Multispectral, Hyperspectral, and Ultraspectral Remote Sensing Technology, Techniques and Applications VII. Honolulu, United States 24 - 26 Sep 2018 Bellingham, Washington, United States. https://doi.org/10.1117/12.2324451Defining and Enabling Scholarship of Teaching, Learning, and Employability (SoTLE) Through a Multi-Institution Faculty Learning Community
Kinash, Shelley, Male, Sally A., Mouat, Clare M., Spagnoli, Dino, McDougall, Kevin, Thomas, Jasmine and Mortimer, Chloe Jayde. 2021. "Defining and Enabling Scholarship of Teaching, Learning, and Employability (SoTLE) Through a Multi-Institution Faculty Learning Community." Learning Communities Journal. 13 (1), pp. 81-103.Axial behaviour of rock bolts - Part (B) Numerical study
Nourizadeh, Hadi, Mirzaghorbanali, Ali, McDougall, Kevin, Aziz, Naj and Serati, Mehdi. 2021. "Axial behaviour of rock bolts - Part (B) Numerical study." Aziz, Naj and Mirzaghorbanali, Ali (ed.) 2021 Resource Operators Conference (ROC2021). Springfield, Australia 10 - 12 Feb 2021 Australia.Axial behaviour of rock bolts - Part (A) Experimental study
Nourizadeh, Hadi, Williams, Sally, Mirzaghorbanali, Ali, McDougall, Kevin, Aziz, Naj and Serati, Mehdi. 2021. "Axial behaviour of rock bolts - Part (A) Experimental study." Aziz, Naj and Mirzaghorbanali, Ali (ed.) 2021 Resource Operators Conference (ROC2021). Springfield, Australia 10 - 12 Feb 2021 Australia.Introduction to new methods of static and dynamic pull testing of rock bolts and cable bolts
Anzanpour, Sina, Aziz, Naj, Nemcik, Jan, Mirzaghorbanali, Ali, Wallace, Jordan, Marshall, Travis and Khaleghparast, Saman. 2021. "Introduction to new methods of static and dynamic pull testing of rock bolts and cable bolts." Aziz, Naj and Mirzaghorbanali, Ali (ed.) 2021 Resource Operators Conference (ROC2021). Springfield, Australia 10 - 12 Feb 2021 Australia.Application of Monte Carlo simulation to quantify uncertainties of first weighting interval estimation
Mohammadi, Sadjad, Ataei, Mohammad, Mirzaghorbanali, Ali and Aziz, Naj. 2021. "Application of Monte Carlo simulation to quantify uncertainties of first weighting interval estimation." Aziz, Naj and Mirzaghorbanali, Ali (ed.) 2021 Resource Operators Conference (ROC2021). Springfield, Australia 10 - 12 Feb 2021 Australia.Pretension effect on the performance of cable bolts in underground coal mines
Li, Xu, Si, Guangyao, Oh, Joung, Xiang, Zizhuo, Aziz, Naj and Mirzaghorbanali, Ali. 2021. "Pretension effect on the performance of cable bolts in underground coal mines." Aziz, Naj and Mirzaghorbanali, Ali (ed.) 2021 Resource Operators Conference (ROC2021). Springfield, Australia 10 - 12 Feb 2021 Australia.Effect of surface profile on axial load transfer mechanism of tendons
Rastegarmanesh, Ashkan, Misa, Joel, Mirzaghorbanali, Ali, Aziz, Naj and McDougall, Kevin. 2021. "Effect of surface profile on axial load transfer mechanism of tendons." Aziz, Naj and Mirzaghorbanali, Ali (ed.) 2021 Resource Operators Conference (ROC2021). Springfield, Australia 10 - 12 Feb 2021 Australia.Angled shear testing of 15.2 mm seven wire cable bolt
Aziz, Naj, Anzanpour, Sina, Khaleghparast, Saman, Rastegarmanesh, Ashkan, Mirzaghorbanali, Ali, Remmenikov, Alex, Nemcik, Jan, Oh, Joung and Si, Guangyao. 2021. "Angled shear testing of 15.2 mm seven wire cable bolt." Aziz, Naj and Mirzaghorbanali, Ali (ed.) 2021 Resource Operators Conference (ROC2021). Springfield, Australia 10 - 12 Feb 2021 Australa.Proceedings of the 2021 Resource Operators Conference
Aziz, Naj and Mirzaghorbanali, Ali. Aziz, Naj and Kininmonth, Bob (ed.) 2021. Proceedings of the 2021 Resource Operators Conference. Wollongong, Australia. University of Wollongong.Using geospatial tools to combat fire ants
Scarmana, Gabriel and McDougall, Kevin. 2021. "Using geospatial tools to combat fire ants." Position Magazine.ISPRS Annals of the Photogrammetry, Remote Sensing and Spatial Information Sciences [Special issue]
McDougall, Kevin, Bhatta, Ganesh Prasad, Paudyal, Dev Raj, Shrestha, Reshma, Upadhyaya, Pradeep Sapkota, Dahal, Tank Prasad, Ranjit, Bhuwan, Shrestha, Sanjeevan and Thapa, Laxmi. 2019. ISPRS Annals of the Photogrammetry, Remote Sensing and Spatial Information Sciences [Special issue]. Germany. Copernicus GmbH.The International Archives of the Photogrammetry, Remote Sensing and Spatial Information Sciences
