Data-Driven Optimised XGBoost for Predicting the Performance of Axial Load Bearing Capacity of Fully Cementitious Grouted Rock Bolting Systems
Article
Article Title | Data-Driven Optimised XGBoost for Predicting the Performance of Axial Load Bearing Capacity of Fully Cementitious Grouted Rock Bolting Systems |
---|---|
ERA Journal ID | 211776 |
Article Category | Article |
Authors | Jodeiri Shokri, Behshad, Mirzaghorbanali, Ali, McDougall, Kevin, Karunasena, Warna, Nourizadeh, Hadi, Entezam, Shima, Hosseini, Shahab and Aziz, Naj |
Editors | Kim, K.S. |
Journal Title | Applied Sciences |
Journal Citation | 14 |
Article Number | 9925 |
Number of Pages | 26 |
Year | 2024 |
Publisher | MDPI AG |
Place of Publication | Switzerland |
ISSN | 2076-3417 |
Digital Object Identifier (DOI) | https://doi.org/10.3390/app14219925 |
Web Address (URL) | https://www.mdpi.com/2076-3417/14/21/9925 |
Abstract | This article investigates the application of eXtreme gradient boosting (XGBoost) and hybrid metaheuristics optimisation techniques to predict the axial load bearing capacity of fully grouted rock bolting systems. For this purpose, a comprehensive dataset of 72 pull-out tests was built, considering various influential parameters such as three water-to-grout (W/G) ratios, five ranges of curing time (CT), three different grout admixtures with two different fly ash (FA) contents, and two different diameter confinements (DCs). Additionally, to find out the effect of the mechanical behaviour of grouts on the performance of fully grouted rock bolting systems, seventy-two uniaxial compression strength (UCS) samples were cast and tested simultaneously with pull-out samples. The UCS samples were prepared with the same details as the pull-out samples to avoid any inconsistency. The results highlight that peak load values generally increase with longer curing times, lower W/G, and higher UCS and DC values. The main novelty of this paper lies in its data-driven approach, using various XGBoost models. This method offers a time-, cost-, and labour-efficient alternative to traditional experimental methods for predicting rock bolt performance. For this purpose, after building the dataset and dividing it randomly into two training and testing datasets, five different XGBoost models were developed: a standalone XGBoost model and four hybrid models incorporating Harris hawk optimisation (HHO), the jellyfish search optimiser (JSO), the dragonfly algorithm (DA), and the firefly algorithm (FA). These models were subsequently evaluated for their ability to predict peak load values. The results demonstrate that all models effectively predicted peak load values, but the XGBoost-JSO hybrid model demonstrated superior performance, achieving the highest R-squared coefficients of 0.987 and 0.988 for the training and testing datasets, respectively. Sensitivity analysis revealed that UCS values were the most influential parameter, while FA content had the least impact on the maximum peak load values of fully cementitious grouted rock bolts. |
Keywords | fully grouted rock bolt; machine learning; prediction; axial load bearing capacity; XGBoost |
Contains Sensitive Content | Does not contain sensitive content |
ANZSRC Field of Research 2020 | 401902. Geomechanics and resources geotechnical engineering |
400502. Civil geotechnical engineering | |
Byline Affiliations | Centre for Future Materials |
School of Engineering | |
School of Surveying and Built Environment | |
Tarbiat Modares University, Iran | |
University of Wollongong |
https://research.usq.edu.au/item/zq0z5/data-driven-optimised-xgboost-for-predicting-the-performance-of-axial-load-bearing-capacity-of-fully-cementitious-grouted-rock-bolting-systems
Download files
106
total views15
total downloads3
views this month0
downloads this month
Export as
Related outputs
Numerical simulation of a single shear scenario in fibreglass rock bolts
Jodeiri Shokri, Behshad, Entezam, Shima, Gregor, Peter, Nourizadeh, Hadi, Entezam, Alireza, Mirzaghorbanali, Ali, McDougall, Kevin, Karunasena, Karu and Aziz, Naj. 2025. "Numerical simulation of a single shear scenario in fibreglass rock bolts." Aziz, Naj, Mirzaghorbanali, Ali, Hoelle, John, Shan, Zhenjun, Marston, Kevin, Nemcik, Jan, Campbell, Richard and Mottahedi, Adel (ed.) 2025 Resource Operator Conference (ROC2025). University of Southern Queensland 06 - 07 Feb 2025 Australia.Sustainability in underground mining and geotechnical practices
Nourizadeh, Hadi, Entezam, Alireza, Mirzaghorbanali, Ali, Burey, Polly, McDougall, Kevin, Jodeiri Shokri, Behshad, Entezam, Shima, Criag, Peter and Aziz, Naj. 2025. "Sustainability in underground mining and geotechnical practices." Aziz, Naj, Mirzaghorbanali, Ali, Hoelle, John, Shan, Zhenjun, Marston, Kevin, Nemcik, Jan, Campbell, Richard and Mottahedi, Adel (ed.) 2025 Resource Operator Conference (ROC2025). University of Southern Queensland 06 - 07 Feb 2025 Australia.Investigating the rotation of cable bolts during pull out tests
Rastegarmanesh, Ashkan, Mirzaghorbanali, Ali, McDougall, Kevin, Aziz, Naj, Anzanpour, Sina, Nourizadeh, Hadi, Moosavi, Mahdi and Taheri, Abbas. 2025. "Investigating the rotation of cable bolts during pull out tests." Aziz, Naj, Mirzaghorbanali, Ali, Hoelle, John, Shan, Zhenjun, Marston, Kevin, Nemcik, Jan, Campbell, Richard and Mottahedi, Adel (ed.) 2025 Resource Operator Conference (ROC2025). University of Southern Queensland 06 - 07 Feb 2025 Australia.Analysis of wedge stability in the excavation of dam diversion tunnels: a case study
Farhadian, Hadi, Jodeiri Shokri, Behshad, Nourizadeh, Hadi and Mirzaghorbanali, Ali. 2025. "Analysis of wedge stability in the excavation of dam diversion tunnels: a case study ." Aziz, Naj, Mirzaghorbanali, Ali, Hoelle, John, Shan, Zhenjun, Marston, Kevin, Nemcik, Jan, Campbell, Richard and Mottahedi, Adel (ed.) 2025 Resource Operator Conference (ROC2025). University of Southern Queensland 06 - 07 Feb 2025 Australia.Proceedings of the 2025 Resource Operators Conference
Aziz, Naj, Mirzaghorbanali, Ali, Hoelle, John, Shan, Zhenjun, Marston, Kevin, Nemcik, Jan, Campbell, Richard and Mottahedi, Adel. Aziz, Naj, Mirzaghorbanali, Ali, Hoelle, John, Shan, Zhenjun, Marston, Kevin, Nemcik, Jan, Campbell, Richard and Mottahedi, Adel (ed.) 2025. Proceedings of the 2025 Resource Operators Conference . Australia. University of Wollongong.ACARP 34018: Carbolt™: Pre-Commercial Fixed Length Carbolt Prototype
John, David, Peerzada, Mazhar, Mirzaghorbanali, Ali, Jodeiri Shokri, Behshad, Nourizadeh, Hadi, Barnes, Scott and Hoehn, Karsten. 2025. ACARP 34018: Carbolt™: Pre-Commercial Fixed Length Carbolt Prototype. Australia. Australian Coal Association Research Program (ACARP).Analytical model to characterise thermal loads and deformation of internal replacement pipe systems
Ahmadi, Hamid, Manalo, Allan, Kiriella, Shanika, Karunasena, Warna, O'Rourke, Thomas D. and Wham, Brad P.. 2025. "Analytical model to characterise thermal loads and deformation of internal replacement pipe systems." Thin-Walled Structures. 209. https://doi.org/10.1016/j.tws.2025.112935Influence of bonding level on the bending fatigue behaviour of internal replacement pipe systems
Kiriella, Shanika, Manalo, Allan, Tien, Cam M. T., Ahmadi, Hamid, Karunasena, Warna, Dixon, Patrick G., Salah, Ahmad and Wham, Brad P.. 2025. "Influence of bonding level on the bending fatigue behaviour of internal replacement pipe systems." Steel and Composite Structures: an international journal. 54 (2), pp. 175-190. https://doi.org/10.12989/scs.2025.54.2.175Performance of the corrugated metal pipe sliplining method using FRP pipes
Premasuriya, Aluth, Lokuge, Weena, Karunasena, Warna and Manalo, Allan C.. 2025. "Performance of the corrugated metal pipe sliplining method using FRP pipes ." Tunnelling and Underground Space Technology. 162. https://doi.org/10.1016/j.tust.2025.106603A Critical Review on Development of Laboratory Testing Technologies of Tendons Applied to Stabilize Underground Structures
Mottahedi, Adel, Aziz, Naj, Remennikov, Alex, Mirzaghorbanali, Ali and Marston, Kevin. 2025. "A Critical Review on Development of Laboratory Testing Technologies of Tendons Applied to Stabilize Underground Structures ." Rock Mechanics and Rock Engineering. https://doi.org/10.1007/s00603-025-04518-4Impact of Diverse Parameters on CO2 Adsorption in CO2 Sequestration: Utilizing a Novel Triaxial Testing Apparatus
Ardehjani, Emad Ansari, Ataei, Mohammad, Sereshki, Farhang, Mirzaghorbanali, Ali and Aziz, Naj. 2025. "Impact of Diverse Parameters on CO2 Adsorption in CO2 Sequestration: Utilizing a Novel Triaxial Testing Apparatus ." Greenhouse Gases: science and technology. 15 (1), pp. 53-67. https://doi.org/10.1002/ghg.2322Unveiling Axial Load Transfer Mechanism in Fully Encapsulated Rock Bolts
Nourizadeh, Hadi, Mirzaghorbanali, Ali, McDougall, Kevin, Mitri, Hani, Craig, Peter, Rastegarmanesh, Ashakan and Aziz, Naj. 2025. "Unveiling Axial Load Transfer Mechanism in Fully Encapsulated Rock Bolts ." Rock Mechanics and Rock Engineering. https://doi.org/10.1007/s00603-024-04347-xPerformance of Cable Bolts in Small- and Large-Scale Laboratory Pullout Tests
Rastegarmanesh, Ashkan, Mirzaghorbanali, Ali, McDougall, Kevin, Aziz, Naj, Anzanpour, Sina and Nourizadeh, Hadi. 2025. "Performance of Cable Bolts in Small- and Large-Scale Laboratory Pullout Tests." Geotechnical and Geological Engineering: an international journal. 43 (67). https://doi.org/10.1007/s10706-024-03019-5Exploring the axial performance of protective sheathed rock bolts through large-scale testing
Nourizadeh, Hadi, Mirzaghorbanali, Ali, McDougall, Kevin and Aziz, Naj. 2025. "Exploring the axial performance of protective sheathed rock bolts through large-scale testing." Tunnelling and Underground Space Technology. 155 (1). https://doi.org/10.1016/j.tust.2024.106157Evaluation of Dozer Bund Design for Improved Safety in Saraji Open Cut Coal Mines (BHP Group Limited)
Mirzaghorbanali, Ali, Nourizadeh, Hadi and Jodeiri Shokri, Behshad. 2024. Evaluation of Dozer Bund Design for Improved Safety in Saraji Open Cut Coal Mines (BHP Group Limited). Australia. University of Southern Queensland. https://doi.org/10.26192/zx4q6Potential Utilisation of Organic Waste in Concrete at OT Mine: Exploring Mechanical Properties of Amended Concrete (Phase 2)
Mirzaghorbanali, Ali, McDougall, Kevin, Nourizadeh, Hadi, Karunasena, Karu, Trzcinski, Antoine, Entezam, Alireza and Ganthavee, Voravich. 2024. Potential Utilisation of Organic Waste in Concrete at OT Mine: Exploring Mechanical Properties of Amended Concrete (Phase 2). Australia. University of Southern Queensland. https://doi.org/10.26192/zx4q2Investigation of Debonding Effect in Internal Replacement Pipe System Under Lateral Loading
Tien, Tri C. M., Manalo, Allan, Dixon, Patrick, Karunasena, Warna, Ahmadi, Hamid, Kiriella, Shanika, Salah, Ahmad and Wham, Brad P.. 2024. "Investigation of Debonding Effect in Internal Replacement Pipe System Under Lateral Loading." Applied Sciences. 14 (22). https://doi.org/10.3390/app142210509Effect of internal pressure on the flexural fatigue behaviour of trenchless internal replacement pipe systems
Kiriella, Shanika, Manalo, Allan, Tien, Cam Minh Tri, Ahmadi, Hamid, Karunasena, Warna, Dixon, Patrick G., Salah, Ahmad and Wham, Brad P.. 2024. "Effect of internal pressure on the flexural fatigue behaviour of trenchless internal replacement pipe systems." Tunnelling and Underground Space Technology. 154. https://doi.org/10.1016/j.tust.2024.106111Impact Loading of Tendon Reinforcement Systems Used for Ground Control: A Critical Literature Review
Mottahedi, Adel, Aziz, Naj, Remennikov, A;ex, Mirzaghorbanali, Ali and Marston, Kevin. 2024. "Impact Loading of Tendon Reinforcement Systems Used for Ground Control: A Critical Literature Review." Archive of Mining Science. 69 (4), pp. 711-743. https://doi.org/10.24425/ams.2024.1525Digital Twin Technology in Built Environment: A Review of Applications, Capabilities and Challenges
Mousavi, Yalda, Gharineiat, Zahra, Agha Karimi, Armin Agha, McDougall, Kevin, Rossi, Adriana and Barsanti, Sara Gonizzi. 2024. "Digital Twin Technology in Built Environment: A Review of Applications, Capabilities and Challenges." Smart Cities. 7 (5), pp. 2594-2615. https://doi.org/10.3390/smartcities7050101Granular Soils and Contaminant Modeling in Tailing Dams
Farhadian, Hadi, Jodeiri Shokri, Behshad and Mirzaghorbanali, Ali. 2024. "Granular Soils and Contaminant Modeling in Tailing Dams." Minerals. 14 (11).Examining the Impact of Coal Gas Emissions on the Stability Analysis of Coal Pillars: A Critical Literature Review
Ardehjani,Emad Ansari, Ataei, Mohammad, Sereshki, Farhang, Mirzaghorbanali, Ali and Aziz, Naj. 2024. "Examining the Impact of Coal Gas Emissions on the Stability Analysis of Coal Pillars: A Critical Literature Review." Mining-Geology-Petroleum Engineering Bulletin. 39 (3), pp. 77-94. https://doi.org/10.17794/rgn.2024.3.7Small scale laboratory monotonic and cyclic pull out testing on grout and resin encapsulated cable bolts
Rastegarmanesh, Ashkan, Mirzaghorbanali, Ali, McDougall, Kevin, Aziz, Naj, Anzanpour, Sina, Nourizadeh, Hadi and Moosavi, Mahdi. 2024. "Small scale laboratory monotonic and cyclic pull out testing on grout and resin encapsulated cable bolts ." International Journal of Rock Mechanics and Mining Sciences. 183. https://doi.org/10.1016/j.ijrmms.2024.105914Analysing Double Shearing Mechanism in Fiberglass Rock Bolting Systems: a Comprehensive Analytical Model and Numerical Simulation Approach
Gregor, Peter, Mirzaghorbanali, Ali, McDougall, Kevin, Aziz, Naj, Jodeiri Shokri, Behshad, Nourizadeh, Hadi and Taheri, Abbas. 2024. "Analysing Double Shearing Mechanism in Fiberglass Rock Bolting Systems: a Comprehensive Analytical Model and Numerical Simulation Approach." Geotechnical and Geological Engineering: an international journal. 42 (8), pp. 7339-7370. https://doi.org/10.1007/s10706-024-02929-8Predicting axial-bearing capacity of fully grouted rock bolting systems by applying an ensemble system
Hosseini, Shahab, Jodeiri Shokri, Behshad, Mirzaghorbanali, Ali, Nourizadeh, Hadi, Entezam, Shima, Motallebiyan, Amin, Entezam, Alireza, McDougall, Kevin, Karunasena, Warna and Aziz, Naj. 2024. "Predicting axial-bearing capacity of fully grouted rock bolting systems by applying an ensemble system." Soft Computing. 28, pp. 10491-10518. https://doi.org/10.1007/s00500-024-09828-3Predicting grout’s uniaxial compressive strength (UCS) for fully grouted rock bolting system by applying ensemble machine learning techniques
