Influence of image noise on crack detection performance of deep convolutional neural networks
Paper
Paper/Presentation Title | Influence of image noise on crack detection performance of deep convolutional neural networks |
---|---|
Presentation Type | Paper |
Authors | Chianese, R. (Author), Nguyen, A. (Author), Gharehbaghi, V. R. (Author), Aravinthan, T. (Author) and Noori, M. (Author) |
Editors | Cunha, A. and Caetano, E. |
Journal or Proceedings Title | Proceedings of the 10th International Conference on Structural Health Monitoring of Intelligent Infrastructure (SHMII-10) |
ERA Conference ID | 43541 |
Number of Pages | 8 |
Year | 2021 |
Place of Publication | Winnipeg, Canada |
Web Address (URL) of Paper | https://arxiv.org/ftp/arxiv/papers/2111/2111.02079.pdf |
Conference/Event | 10th International Conference on Structural Health Monitoring of Intelligent Infrastructure: Advanced Research and Real-World Applications (SHMII-10) |
International Conference on Structural Health Monitoring of Intelligent Infrastructure | |
Event Details | International Conference on Structural Health Monitoring of Intelligent Infrastructure Rank A A A A A A A A A A A A A A A A A A A A A |
Event Details | 10th International Conference on Structural Health Monitoring of Intelligent Infrastructure: Advanced Research and Real-World Applications (SHMII-10) Event Date 30 Jun 2021 to end of 02 Jul 2021 Event Location Porto, Portugal |
Abstract | Development of deep learning techniques to analyse image data is an expansive and emerging field. The benefits of tracking, identifying, measuring, and sorting features of interest from image data has endless applications for saving cost, time, and improving safety. Much research has been conducted on classifying cracks from image data using deep convolutional neural networks; however, minimal research has been conducted to study the efficacy of network performance when noisy images are used. This paper will address the problem and is dedicated to investigating the influence of image noise on network accuracy. The methods used incorporate a benchmark image data set, which is purposely deteriorated with two types of noise, followed by treatment with image enhancement pre-processing techniques. These images, including their native counterparts, are then used to train and validate two different networks to study the differences in accuracy and performance. Results from this research reveal that noisy images have a moderate to high impact on the network's capability to accurately classify images despite the application of image pre-processing. A new index has been developed for finding the most efficient method for classification in terms of computation timing and accuracy. Consequently, AlexNet was selected as the most efficient model based on the proposed index |
Keywords | crack detection; transfer learning; deep convolution neural network; image noise; network performance |
ANZSRC Field of Research 2020 | 400508. Infrastructure engineering and asset management |
Byline Affiliations | School of Civil Engineering and Surveying |
Kharazmi University, Iran | |
California Polytechnic State University, United States | |
Institution of Origin | University of Southern Queensland |
https://research.usq.edu.au/item/q6y18/influence-of-image-noise-on-crack-detection-performance-of-deep-convolutional-neural-networks
Download files
148
total views73
total downloads3
views this month1
downloads this month
Export as
Related outputs
Effect of Camera Choice on Image-Classification Inference
Brown, Jason, Nguyen, Andy and Raj, Nawin. 2025. "Effect of Camera Choice on Image-Classification Inference." Applied Sciences. 15 (1). https://doi.org/10.3390/app15010246A Supervised Machine Learning Approach for Structural Overload Classification in Railway Bridges using Weigh-in-Motion Data
Le, N.T., Keenan, M., Nguyen, A., Ghazvineh, S., Yu, Y., Li, J. and Manalo, A.. 2025. "A Supervised Machine Learning Approach for Structural Overload Classification in Railway Bridges using Weigh-in-Motion Data." Structures. 71. https://doi.org/10.1016/j.istruc.2024.108005Optimising Concrete Crack Detection: A Study of Transfer Learning with Application on Nvidia Jetson Nano