McDougall, Kevin, Bhatta, Ganesh Prasad, Paudyal, Dev Raj, Shrestha, Reshma, Upadhyaya, Pradeep Sapkota, Dahal, Tank Prasad, Ranjit, Bhuwan, Shrestha, Sanjeevan and Thapa, Laxmi. McDougall, Kevin, Paudyal, Dev Raj, Bhatta, Ganesh Prasad, Thapa, Laxmi, Dahal, Tank Prasad, Bhuwan, Ranjit, Sanjeevan, Shrestha, Shrestha, Reshma and Upadhyaya, Pradeep S (ed.) 2019. The International Archives of the Photogrammetry, Remote Sensing and Spatial Information Sciences. Germany. Copernicus GmbH.Static and dynamic testing of tendons
Aziz, Naj, Khaleghparast, Saman, Anzanpour, Sina, Remennikov, Alex and Mirzaghorbanali, Ali. 2020. "Static and dynamic testing of tendons." Rasskazov, Igor and Tkach, Sergei (ed.) VIII International Scientific Conference “Problems of Complex Development of Georesources” (PCDG 2020). Khabarovsk, Russian Federation 08 - 10 Sep 2020 France. https://doi.org/10.1051/e3sconf/202019201005Numerical simulation of influence parameters on induced stresses distribution during first weighting process of longwall mining
Mohammadi, S., Ataei, M., Kakaie, R. and Mirzaghorbanali, A.. 2021. "Numerical simulation of influence parameters on induced stresses distribution during first weighting process of longwall mining." Journal of Mining Engineering.A Probabilistic Model to Determine Main Caving Span by Evaluating Cavability of Immediate Roof Strata in Longwall Mining
Mohammadi, Sadjad, Ataei, Mohammad, Kakaie, Reza, Mirzaghorbanali, Ali and Aziz, Naj. 2021. "A Probabilistic Model to Determine Main Caving Span by Evaluating Cavability of Immediate Roof Strata in Longwall Mining." Geotechnical and Geological Engineering: an international journal. 39 (3), pp. 2221-2237. https://doi.org/10.1007/s10706-020-01620-yUnderstanding Contextual Attractiveness Factors of Transit Orientated Shopping Mall Developments (Tosmds) for Shopping Mall Passengers on the Dubai Metro Red Line
Abutaleb, Ayman, McDougall, Kevin, Basson, Marita, Hassan, Rumman and Mahmood, Muhammad Nateque. 2021. "Understanding Contextual Attractiveness Factors of Transit Orientated Shopping Mall Developments (Tosmds) for Shopping Mall Passengers on the Dubai Metro Red Line." Planning Practice and Research. 36 (3), pp. 292-313. https://doi.org/10.1080/02697459.2020.1829282The Impact of Transit-Oriented Shopping Mall Developments (TOSMDs) on Metro Station Ridership: Dubai Metro Redline
Abutaleb, Ayman, McDougall, Kevin, Basson, Marita, Hassan, Rumman and Mahmood, Muhammad Nateque. 2020. "The Impact of Transit-Oriented Shopping Mall Developments (TOSMDs) on Metro Station Ridership: Dubai Metro Redline." Urban Rail Transit. 6 (3), pp. 157-170. https://doi.org/10.1007/s40864-020-00129-0Static and dynamic testing of tendons
Khaleghparast, Saman, Anzanpour, Sina, Aziz, Naj, Remennikov, Alex and Mirzaghorbanali, Ali. 2020. "Static and dynamic testing of tendons." Aziz, Naj and Kininmonth, Bob (ed.) 2020 Coal Operators' Conference. Wollongong, Australia 18 - 20 Feb 2019 Wollongong, Australia.A review of overburden fracturing and changes in hydraulic characteristics due to longwall mining
Nourizadeh, Hadi, Canbulat, Ismet, Oh, Joung, Zhang, Chengguo, Aziz, Naj, Mirzaghorbanali, Ali and McDougall, Kevin. 2020. "A review of overburden fracturing and changes in hydraulic characteristics due to longwall mining." Aziz, Naj and Kininmonth, Bob (ed.) 2020 Coal Operators' Conference. Wollongong, Australia 18 - 20 Feb 2019 Wollongong, Australia.Shear strength properties of artificial rock joints
Zohaib, Muhammad, Mirzaghorbanali, Ali, Helwig, Andreas, Aziz, Naj, Gregor, Peter, Rastegarmanesh, Ashkan and McDougall, Kevin. 2020. "Shear strength properties of artificial rock joints." Aziz, Naj and Kininmonth, Bob (ed.) 2020 Coal Operators' Conference. Wollongong, Australia 18 - 20 Feb 2019 Wollongong, Australia.A process for the accurate reconstruction of pre-filtered and compressed digital aerial images
Scarmana, Gabriel and McDougall, Kevin. 2020. "A process for the accurate reconstruction of pre-filtered and compressed digital aerial images." 11th International Symposium on Digital Earth (ISDE 11). Florence, Italy 24 - 27 Sep 2019 Florence, Italy. https://doi.org/10.1088/1755-1315/509/1/012047A roof cavability classification system and its use for estimation of main caving interval in longwall mining
Mohammadi, Sodjad, Ataei, Mohammad, Kakaie, Reza, Mirzaghorbanali, Ali, Faraji Rad, Zahra and Aziz, Naj. 2020. "A roof cavability classification system and its use for estimation of main caving interval in longwall mining." Aziz, Naj and Kininmonth, Bob (ed.) 2020 Coal Operators' Conference. Wollongong, Australia 18 - 20 Feb 2019 Wollongong, Australia.A geometric model for estimating the volume and surface area of apples