Hosseini, Shahab, Jodeiri Shokri, Behshad, Entezam, Shima, Mirzaghorbanali, Ali, Nourizadeh, Hadi, Motallebiyan, Amin, Entezam, Alireza, McDougall, Kevin, Karunasena, Warna and Aziz, Naj. 2024. "Predicting grout’s uniaxial compressive strength (UCS) for fully grouted rock bolting system by applying ensemble machine learning techniques." Neural Computing and Applications. 36, pp. 18387-18412. https://doi.org/10.1007/s00521-024-10128-yLinear and nonlinear axial behaviour of internal replacement pipe systems for pipeline rehabilitation
Ahmadi, Hamid, Salah, Ahmad, Manalo, Allan, Dixon, Patrick G., Karunasena, Warna, Karunasena, Warna, Tien, Cam Minh Tri, Kiriella, Shanika, O’Rourke, Thomas D. and Wham, Brad P.. 2024. "Linear and nonlinear axial behaviour of internal replacement pipe systems for pipeline rehabilitation." Structure and Infrastructure Engineering: maintenance, management, life-cycle design and performance. https://doi.org/10.1080/15732479.2024.2356673Temperature change-induced linear and nonlinear axial responses of internal replacement pipe (IRP) systems for pipeline rehabilitation incorporating the effects of soil friction
Ahmadi, Hamid, Manalo, Allan, Dixon, Patrick G., Salah, Ahmad, Karunasena, Warna, Tien, Cam Minh Tri, Kiriella, Shanika, O’Rourke, Thomas D. and Wham, Brad P.. 2024. "Temperature change-induced linear and nonlinear axial responses of internal replacement pipe (IRP) systems for pipeline rehabilitation incorporating the effects of soil friction." Structures. 62. https://doi.org/10.1016/j.istruc.2024.106247Axial Load Transfer Mechanism in Fully Grouted Rock Bolting System: A Systematic Review
Jodeiri Shokri, Behshad, Mirzaghorbanali, Ali, Nourizadeh, Hadi, McDougall, Kevin, Karunasena, Warna, Aziz, Naj, Entezam, Shima and Entezam, Alireza. 2024. "Axial Load Transfer Mechanism in Fully Grouted Rock Bolting System: A Systematic Review ." Applied Sciences. 14 (12). https://doi.org/10.3390/app14125232Aspects of testing tendon supports for strength, effective installation, and performance in different ground formation
Mottahedi, Adel, Aziz, Naj, Mirzaghorbanali, Ali, Remmenikov, Alex and Anzanpour, Sina. 2024. "Aspects of testing tendon supports for strength, effective installation, and performance in different ground formation." Aziz, N. and Mirzaghorbanali, A. (ed.) 2024 Resource Operators Conference (ROC2024). Wollongong, Australia 13 - 16 Feb 2024 Australia. University of Wollongong.Application of waste glass powder in cemenitious grouts
Entezam, Alireza, Nourizadeh, Hadi, Mirzaghorbanali, Ali, Burey, Polly, Shelley, Tristan and Aziz, Naj. 2024. "Application of waste glass powder in cemenitious grouts." Aziz, N. and Mirzaghorbanali, A. (ed.) 2024 Resource Operators Conference (ROC2024). Wollongong, Australia 13 - 16 Feb 2024 Australia. University of Wollongong.Finite element modelling of fully encapsulated cable bolts in laboratory large scale pull out test
Mirzaghorbanali, Ashkan, Rastegarmanesh, Ali, McDougall, Kevin, Aziz, Naj, Anzanpour, Sina and Nourizadeh, Hadi. 2024. "Finite element modelling of fully encapsulated cable bolts in laboratory large scale pull out test." Aziz, N. and Mirzaghorbanali, A. (ed.) 2024 Resource Operators Conference (ROC2024). Wollongong, Australia 13 - 16 Feb 2024 Australia. University of Wollongong.Investigating the axial and shear performance of fiberglass rock boltss
Entezam, Shima, Jodeiri Shokri, Behshad, Nourizadeh, Hadi, Gregor, Peter, Entezam, Alireza, Mirzaghorbanali, Ali, McDougall, Kevin, Karunasena, Karu and Aziz, Naj. 2024. "Investigating the axial and shear performance of fiberglass rock boltss." Aziz, N. and Mirzaghorbanali, A. (ed.) 2024 Resource Operators Conference (ROC2024). Wollongong, Australia 13 - 16 Feb 2024 Australia. University of Wollongong.Finite element simulation of fully grouted rock bolts behaviour across varied bore hole diameters
Jodeiri Shokri, Behshad, Mirzaghorbanali, Ali, Karunasena, Waru, McDougall, Kevin, Aziz, Naj, Entezam, Shima, Nourizadeh, Hadi, Motallebiyan, Amin and Entezam, Alireza. 2024. "Finite element simulation of fully grouted rock bolts behaviour across varied bore hole diameters." Aziz, N. and Mirzaghorbanali, A. (ed.) 2024 Resource Operators Conference (ROC2024). Wollongong, Australia 13 - 16 Feb 2024 Australia. University of Wollongong.Identifying the Impact Factors on the Land Market in Nepal from Land Use Regulation
Subedi, Nab Raj, McDougall, Kevin and Paudyal, Dev Raj. 2024. "Identifying the Impact Factors on the Land Market in Nepal from Land Use Regulation." Urban Science. 8 (2). https://doi.org/10.3390/urbansci8020058Mapping Erosion Hotspots: Coherent Change Detection in the Quilpie Region, Queensland, Australia
Cook, Kyran, Agha Karimi, Armin, Grinham, Alistair and McDougall, Kevin. 2024. "Mapping Erosion Hotspots: Coherent Change Detection in the Quilpie Region, Queensland, Australia." Remote Sensing. 16 (7). https://doi.org/10.3390/rs16071263Bending fatigue behaviour of internal replacement pipe systems
Kiriella, Shanika, Manalo, Allan, Tien, Cam Minh Tri, Ahmadi, Hamid, Dixon, Patrick G., Karunasena, Warna, Salah, Ahmad and Wham, Brad P.. 2024. "Bending fatigue behaviour of internal replacement pipe systems." Composite Structures. 331. https://doi.org/10.1016/j.compstruct.2024.117910A look at the performance of barrel and wedge assembly in cable bolts applications
Rastegarmanesh, Ashkan, Mirzaghorbanali, Ali, McDougall, Kevin, Aziz, Naj, Anzanpour, Sina, Nourizadeh, Hadi and Taheri, Abbas. 2024. "A look at the performance of barrel and wedge assembly in cable bolts applications ." Scientific Reports. 14. https://doi.org/10.1038/s41598-024-54999-6Qualitative investigations into floodways under extreme flood loading
Greene, Isaac Samuel, Gunasekara, Chamila, Lokuge, Weena and Karunasena, Warna. 2024. "Qualitative investigations into floodways under extreme flood loading." Journal of Flood Risk Management. 17 (2). https://doi.org/10.1111/jfr3.12967Failure characterization of fully grouted rock bolts under triaxial testing
Nourizadeh, Hadi, Mirzaghorbanali, Ali, Serati, Mehdi, Mahmoud, Mutaz El-Amin, McDougall, Kevin and Aziz, Naj. 2024. "Failure characterization of fully grouted rock bolts under triaxial testing." Journal of Rock Mechanics and Geotechnical Engineering. 16 (3), pp. 778-789. https://doi.org/10.1016/j.jrmge.2023.08.013Investigating shear behaviour of fibreglass rock bolts reinforcing infilled discontinuities for various pretension loads
Gregor, Peter, Mirzaghorbanali, Ali, McDougall, Kevin, Aziz, Naj and Jodeiri Shokri, Behshad. 2024. "Investigating shear behaviour of fibreglass rock bolts reinforcing infilled discontinuities for various pretension loads." Canadian Geotechnical Journal. 61 (3). https://doi.org/10.1139/cgj-2022-0619Potential utilisation of organic waste in concrete at OT mine: Project Progress Report – Phase 1
Mirzaghorbanali, Ali, McDougall, Kevin, Karunasena, Karu, Trzcinski, Antoine, Eberhard, Susette, Nourizadeh, Hadi and Weerasundara, Lakshika. 2023. Potential utilisation of organic waste in concrete at OT mine: Project Progress Report – Phase 1 . Australia. University of Southern Queensland. https://doi.org/10.26192/zx49zA comprehensive study on the failure mechanisms and integrity of sheath protected rock bolts when subject to axial deformation
Mirzaghorbanali, Ali and Nourizadeh, Hadi. 2023. A comprehensive study on the failure mechanisms and integrity of sheath protected rock bolts when subject to axial deformation . Australia. University of Southern Queensland. https://doi.org/10.26192/zx486Testing procedure development and preliminary testing of sheath protected rock bolt subject to axial loading
Mirzaghorbanali, Ali and Nourizadeh, Hadi. 2023. Testing procedure development and preliminary testing of sheath protected rock bolt subject to axial loading. Australia. University of Southern Queensland. https://doi.org/10.26192/zx484Development of new eco-friendly grout products using waste materials for sustainable mining and construction
Nourizadeh, Hadi. 2023. "Development of new eco-friendly grout products using waste materials for sustainable mining and construction." Entezam, A., Mirzaghorbanali, A., Burey, P. and Shelley, T. (ed.) Climate Smart Engineering 2023. Melbourne, Australia 29 - 30 Nov 2023A simplified theoretical prediction of lateral deformation behaviour of structural pipe repair systems
Kiriella, Shanika, Manalo, Allan, Tien, , Cam Minh Tri, Karunasena, Warna, Ahmadi, Hamid, Salah, Ahmad, Dixon, Patrick, Wham, Brad P. and O'Rourke, Thomas D.. 2023. "A simplified theoretical prediction of lateral deformation behaviour of structural pipe repair systems." No-Dig Down Under 2023 Conference. Brisbane, Australia 12 - 14 Sep 2023 Australia.Axial load transfer mechanism of rock bolts
Nourizadeh, Hadi. 2023. Axial load transfer mechanism of rock bolts. PhD by Publication Doctor of philosophy. University of Southern Queensland. https://doi.org/10.26192/z4w00Effects of the legacy pipe ends on the behaviour of pipe-in-pipe repair systems under internal pressure
Tien, C.M.T., Manalo, A., Dixon, P., Tafsirojjaman, T., Karunasena, W., Flood, W.W., Ahmadi, H., Kiriella, S., Salah, A. and Wham, B.P.. 2023. "Effects of the legacy pipe ends on the behaviour of pipe-in-pipe repair systems under internal pressure." Engineering Failure Analysis. 144. https://doi.org/10.1016/j.engfailanal.2022.106957Lateral deformation behaviour of structural internal replacement pipe repair systems
Kiriella, Shanika, Manalo, Allan, Tien, Cam Minh Tri, Ahmadi, Hamid, Wham, Brad P., Salah, Ahmad, Tafsirojjaman, T., Karunasena, Warna, Dixon, Patrick and O'Rourke, Thomas D.. 2023. "Lateral deformation behaviour of structural internal replacement pipe repair systems." Composite Structures. 319, p. 117144. https://doi.org/10.1016/j.compstruct.2023.117144Maximizing the Use of Out-of-Grade Hybrid Pine in Engineered Wood Products: Bond Performance, the Effect of Resin Streaking, Knots, and Pith
Cherry, Rebecca, Karunasena, Warna and Manalo, Allan. 2023. "Maximizing the Use of Out-of-Grade Hybrid Pine in Engineered Wood Products: Bond Performance, the Effect of Resin Streaking, Knots, and Pith." Forests. 14 (9), pp. 1916-1947. https://doi.org/10.3390/f14091916From we ask to iASK: a self-reflection strategy enabling students to connect assessment and employability
Spagnoli, Dino, Kinash, Shelley, Jorre de st Jorre, Trina, Male, Sally, Mouat, Clare M. and McDougall, Kevin. 2023. "From we ask to iASK: a self-reflection strategy enabling students to connect assessment and employability." Journal of Teaching and Learning for Graduate Employability. 14 (1), pp. 71-87. https://doi.org/10.21153/jtlge2023vol14no1art1654An effective high-order five-point stencil, based on integrated-RBF approximations, for the first biharmonic equation and its applications in fluid dynamics
Mai-Duy, Nam, Tien, Cam Minh Tri, Strunin, Dmitry and Karunasena, Karu. 2023. "An effective high-order five-point stencil, based on integrated-RBF approximations, for the first biharmonic equation and its applications in fluid dynamics." International Journal of Numerical Methods for Heat and Fluid Flow. 33 (7), pp. 2593-2616. https://doi.org/10.1108/HFF-11-2022-0673Shear Behaviour of Fibreglass Rock Bolts for Various Pretension Loads
Gregor, Peter, Mirzaghorbanali, Ali, McDougall, Kevin, Aziz, Naj and Jodeiri Shokri, Behshad. 2023. "Shear Behaviour of Fibreglass Rock Bolts for Various Pretension Loads." Rock Mechanics and Rock Engineering. 56 (11), pp. 8083-8113. https://doi.org/10.1007/s00603-023-03474-1An Alternative Method for Estimating the Peak Flow for a Regional Catchment Considering the Uncertainty via Continuous Simulation
Brown, Ian, McDougall, Kevin, Chadalavada, Sreeni and Alam, Md Jahangir. 2023. "An Alternative Method for Estimating the Peak Flow for a Regional Catchment Considering the Uncertainty via Continuous Simulation ." Water. 15 (19). https://doi.org/10.3390/w15193355Development of sustainable concrete using recycled polyethylene terephthalate (PET) granules as fine aggregate
Eyni Kangavar, Mohammad, Lokuge, Weena, Manalo, Allan, Karunasena, Warna and Ozbakkaloglu, Togay. 2023. "Development of sustainable concrete using recycled polyethylene terephthalate (PET) granules as fine aggregate ." Developments in the Built Environment. 15. https://doi.org/10.1016/j.dibe.2023.100192Characterization of mechanical and bonding properties of anchoring resins under elevated temperature
Nourizadeh, Hadi, Mirzaghorbanali, Ali, McDougall, Kevin, Jeewantha, L.H.J., Craig, Peter, Motallebiyan, Amin, Jodeiri Shokri, Behshad Jodeiri, Rastegarmanesh, Ashakan and Aziz, Naj. 2023. "Characterization of mechanical and bonding properties of anchoring resins under elevated temperature." International Journal of Rock Mechanics and Mining Sciences. 170. https://doi.org/10.1016/j.ijrmms.2023.105506Axial Response of Resin Encapsulated Cable Bolts in Monotonic and Cyclic Loading
Rastegarmanesh, Ashkan, Mirzaghorbanali, Ali, McDougall, Kevin, Aziz, Naj, Anzanpour, Sina, Nourizadeh, Hadi and Moosavi, Mahdi. 2023. "Axial Response of Resin Encapsulated Cable Bolts in Monotonic and Cyclic Loading ." Canadian Geotechnical Journal. 60 (11). https://doi.org/10.1139/cgj-2022-0379Assessing the relationship between tree dimensions in an urban environment
Scarmana, G. and McDougall, K.. 2023. "Assessing the relationship between tree dimensions in an urban environment ." 12th International Symposium on Mobile Mapping Technology (MMT 2023). Padua, Italy 24 - 26 May 2023 Italy. https://doi.org/10.5194/isprs-archives-XLVIII-1-W1-2023-437-2023Construction and Deconstruction: Materials and substances from 'waste'
Burey, Paulomi, Feldman, Jessica, Seligmann, Hannah, Song, Eric, Flynn, Matthew, Helwig, Andreas, Gharineiat, Zahra, Seneviratne, Dinuki, Whiteside, Eliza, Shelley, Tristan, Priesler, Nils, Manalo, Allan, Mirzaghorbanali, Ali, Nourizadeh, Hadi, Roberts, Michae, Nicol, Rose, Redmond, Petrea, Lynch, Mark, Dearnaley, John, ..., Germon, Geoff. 2023. "Construction and Deconstruction: Materials and substances from 'waste'." Chemistry in Australia. (June-August 2023), pp. 16-21.Shear strength properties of clean and clay infilled rock joints: an analysis of the impast of moisture content under CNL conditions
Downing, Elizabeth, Mirzaghorbanali, Ali and Aziz, Naj. 2023. "Shear strength properties of clean and clay infilled rock joints: an analysis of the impast of moisture content under CNL conditions." Aziz, Naj, Marston, Kevin and Mirzaghorbanali, Ali (ed.) 2023 Resource Operators conference (ROC2023). Wollongong, Australia 09 - 10 Feb 2023 Australia. University of Wollongong.Investigation of the Effect of Using Fly Ash in the Grout Mixture on Performing the Fully Grouted Rock Bolt Systems