Scarmana, Gabriel, McDougall, Kevin and Stepanyants, Yury. 2020. "A geometric model for estimating the volume and surface area of apples." Smart Surveyors for Land and Water Management (2020). Amsterdam, Netherlands 10 - 14 May 2020 Copenhagen, Denmark.Using a Mixed Method Research to Develop Spatial Information Sharing Strategies Across Natural Resource Management Communities
Paudyal, Dev Raj, McDougall, Kevin and Apan, Armando. 2019. "Using a Mixed Method Research to Develop Spatial Information Sharing Strategies Across Natural Resource Management Communities." Inaugural Australasia & Pacific Regional Mixed Methods International Research Association Conference 2019: Expanding Conceptual and Methodological Boundaries (MMIRA 2019). Wellington, New Zealand 04 - 06 Dec 2019 Indianapolis, United States.Numerical exploration of performance of cable bolts in shear loading
Rasekh, Haleh, Aziz, Naj, Nemcik, Jan and Mirzaghorbanali, Ali. 2019. "Numerical exploration of performance of cable bolts in shear loading." 4th Australasian Conference on Computational Mechanics (ACCM-4). Hobart, Australia 27 - 29 Nov 2019 Sydney, Australia.Towards a Conceptual Framework for Understanding the Attractiveness of Rail Transit-Oriented Shopping Mall Developments (TOSMDs)
Abutaleb, Ayman, McDougall, Kevin, Basson, Marita, Hassan, Rumman and Mahmood, Nateque. 2019. "Towards a Conceptual Framework for Understanding the Attractiveness of Rail Transit-Oriented Shopping Mall Developments (TOSMDs)." Urban Rail Transit. 5 (4), pp. 225-239. https://doi.org/10.1007/s40864-019-00112-4A new Roof Strata Cavability Index (RSCi) for longwall mining incorporating new rating system
Mohammadi, Sadjad, Ataei, Mohammad, Kakaie, Reza and Mirzaghorbanali, Ali. 2019. "A new Roof Strata Cavability Index (RSCi) for longwall mining incorporating new rating system." Geotechnical and Geological Engineering: an international journal. 37 (5), pp. 3619-3636. https://doi.org/10.1007/s10706-019-00857-6Numerical simulation of stress distribution in longwall panels during the first caving interval
Mohammadi, Sadjad, Ataei, Mohammad, Kakaie, Reza, Mirzaghorbanali, Ali, Aziz, Naj and Rastegarmanesh, Ashkan. 2019. "Numerical simulation of stress distribution in longwall panels during the first caving interval." Aziz, Naj and Kininmonth, Bob (ed.) 2019 Coal Operators' Conference. Wollongong, Australia 18 - 20 Feb 2019 Wollongong, Australia.Strength properties of grout for strata reinforcement
Mirzaghorbanali, Ali, Gregor, Peter, Ebrahim, Zeinab, Alfahed, Abdullah, Aziz, Naj and McDougall, Kevin. 2019. "Strength properties of grout for strata reinforcement." Aziz, Naj and Kininmonth, Bob (ed.) 2019 Coal Operators' Conference. Wollongong, Australia 18 - 20 Feb 2019 Wollongong, Australia.Shear strength of rock joints under constant normal loading conditions
Mirzaghorbanali, Ali, Alenzi, Faizal, Gregor, Peter, Aziz, Naj and McDougall, Kevin. 2019. "Shear strength of rock joints under constant normal loading conditions." Aziz, Naj and Kininmonth, Bob (ed.) 2019 Coal Operators' Conference. Wollongong, Australia 18 - 20 Feb 2019 Wollongong, Australia.Modelling of rebar and cable bolt behaviour in tension/shear
Li, Xuwei, Nemcik, Jan, Aziz, Naj, Mirzaghorbanali, Ali and Rasekh, Haleh. 2015. "Modelling of rebar and cable bolt behaviour in tension/shear." Aziz, Naj and Kininmonth, Bob (ed.) 2015 Coal Operators' Conference. Wollongong, Australia 11 - 13 Feb 2015 Australia.Effect of air column in transport canisters on measured gas contents of coal
Aziz, N. and Mirzaghorbanali, A.. 2017. "Effect of air column in transport canisters on measured gas contents of coal." Journal of Mining and Environment. 8 (1), pp. 1-9. https://doi.org/10.22044/jme.2016.631A study on the shear behaviour of infilled rock joints under cyclic loading and constant normal stiffness conditions
Mirzaghorbanali, Ali, Nemcik, Jan and Aziz, Naj. 2014. "A study on the shear behaviour of infilled rock joints under cyclic loading and constant normal stiffness conditions." Aziz, Naj (ed.) 2014 Coal Operators' Conference. Wollongong, Australia 12 - 14 Feb 2014 Wollongong, Australia.Suggested methods for the preparation and testing of various properties of resins and grouts
Aziz, Naj, Nemcik, Jan, Mirzaghorbanali, Ali, Foldi, Stephen, Joyce, David, Moslemi, Arash, Ghojavand, Hooman, Ma, Shuqi, Li, Xuwei and Rasekh, Haleh. 2014. "Suggested methods for the preparation and testing of various properties of resins and grouts." Aziz, Naj (ed.) 2014 Coal Operators' Conference. Wollongong, Australia 12 - 14 Feb 2014 Wollongong, Australia.Numerical modelling of cyclic shear behaviour of rock joints under constant normal stiffness condition
Mirzaghorbanali, Ali and Nemcik, Jan. 2013. "Numerical modelling of cyclic shear behaviour of rock joints under constant normal stiffness condition." Aziz, Naj, Kininmonth, Bob, Nemcik, Jan, Ren, Ting and Hoelle, John (ed.) 2013 Coal Operators' Conference. Wollongong, Australia 14 - 15 Feb 2013 Wollongong, Australia.Single shear testing of various cable bolts used in Australian mines
Aziz, Naj, Rink, Owen, Rasekh, Haleh, Hawkins, Ellie, Mirzaghorbanali, Ali, Yang, Guanyu, Khaleghparast, Saman, Mills, Kenneth, Nemcik, Jan and Li, Xuwei. 2017. "Single shear testing of various cable bolts used in Australian mines." Aziz, Naj and Kininmonth, Bob (ed.) 2017 Coal Operators' Conference. Wollongong, Australia 08 - 10 Feb 2017 Wollongong, Australia.Behaviour of cable bolts in shear; experimental study and mathematical modelling
Aziz, Naj, Craig, Peter, Mirzaghorbanali, Ali, Rasekh, Haleh, Nemcik, Jan and Li, Xuwei. 2015. "Behaviour of cable bolts in shear; experimental study and mathematical modelling." Aziz, Naj and Kininmonth, Bob (ed.) 2015 Coal Operators' Conference. Wollongong, Australia 11 - 13 Feb 2015 Wollongong, Australia.Strength characteristics of Secura Hollow Groutable Cable Bolts
Aziz, Naj, Hawker, Robert, Mirzaghorbanali, Ali, Nemcik, Jan, Li, Xuwei and Rasekh, Haleh. 2015. "Strength characteristics of Secura Hollow Groutable Cable Bolts." Aziz, Naj and Kininmonth, Bob (ed.) 2015 Coal Operators' Conference. Wollongong, Australia 11 - 13 Feb 2015 Wollongong, Australia.Strength properties of fibre glass dowels used for strata reinforcement in coal mines
Gilbert, David, Mirzaghorbanali, Ali, Li, Xuwei, Rasekh, Haleh, Aziz, Naj and Nemcik, Jan. 2015. "Strength properties of fibre glass dowels used for strata reinforcement in coal mines." Aziz, Naj and Kininmonth, Bob (ed.) 2015 Coal Operators' Conference. Wollongong, Australia 11 - 13 Feb 2015 Wollongong, Australia.An experimental study on the contact surface area of cabled bolted strata
Rasekh, Haleh, Aziz, Naj, Nemcik, Jan, Mirzaghorbanali, Ali and Li, Xuwei. 2015. "An experimental study on the contact surface area of cabled bolted strata." Aziz, Naj and Kininmonth, Bob (ed.) 2015 Coal Operators' Conference. Wollongong, Australia 11 - 13 Feb 2015 Wollongong, Australia.Numerical investigation of the shear behaviour of a cable bolt in single shear test
Li, Xuwei, Yang, Guanyu, Nemcik, Jan, Mirzaghorbanali, Ali and Aziz, Naj. 2019. "Numerical investigation of the shear behaviour of a cable bolt in single shear test." Tunnelling and Underground Space Technology. 84, pp. 227-236. https://doi.org/10.1016/j.tust.2018.11.016Mechanical behaviours of grout for strata reinforcement
Mirzaghorbanali, Ali, Gregor, Peter, Alkandari, Hamed, Aziz, Naj and McDougall, Kevin. 2018. "Mechanical behaviours of grout for strata reinforcement." Aziz, Naj and Kininmonth, Bob (ed.) 2018 Coal Operators' Conference. Wollongong, Australia 07 - 09 Feb 2018 Wollongong, Australia.ESTIMATION OF FIRST ROOF WEIGHTING INTERVAL INCORPORATING ROOF STRATA CAVABILITY CONCEPT
Mohammadi, S., Ataei, M., Kakaie, R. and Mirzaghorbanali, A.. 2019. "ESTIMATION OF FIRST ROOF WEIGHTING INTERVAL INCORPORATING ROOF STRATA CAVABILITY CONCEPT." Iranian Journal of Mining Engineering- IRJME.Fuzzy analysis of airborne LiDAR data for rainforest boundary determination
Zhang, Zhenyu, Liu, Xiaoye, McDougall, Kevin and Wright, Wendy. 2017. "Fuzzy analysis of airborne LiDAR data for rainforest boundary determination." 6th International Conference on Telecommunications and Remote Sensing (ICTRS'17). Delft, Netherlands 06 - 07 Nov 2017 New york, United States. https://doi.org/10.1145/3152808.3152816Relevance assessment of crowdsourced data (CSD) using semantics and geographic information retrieval (GIR) techniques
Koswatte, Saman, McDougall, Kevin and Liu, Xiaoye. 2018. "Relevance assessment of crowdsourced data (CSD) using semantics and geographic information retrieval (GIR) techniques." ISPRS International Journal of Geo-Information. 7 (7), pp. 1-18. https://doi.org/10.3390/ijgi7070256Innovative approach to strata reinforcement in coal mines with reference to evaluation cable bolts shear strength