Entezam, Shima, Jodeiri Shokri, Behshad, Nourizadeh, Hadi, Motallebiyan, Amin, Mirzaghorbanali, Ali, McDougall, Kevin, Aziz, Naj and Karunasena, Karu. 2023. "Investigation of the Effect of Using Fly Ash in the Grout Mixture on Performing the Fully Grouted Rock Bolt Systems." Aziz, Naj, Marston, Kevin and Mirzaghorbanali, Ali (ed.) 2023 Resource Operators conference (ROC2023). Wollongong, Australia 09 - 10 Feb 2023 Australia. University of Wollongong.Substitution of Recycled Plastic Aggregates (RPA) in concrete and its influence on pullout capacity of mechanical and chemical anchors
Akhtar, Raza, Skellern, Graham and Mirzaghorbanali, Ali. 2023. "Substitution of Recycled Plastic Aggregates (RPA) in concrete and its influence on pullout capacity of mechanical and chemical anchors." Aziz, Naj, Manson, Kevin and Mirzaghorbanali, Ali (ed.) 2023 Resource Operators conference (ROC2023). Wollongong, Australia 09 - 10 Feb 2023 Australia. University of Wollongong.SPT-CPT Correlation in Southeast Queensland, Australia
Faivre, Yohanne, Mirzaghorbanali, Ali, Nourizadeh, Hadi, Jodeiri Shokri, Behshad, McDougall, Kevin and Aziz, Naj. 2023. "SPT-CPT Correlation in Southeast Queensland, Australia." Aziz, Naj, Marston, Kevin and Mirzaghorbanali, Ali (ed.) 2023 Resource Operators conference (ROC2023). Wollongong, Australia 09 - 10 Feb 2023 Australia. University of Wollongong.The effect of changing confinement diameter on axial load transfer mechanisms of fully grouted rock bolts
Jodeiri Shokri, Behshad Jodeiri, Entezam, Shima, Nourizadeh, Hadi, Motallebiyan, Amin, Mirzaghorbanali, Ali, McDougall, Kevin, Aziz, Naj and Karunasena, Karu. 2023. "The effect of changing confinement diameter on axial load transfer mechanisms of fully grouted rock bolts." Aziz, Naj, Marston, Kevin and Mirzaghorbanali, Ali (ed.) 2023 Resource Operators conference (ROC2023). Wollongong, Australia 09 - 10 Feb 2023 University of Wollongong. University of Wollongong.Finite Element Numerical Modelling of Rock Bolt Axial Behaviour Subject to Different Geotechnical Conditions
Nourizadeh, Hadi, Mirzaghorbanali, Ali, Aziz, Naj, McDougall, Kevin, Jodeiri Shokri, Behshad, Sahebi, Akbar, Motallebiyan, Amin and Entezam, Shima. 2023. "Finite Element Numerical Modelling of Rock Bolt Axial Behaviour Subject to Different Geotechnical Conditions." Aziz, Naj, Marston, Kevin and Mirzaghorbanali, Ali (ed.) 2023 Resource Operators conference (ROC2023). Wollongong, Australia 09 - 10 Feb 2023 Australia. University of Wollongong.Application of numerical modeling in study of the behaviour of cable bolts under axial loading
Anzanpour, Sina, Aziz, Naj, Topa, Ameen, Nemcik, Jan and Mirzaghorbanali, Ali. 2023. "Application of numerical modeling in study of the behaviour of cable bolts under axial loading." Aziz, Naj, Marston, Kevin and Mirzaghorbanali, Ali (ed.) 2023 Resource Operators conference (ROC2023). Wollongong, Australia 09 - 10 Feb 2023 Australia. University of Wollongong.Effects of rib distances on axial load transfer mechanisms of fully grouted rock bolts
Nourizadeh, Hadi, Motallebiyan, Amin, Jodeiri Shokri, Behshad, Entezam, Shima, Mirzaghorbanali, Ali, Aziz, Naj and McDougall, Kevin. 2023. "Effects of rib distances on axial load transfer mechanisms of fully grouted rock bolts." Aziz, Naj, Marston, Kevin and Mirzaghorbanali, Ali (ed.) 2023 Resource Operators conference (ROC2023). Wollongong, Australia 09 - 10 Feb 2023 Australia. University of Wollongong.Investigating the effect of coal macerals on the recovery rate of the flotation process- a case study
Jodeiri Shokri, Behshad, Entezam, Shima, Entezam, Alireza and Mirzaghorbanali, Ali. 2023. "Investigating the effect of coal macerals on the recovery rate of the flotation process- a case study." Aziz, Naj, Marston, Kevin and Mirzaghorbanali, Ali (ed.) 2023 Resource Operators conference (ROC2023). Wollongong, Australia 09 - 10 Feb 2023 Australia. University of Wollongong.Crowd-Assisted Flood Disaster Management
Koswatte, S., McDougall, K. and Liu, X.. 2023. "Crowd-Assisted Flood Disaster Management." Singh, Vijay P., Yadav, Shalini, Yadac, Krishna Kumar, Corzo, Gerald Augusto, Munoz-Arriola, Francisco and Yadava, Ram Narayan (ed.) Application of Remote Sensing and GIS in Natural Resources and Built Infrastructure Management. Switzerland. Springer. pp. 39-55Using an inventory cluster approach for assessing bushfire preparedness and information needs in vulnerable communities
Ryan, Barbara, King, Rachel, Lokuge, Weena, Karunasena, Warna and Anderson, Esther. 2023. "Using an inventory cluster approach for assessing bushfire preparedness and information needs in vulnerable communities." Natural Hazards. 115, p. 1697–1714. https://doi.org/10.1007/s11069-022-05614-2A new high-order nine-point stencil, based on integrated-RBF approximations, for the first biharmonic equation
Mai-Duy, N., Strunin, D. and Karunasena, W.. 2022. "A new high-order nine-point stencil, based on integrated-RBF approximations, for the first biharmonic equation." Engineering Analysis with Boundary Elements. 143, pp. 687-699. https://doi.org/10.1016/j.enganabound.2022.07.014Laboratory study of the behaviour of grouted cable bolts under static and dynamic axial loading
Anzanpour, S., Aziz, N., Remennikov, A., Nemcik, J., Mirzaghorbanali, A. and Rastegarmanesh, A.. 2022. "Laboratory study of the behaviour of grouted cable bolts under static and dynamic axial loading." Eurock 2022: Rock and Fracture Mechanics in Rock Engineering and Mining. Espoo, Finland 12 - 15 Sep 2022 Finland.Markov-based deterioration prediction and asset management of floodway structures
Tran, Huu, Lokuge, Weena, Karunasena, Warna and Setunge, Sujeeva. 2022. "Markov-based deterioration prediction and asset management of floodway structures." Sustainable and Resilient Infrastructure. 7 (6), pp. 789-802. https://doi.org/10.1080/23789689.2022.2067950Use of time-lapse 2D and 3D geoelectrical inverse models for monitoring acid mine drainage - a case study
Shokri, Behshad Jodeiri, Shafaei, Foojan, Ardejani, Faramarz Doulati, Mirzaghorbanali, Ali and Entezam, Shima. 2023. "Use of time-lapse 2D and 3D geoelectrical inverse models for monitoring acid mine drainage - a case study." Soil and Sediment Contamination: an international journal. 32 (4), pp. 376-399. https://doi.org/10.1080/15320383.2022.2090895Static and dynamic tendon pull-out test research at the University of Wollongong
Anzanpour, Sina, Aziz, Naj, Nemcik, Naj, Remennikov, Alex, Mirzaghorbanali, Ali and Wallace, Jordan. 2022. "Static and dynamic tendon pull-out test research at the University of Wollongong." Aziz, Naj and Mirzaghorbanali, Ali (ed.) 2022 Resource Operators Conference (ROC2022). Australia Feb 2022 Australia.Crack damage evolution in coal at elevated temperatures
Serati, Mehdi, Kwan, King Ho Benny, Gordon, Nick, Moravej, Sasan, Bahaaddini, Mojtaba and Mirzaghorbanali, Ali. 2022. "Crack damage evolution in coal at elevated temperatures." Aziz, Naj and Mirzaghorbanali, Ali (ed.) 2022 Resource Operators Conference (ROC2022). Australia Feb 2022 Wollongong, Australia.Development of a wireless system to measure the strain/deformation of rock bolts
Nourizadeh, Hadi, Mirzaghorbanali, Ali, Aziz, Naj, McDougall, Kevin and Sahebi, Ali Akbar. 2022. "Development of a wireless system to measure the strain/deformation of rock bolts." Aziz, Naj and Mirzaghorbanali, Ali (ed.) 2022 Resource Operators Conference (ROC2022). Australia Feb 2022 Australia.Application of a geographic information system (GIS) for selecting the location of a coal wastes dump - a case study
Shokri, Behshad Jodeiri, Ardejani, Faramarz Doulati, Bagheri, Mehdi, Entezam, Shima, Mirzaghorbanali, Ali, McDougall, Kevin and Aziz, Naj. 2022. "Application of a geographic information system (GIS) for selecting the location of a coal wastes dump - a case study." Aziz, Naj and Mirzaghorbanali, Ali (ed.) 2022 Resource Operators Conference (ROC2022). Australia Feb 2022 Australia.Shear behavior of clayey infilled rock joints having triangular and sinusoidal asperities
Mirzaghorbanali, Ali, Ghimire, Basanta, Rastegarmanesh, Ashkan, Nourizadeh, Hadi, McDougall, Kevin and Aziz, Naj. 2022. "Shear behavior of clayey infilled rock joints having triangular and sinusoidal asperities." Aziz, Naj and Mirzaghorbanali, Ali (ed.) 2022 Resource Operators Conference (ROC2022). Australia Feb 2022 Australia.Predicting the pyrite oxidation process within coal waste piles using multiple linear regression (MLR) and teaching-learning-based optimization (TLBO) algorithm
Entezam, Shima, Shokri, Behshad Jodeiri, Ardejani, Shaghayesh Doulati, Mirzaghorbanali, Ali, McDougall, Kevin and Aziz, Naj. 2022. "Predicting the pyrite oxidation process within coal waste piles using multiple linear regression (MLR) and teaching-learning-based optimization (TLBO) algorithm." Aziz, Naj and Mirzaghorbanali, Ali (ed.) 2022 Resource Operators Conference (ROC2022). Australia Feb 2022 Australia.Probabilistic risk assessment of acid mine drainage generation resulted from chalcopyrite oxidation process within Sarcheshmeh copper mine tailings
Entezam, Shima, Shokri, Behshad Jodeiri, Ardejani, Faramarz Doulati, Mirzaghorbanali, Ali, McDougall, Kevin and Aziz, Naj. 2022. "Probabilistic risk assessment of acid mine drainage generation resulted from chalcopyrite oxidation process within Sarcheshmeh copper mine tailings." Aziz, Naj and Mirzaghorbanali, Ali (ed.) 2022 Resource Operators Conference (ROC2022). Australia Feb 2022 Australia.Angle Shear Testing of 15.2 mm Seven Wire Cable Bolt
Aziz, Naj, Mirzaghorbanali, Ali, Anzanpour, Sina, Rastegarmanesh, Ashkan, Khaleghparast, Saman, Nemcik, Jan, Oh, Joung and Si, Guangyao. 2022. "Angle Shear Testing of 15.2 mm Seven Wire Cable Bolt." Rock Mechanics and Rock Engineering. 55 (7), pp. 3919-3937. https://doi.org/10.1007/s00603-022-02847-2Mechanical Properties of Low-Stiffness Out-of-Grade Hybrid Pine — Effects of Knots, Resin and Pith
Cherry, Rebecca, Karunasena, Warna and Manalo, Allan. 2022. "Mechanical Properties of Low-Stiffness Out-of-Grade Hybrid Pine — Effects of Knots, Resin and Pith." Forests. 13 (927), pp. 1-32. https://doi.org/10.3390/f13060927Flexural behaviour of circular timber columns strengthened by glass fibre reinforced polymer wrapping system
Otoom, Omar F., Lokuge, Weena, Karunasena, Warna, Manalo, Allan C., Ozbakkaloglu, Togay and Ehsani, Mohammad R.. 2022. "Flexural behaviour of circular timber columns strengthened by glass fibre reinforced polymer wrapping system." Structures. 38, pp. 1349-1367. https://doi.org/10.1016/j.istruc.2022.02.070Effect of pretension on the performance of cable bolts and its optimisation in underground coal mines with various geological conditions
Li, Xu, Si, Guangyao, Oh, Joung, Corbett, Peter, O'Sullivan, Terri, Xiang, Zizhuo, Aziz, Naj and Mirzaghorbanali, Ali. 2022. "Effect of pretension on the performance of cable bolts and its optimisation in underground coal mines with various geological conditions." International Journal of Rock Mechanics and Mining Sciences. 152, pp. 1-21. https://doi.org/10.1016/j.ijrmms.2022.105076Calibration of a continuous hydrologic simulation model in the urban Gowrie Creek catchment in Toowoomba, Australia
Brown, I. W., McDougall, K., Alam, Md. Jahangir, Chowdhury, R. and Chadalavada, S.. 2022. "Calibration of a continuous hydrologic simulation model in the urban Gowrie Creek catchment in Toowoomba, Australia." Journal of Hydrology: Regional Studies. 40, pp. 1-16. https://doi.org/10.1016/j.ejrh.2022.101021Investigation on the properties of concrete with recycled polyethylene terephthalate (PET) granules as fine aggregate replacement
Eyni Kangavar, Mohammad, Lokuge, Weena, Manalo, Allan, Karunasena, Warna and Frigione, Mariaenrica. 2022. "Investigation on the properties of concrete with recycled polyethylene terephthalate (PET) granules as fine aggregate replacement." Case Studies in Construction Materials. 16, pp. 1-14. https://doi.org/10.1016/j.cscm.2022.e00934Fracture propagation mode of coal under indirect tensile stresses
Serati, Mehdi, Roshan, Hamid, Mirzaghorbanali, Ali, Mahmoud, Mutaz El-Amin and Thejaswee, Valluru. 2021. "Fracture propagation mode of coal under indirect tensile stresses." Aziz, Naj and Mirzaghorbanali, Ali (ed.) 2021 Resource Operators Conference (ROC2021). Springfield, Australia 10 - 12 Feb 2021 Australia.Network Deterioration Prediction for Reinforced Concrete Pipe and Box Culverts Using Markov Model: Case Study
Tran, Huu, Lokuge, Weena, Setunge, Sujeeva and Karunasena, Warna. 2022. "Network Deterioration Prediction for Reinforced Concrete Pipe and Box Culverts Using Markov Model: Case Study." Journal of Performance of Constructed Facilities. 36 (6), pp. 1-12. https://doi.org/10.1061/(ASCE)CF.1943-5509.0001766Strength properties of grout for strata reinforcement
Majoor, Dean, Mirzaghorbanali, Ali and Aziz, Naj. 2017. "Strength properties of grout for strata reinforcement." Aziz, Naj and Kinnemonth, Bob (ed.) 2017 Coal Operators' Conference. Wollongong, Australia 08 - 10 Feb 2017 Wollongong, Australia.The extent of shearing and the integrity of protective sleeve coating of cable bolts
Aziz, Naj, Mirzaghorbanali, Ali and Holden, Matthew. 2017. "The extent of shearing and the integrity of protective sleeve coating of cable bolts." Aziz, Naj and Kinnemonth, Bob (ed.) 2017 Coal Operators' Conference. Wollongong, Australia 08 - 10 Feb 2017 Wollongong, Australia.Axial Performance of Cementitious Grouted Cable Bolts Under Rotation Constraint Scenarios
Rastegarmanesh, Ashkan, Mirzaghorbanali, Ali, McDougall, Kevin, Aziz, Naj, Anzanpour, Sina, Nourizadeh, Hadi and Moosavi, Mahdi. 2022. "Axial Performance of Cementitious Grouted Cable Bolts Under Rotation Constraint Scenarios." Rock Mechanics and Rock Engineering. 55 (9), pp. 5773-5788. https://doi.org/10.1007/s00603-022-02950-4A case of Longwall coal mining productivity & safety optimisation
Ardehali, Sahar, Alehossein, Habib, Aziz, Naj, Bowerman, Matthew and Robbins, Matt. 2021. "A case of Longwall coal mining productivity & safety optimisation." Aziz, Naj and Mirzaghorbanali, Ali (ed.) 2021 Resource Operators Conference (ROC2021). Springfield, Australia 10 - 12 Feb 2021 Australia.Flexural behaviour of circular reinforced concrete columns strengthened by glass fibre reinforced polymer wrapping system