Aziz, N., Mirzaghorbanali, A., Yang, G., Khaleghparast, S. and Rasekh, H.. 2018. "Innovative approach to strata reinforcement in coal mines with reference to evaluation cable bolts shear strength." Journal of Mining and Environment. 9 (3), pp. 703-715. https://doi.org/10.22044/jme.2018.6918.1525Prediction of the main caving span in longwall mining using fuzzy MCDM technique and statistical method
Mohammadi, S., Ataei, M., Khaloo Kakaie, R. and Mirzaghorbanali, A.. 2018. "Prediction of the main caving span in longwall mining using fuzzy MCDM technique and statistical method." Journal of Mining and Environment. 9 (3), pp. 717-726. https://doi.org/10.22044/jme.2018.6715.1492The effects of installation procedure on bond characteristics of fully grouted rock bolts
Ma, Shuji, Aziz, Naj, Nemcik, Jan and Mirzaghorbanali, Ali. 2017. "The effects of installation procedure on bond characteristics of fully grouted rock bolts." Geotechnical Testing Journal. 40 (5), pp. 846-857. https://doi.org/10.1520/GTJ20160239Simulation of macerals effects on methane emission during gas drainage in coal mines
Taheri, Adel, Sereshki, Farhang, Ardejani, Faramarz Doulati and Mirzaghorbanali, Ali. 2017. "Simulation of macerals effects on methane emission during gas drainage in coal mines." Fuel: the science and technology of fuel and energy. 210, pp. 659-665. https://doi.org/10.1016/j.fuel.2017.08.081Shear strength properties of cable bolts using a new double shear instrument, experimental study, and numerical simulation
Mirzaghorbanali, Ali, Rasekh, Haleh, Aziz, Naj, Yang, Guanyu, Khaleghparast, Saman and Nemcik, Jan. 2017. "Shear strength properties of cable bolts using a new double shear instrument, experimental study, and numerical simulation." Tunnelling and Underground Space Technology. 70, pp. 240-253. https://doi.org/10.1016/j.tust.2017.07.018An experimental study on the shear performance of fully encapsulated cable bolts in single shear test
Aziz, Naj, Rasekh, Haleh, Mirzaghorbanali, Ali, Yang, Guanyu, Khaleghparast, Saman and Nemcik, Jan. 2018. "An experimental study on the shear performance of fully encapsulated cable bolts in single shear test." Rock Mechanics and Rock Engineering. 51 (7), pp. 2207-2221. https://doi.org/10.1007/s00603-018-1450-0Effects of cyclic loading on the shear behaviour of infilled rock joints under constant normal stiffness conditions
Mirzaghorbanali, Ali, Nemcik, Jan and Aziz, Naj. 2014. "Effects of cyclic loading on the shear behaviour of infilled rock joints under constant normal stiffness conditions." Rock Mechanics and Rock Engineering. 47 (4), pp. 1373-1391. https://doi.org/10.1007/s00603-013-0452-1Shear strength properties of plain and spirally profiled cable bolts
Aziz, Naj, Mirzaghorbanali, Ali, Nemcik, Jan, Heeman, Kay and Mayer, Stefan. 2015. "Shear strength properties of plain and spirally profiled cable bolts." Canadian Geotechnical Journal. 52 (10), pp. 1490-1495. https://doi.org/10.1139/cgj-2014-0504Vulnerability assessment of urban community and critical infrastructures for integrated flood risk management and climate adaptation strategies
Espada, Rudolf, Apan, Armando and McDougall, Kevin. 2017. "Vulnerability assessment of urban community and critical infrastructures for integrated flood risk management and climate adaptation strategies." International Journal of Disaster Resilience in the Built Environment. 8 (4), pp. 375-411. https://doi.org/10.1108/IJDRBE-03-2015-0010VGI and crowdsourced data credibility analysis using spam email detection techniques
Koswatte, Saman, McDougall, Kevin and Liu, Xiaoye. 2018. "VGI and crowdsourced data credibility analysis using spam email detection techniques." International Journal of Digital Earth: a new journal for a new vision. 11 (5), pp. 520-532. https://doi.org/10.1080/17538947.2017.1341558Semantic location extraction from crowdsourced data
Koswatte, Saman, McDougall, Kevin and Liu, Xiaoye. 2016. "Semantic location extraction from crowdsourced data." 23rd International Society for Photogrammetry and Remote Sensing (ISPRS 2016). Prague, Czech Republic 12 - 19 Jul 2016 Germany. https://doi.org/10.5194/isprsarchives-XLI-B2-543-2016Double shear testing of cable bolts with no concrete face contacts
Rasekh, Haleh, Aziz, Naj, Mirzaghorbanali, Ali, Nemcik, Jan, Li, Xuwei, Yang, Guanyu and Khaleghparast, Saman. 2017. "Double shear testing of cable bolts with no concrete face contacts." Procedia Engineering. 191, pp. 1169-1177. https://doi.org/10.1016/j.proeng.2017.05.292Strength properties of grout for strata reinforcement
Aziz, Naj, Majoor, Dean and Mirzaghorbanali, Ali. 2017. "Strength properties of grout for strata reinforcement." Procedia Engineering. 191, pp. 1178-1184. https://doi.org/10.1016/j.proeng.2017.05.293A new equation for the shear strength of cable bolts incorporating the energy balance theory
Rasekh, Haleh, Mirzaghorbanali, Ali, Nemcik, Jan, Aziz, Naj and Li, Xuwei. 2017. "A new equation for the shear strength of cable bolts incorporating the energy balance theory." Geotechnical and Geological Engineering: an international journal. 35 (4), pp. 1529-1548. https://doi.org/10.1007/s10706-017-0191-2An application of space filling curves to digital terrain models
Scarmana, Gabriel and McDougall, Kevin. 2016. "An application of space filling curves to digital terrain models." Jarosz, Andrew (ed.) 16th International Congress for Mine Surveying: Connecting Education and Industry. Brisbane, Australia 12 - 16 Sep 2016 Brisbane, Australia.Review of Australian land use mapping and land management practice
Baral, Govinda Prasad, McDougall, Kevin and Chong, Albert. 2011. "Review of Australian land use mapping and land management practice." 2011 Surveying and Spatial Sciences Conference: Innovation in Action: Working Smarter (SSSC 2011). Wellington, New Zealand 21 - 25 Nov 2011 Adelaide, Australia.Using volunteered information to map the Queensland floods
McDougall, Kevin. 2011. "Using volunteered information to map the Queensland floods." 2011 Surveying and Spatial Sciences Conference: Innovation in Action: Working Smarter (SSSC 2011). Wellington, New Zealand 21 - 25 Nov 2011 Adelaide, Australia.Comparison of the shear test results of a cable bolt on three laboratory test apparatuses
Li, Xuwei, Aziz, Naj, Mirzaghorbanali, Ali and Nemcik, Jan. 2017. "Comparison of the shear test results of a cable bolt on three laboratory test apparatuses." Tunnelling and Underground Space Technology. 61, pp. 82-89. https://doi.org/10.1016/j.tust.2016.10.003Analytical model of shear behaviour of a fully grouted cable bolt subjected to shearing
Li, Xuwei, Nemcik, Jan, Mirzaghorbanali, Ali, Aziz, Naj and Rasekh, Haleh. 2015. "Analytical model of shear behaviour of a fully grouted cable bolt subjected to shearing." International Journal of Rock Mechanics and Mining Sciences. 80, pp. 31-39. https://doi.org/10.1016/j.ijrmms.2015.09.005Mechanical properties of grouts at various curing times
Mirza, Ali, Aziz, Naj, Ye, Wang and Nemcik, Jan. 2016. "Mechanical properties of grouts at various curing times." Aziz, Naj and Kininmonth, Bob (ed.) 2016 Coal Operators' Conference. Wollongong, Australia 10 - 12 Feb 2016 Wollongong, Australia.Modelling simulation of the performance of cable bolts in shear
Rasekh, Haleh, Mirza, Ali, Aziz, Naj, Nemcik, Jan and Li, Xuwei. 2016. "Modelling simulation of the performance of cable bolts in shear." Aziz, Naj and Kininmonth, Bob (ed.) 2016 Coal Operators' Conference. Wollongong, Australia 10 - 12 Feb 2016 Wollongong, Australia.SDI and crowdsourced spatial information management automation for disaster management
Koswatte, S., McDougall, K. and Liu, X.. 2015. "SDI and crowdsourced spatial information management automation for disaster management." Survey Review. 47 (344), pp. 307-315. https://doi.org/10.1179/1752270615Y.0000000008SDI and crowdsourced spatial information management automation for disaster management
Koswatte, Saman, McDougall, Kevin and Liu, Xiaoye. 2014. "SDI and crowdsourced spatial information management automation for disaster management." FIG Commission 3 Workshop 2014. Bologna, Italy 04 - 07 Nov 2014An elasto-plastic constitutive model for rock joints under cyclic loading and constant normal stiffness conditions
Nemcik, Jan, Mirzaghorbanali, Ali and Aziz, Naj. 2014. "An elasto-plastic constitutive model for rock joints under cyclic loading and constant normal stiffness conditions." Geotechnical and Geological Engineering: an international journal. 32 (2), pp. 321-335. https://doi.org/10.1007/s10706-013-9716-5Effects of shear rate on cyclic loading shear behaviour of rock joints under constant normal stiffness conditions