Otoom, Omar F., Lokuge, Weena, Karunasena, Warna, Manalo, Allan C., Ozbakkaloglu, Togay and Ehsani, Mohammad R.. 2022. "Flexural behaviour of circular reinforced concrete columns strengthened by glass fibre reinforced polymer wrapping system." Structures. 38, pp. 1326-1348. https://doi.org/10.1016/j.istruc.2022.02.071Proceedings of the 2022 Resource Operators Conference
Aziz, Naj and Mirzaghorbanali, Ali. Aziz, Naj and Mirzaghorbanali, Ali (ed.) 2022. Proceedings of the 2022 Resource Operators Conference. Wollongong, Australia. University of Wollongong.Computing high-order derivatives in compact integrated-RBF stencils
Mai-Duy, N., Strunin, D. and Karunasena, W.. 2022. "Computing high-order derivatives in compact integrated-RBF stencils." Engineering Analysis with Boundary Elements. 135, pp. 369-381. https://doi.org/10.1016/j.enganabound.2021.11.025Numerical simulation of influence parameters on induced stresses distribution during first weighting process of longwall mining
Mohammadi, Sadjad, Ataei, Mohammad, Kakaie, Reza and Mirzaghorbanali, Ali. 2022. "Numerical simulation of influence parameters on induced stresses distribution during first weighting process of longwall mining." Iranian Journal of Mining Engineering. 16 (53), pp. 1-16. https://doi.org/10.22034/ijme.2021.115543.1757Prediction of Fly-rock using Gene Expression Programming and Teaching– learning-based Optimization Algorithm
Shamsi, R., Amin, Mohammad Saeed, Dehghani, Hesam, Bascompta, Marc, Jodeiri Shokri, Behshad and Entezam, Shima. 2022. "Prediction of Fly-rock using Gene Expression Programming and Teaching– learning-based Optimization Algorithm." Journal of Mining and Environment. 13 (2), pp. 391-406. https://doi.org/10.22044/jme.2022.11825.2171Determination of ozone concentration using gene expression programming algorithm (GEP)- Zrenjanin, Serbia
Dehghani, Hesam, Velicković, Milica, Jodeiri Shokri, Behshad, Mihajlovic, Ivan, Nikolic, Djordje and Panic, Marija. 2022. "Determination of ozone concentration using gene expression programming algorithm (GEP)- Zrenjanin, Serbia." International Journal of Mining and Geo-Engineering. 56 (1), pp. 1-9. https://doi.org/10.22059/IJMGE.2021.313278.594874Developing a Conceptual Framework of Green Mining Strategy in Coal Mines: Integrating Socio-economic, Health, and Environmental Factors
Ardejani, Faramarz Doulati, Maghsoudy, Soroush, Shahhosseini, Majid, Jodeiri Shokri, Behshad, Ardejani, Shaghayegh Doulati, Shafaei, Foojan, Shiraz, Farzin Amirkhani and Rajaee, Ali. 2022. "Developing a Conceptual Framework of Green Mining Strategy in Coal Mines: Integrating Socio-economic, Health, and Environmental Factors." Journal of Mining and Environment. 13 (1), pp. 101-115. https://doi.org/10.22044/jme.2022.11704.2161Nonlinear dynamic instability of edge-cracked functionally graded graphene-reinforced composite beams
Song, Mitao, Zhou, Lei, Karunasena, Warna, Yang, Jie and Kitipornchai, Sritawat. 2022. "Nonlinear dynamic instability of edge-cracked functionally graded graphene-reinforced composite beams." Nonlinear Dynamics. 109 (4), pp. 2423 - 2441. https://doi.org/10.1007/s11071-022-07607-zAnalysis of failure modes in pipe-in-pipe repair systems for water and gas pipelines
Tafsirojjaman, T., Manalo, Allan, Tien, Cam Minh Tri, Wham, Brad P., Salah (Student), Ahmad, Kiriella, Shanika, Karunasena, Warna and Dixon, Patrick. 2022. "Analysis of failure modes in pipe-in-pipe repair systems for water and gas pipelines." Engineering Failure Analysis. 140, pp. 1-26. https://doi.org/10.1016/j.engfailanal.2022.106510Next Generation “Living” Laboratory for Engineering Education and Engagement
Nguyen, Andy, Le, Ngoc Thach, Landers, Richard, Byrne, Terry, McDougall, Kevin, Gharehbaghi, Vahid Reza, Brown, Jason, Nguyen, Canh Long and Karunasena, Warna. 2022. "Next Generation “Living” Laboratory for Engineering Education and Engagement." 33rd Annual Conference of the Australasian Association of Engineering Education (AAEE 2022). Sydney, Australia 04 - 07 Dec 2022 Australia.A convolution-deconvolution method for improved storage and communication of remotely-sensed image data
Scarmana, Gabriel and McDougall, Kevin. 2018. "A convolution-deconvolution method for improved storage and communication of remotely-sensed image data." Larar, Allen M., Suzuki, Makoto and Wang, Jianyu (ed.) 11th SPIE Asia-Pacific Remote Sensing Symposium 2018: Multispectral, Hyperspectral, and Ultraspectral Remote Sensing Technology, Techniques and Applications VII. Honolulu, United States 24 - 26 Sep 2018 Bellingham, Washington, United States. https://doi.org/10.1117/12.2324451Defining and Enabling Scholarship of Teaching, Learning, and Employability (SoTLE) Through a Multi-Institution Faculty Learning Community
Kinash, Shelley, Male, Sally A., Mouat, Clare M., Spagnoli, Dino, McDougall, Kevin, Thomas, Jasmine and Mortimer, Chloe Jayde. 2021. "Defining and Enabling Scholarship of Teaching, Learning, and Employability (SoTLE) Through a Multi-Institution Faculty Learning Community." Learning Communities Journal. 13 (1), pp. 81-103.Axial behaviour of rock bolts - Part (B) Numerical study
Nourizadeh, Hadi, Mirzaghorbanali, Ali, McDougall, Kevin, Aziz, Naj and Serati, Mehdi. 2021. "Axial behaviour of rock bolts - Part (B) Numerical study." Aziz, Naj and Mirzaghorbanali, Ali (ed.) 2021 Resource Operators Conference (ROC2021). Springfield, Australia 10 - 12 Feb 2021 Australia.Axial behaviour of rock bolts - Part (A) Experimental study
Nourizadeh, Hadi, Williams, Sally, Mirzaghorbanali, Ali, McDougall, Kevin, Aziz, Naj and Serati, Mehdi. 2021. "Axial behaviour of rock bolts - Part (A) Experimental study." Aziz, Naj and Mirzaghorbanali, Ali (ed.) 2021 Resource Operators Conference (ROC2021). Springfield, Australia 10 - 12 Feb 2021 Australia.Introduction to new methods of static and dynamic pull testing of rock bolts and cable bolts
Anzanpour, Sina, Aziz, Naj, Nemcik, Jan, Mirzaghorbanali, Ali, Wallace, Jordan, Marshall, Travis and Khaleghparast, Saman. 2021. "Introduction to new methods of static and dynamic pull testing of rock bolts and cable bolts." Aziz, Naj and Mirzaghorbanali, Ali (ed.) 2021 Resource Operators Conference (ROC2021). Springfield, Australia 10 - 12 Feb 2021 Australia.Application of Monte Carlo simulation to quantify uncertainties of first weighting interval estimation
Mohammadi, Sadjad, Ataei, Mohammad, Mirzaghorbanali, Ali and Aziz, Naj. 2021. "Application of Monte Carlo simulation to quantify uncertainties of first weighting interval estimation." Aziz, Naj and Mirzaghorbanali, Ali (ed.) 2021 Resource Operators Conference (ROC2021). Springfield, Australia 10 - 12 Feb 2021 Australia.Pretension effect on the performance of cable bolts in underground coal mines
Li, Xu, Si, Guangyao, Oh, Joung, Xiang, Zizhuo, Aziz, Naj and Mirzaghorbanali, Ali. 2021. "Pretension effect on the performance of cable bolts in underground coal mines." Aziz, Naj and Mirzaghorbanali, Ali (ed.) 2021 Resource Operators Conference (ROC2021). Springfield, Australia 10 - 12 Feb 2021 Australia.Effect of surface profile on axial load transfer mechanism of tendons
Rastegarmanesh, Ashkan, Misa, Joel, Mirzaghorbanali, Ali, Aziz, Naj and McDougall, Kevin. 2021. "Effect of surface profile on axial load transfer mechanism of tendons." Aziz, Naj and Mirzaghorbanali, Ali (ed.) 2021 Resource Operators Conference (ROC2021). Springfield, Australia 10 - 12 Feb 2021 Australia.Angled shear testing of 15.2 mm seven wire cable bolt
Aziz, Naj, Anzanpour, Sina, Khaleghparast, Saman, Rastegarmanesh, Ashkan, Mirzaghorbanali, Ali, Remmenikov, Alex, Nemcik, Jan, Oh, Joung and Si, Guangyao. 2021. "Angled shear testing of 15.2 mm seven wire cable bolt." Aziz, Naj and Mirzaghorbanali, Ali (ed.) 2021 Resource Operators Conference (ROC2021). Springfield, Australia 10 - 12 Feb 2021 Australa.Proceedings of the 2021 Resource Operators Conference
Aziz, Naj and Mirzaghorbanali, Ali. Aziz, Naj and Kininmonth, Bob (ed.) 2021. Proceedings of the 2021 Resource Operators Conference. Wollongong, Australia. University of Wollongong.Using geospatial tools to combat fire ants
Scarmana, Gabriel and McDougall, Kevin. 2021. "Using geospatial tools to combat fire ants." Position Magazine.Experimental and numerical evaluation of the compression behaviour of GFRP-wrapped infill materials
Otoom, Omar F., Lokuge, Weena, Karunasena, Warna, Manalo, Allan C., Ozbakkaloglu, Togay and Thambiratnam, David. 2021. "Experimental and numerical evaluation of the compression behaviour of GFRP-wrapped infill materials." Case Studies in Construction Materials. 15. https://doi.org/10.1016/j.cscm.2021.e00654Bushfire mitigation and preparation on the Toowoomba Region Escarpment: a research report for Toowoomba Regional Council
Ryan, Barbara, King, Rachel, Lokuge, Weena, Karunasena, Karu and Anderson, Esther. 2020. Bushfire mitigation and preparation on the Toowoomba Region Escarpment: a research report for Toowoomba Regional Council. Toowoomba, Australia. University of Southern Queensland.ISPRS Annals of the Photogrammetry, Remote Sensing and Spatial Information Sciences [Special issue]
McDougall, Kevin, Bhatta, Ganesh Prasad, Paudyal, Dev Raj, Shrestha, Reshma, Upadhyaya, Pradeep Sapkota, Dahal, Tank Prasad, Ranjit, Bhuwan, Shrestha, Sanjeevan and Thapa, Laxmi. 2019. ISPRS Annals of the Photogrammetry, Remote Sensing and Spatial Information Sciences [Special issue]. Germany. Copernicus GmbH.The International Archives of the Photogrammetry, Remote Sensing and Spatial Information Sciences
McDougall, Kevin, Bhatta, Ganesh Prasad, Paudyal, Dev Raj, Shrestha, Reshma, Upadhyaya, Pradeep Sapkota, Dahal, Tank Prasad, Ranjit, Bhuwan, Shrestha, Sanjeevan and Thapa, Laxmi. McDougall, Kevin, Paudyal, Dev Raj, Bhatta, Ganesh Prasad, Thapa, Laxmi, Dahal, Tank Prasad, Bhuwan, Ranjit, Sanjeevan, Shrestha, Shrestha, Reshma and Upadhyaya, Pradeep S (ed.) 2019. The International Archives of the Photogrammetry, Remote Sensing and Spatial Information Sciences. Germany. Copernicus GmbH.Static and dynamic testing of tendons
Aziz, Naj, Khaleghparast, Saman, Anzanpour, Sina, Remennikov, Alex and Mirzaghorbanali, Ali. 2020. "Static and dynamic testing of tendons." Rasskazov, Igor and Tkach, Sergei (ed.) VIII International Scientific Conference “Problems of Complex Development of Georesources” (PCDG 2020). Khabarovsk, Russian Federation 08 - 10 Sep 2020 France. https://doi.org/10.1051/e3sconf/202019201005ACARP PROJECT C27040: Development of a Numerical Modelling Approach to Better Understand the Effect of Cable Bolt Performance on Roof Failure Mechanisms in Varying Rock Mass Conditions
Si, Guangyao, Oh, Joung, Hagan, Paul, Li, Xu, Mirzaghorbanali, Ali, Rastegarmanesh, Ashkan, Aziz, Naj, Anzanpour, Sina and Khaleghparast, Saman. 2021. ACARP PROJECT C27040: Development of a Numerical Modelling Approach to Better Understand the Effect of Cable Bolt Performance on Roof Failure Mechanisms in Varying Rock Mass Conditions. Australia. Australian Coal Association Research Program (ACARP).Comparison of the shear test results of a cable bolt on three laboratory test apparatuses
Li, Xuwei, Aziz, Naj, Mirzaghorbanali, Ali and Nemcik, Jan. 2017. "Comparison of the shear test results of a cable bolt on three laboratory test apparatuses." Tunnelling and Underground Space Technology. 61, pp. 82-89. https://doi.org/10.1016/j.tust.2016.10.003A Probabilistic Model to Determine Main Caving Span by Evaluating Cavability of Immediate Roof Strata in Longwall Mining
Mohammadi, Sadjad, Ataei, Mohammad, Kakaie, Reza, Mirzaghorbanali, Ali and Aziz, Naj. 2021. "A Probabilistic Model to Determine Main Caving Span by Evaluating Cavability of Immediate Roof Strata in Longwall Mining." Geotechnical and Geological Engineering: an international journal. 39 (3), pp. 2221-2237. https://doi.org/10.1007/s10706-020-01620-yUnderstanding Contextual Attractiveness Factors of Transit Orientated Shopping Mall Developments (Tosmds) for Shopping Mall Passengers on the Dubai Metro Red Line
Abutaleb, Ayman, McDougall, Kevin, Basson, Marita, Hassan, Rumman and Mahmood, Muhammad Nateque. 2021. "Understanding Contextual Attractiveness Factors of Transit Orientated Shopping Mall Developments (Tosmds) for Shopping Mall Passengers on the Dubai Metro Red Line." Planning Practice and Research. 36 (3), pp. 292-313. https://doi.org/10.1080/02697459.2020.1829282The Impact of Transit-Oriented Shopping Mall Developments (TOSMDs) on Metro Station Ridership: Dubai Metro Redline
Abutaleb, Ayman, McDougall, Kevin, Basson, Marita, Hassan, Rumman and Mahmood, Muhammad Nateque. 2020. "The Impact of Transit-Oriented Shopping Mall Developments (TOSMDs) on Metro Station Ridership: Dubai Metro Redline." Urban Rail Transit. 6 (3), pp. 157-170. https://doi.org/10.1007/s40864-020-00129-0Static and dynamic testing of tendons
Khaleghparast, Saman, Anzanpour, Sina, Aziz, Naj, Remennikov, Alex and Mirzaghorbanali, Ali. 2020. "Static and dynamic testing of tendons." Aziz, Naj and Kininmonth, Bob (ed.) 2020 Coal Operators' Conference. Wollongong, Australia 18 - 20 Feb 2019 Wollongong, Australia.A review of overburden fracturing and changes in hydraulic characteristics due to longwall mining
Nourizadeh, Hadi, Canbulat, Ismet, Oh, Joung, Zhang, Chengguo, Aziz, Naj, Mirzaghorbanali, Ali and McDougall, Kevin. 2020. "A review of overburden fracturing and changes in hydraulic characteristics due to longwall mining." Aziz, Naj and Kininmonth, Bob (ed.) 2020 Coal Operators' Conference. Wollongong, Australia 18 - 20 Feb 2019 Wollongong, Australia.Shear strength properties of artificial rock joints
Zohaib, Muhammad, Mirzaghorbanali, Ali, Helwig, Andreas, Aziz, Naj, Gregor, Peter, Rastegarmanesh, Ashkan and McDougall, Kevin. 2020. "Shear strength properties of artificial rock joints." Aziz, Naj and Kininmonth, Bob (ed.) 2020 Coal Operators' Conference. Wollongong, Australia 18 - 20 Feb 2019 Wollongong, Australia.A process for the accurate reconstruction of pre-filtered and compressed digital aerial images
Scarmana, Gabriel and McDougall, Kevin. 2020. "A process for the accurate reconstruction of pre-filtered and compressed digital aerial images." 11th International Symposium on Digital Earth (ISDE 11). Florence, Italy 24 - 27 Sep 2019 Florence, Italy. https://doi.org/10.1088/1755-1315/509/1/012047A roof cavability classification system and its use for estimation of main caving interval in longwall mining
Mohammadi, Sodjad, Ataei, Mohammad, Kakaie, Reza, Mirzaghorbanali, Ali, Faraji Rad, Zahra and Aziz, Naj. 2020. "A roof cavability classification system and its use for estimation of main caving interval in longwall mining." Aziz, Naj and Kininmonth, Bob (ed.) 2020 Coal Operators' Conference. Wollongong, Australia 18 - 20 Feb 2019 Wollongong, Australia.A geometric model for estimating the volume and surface area of apples