Mirzaghorbanali, Ali, Nemcik, Jan and Aziz, Naj. 2014. "Effects of shear rate on cyclic loading shear behaviour of rock joints under constant normal stiffness conditions." Rock Mechanics and Rock Engineering. 47 (5), pp. 1931-1938. https://doi.org/10.1007/s00603-013-0453-0A follow up to study the behaviour of cable bolts in shear: experimental study and mathematical modelling
Aziz, Naj, Mirza, Ali, Nemcik, Jan, Rasekh, Haleh and Li, Xuwei. 2016. "A follow up to study the behaviour of cable bolts in shear: experimental study and mathematical modelling." Aziz, Naj and Kininmonth, Bob (ed.) 2016 Coal Operators' Conference. Wollongong, Australia 10 - 12 Feb 2016 Wollongong, Australia.Load transfer characteristics of plain and spiral cable bolts tested in new non rotating pull testing apparatus
Aziz, Naj, Mirza, Ali, Nemcik, Jan, Li, Xuwei, Rasekh, Haleh and Wang, Gaofeng. 2016. "Load transfer characteristics of plain and spiral cable bolts tested in new non rotating pull testing apparatus." Aziz, Naj and Kininmonth, Bob (ed.) 2016 Coal Operators' Conference. Wollongong, Australia 10 - 12 Feb 2016 Wollongong, Australia.Numerical modeling of gas flow in coal pores for methane drainage
Taheri, Adel, Sereshki, Farhang, Ardejani, Faramarz Doulati and Mirzaghorbanali, Ali. 2016. "Numerical modeling of gas flow in coal pores for methane drainage." Journal of Sustainable Mining. 15, pp. 95-99. https://doi.org/10.1016/j.jsm.2016.10.001Behavior of fiber glass bolts, rock bolts and cable bolts in shear
Li, Xuwei, Aziz, Naj, Mirzaghorbanali, Ali and Nemcik, Jan. 2016. "Behavior of fiber glass bolts, rock bolts and cable bolts in shear." Rock Mechanics and Rock Engineering. 49 (7), pp. 2723-2735. https://doi.org/10.1007/s00603-015-0907-7Factors influencing the quality of encapsulation in rock bolting
Aziz, Naj, Craig, Peter, Mirzaghorbanali, Ali and Nemcik, Jan. 2016. "Factors influencing the quality of encapsulation in rock bolting." Rock Mechanics and Rock Engineering. 49 (8), pp. 3189-3203. https://doi.org/10.1007/s00603-016-0973-5An experimental study of axial load transfer mechanisms of cable bolts using axially split embedment apparatus
Mirzaghorbanali, A. and Aziz, N.. 2017. "An experimental study of axial load transfer mechanisms of cable bolts using axially split embedment apparatus." Journal of Mining and Environment. 8 (2), pp. 131-137. https://doi.org/10.22044/jme.2016.732Flood inundation mapping using hydraulic modelling and GIS: a case study in the West Creek sub-catchment
Sahib, Basheer, Liu, Xiaoye and McDougall, Kevin. 2014. "Flood inundation mapping using hydraulic modelling and GIS: a case study in the West Creek sub-catchment." 3rd National Conference on Urban Water Management: Storm water 2014. Adelaide, South Australia 13 - 17 Oct 2014 Australia.ACARP PROJECT C42012 SHEAR TESTING OF THE MAJOR AUSTRALIAN CABLE BOLTS UNDER DIFFERENT PRETENSION LOADS
Aziz, N., Mirzaghorbanali, A., Nemcik, J., Hadi, M. and Remennikov, A.. 2017. ACARP PROJECT C42012 SHEAR TESTING OF THE MAJOR AUSTRALIAN CABLE BOLTS UNDER DIFFERENT PRETENSION LOADS. ACARP.Exploring the application of some common raster scanning paths on lossless compression of elevation images
Scarmana, Gabriel and McDougall, Kevin. 2015. "Exploring the application of some common raster scanning paths on lossless compression of elevation images." IEEE International Geoscience and Remote Sensing Symposium 2015 (IGARSS 2015): Remote Sensing: Understanding the Earth for a Safer World. Milan, Italy 26 - 31 Jul 2015 Milan, Italy.Vulnerability assessment and interdependency analysis of critical infrastructures for climate adaptation and flood mitigation
Espada Jr., Rodolfo, Apan, Armando and McDougall, Kevin. 2015. "Vulnerability assessment and interdependency analysis of critical infrastructures for climate adaptation and flood mitigation." International Journal of Disaster Resilience in the Built Environment. 6 (3), pp. 313-346. https://doi.org/10.1108/IJDRBE-02-2014-0019Spatial modelling of natural disaster risk reduction policies with Markov decision processes
Espada Jr., Rodolfo, Apan, Armando and McDougall, Kevin. 2014. "Spatial modelling of natural disaster risk reduction policies with Markov decision processes." Applied Geography. 53, pp. 284-298. https://doi.org/10.1016/j.apgeog.2014.06.021Characterisation of network objects in natural and anthropic environments