Scarmana, Gabriel, McDougall, Kevin and Stepanyants, Yury. 2020. "A geometric model for estimating the volume and surface area of apples." Smart Surveyors for Land and Water Management (2020). Amsterdam, Netherlands 10 - 14 May 2020 Copenhagen, Denmark.Using a Mixed Method Research to Develop Spatial Information Sharing Strategies Across Natural Resource Management Communities
Paudyal, Dev Raj, McDougall, Kevin and Apan, Armando. 2019. "Using a Mixed Method Research to Develop Spatial Information Sharing Strategies Across Natural Resource Management Communities." Inaugural Australasia & Pacific Regional Mixed Methods International Research Association Conference 2019: Expanding Conceptual and Methodological Boundaries (MMIRA 2019). Wellington, New Zealand 04 - 06 Dec 2019 Indianapolis, United States.Performance of Bridges with Damaged Elements in Extreme Flood Events
Lokuge, W., Fraser, C. and Karunasena, W.. 2020. "Performance of Bridges with Damaged Elements in Extreme Flood Events." Wang, Chien Ming, Ho, Johnny C.M. and Kitipornchai, Sritawat (ed.) 25th Australasian Conference on Mechanics of Structures and Materials (ACMSM25). Brisbane, Australia 04 - 07 Dec 2018 Singapore. https://doi.org/10.1007/978-981-13-7603-0_40Floodway Design Process Revisted
Greene, I., Lokuge, W. and Karunasena, W.. 2020. "Floodway Design Process Revisted." Wang, Chien Ming, Ho, Johnny C.M. and Kitipornchai, Sritawat (ed.) 25th Australasian Conference on Mechanics of Structures and Materials (ACMSM25). Brisbane, Australia 04 - 07 Dec 2018 Singapore. https://doi.org/10.1007/978-981-13-7603-0_94Flexural Behavior of Functionally Graded-Graphene Reinforced Composite Plates
Rasappagari, M.R.R., Karunasena, W. and Lokuge, W.. 2020. "Flexural Behavior of Functionally Graded-Graphene Reinforced Composite Plates." Wang, Chen Ming, Ho, Johnny C.M. and Kitipornchai, Sritawat (ed.) 25th Australasian Conference on Mechanics of Structures and Materials (ACMSM25). Brisbane, Australia 04 - 07 Dec 2018 Singapore. https://doi.org/10.1007/978-981-13-7603-0_22Numerical exploration of performance of cable bolts in shear loading
Rasekh, Haleh, Aziz, Naj, Nemcik, Jan and Mirzaghorbanali, Ali. 2019. "Numerical exploration of performance of cable bolts in shear loading." 4th Australasian Conference on Computational Mechanics (ACCM-4). Hobart, Australia 27 - 29 Nov 2019 Australia.Structural design of floodways under extreme flood loading
Greene, Isaac, Lokuge, Weena and Karunasena, Warna. 2020. "Structural design of floodways under extreme flood loading." International Journal of Disaster Resilience in the Built Environment. 11 (4), pp. 535-555. https://doi.org/10.1108/IJDRBE-10-2019-0072A New Design-Oriented Model of Glass Fiber-Reinforced Polymer-Reinforced Hollow Concrete Columns
Alajarmeh, O. S., Manalo, A. C., Benmokrane, B., Karunasena, W., Ferdous, W. and Mendis, P.. 2020. "A New Design-Oriented Model of Glass Fiber-Reinforced Polymer-Reinforced Hollow Concrete Columns." ACI Structural Journal. 117 (2), pp. 141-156. https://doi.org/10.14359/51720204Out-of-grade sawn pine: A state-of-the-art review on challenges and new opportunities in cross laminated timber (CLT)
Cherry, Rebecca, Manalo, Allan, Karunasena, Warna and Stringer, Geoff. 2019. "Out-of-grade sawn pine: A state-of-the-art review on challenges and new opportunities in cross laminated timber (CLT) ." Construction and Building Materials. 211, pp. 858-868. https://doi.org/10.1016/j.conbuildmat.2019.03.293Joint strength of single-bolted pultruded GFRP square hollow sections with mechanical inserts under elevated temperatures
Hizam, R. M., Manalo, Allan C., Karunasena, Warna and Bai, Yu. 2020. "Joint strength of single-bolted pultruded GFRP square hollow sections with mechanical inserts under elevated temperatures." Journal of Composites for Construction. 24 (1), pp. 1-15. https://doi.org/10.1061/(ASCE)CC.1943-5614.0000984.Towards a Conceptual Framework for Understanding the Attractiveness of Rail Transit-Oriented Shopping Mall Developments (TOSMDs)
Abutaleb, Ayman, McDougall, Kevin, Basson, Marita, Hassan, Rumman and Mahmood, Nateque. 2019. "Towards a Conceptual Framework for Understanding the Attractiveness of Rail Transit-Oriented Shopping Mall Developments (TOSMDs)." Urban Rail Transit. 5 (4), pp. 225-239. https://doi.org/10.1007/s40864-019-00112-4Flexural behavior of geopolymer-concrete beams longitudinally reinforced with GFRP and steel hybrid reinforcements
Maranan, G. B., Manalo, A. C., Benmokrane, B., Karunasena, W., Mendis, P. and Nguyen, T. Q.. 2019. "Flexural behavior of geopolymer-concrete beams longitudinally reinforced with GFRP and steel hybrid reinforcements." Engineering Structures. 182, pp. 141-152. https://doi.org/10.1016/j.engstruct.2018.12.073Floodways and Flood-Related Experiences: A Survey of Industry Experts and Asset Owners
Greene, I., Lokuge, W. and Karunasena, K.. 2020. "Floodways and Flood-Related Experiences: A Survey of Industry Experts and Asset Owners." Engineering for Public Works. 19, pp. 69-75.3D Numerical Simulation and Dynamic Analyses of Debonded GFRP Composite Slabs
Jayatilake, I. N. and Karunasena, W.. 2020. "3D Numerical Simulation and Dynamic Analyses of Debonded GFRP Composite Slabs." 25th Australasian Conference on the Mechanics of Structures and Materials (ACMSM25). Brisbane, Australia 01 - 01 Dec 2018 Singapore. Springer. https://doi.org/10.1007/978-981-13-7603-0_8Hollow concrete columns: review of structural behavior and new designs using GFRP reinforcement
Alajarmeh, O. S., Manalo, A. C., Benmokrane, B., Karunasena, K., Ferdous, W. and Mendis, P.. 2020. "Hollow concrete columns: review of structural behavior and new designs using GFRP reinforcement." Engineering Structures. 203, pp. 1-16. https://doi.org/10.1016/j.engstruct.2019.109829Effect of spiral spacing and concrete strength on behavior of GFRP-reinforced hollow concrete columns
Alajarmeh, Omar S., Manalo, Allan C., Benmokrane, Brahim, Karunasena, Warna and Mendis, Priyan. 2020. "Effect of spiral spacing and concrete strength on behavior of GFRP-reinforced hollow concrete columns." Journal of Composites for Construction. 24 (1), pp. 1-14. https://doi.org/10.1061/(ASCE)CC.1943-5614.0000987Axial performance of hollow concrete columns reinforced with GFRP composite bars with different reinforcement ratios
Alajarmeh, Omar S., Manalo, Allan C., Benmokrane, Brahim, Karunasena, Warna and Mendis, Priyan. 2019. "Axial performance of hollow concrete columns reinforced with GFRP composite bars with different reinforcement ratios." Composite Structures. 213 (1), pp. 153-164. https://doi.org/10.1016/j.compstruct.2019.01.096Compressive behavior of axially loaded circular hollow concrete columns reinforced with GFRP bars and spirals
Alajarmeh, O. S., Manalo, A. C., Benmokrane, B., Karunasena, W., Mendis, P. and Nguyen, K. T. Q.. 2019. "Compressive behavior of axially loaded circular hollow concrete columns reinforced with GFRP bars and spirals." Construction and Building Materials. 194, pp. 12-23. https://doi.org/10.1016/j.conbuildmat.2018.11.016A new Roof Strata Cavability Index (RSCi) for longwall mining incorporating new rating system
Mohammadi, Sadjad, Ataei, Mohammad, Kakaie, Reza and Mirzaghorbanali, Ali. 2019. "A new Roof Strata Cavability Index (RSCi) for longwall mining incorporating new rating system." Geotechnical and Geological Engineering: an international journal. 37 (5), pp. 3619-3636. https://doi.org/10.1007/s10706-019-00857-6Numerical simulation of stress distribution in longwall panels during the first caving interval
Mohammadi, Sadjad, Ataei, Mohammad, Kakaie, Reza, Mirzaghorbanali, Ali, Aziz, Naj and Rastegarmanesh, Ashkan. 2019. "Numerical simulation of stress distribution in longwall panels during the first caving interval." Aziz, Naj and Kininmonth, Bob (ed.) 2019 Coal Operators' Conference. Wollongong, Australia 18 - 20 Feb 2019 Wollongong, Australia.Strength properties of grout for strata reinforcement
Mirzaghorbanali, Ali, Gregor, Peter, Ebrahim, Zeinab, Alfahed, Abdullah, Aziz, Naj and McDougall, Kevin. 2019. "Strength properties of grout for strata reinforcement." Aziz, Naj and Kininmonth, Bob (ed.) 2019 Coal Operators' Conference. Wollongong, Australia 18 - 20 Feb 2019 Wollongong, Australia.Shear strength of rock joints under constant normal loading conditions
Mirzaghorbanali, Ali, Alenzi, Faizal, Gregor, Peter, Aziz, Naj and McDougall, Kevin. 2019. "Shear strength of rock joints under constant normal loading conditions." Aziz, Naj and Kininmonth, Bob (ed.) 2019 Coal Operators' Conference. Wollongong, Australia 18 - 20 Feb 2019 Wollongong, Australia.Modelling of rebar and cable bolt behaviour in tension/shear
Li, Xuwei, Nemcik, Jan, Aziz, Naj, Mirzaghorbanali, Ali and Rasekh, Haleh. 2015. "Modelling of rebar and cable bolt behaviour in tension/shear." Aziz, Naj and Kininmonth, Bob (ed.) 2015 Coal Operators' Conference. Wollongong, Australia 11 - 13 Feb 2015 Australia.Effect of air column in transport canisters on measured gas contents of coal
Aziz, N. and Mirzaghorbanali, A.. 2017. "Effect of air column in transport canisters on measured gas contents of coal." Journal of Mining and Environment. 8 (1), pp. 1-9. https://doi.org/10.22044/jme.2016.631A study on the shear behaviour of infilled rock joints under cyclic loading and constant normal stiffness conditions
Mirzaghorbanali, Ali, Nemcik, Jan and Aziz, Naj. 2014. "A study on the shear behaviour of infilled rock joints under cyclic loading and constant normal stiffness conditions." Aziz, Naj (ed.) 2014 Coal Operators' Conference. Wollongong, Australia 12 - 14 Feb 2014 Wollongong, Australia.Suggested methods for the preparation and testing of various properties of resins and grouts
Aziz, Naj, Nemcik, Jan, Mirzaghorbanali, Ali, Foldi, Stephen, Joyce, David, Moslemi, Arash, Ghojavand, Hooman, Ma, Shuqi, Li, Xuwei and Rasekh, Haleh. 2014. "Suggested methods for the preparation and testing of various properties of resins and grouts." Aziz, Naj (ed.) 2014 Coal Operators' Conference. Wollongong, Australia 12 - 14 Feb 2014 Wollongong, Australia.Single shear testing of various cable bolts used in Australian mines
Aziz, Naj, Rink, Owen, Rasekh, Haleh, Hawkins, Ellie, Mirzaghorbanali, Ali, Yang, Guanyu, Khaleghparast, Saman, Mills, Kenneth, Nemcik, Jan and Li, Xuwei. 2017. "Single shear testing of various cable bolts used in Australian mines." Aziz, Naj and Kininmonth, Bob (ed.) 2017 Coal Operators' Conference. Wollongong, Australia 08 - 10 Feb 2017 Wollongong, Australia.Behaviour of cable bolts in shear; experimental study and mathematical modelling
Aziz, Naj, Craig, Peter, Mirzaghorbanali, Ali, Rasekh, Haleh, Nemcik, Jan and Li, Xuwei. 2015. "Behaviour of cable bolts in shear; experimental study and mathematical modelling." Aziz, Naj and Kininmonth, Bob (ed.) 2015 Coal Operators' Conference. Wollongong, Australia 11 - 13 Feb 2015 Wollongong, Australia.Strength characteristics of Secura Hollow Groutable Cable Bolts
Aziz, Naj, Hawker, Robert, Mirzaghorbanali, Ali, Nemcik, Jan, Li, Xuwei and Rasekh, Haleh. 2015. "Strength characteristics of Secura Hollow Groutable Cable Bolts." Aziz, Naj and Kininmonth, Bob (ed.) 2015 Coal Operators' Conference. Wollongong, Australia 11 - 13 Feb 2015 Wollongong, Australia.Strength properties of fibre glass dowels used for strata reinforcement in coal mines
Gilbert, David, Mirzaghorbanali, Ali, Li, Xuwei, Rasekh, Haleh, Aziz, Naj and Nemcik, Jan. 2015. "Strength properties of fibre glass dowels used for strata reinforcement in coal mines." Aziz, Naj and Kininmonth, Bob (ed.) 2015 Coal Operators' Conference. Wollongong, Australia 11 - 13 Feb 2015 Wollongong, Australia.An experimental study on the contact surface area of cabled bolted strata
Rasekh, Haleh, Aziz, Naj, Nemcik, Jan, Mirzaghorbanali, Ali and Li, Xuwei. 2015. "An experimental study on the contact surface area of cabled bolted strata." Aziz, Naj and Kininmonth, Bob (ed.) 2015 Coal Operators' Conference. Wollongong, Australia 11 - 13 Feb 2015 Wollongong, Australia.Quantitative assessment of flood discharges and floodway failures through cross-cultivation of advancement in knowledge and traditional practices
Wahalathantri, Buddhi, Lokuge, Weena, Karunasena, Warna and Setunge, Sujeeva. 2018. "Quantitative assessment of flood discharges and floodway failures through cross-cultivation of advancement in knowledge and traditional practices." International Journal of Disaster Resilience in the Built Environment. 9 (4-5), pp. 435-456. https://doi.org/10.1108/IJDRBE-09-2017-0051Shear behaviour of geopolymer-concrete beams transversely reinforced with continuous rectangular GFRP composite spirals
Maranan, G. B., Manalo, A. C., Benmokrane, B., Karunasena, W., Mendis, P. and Nguyen, T. Q.. 2018. "Shear behaviour of geopolymer-concrete beams transversely reinforced with continuous rectangular GFRP composite spirals." Composite Structures. 187, pp. 454-465. https://doi.org/10.1016/j.compstruct.2017.12.080Numerical investigation of the shear behaviour of a cable bolt in single shear test
Li, Xuwei, Yang, Guanyu, Nemcik, Jan, Mirzaghorbanali, Ali and Aziz, Naj. 2019. "Numerical investigation of the shear behaviour of a cable bolt in single shear test." Tunnelling and Underground Space Technology. 84, pp. 227-236. https://doi.org/10.1016/j.tust.2018.11.016Mechanical behaviours of grout for strata reinforcement
Mirzaghorbanali, Ali, Gregor, Peter, Alkandari, Hamed, Aziz, Naj and McDougall, Kevin. 2018. "Mechanical behaviours of grout for strata reinforcement." Aziz, Naj and Kininmonth, Bob (ed.) 2018 Coal Operators' Conference. Wollongong, Australia 07 - 09 Feb 2018 Wollongong, Australia.Behaviour of pultruded GFRP truss system connected using through-bolt with mechanical insert
Hizam, R. M., Manalo, Allan C., Karunasena, Warna and Bai, Yu. 2019. "Behaviour of pultruded GFRP truss system connected using through-bolt with mechanical insert." Composites Part B: Engineering. 168, pp. 44-57. https://doi.org/10.1016/j.compositesb.2018.12.052Estimation of the first roof weighting interval incorporating roof strata cavability concept
Mohammadi, Sadjad, Ataei, Mohammad, Kakaie, Reza and Mirzaghorbanali, Ali. 2019. "Estimation of the first roof weighting interval incorporating roof strata cavability concept ." Iranian Journal of Mining Engineering. 14 (73). https://doi.org/10.22034/ijme.2019.36639Role of structural analysis in floodway design process
Lokuge, Weena, Greene, Isaac, Etemadi, Elahe and Karunasena, Warna. 2019. "Role of structural analysis in floodway design process ." Australian Journal of Civil Engineering. 17 (2), pp. 110-119. https://doi.org/10.1080/14488353.2019.1653246Flexural behavior of glued GFRP tubes filled with concrete
Muttashar, Majid, Manalo, Allan, Karunasena, Warna and Lokuge, Weena. 2017. "Flexural behavior of glued GFRP tubes filled with concrete." 6th Asia-Pacific Conference on FRP in Structures (APFIS2017). Singapore 19 - 21 Jul 2017 Singapore.Effect of bolt threads on the double lap joint strength of pultruded fibre reinforced polymer composite materials
Hizam, R. M., Manalo, Allan C., Karunasena, Warna and Bai, Yu. 2018. "Effect of bolt threads on the double lap joint strength of pultruded fibre reinforced polymer composite materials." Construction and Building Materials. 181, pp. 185-198. https://doi.org/10.1016/j.conbuildmatat.2018.06.061Fuzzy analysis of airborne LiDAR data for rainforest boundary determination
Zhang, Zhenyu, Liu, Xiaoye, McDougall, Kevin and Wright, Wendy. 2017. "Fuzzy analysis of airborne LiDAR data for rainforest boundary determination." 6th International Conference on Telecommunications and Remote Sensing (ICTRS'17). Delft, Netherlands 06 - 07 Nov 2017 New york, United States. https://doi.org/10.1145/3152808.3152816Relevance assessment of crowdsourced data (CSD) using semantics and geographic information retrieval (GIR) techniques
Koswatte, Saman, McDougall, Kevin and Liu, Xiaoye. 2018. "Relevance assessment of crowdsourced data (CSD) using semantics and geographic information retrieval (GIR) techniques." ISPRS International Journal of Geo-Information. 7 (7), pp. 1-18. https://doi.org/10.3390/ijgi7070256Free vibration of functionally graded-GPL reinforced composite plates with different boundary conditions
Reddy, R. Muni Rami, Karunasena, W. and Lokuge, W.. 2018. "Free vibration of functionally graded-GPL reinforced composite plates with different boundary conditions." Aerospace Science and Technology. 78, pp. 147-156. https://doi.org/10.1016/j.ast.2018.04.019Innovative approach to strata reinforcement in coal mines with reference to evaluation cable bolts shear strength
Aziz, N., Mirzaghorbanali, A., Yang, G., Khaleghparast, S. and Rasekh, H.. 2018. "Innovative approach to strata reinforcement in coal mines with reference to evaluation cable bolts shear strength." Journal of Mining and Environment. 9 (3), pp. 703-715. https://doi.org/10.22044/jme.2018.6918.1525Prediction of the main caving span in longwall mining using fuzzy MCDM technique and statistical method
Mohammadi, S., Ataei, M., Khaloo Kakaie, R. and Mirzaghorbanali, A.. 2018. "Prediction of the main caving span in longwall mining using fuzzy MCDM technique and statistical method." Journal of Mining and Environment. 9 (3), pp. 717-726. https://doi.org/10.22044/jme.2018.6715.1492Behavior of pultruded multi-celled GFRP hollow beams with low-strength concrete infill
Muttashar, M., Karunasena, W., Manalo, A. and Lokuge, W.. 2017. "Behavior of pultruded multi-celled GFRP hollow beams with low-strength concrete infill." Hao, Hong and Zhang, Chunwei (ed.) 24th Australasian Conference on the Mechanics of Structures and Materials (ACMSM24). Perth, Australia 06 - 09 Dec 2016 London, United Kingdom.The effects of installation procedure on bond characteristics of fully grouted rock bolts
Ma, Shuji, Aziz, Naj, Nemcik, Jan and Mirzaghorbanali, Ali. 2017. "The effects of installation procedure on bond characteristics of fully grouted rock bolts." Geotechnical Testing Journal. 40 (5), pp. 846-857. https://doi.org/10.1520/GTJ20160239Simulation of macerals effects on methane emission during gas drainage in coal mines
Taheri, Adel, Sereshki, Farhang, Ardejani, Faramarz Doulati and Mirzaghorbanali, Ali. 2017. "Simulation of macerals effects on methane emission during gas drainage in coal mines." Fuel: the science and technology of fuel and energy. 210, pp. 659-665. https://doi.org/10.1016/j.fuel.2017.08.081Shear strength properties of cable bolts using a new double shear instrument, experimental study, and numerical simulation
Mirzaghorbanali, Ali, Rasekh, Haleh, Aziz, Naj, Yang, Guanyu, Khaleghparast, Saman and Nemcik, Jan. 2017. "Shear strength properties of cable bolts using a new double shear instrument, experimental study, and numerical simulation." Tunnelling and Underground Space Technology. 70, pp. 240-253. https://doi.org/10.1016/j.tust.2017.07.018An experimental study on the shear performance of fully encapsulated cable bolts in single shear test
Aziz, Naj, Rasekh, Haleh, Mirzaghorbanali, Ali, Yang, Guanyu, Khaleghparast, Saman and Nemcik, Jan. 2018. "An experimental study on the shear performance of fully encapsulated cable bolts in single shear test." Rock Mechanics and Rock Engineering. 51 (7), pp. 2207-2221. https://doi.org/10.1007/s00603-018-1450-0Flexural behaviour of multi-celled GFRP composite beams with concrete infill: experiment and theoretical analysis
Muttashar, Majid, Manalo, Allan, Karunasena, Warna and Lokuge, Weena. 2017. "Flexural behaviour of multi-celled GFRP composite beams with concrete infill: experiment and theoretical analysis." Composite Structures. 159, pp. 21-33. https://doi.org/10.1016/j.compstruct.2016.09.049Temperature-sensitive mechanical properties of GFRP composites in longitudinal and transverse directions: a comparative study
Manalo, Allan, Maranan, Ginghis, Sharma, Sukrant, Karunasena, Warna and Bai, Yu. 2017. "Temperature-sensitive mechanical properties of GFRP composites in longitudinal and transverse directions: a comparative study." Composite Structures. 173, pp. 255-267. https://doi.org/10.1016/j.compstruct.2017.04.040Comparison of the shear behaviour of geopolymer concrete beams with GFRP and steel transverse reinforcements
Maranan, Ginghis B., Manalo, Allan C., Karunasena, Warna M., Benmokrane, Brahim and Mendis, Priyan A.. 2015. "Comparison of the shear behaviour of geopolymer concrete beams with GFRP and steel transverse reinforcements." Wu, Zhishen, Wu, Gang and Wang, Xin (ed.) Joint Conference of the 12th International Symposium on Fiber Reinforced Polymers for Reinforced Concrete Structures (FRPRCS-12) & the 5th Asia-Pacific Conference on Fiber Reinforced Polymers in Structures (APFIS-2015). Nanjing, China 14 - 16 Dec 2015 China.Effects of cyclic loading on the shear behaviour of infilled rock joints under constant normal stiffness conditions
Mirzaghorbanali, Ali, Nemcik, Jan and Aziz, Naj. 2014. "Effects of cyclic loading on the shear behaviour of infilled rock joints under constant normal stiffness conditions." Rock Mechanics and Rock Engineering. 47 (4), pp. 1373-1391. https://doi.org/10.1007/s00603-013-0452-1Shear strength properties of plain and spirally profiled cable bolts
Aziz, Naj, Mirzaghorbanali, Ali, Nemcik, Jan, Heeman, Kay and Mayer, Stefan. 2015. "Shear strength properties of plain and spirally profiled cable bolts." Canadian Geotechnical Journal. 52 (10), pp. 1490-1495. https://doi.org/10.1139/cgj-2014-0504Finite element based dynamic analysis of multilayer fibre composite sandwich plates with interlayer delaminations
Jayatilake, Indunil N., Karunasena, Warna and Lokuge, Weena. 2016. "Finite element based dynamic analysis of multilayer fibre composite sandwich plates with interlayer delaminations." Advances in Aircraft and Spacecraft Science. 3 (1), pp. 15-28. https://doi.org/10.12989/aas.2016.3.1.015Influence of infill concrete strength on the flexural behaviour of pultruded GFRP square beams
Muttashar, Majid, Manalo, Allan, Karunasena, Warna and Lokuge, Weena. 2016. "Influence of infill concrete strength on the flexural behaviour of pultruded GFRP square beams." Composite Structures. 145, pp. 58-67. https://doi.org/10.1016/j.compstruct.2016.02.071Vulnerability assessment of urban community and critical infrastructures for integrated flood risk management and climate adaptation strategies
Espada, Rudolf, Apan, Armando and McDougall, Kevin. 2017. "Vulnerability assessment of urban community and critical infrastructures for integrated flood risk management and climate adaptation strategies." International Journal of Disaster Resilience in the Built Environment. 8 (4), pp. 375-411. https://doi.org/10.1108/IJDRBE-03-2015-0010VGI and crowdsourced data credibility analysis using spam email detection techniques
Koswatte, Saman, McDougall, Kevin and Liu, Xiaoye. 2018. "VGI and crowdsourced data credibility analysis using spam email detection techniques." International Journal of Digital Earth: a new journal for a new vision. 11 (5), pp. 520-532. https://doi.org/10.1080/17538947.2017.1341558ACARP PROJECT C42012: Shear testing of the major Australian cable bolts under different pretension loads
Aziz, Naj, Mirzaghorbanali, Ali, Nemcik, Jan, Hadi, Muhammad and Remennikov, Alex. 2017. ACARP PROJECT C42012: Shear testing of the major Australian cable bolts under different pretension loads. Australia. Australian Coal Association Research Program (ACARP).Fibre-reinforced polymer (FRP) repair systems for corroded steel pipelines
Sirimanna, C. S., Manalo, A. C., Karunasena, W., Banerjee, S. and McGarva, L.. 2015. "Fibre-reinforced polymer (FRP) repair systems for corroded steel pipelines." Karbhari, Vistasp M. (ed.) Rehabilitation of pipelines using fibre-reinforced polymer (FRP) composites. United Kingdom. Woodhead Publishing Limited. pp. 267-285Semantic location extraction from crowdsourced data
Koswatte, Saman, McDougall, Kevin and Liu, Xiaoye. 2016. "Semantic location extraction from crowdsourced data." 23rd International Society for Photogrammetry and Remote Sensing (ISPRS 2016). Prague, Czech Republic 12 - 19 Jul 2016 Germany. https://doi.org/10.5194/isprsarchives-XLI-B2-543-2016Double shear testing of cable bolts with no concrete face contacts
Rasekh, Haleh, Aziz, Naj, Mirzaghorbanali, Ali, Nemcik, Jan, Li, Xuwei, Yang, Guanyu and Khaleghparast, Saman. 2017. "Double shear testing of cable bolts with no concrete face contacts." Procedia Engineering. 191, pp. 1169-1177. https://doi.org/10.1016/j.proeng.2017.05.292Strength properties of grout for strata reinforcement
Aziz, Naj, Majoor, Dean and Mirzaghorbanali, Ali. 2017. "Strength properties of grout for strata reinforcement." Procedia Engineering. 191, pp. 1178-1184. https://doi.org/10.1016/j.proeng.2017.05.293A new equation for the shear strength of cable bolts incorporating the energy balance theory
Rasekh, Haleh, Mirzaghorbanali, Ali, Nemcik, Jan, Aziz, Naj and Li, Xuwei. 2017. "A new equation for the shear strength of cable bolts incorporating the energy balance theory." Geotechnical and Geological Engineering: an international journal. 35 (4), pp. 1529-1548. https://doi.org/10.1007/s10706-017-0191-2An application of space filling curves to digital terrain models
Scarmana, Gabriel and McDougall, Kevin. 2016. "An application of space filling curves to digital terrain models." Jarosz, Andrew (ed.) 16th International Congress for Mine Surveying: Connecting Education and Industry. Brisbane, Australia 12 - 16 Sep 2016 Brisbane, Australia.Shear behavior of geopolymer concrete beams reinforced with glass fiber-reinforced polymer bars
Maranan, G. B., Manalo, A. C., Benmokrane, B., Karunasena, W. and Mendis, P.. 2017. "Shear behavior of geopolymer concrete beams reinforced with glass fiber-reinforced polymer bars." ACI Structural Journal. 114 (2), pp. 337-348. https://doi.org/10.14359/51689150Durability characteristics and property prediction of glass fibre reinforced mixed plastics composites
Bajracharya, Rohan Muni, Manalo, Allan C., Karunasena, Warna and Lau, Kin-tak. 2017. "Durability characteristics and property prediction of glass fibre reinforced mixed plastics composites." Composites Part B: Engineering. 116, pp. 16-29. https://doi.org/10.1016/j.compositesb.2017.02.027Review of Australian land use mapping and land management practice
Baral, Govinda Prasad, McDougall, Kevin and Chong, Albert. 2011. "Review of Australian land use mapping and land management practice." 2011 Surveying and Spatial Sciences Conference: Innovation in Action: Working Smarter (SSSC 2011). Wellington, New Zealand 21 - 25 Nov 2011 Adelaide, Australia.Using volunteered information to map the Queensland floods
McDougall, Kevin. 2011. "Using volunteered information to map the Queensland floods." 2011 Surveying and Spatial Sciences Conference: Innovation in Action: Working Smarter (SSSC 2011). Wellington, New Zealand 21 - 25 Nov 2011 Adelaide, Australia.Analytical model of shear behaviour of a fully grouted cable bolt subjected to shearing
Li, Xuwei, Nemcik, Jan, Mirzaghorbanali, Ali, Aziz, Naj and Rasekh, Haleh. 2015. "Analytical model of shear behaviour of a fully grouted cable bolt subjected to shearing." International Journal of Rock Mechanics and Mining Sciences. 80, pp. 31-39. https://doi.org/10.1016/j.ijrmms.2015.09.005Dynamic analysis of multilayer GFRP sandwich slabs with interlayer delaminations
Jayatilake, Indunil N., Karunasena, Warna and Lokuge, Weena. 2014. "Dynamic analysis of multilayer GFRP sandwich slabs with interlayer delaminations." Sabu, John and Das, Raj (ed.) 8th Australasian Congress on Applied Mechanics (ACAM 8). Melbourne, Australia 24 - 28 Nov 2014 Australia.Dynamic behaviour of fibre composite multilayer sandwich plates with delaminations
Jayatilake, Indunil, Karunasena, Warna and Lokuge, Weena. 2014. "Dynamic behaviour of fibre composite multilayer sandwich plates with delaminations." Smith, Scott T. (ed.) 23rd Australasian Conference on the Mechanics of Structures and Materials (ACMSM23). Byron Bay, Australia 09 - 12 Dec 2014 Lismore, Australia.Bond-slip behaviour of GFRP bars into geopolymer concrete
Maranan, Ginghis B., Manalo, Allan C., Karunasena, Karu and Benmokrane, Brahim. 2014. "Bond-slip behaviour of GFRP bars into geopolymer concrete." El-Hacha, Raafat (ed.) 7th International Conference on FRP Composites in Civil Engineering (CICE 2014). Vancouver, Canada 20 - 22 Aug 2014 Calgary, AL. Canada.Geopolymer concrete with FRP confinement
Lembo, Kurt, Lokuge, Weena and Karunasena, Warna. 2014. "Geopolymer concrete with FRP confinement." Smith, Scott (ed.) 23rd Australasian Conference on the Mechanics of Structures and Materials (ACMSM23). Byron Bay, Australia 09 - 12 Dec 2014 Byron Bay, Australia.Geopolymer concrete: the green alternative with suitable structural properties
Muttashar, Majid, Lokuge, Weena and Karunasena, Warna. 2014. "Geopolymer concrete: the green alternative with suitable structural properties." Smith, Scott T. (ed.) 23rd Australasian Conference on the Mechanics of Structures and Materials (ACMSM23). Byron Bay, Australia 09 - 12 Dec 2014 Lismore, Australia.Mechanical properties of grouts at various curing times