Harris, Bruce, McDougall, Kevin and Barry, Michael. 2014. "Characterisation of network objects in natural and anthropic environments." Dragicevic, S. and Li, S. (ed.) ISPRS Technical Commission II Midterm Symposium 2014. Toronto, Canada 06 - 08 Oct 2014 London, United Kingdom. https://doi.org/10.5194/isprsarchives-XL-2-161-2014The impact of varying statutory arrangements on spatial data sharing and access in regional NRM bodies
Paudyal, D. R., McDougall, K. and Apan, A.. 2014. "The impact of varying statutory arrangements on spatial data sharing and access in regional NRM bodies." ISPRS Annals of the Photogrammetry, Remote Sensing and Spatial Information Sciences. II (8), pp. 193-197. https://doi.org/10.5194/isprsannals-II-8-193-2014The Strength Properties of Fibreglass and Other Polymer-based Dowels for Strata Reinforcement in Coalmines
Aziz, N., Gilbert, D., Nemcik, J., Mirzaghorbanali, A. and Burton, R.. 2015. "The Strength Properties of Fibreglass and Other Polymer-based Dowels for Strata Reinforcement in Coalmines." AusRock 2014. AusIMM.A pyramid approach to lossless data compression of grid-based digital elevation models
Scarmana, Gabriel and McDougall, Kevin. 2014. "A pyramid approach to lossless data compression of grid-based digital elevation models." IEEE International Geoscience and Remote Sensing Symposium 2014 (IGARSS 2014): Energy and our Changing Planet. Quebec City, Canada 13 - 18 Jul 2014 Piscataway, NJ. United States. https://doi.org/10.1109/IGARSS.2014.6946981Effects of shearing direction on shear behaviour of rock joints
Mirzaghorbanali, Ali, Rasekh, Haleh, Aziz, Naj and Nemcik, Jan. 2014. "Effects of shearing direction on shear behaviour of rock joints." Aziz, Naj (ed.) 2014 Coal Operators' Conference. Wollongong, Australia 12 - 14 Feb 2014 Australia.Developing spatial information sharing strategies across natural resource management communities
Paudyal, Dev Raj, McDougall, Kevin and Apan, Armando. 2013. "Developing spatial information sharing strategies across natural resource management communities." Onsrud, Harlan and Rajabifard, Abbas (ed.) Spatial Enablement in Support of Economic Development and Poverty Reduction: Research, Development and Education Perspectives. Needham, MA, USA. GSDI Association Press. pp. 141-168Using spatial modelling to develop flood risk and climate adaptation capacity metrics for vulnerability assessments of urban community and critical water supply infrastructure
Espada Jr., Rodolfo, Apan, Armando and McDougall, Kevin. 2013. "Using spatial modelling to develop flood risk and climate adaptation capacity metrics for vulnerability assessments of urban community and critical water supply infrastructure." Featherstone, Jeffrey (ed.) 49th World Congress of the International Society of City and Regional Planners (ISOCARP 2013): Frontiers of Planning: Evolving and Declining Models of City Planning Practice. Brisbane, Australia 01 - 04 Oct 2013 Netherlands.A comparison between static and dynamic foot mobility magnitude measures
Alshadli, Duaa, Chong, Albert K., Newsham-West, Richard, Al-Baghdadi, Jasim Ahmed Ali, Milburn, Peter and McDougall, Kevin. 2013. "A comparison between static and dynamic foot mobility magnitude measures." Toby, Hung (ed.) 31st International Conference on Biomechanics in Sports (ISBS 2013). Taipei, Taiwan 07 - 11 Jul 2013 Taipei, Taiwan.Human foot plantar surface shape change during gait
AL-Baghdadi, Jasim Ahmed Ali, Chong, Albert K., McDougall, Kevin, Milburn, Peter and Newsham-West, Richard. 2013. "Human foot plantar surface shape change during gait." Toby, Hung (ed.) 31st International Conference on Biomechanics in Sports (ISBS 2013). Taipei, Taiwan 07 - 11 Jul 2013 Taipei, Taiwan.Using spatial modelling to develop flood risk and climate adaptation capacity metrics for assessing urban community and critical electricity infrastructure vulnerability
Espada Jr., R., Apan, A. and McDougall, K.. 2013. "Using spatial modelling to develop flood risk and climate adaptation capacity metrics for assessing urban community and critical electricity infrastructure vulnerability." Piantadosi, J., Anderssen, R. S. and Boland, J. (ed.) 20th International Congress on Modelling and Simulation (MODSIM2013). Adelaide, Australia 01 - 06 Dec 2013 Australia. Modelling and Simulation Society of Australia and New Zealand . https://doi.org/10.36334/modsim.2013.L1.espadaThe global cadastre
McDougall, Kevin, Bennett, Rohan and Molen, Paul van der. 2013. "The global cadastre." GIM International. 27 (7), pp. 30-34.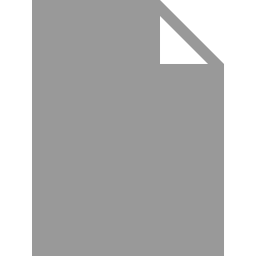