Mirza, Ali, Aziz, Naj, Ye, Wang and Nemcik, Jan. 2016. "Mechanical properties of grouts at various curing times." Aziz, Naj and Kininmonth, Bob (ed.) 2016 Coal Operators' Conference. Wollongong, Australia 10 - 12 Feb 2016 Wollongong, Australia.Modelling simulation of the performance of cable bolts in shear
Rasekh, Haleh, Mirza, Ali, Aziz, Naj, Nemcik, Jan and Li, Xuwei. 2016. "Modelling simulation of the performance of cable bolts in shear." Aziz, Naj and Kininmonth, Bob (ed.) 2016 Coal Operators' Conference. Wollongong, Australia 10 - 12 Feb 2016 Wollongong, Australia.Experimental investigation on the flexural behaviour of pultruded GFRP beams filled with different concrete strengths
Muttashar, M., Manalo, A., Karunasena, W. and Lokuge, W.. 2016. "Experimental investigation on the flexural behaviour of pultruded GFRP beams filled with different concrete strengths." Teng, J. G. and Dai, J. G. (ed.) 8th International Conference on Fibre-Reinforced Polymer (FRP) Composites in Civil Engineering (CICE 2016). Hong Kong, China 14 - 16 Dec 2016 China.SDI and crowdsourced spatial information management automation for disaster management
Koswatte, S., McDougall, K. and Liu, X.. 2015. "SDI and crowdsourced spatial information management automation for disaster management." Survey Review. 47 (344), pp. 307-315. https://doi.org/10.1179/1752270615Y.0000000008SDI and crowdsourced spatial information management automation for disaster management
Koswatte, Saman, McDougall, Kevin and Liu, Xiaoye. 2014. "SDI and crowdsourced spatial information management automation for disaster management." FIG Commission 3 Workshop 2014. Bologna, Italy 04 - 07 Nov 2014Challenges of teaching engineering analysis software to distance education students
Banerjee, Sourish, Karunasena, Warna and Kist, Alexander A.. 2016. "Challenges of teaching engineering analysis software to distance education students." Smith, Scott, Lim, Yee Yan, Bahadori, Alireza, Lake, Neal, Padilla, Ricardo Vasquez, Rose, Andrew and Doust, Ken (ed.) 27th Annual Conference of the Australasian Association for Engineering Education (AAEE 2016). Coffs Harbour, Australia 04 - 07 Dec 2016 Lismore, Australia.An elasto-plastic constitutive model for rock joints under cyclic loading and constant normal stiffness conditions
Nemcik, Jan, Mirzaghorbanali, Ali and Aziz, Naj. 2014. "An elasto-plastic constitutive model for rock joints under cyclic loading and constant normal stiffness conditions." Geotechnical and Geological Engineering: an international journal. 32 (2), pp. 321-335. https://doi.org/10.1007/s10706-013-9716-5Effect of skin-core debonding on the dynamic behaviour of GFRP composite beams
Jayatilake, Indunil, Karunasena, Warna and Lokuge, Weena. 2013. "Effect of skin-core debonding on the dynamic behaviour of GFRP composite beams." Epaarachchi, Jayantha A., Lau, Alan Kin-tak and Leng, Jinsong (ed.) 4th International Conference on Smart Materials and Nanotechnology in Engineering (SMN 2013). Gold Coast, Australia 10 - 12 Jul 2013 United States. https://doi.org/10.1117/12.2026751Effects of shear rate on cyclic loading shear behaviour of rock joints under constant normal stiffness conditions
Mirzaghorbanali, Ali, Nemcik, Jan and Aziz, Naj. 2014. "Effects of shear rate on cyclic loading shear behaviour of rock joints under constant normal stiffness conditions." Rock Mechanics and Rock Engineering. 47 (5), pp. 1931-1938. https://doi.org/10.1007/s00603-013-0453-0A follow up to study the behaviour of cable bolts in shear: experimental study and mathematical modelling
Aziz, Naj, Mirza, Ali, Nemcik, Jan, Rasekh, Haleh and Li, Xuwei. 2016. "A follow up to study the behaviour of cable bolts in shear: experimental study and mathematical modelling." Aziz, Naj and Kininmonth, Bob (ed.) 2016 Coal Operators' Conference. Wollongong, Australia 10 - 12 Feb 2016 Wollongong, Australia.Load transfer characteristics of plain and spiral cable bolts tested in new non rotating pull testing apparatus
Aziz, Naj, Mirza, Ali, Nemcik, Jan, Li, Xuwei, Rasekh, Haleh and Wang, Gaofeng. 2016. "Load transfer characteristics of plain and spiral cable bolts tested in new non rotating pull testing apparatus." Aziz, Naj and Kininmonth, Bob (ed.) 2016 Coal Operators' Conference. Wollongong, Australia 10 - 12 Feb 2016 Wollongong, Australia.Numerical modeling of gas flow in coal pores for methane drainage
Taheri, Adel, Sereshki, Farhang, Ardejani, Faramarz Doulati and Mirzaghorbanali, Ali. 2016. "Numerical modeling of gas flow in coal pores for methane drainage." Journal of Sustainable Mining. 15, pp. 95-99. https://doi.org/10.1016/j.jsm.2016.10.001Behavior of fiber glass bolts, rock bolts and cable bolts in shear
Li, Xuwei, Aziz, Naj, Mirzaghorbanali, Ali and Nemcik, Jan. 2016. "Behavior of fiber glass bolts, rock bolts and cable bolts in shear." Rock Mechanics and Rock Engineering. 49 (7), pp. 2723-2735. https://doi.org/10.1007/s00603-015-0907-7Factors influencing the quality of encapsulation in rock bolting
Aziz, Naj, Craig, Peter, Mirzaghorbanali, Ali and Nemcik, Jan. 2016. "Factors influencing the quality of encapsulation in rock bolting." Rock Mechanics and Rock Engineering. 49 (8), pp. 3189-3203. https://doi.org/10.1007/s00603-016-0973-5An experimental study of axial load transfer mechanisms of cable bolts using axially split embedment apparatus
Mirzaghorbanali, A. and Aziz, N.. 2017. "An experimental study of axial load transfer mechanisms of cable bolts using axially split embedment apparatus." Journal of Mining and Environment. 8 (2), pp. 131-137. https://doi.org/10.22044/jme.2016.732The effect of shear span-to-depth ratio on the failure mode and strength of pultruded GFRP beams
Muttashar, Majid, Karunasena, Warna, Manalo, Allan and Lokuge, Weena. 2015. "The effect of shear span-to-depth ratio on the failure mode and strength of pultruded GFRP beams." Joint Conference of the 12th International Symposium on Fiber Reinforced Polymers for Reinforced Concrete Structures (FRPRCS-12) & the 5th Asia-Pacific Conference on Fiber Reinforced Polymers in Structures (APFIS-2015). Nanjing, China 14 - 16 Dec 2015 China.Testing and characterization of pultruded glass fiber reinforced polymer (GFRP) beams
Muttashar, Majid, Karunasena, Warna, Manalo, Allan and Lokuge, Weena. 2015. "Testing and characterization of pultruded glass fiber reinforced polymer (GFRP) beams." Joint Conference of the 12th International Symposium on Fiber Reinforced Polymers for Reinforced Concrete Structures (FRPRCS-12) & the 5th Asia-Pacific Conference on Fiber Reinforced Polymers in Structures (APFIS-2015). Nanjing, China 14 - 16 Dec 2015 China.Effect of elevated temperature on the tensile properties of recycled mixed plastic waste
Bajracharya, Rohan Muni, Manalo, Allan C., Karunasena, Warna and Lau, Kin-tak. 2014. "Effect of elevated temperature on the tensile properties of recycled mixed plastic waste." Smith, S. T. (ed.) 23rd Australasian Conference on the Mechanics of Structures and Materials (ACMSM23). Byron Bay, Australia 09 - 12 Dec 2014 Lismore, Australia.Glass fibre and recycled mixed plastic wastes: recent developments and applications
Bajracharya, Rohan M., Manalo, Allan C., Karunasena, Warna and Lau, Kin-tak. 2014. "Glass fibre and recycled mixed plastic wastes: recent developments and applications." Smith, S. T. (ed.) 23rd Australasian Conference on the Mechanics of Structures and Materials (ACMSM23). Byron Bay, Australia 09 - 12 Dec 2014 Australia.Flexural response of GFRP-reinforced geopolymer concrete beams
Maranan, Ginghis B., Manalo, Allan C., Karunasena, Warna, Benmokrane, Brahim and Mendis, Priyan. 2015. "Flexural response of GFRP-reinforced geopolymer concrete beams." Sanjayan, Jay (ed.) 27th Biennial National Conference of the Concrete Institute of Australia (Concrete 2015) in conjunction with the 69th RILEM Week: Construction Innovations, Research into Practice. Melbourne, Australia 30 Aug - 02 Sep 2015 Australia.Flood inundation mapping using hydraulic modelling and GIS: a case study in the West Creek sub-catchment
Sahib, Basheer, Liu, Xiaoye and McDougall, Kevin. 2014. "Flood inundation mapping using hydraulic modelling and GIS: a case study in the West Creek sub-catchment." 3rd National Conference on Urban Water Management: Storm water 2014. Adelaide, South Australia 13 - 17 Oct 2014 Australia.Flexural behaviour of glass fibre reinforced polymer (GFRP) bars subjected to elevated temperature
Maranan, G. B., Manalo, A. C., Karunasena, W., Benmokrane, B. and Lutze, D.. 2014. "Flexural behaviour of glass fibre reinforced polymer (GFRP) bars subjected to elevated temperature." Smith, Scott T. (ed.) 23rd Australasian Conference on the Mechanics of Structures and Materials (ACMSM23). Byron Bay, Australia 09 - 12 Dec 2014 Lismore, Australia.Framework to inspect floodways towards estimating damage
Wahalathantri, Buddhi L., Lokuge, Weena, Karunasena, Warna and Setunge, Sujeeva. 2015. "Framework to inspect floodways towards estimating damage." Annual Conference of the Australasian Fire and Emergency Service Authorities Council and Bushfire & Natural Hazards CRC (AFAC15): New Directions in Emergency Management. Adelaide, Australia 01 - 03 Sep 2015 Australia.Exploring the application of some common raster scanning paths on lossless compression of elevation images
Scarmana, Gabriel and McDougall, Kevin. 2015. "Exploring the application of some common raster scanning paths on lossless compression of elevation images." IEEE International Geoscience and Remote Sensing Symposium 2015 (IGARSS 2015): Remote Sensing: Understanding the Earth for a Safer World. Milan, Italy 26 - 31 Jul 2015 Milan, Italy.Behaviour of concentrically loaded geopolymer-concrete circular columns reinforced longitudinally and transversely with GFRP bars
Maranan, G. B., Manalo, A. C., Benmokrane, B., Karunasena, W. and Mendis, P.. 2016. "Behaviour of concentrically loaded geopolymer-concrete circular columns reinforced longitudinally and transversely with GFRP bars." Engineering Structures. 117, pp. 422-436. https://doi.org/10.1016/j.engstruct.2016.03.036Experimental and theoretical studies on the properties of injection moulded glass fibre reinforced mixed plastics composites
Bajracharya, Rohan Muni, Manalo, Allan C., Karunasena, Warna and Lau, Kin-tak. 2016. "Experimental and theoretical studies on the properties of injection moulded glass fibre reinforced mixed plastics composites." Composites Part A: Applied Science and Manufacturing. 84, pp. 393-405. https://doi.org/10.1016/j.compositesa.2016.02.025Pre-impregnated carbon fibre reinforced composite system for patch repair of steel I-beams
Manalo, Allan, Sirimanna, Chamila, Karunasena, Warna, McGarva, Lance and Falzon, Paul. 2016. "Pre-impregnated carbon fibre reinforced composite system for patch repair of steel I-beams." Construction and Building Materials. 105, pp. 365-376. https://doi.org/10.1016/j.conbuildmat.2015.12.172Behaviour of new building products from recycled-mixed plastic wastes
Bajracharya, Rohan Muni, Manalo, Allan C., Karunasena, Warna and Lau, Kin-tak. 2015. "Behaviour of new building products from recycled-mixed plastic wastes." 12th Composites Australia and CRC-ACS Conference 2015: Game On! Winning with Composites. Gold Coast, Australia 21 - 23 Apr 2015Characterisation of recycled mixed plastic solid wastes: coupon and full-scale investigation
Bajracharya, Rohan Muni, Manalo, Allan C., Karunasena, Warna and Lau, Kin-tak. 2016. "Characterisation of recycled mixed plastic solid wastes: coupon and full-scale investigation." Waste Management. 48, pp. 72-80. https://doi.org/10.1016/j.wasman.2015.11.017Emergency service demand in Queensland during natural disasters
Wahalathantri, Buddhi, Lokuge, Weena, Karunasena, Warna and Setunge, Sujeeva. 2015. "Emergency service demand in Queensland during natural disasters." 5th International Conference on Building Resilience. Newcastle, Australia 15 - 17 Jul 2015 Newcastle, Australia.Natural fibers and their characterization
Azwa, Z. N., Yousif, B. F., Manalo, A. C. and Karunasena, W.. 2016. "Natural fibers and their characterization." Campilho, R. D. S. G. (ed.) Natural fiber composites. Boca Raton, FL, United States. CRC Press. pp. 35-64Influence of single and multiple skin-core debonding on free vibration characteristics of innovative GFRP sandwich panels
Jayatilake, Indunil, Karunasena, Warna and Lokuge, Weena. 2016. "Influence of single and multiple skin-core debonding on free vibration characteristics of innovative GFRP sandwich panels." International Journal of Mechanical, Aerospace, Industrial and Mechatronics Engineering. 9 (5), pp. 800-804.Analysis of retrofitted corroded steel pipes using internally bonded FRP composite repair systems
Sirimanna, C. S., Banerjee, S., Karunasena, W., Manalo, A. C. and McGarva, L.. 2015. "Analysis of retrofitted corroded steel pipes using internally bonded FRP composite repair systems." Australian Journal of Structural Engineering. 16 (3), pp. 187-198. https://doi.org/10.1080/13287982.2015.1092681Vulnerability assessment and interdependency analysis of critical infrastructures for climate adaptation and flood mitigation
Espada Jr., Rodolfo, Apan, Armando and McDougall, Kevin. 2015. "Vulnerability assessment and interdependency analysis of critical infrastructures for climate adaptation and flood mitigation." International Journal of Disaster Resilience in the Built Environment. 6 (3), pp. 313-346. https://doi.org/10.1108/IJDRBE-02-2014-0019Behaviour of hollow pultruded GFRP square beams with different shear span-to-depth ratios
Muttashar, Majid, Karunasena, Warna, Manalo, Allan and Lokuge, Weena. 2016. "Behaviour of hollow pultruded GFRP square beams with different shear span-to-depth ratios." Journal of Composite Materials. 50 (21), pp. 2925-2940. https://doi.org/10.1177/0021998315614993Vulnerability of floodways under extreme flood events
Wahalathantri, Buddhi Lankananda, Lokuge, Weena, Karunasena, Warna and Setunge, Sujeeva. 2015. "Vulnerability of floodways under extreme flood events." Natural Hazards Review. 17 (1). https://doi.org/10.1061/(ASCE)NH.1527-6996.0000194Ductility enhancement of geopolymer concrete columns using FRP confinement
Lokuge, Weena and Karunasena, Warna. 2016. "Ductility enhancement of geopolymer concrete columns using FRP confinement." Journal of Composite Materials. 50 (14), pp. 1887-1896. https://doi.org/10.1177/0021998315597553Effects of alkali treatment and elevated temperature on the mechanical properties of bamboo fibre-polyester composites
Manalo, Allan C., Wani, Evans, Zulkarnain, Noor Azwa, Karunasena, Warna and Lau, Kin-tak. 2015. "Effects of alkali treatment and elevated temperature on the mechanical properties of bamboo fibre-polyester composites." Composites Part B: Engineering. 80 (1), pp. 73-83. https://doi.org/10.1016/j.compositesb.2015.05.033Evaluation of the flexural strength and serviceability of geopolymer concrete beams reinforced with glass-fibre-reinforced polymer (GFRP) bars
Maranan, G., Manalo, A. C., Benmokrane, B., Karunasena, W. and Mendis, P.. 2015. "Evaluation of the flexural strength and serviceability of geopolymer concrete beams reinforced with glass-fibre-reinforced polymer (GFRP) bars." Engineering Structures. 101, pp. 529-541. https://doi.org/10.1016/j.engstruct.2015.08.003Pullout behaviour of GFRP bars with anchor head in geopolymer concrete
Maranan, G.B., Manalo, A.C., Karunasena, W. and Benmokrane, B.. 2015. "Pullout behaviour of GFRP bars with anchor head in geopolymer concrete." Composite Structures. 132, pp. 1113-1121. https://doi.org/10.1016/j.compstruct.2015.07.021Spatial modelling of natural disaster risk reduction policies with Markov decision processes
Espada Jr., Rodolfo, Apan, Armando and McDougall, Kevin. 2014. "Spatial modelling of natural disaster risk reduction policies with Markov decision processes." Applied Geography. 53, pp. 284-298. https://doi.org/10.1016/j.apgeog.2014.06.021Characterisation of network objects in natural and anthropic environments
Harris, Bruce, McDougall, Kevin and Barry, Michael. 2014. "Characterisation of network objects in natural and anthropic environments." Dragicevic, S. and Li, S. (ed.) ISPRS Technical Commission II Midterm Symposium 2014. Toronto, Canada 06 - 08 Oct 2014 London, United Kingdom. https://doi.org/10.5194/isprsarchives-XL-2-161-2014The impact of varying statutory arrangements on spatial data sharing and access in regional NRM bodies
Paudyal, D. R., McDougall, K. and Apan, A.. 2014. "The impact of varying statutory arrangements on spatial data sharing and access in regional NRM bodies." ISPRS Annals of the Photogrammetry, Remote Sensing and Spatial Information Sciences. II (8), pp. 193-197. https://doi.org/10.5194/isprsannals-II-8-193-2014Vulnerability of road bridge infrastructure under extreme flood events
Setunge, Sujeeva, Lokuge, Weena, Mohseni, Hessam and Karunasena, Warna. 2014. "Vulnerability of road bridge infrastructure under extreme flood events." AFAC and Bushfire and Natural Hazards CRC Conference 2014. Wellington, New Zealand 02 - 05 Sep 2014Bond stress-slip behavior: case of GFRP bars in geopolymer concrete
Maranan, Ginghis, Manalo, Allan, Karunasena, Karu and Benmokrane, Brahim. 2015. "Bond stress-slip behavior: case of GFRP bars in geopolymer concrete." Journal of Materials in Civil Engineering. 27 (1), pp. 4014116-1. https://doi.org/10.1061/(ASCE)MT.1943-5533.0001046A pyramid approach to lossless data compression of grid-based digital elevation models
Scarmana, Gabriel and McDougall, Kevin. 2014. "A pyramid approach to lossless data compression of grid-based digital elevation models." IEEE International Geoscience and Remote Sensing Symposium 2014 (IGARSS 2014): Energy and our Changing Planet. Quebec City, Canada 13 - 18 Jul 2014 Piscataway, NJ. United States. https://doi.org/10.1109/IGARSS.2014.6946981Glass-fibre reinforced recycled mixed plastics (GMP): totally recyclable and renewable composites
Bajracharya, Rohan Muni, Manalo, Allan, Karunasena, Karu and Lau, Kin-tak. 2014. "Glass-fibre reinforced recycled mixed plastics (GMP): totally recyclable and renewable composites." IEEE Australia and New Zealand Student and Young Professional Congress (ANZSCON 2014). Brisbane, Australia 03 - 05 Jul 2014Fibre reinforced geopolymer concrete with ambient curing for in-situ applications
Reed, Mark, Lokuge, Weena and Karunasena, Warna. 2014. "Fibre reinforced geopolymer concrete with ambient curing for in-situ applications." Journal of Materials Science. 49 (12), pp. 4297-4304. https://doi.org/10.1007/s10853-014-8125-3Investigating the performance of floodway in an extreme flood event
Lokuge, Weena, Setunge, Sujeeva and Karunasena, Warna. 2014. "Investigating the performance of floodway in an extreme flood event." Li, Chun Qing, Setunge, Sujeeva and de Silva, Saman (ed.) 1st International Conference on Infrastructure Failures and Consequences (ICIFC 2014). Melbourne, Australia 16 - 20 Jul 2014 Melbourne, Australia.Investigation into fibre composites jacket with an innovative joining system
Manalo, Allan C., Karunasena, Warna, Sirimanna, Chamila and Maranan, Ginghis B.. 2014. "Investigation into fibre composites jacket with an innovative joining system." Construction and Building Materials. 65, pp. 270-281. https://doi.org/10.1016/j.conbuildmat.2014.04.125Effect of strain rate on geopolymer concrete
Lokuge, Weena, Karunasena, W. and Heemskerk, Colin. 2013. "Effect of strain rate on geopolymer concrete." Dao, Vinh and Dux, Peter (ed.) Concrete Institute of Australia's Biennial National Conference (Concrete 2013): Understanding Concrete. Gold Coast, Australia 16 - 18 Oct 2013 Sydney, Australia.Effects of shearing direction on shear behaviour of rock joints
Mirzaghorbanali, Ali, Rasekh, Haleh, Aziz, Naj and Nemcik, Jan. 2014. "Effects of shearing direction on shear behaviour of rock joints." Aziz, Naj (ed.) 2014 Coal Operators' Conference. Wollongong, Australia 12 - 14 Feb 2014 Australia.Flexural and axial behavior of pultruded GFRP hollow sections filled with low strength concrete
Muttashar, Majid, Manalo, Allan, Karunasena, Warna and Lokuge, Weena. 2014. "Flexural and axial behavior of pultruded GFRP hollow sections filled with low strength concrete." Australasian Composites Conference (CA 2014): Materials for a Lighter and Smarter World. Newcastle, Australia 07 - 09 Apr 2014 Melbourne, Australia.An experimental investigation on the effect of threaded bolt on the double lap joint strength of pultruded fibre reinforced polymer
Hizam, R. M., Karunasena, Warna and Manalo, Allan C.. 2014. "An experimental investigation on the effect of threaded bolt on the double lap joint strength of pultruded fibre reinforced polymer." Australasian Composites Conference (CA 2014): Materials for a Lighter and Smarter World. Newcastle, Australia 07 - 09 Apr 2014 Melbourne, Australia.An overview of mechanical properties and durability of glass-fibre reinforced recycled mixed plastic waste composites
Bajracharya, Rohan Muni, Manalo, Allan C., Karunasena, Warna and Lau, Kin-tak. 2014. "An overview of mechanical properties and durability of glass-fibre reinforced recycled mixed plastic waste composites." Materials and Design. 62, pp. 98-112. https://doi.org/10.1016/j.matdes.2014.04.081Tensile properties of glass fibre reinforced recycled mixed plastic composites
Bajracharya, Rohan Muni, Manalo, Allan C., Karunasena, Warna and Lau, Kin-tak. 2014. "Tensile properties of glass fibre reinforced recycled mixed plastic composites." Australasian Composites Conference (CA 2014): Materials for a Lighter and Smarter World. Newcastle, Australia 07 - 09 Apr 2014 Melbourne, Australia.Free vibration behaviour of fibre composite sandwich beams with debonds
Karunasena, W., Jayatilake, I. and Lokuge, W.. 2013. "Free vibration behaviour of fibre composite sandwich beams with debonds." Dissanayake, Ranjith and Jayasinghe, Thishan (ed.) 4th International Conference on Structural Engineering and Construction Management 2013 (ICSECM 2013). Kandy, Sri Lanka 13 - 15 Dec 2013 Peradeniya, Sri Lanka.A review of FRP composite truss systems and its connection
Hizam, R. M., Manalo, A. C. and Karunasena, W.. 2013. "A review of FRP composite truss systems and its connection." Samali, Bijan, Attard, Mario M. and Song, Chongmin (ed.) 22nd Australasian Conference on the Mechanics of Structures and Materials (ACMSM22). Sydney, Australia 11 - 14 Dec 2012 London, United Kingdom.Developing spatial information sharing strategies across natural resource management communities
Paudyal, Dev Raj, McDougall, Kevin and Apan, Armando. 2013. "Developing spatial information sharing strategies across natural resource management communities." Onsrud, Harlan and Rajabifard, Abbas (ed.) Spatial Enablement in Support of Economic Development and Poverty Reduction: Research, Development and Education Perspectives. Needham, MA, USA. GSDI Association Press. pp. 141-168Using spatial modelling to develop flood risk and climate adaptation capacity metrics for vulnerability assessments of urban community and critical water supply infrastructure
Espada Jr., Rodolfo, Apan, Armando and McDougall, Kevin. 2013. "Using spatial modelling to develop flood risk and climate adaptation capacity metrics for vulnerability assessments of urban community and critical water supply infrastructure." Featherstone, Jeffrey (ed.) 49th World Congress of the International Society of City and Regional Planners (ISOCARP 2013): Frontiers of Planning: Evolving and Declining Models of City Planning Practice. Brisbane, Australia 01 - 04 Oct 2013 Netherlands.A comparison between static and dynamic foot mobility magnitude measures
Alshadli, Duaa, Chong, Albert K., Newsham-West, Richard, Al-Baghdadi, Jasim Ahmed Ali, Milburn, Peter and McDougall, Kevin. 2013. "A comparison between static and dynamic foot mobility magnitude measures." Toby, Hung (ed.) 31st International Conference on Biomechanics in Sports (ISBS 2013). Taipei, Taiwan 07 - 11 Jul 2013 Taipei, Taiwan.Human foot plantar surface shape change during gait
AL-Baghdadi, Jasim Ahmed Ali, Chong, Albert K., McDougall, Kevin, Milburn, Peter and Newsham-West, Richard. 2013. "Human foot plantar surface shape change during gait." Toby, Hung (ed.) 31st International Conference on Biomechanics in Sports (ISBS 2013). Taipei, Taiwan 07 - 11 Jul 2013 Taipei, Taiwan.A review on the degradability of polymeric composites based on natural fibres
Azwa, Z. N., Yousif, B. F., Manalo, A. C. and Karunasena, W.. 2013. "A review on the degradability of polymeric composites based on natural fibres." Materials and Design. 47, pp. 424-442. https://doi.org/10.1016/j.matdes.2012.11.025Fibre composites pile rehabilitation and concrete formwork jacket: concept development and finite element analysis
Manalo, A. C., Karunasena, W., Sirimanna, C. and Maranan, G.. 2013. "Fibre composites pile rehabilitation and concrete formwork jacket: concept development and finite element analysis." Al-Mahaidi, Riadh, Zhao, Xiao-Ling, Smith, Scott T. and Bai, Yu (ed.) 4th Asia-Pacific Conference on FRP in Structures (APFIS 2013): Towards Sustainable Infrastructure with FRP Composites. Melbourne, Australia 11 - 13 Dec 2013 Melbourne, Australia.Mechanical properties of bamboo fiber-polyester composites
Manalo, A. C., Karunasena, W. and Lau, K. T.. 2013. "Mechanical properties of bamboo fiber-polyester composites." Samali, Bijan, Attard, Mario and Song, Chongmin (ed.) 22nd Australasian Conference on the Mechanics of Structures and Materials (ACMSM22). Sydney, Australia 11 - 14 Dec 2012 Leiden, Netherlands.Clogging mechanism of permeable concrete: A review
Mishra, Krishna, Zhuge, Yan and Karunasena, Karu. 2013. "Clogging mechanism of permeable concrete: A review." Dao, Vinh and Dux, Peter (ed.) Concrete Institute of Australia's Biennial National Conference (Concrete 2013): Understanding Concrete. Gold Coast, Australia 16 - 18 Oct 2013 Sydney, Australia.Structural evaluation of concrete expanded polystyrene sandwich panels for slab applications
Bajracharya, R. M., Lokuge, W. P., Karunasena, W., Lau, K. T. and Mosallam, A. S.. 2013. "Structural evaluation of concrete expanded polystyrene sandwich panels for slab applications." Samali, Bijan, Attard, Mario M. and Song, Chongmin (ed.) 22nd Australasian Conference on the Mechanics of Structures and Materials (ACMSM22). Sydney, Australia 11 - 14 Dec 2012 London, United Kingdom.Dynamic behaviour of debonded GFRP composite beams
Jayatilake, Indunil, Karunasena, Warna and Lokuge, Weena. 2013. "Dynamic behaviour of debonded GFRP composite beams." Journal of Multifunctional Composites. 1 (2), pp. 113-122. https://doi.org/10.12783/issn.2168-4286/1.2/JayatilakeShear behaviour of glued structural fibre composite sandwich beams
Manalo, A. C., Aravinthan, T. and Karunasena, W.. 2013. "Shear behaviour of glued structural fibre composite sandwich beams." Construction and Building Materials. 47, pp. 1317-1327. https://doi.org/10.1016/j.conbuildmat.2013.06.025Using spatial modelling to develop flood risk and climate adaptation capacity metrics for assessing urban community and critical electricity infrastructure vulnerability
Espada Jr., R., Apan, A. and McDougall, K.. 2013. "Using spatial modelling to develop flood risk and climate adaptation capacity metrics for assessing urban community and critical electricity infrastructure vulnerability." Piantadosi, J., Anderssen, R. S. and Boland, J. (ed.) 20th International Congress on Modelling and Simulation (MODSIM2013). Adelaide, Australia 01 - 06 Dec 2013 Australia. Modelling and Simulation Society of Australia and New Zealand . https://doi.org/10.36334/modsim.2013.L1.espadaACARPC21011: Development Of New Testing Procedure For The Assessment Of Resin Performance For Improved Encapsulated Roof Bolt Installation In Coal Mines
Aziz, Naj, Nemcik, Jan, Craig, Peter, Hawker, Robert, Devenish, Colin, Mirza, Ali, Moslemi, Arash, Ghojavand, Hooman, Bedford, Mark, Gaudry, Tim and Joyce, David. 2014. ACARPC21011: Development Of New Testing Procedure For The Assessment Of Resin Performance For Improved Encapsulated Roof Bolt Installation In Coal Mines . Australia. Australian Coal Association Research Program (ACARP).The Strength Properties of Fibreglass and Other Polymer-based Dowels for Strata Reinforcement in Coalmines
Aziz, N, Gilbert, D, Nemcik, J, Mirzaghorbanali, A and Burton, R. 2014. "The Strength Properties of Fibreglass and Other Polymer-based Dowels for Strata Reinforcement in Coalmines." 3rd Australasian Ground Control in Mining Conference (AusRock 2014). Sydney, Australia 05 - 06 Nov 2014 Australia.The global cadastre
McDougall, Kevin, Bennett, Rohan and Molen, Paul van der. 2013. "The global cadastre." GIM International. 27 (7), pp. 30-34.Effect of eccentricity on the behavior of pultruded FRP bolted joint
Hizam, R. M., Manalo, A. C. and Karunasena, W.. 2013. "Effect of eccentricity on the behavior of pultruded FRP bolted joint." 3rd Malaysian Postgraduate Conference (MPC 2013). Sydney, Australia 04 - 05 Jul 2013 Sydney, Australia.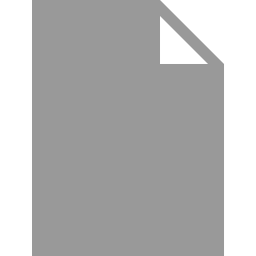