Nguyen, C. Long, Nguyen, Andy, Brown, Jason, Byrne, Terry, Ngo, Binh Thanh and Luong, Chieu Xuan. 2024. "Optimising Concrete Crack Detection: A Study of Transfer Learning with Application on Nvidia Jetson Nano ." Sensors. 24 (23). https://doi.org/10.3390/s24237818Damage Identification in Large-scale Bridge Girders Using Output-only Modal Flexibility-based Deflections and Span-similar Virtual Beam Models
Le, N. T., Nguyen, A., Chan, T. H. T. and Thambiratnam, D. P.. 2024. "Damage Identification in Large-scale Bridge Girders Using Output-only Modal Flexibility-based Deflections and Span-similar Virtual Beam Models." Structural Control and Health Monitoring. 2024. https://doi.org/10.1155/2024/4087831Experimental validation of an efficient strategy for FE model updating and damage identification in tubular structures
Ghannadi, Parsa, Kourehli, Seyed Sina and Nguyen, Andy. 2024. "Experimental validation of an efficient strategy for FE model updating and damage identification in tubular structures." Nondestructive Testing and Evaluation. https://doi.org/10.1080/10589759.2024.2402887Robustness of Deep Transfer Learning-Based Crack Detection against Uncertainty in Hyperparameter Tuning and Input Data
Nguyen, Andy, Chianese, Riccardo R., Gharehbaghi, Vahid R., Perera, Ruveen, Low, Tobias, Aravinthan, Thiru, Yu, Yang, Samali, Bijan, Guan, Hong, Khuc, Tung and Le, Thach N.. 2022. "Robustness of Deep Transfer Learning-Based Crack Detection against Uncertainty in Hyperparameter Tuning and Input Data." Guan, Hong, Chan, Tommy H. T. and Li, Jianchun (ed.) Recent Advances in Structural Health Monitoring Research in Australia. New York, United States. Nova Science Publishers. pp. 215-243Pavement Crack Segmentation Using an Attention-Based Deep Learning Model
Dao, Hieu, Khuc, Tung, Truong, Quan, Dinh, Cang and Nguyen, Andy. 2024. "Pavement Crack Segmentation Using an Attention-Based Deep Learning Model." 4th International Conference on Sustainability in Civil Engineering (ICSCE 2022). Hanoi, Vietnam 25 - 27 Nov 2022 Singapore . Springer. https://doi.org/10.1007/978-981-99-2345-8_75ASR crack identification in bridges using deep learning and texture analysis
Nguyen, Andy, Gharehbaghi, Vahidreza, Le, Ngoc Thach, Sterling, Lucinda, Chaudhry, Umar Inayat and Crawford, Shane. 2023. "ASR crack identification in bridges using deep learning and texture analysis." Structures. 50, pp. 494-507. https://doi.org/10.1016/j.istruc.2023.02.042Finite element model updating and damage identification using semi-rigidly connected frame element and optimization procedure: An experimental validation
Ghannadi, Parsa, Khatir, Samir, Kourehli, Seyed Sina, Nguyen, Andy, Boutchicha, Djilali and Wahab, Magd Abdel. 2023. "Finite element model updating and damage identification using semi-rigidly connected frame element and optimization procedure: An experimental validation." Structures. 50, pp. 1173-1190. https://doi.org/10.1016/j.istruc.2023.02.008A data-driven approach for linear and nonlinear damage detection using variational mode decomposition and GARCH model
Gharehbaghi, Vahid Reza, Kalbkhani, Hashem, Farsangi, Ehsan Noroozinejad, Yang, T. Y. and Mirjalili, Seyedali. 2023. "A data-driven approach for linear and nonlinear damage detection using variational mode decomposition and GARCH model." Engineering with Computers: an international journal for simulation-based engineering. 39 (3), pp. 2017-2034. https://doi.org/10.1007/s00366-021-01568-4The Differential Evolution Algorithm: An analysis of more than two decades of application in structural damage detection (2001-2022)
Ghannadi, Parsa, Kourehli, Seyed Sina and Nguyen, Aandy. 2023. "The Differential Evolution Algorithm: An analysis of more than two decades of application in structural damage detection (2001-2022)." Noori, Mohammad, Rainieri, Carlo, Domaneschi, Marco and Sarhosis, Vasilis (ed.) Data Driven Methods for Civil Structural Health Monitoring and Resilience. United States. CRC Press. pp. 14-57Shape memory polymer composite circular and square hollow members for deployable structures
Emmanuel, K.D.C., Jeewantha, L.H.J., Herath, H.M.C.M., Epaarachchi, J.A. and Aravinthan, T.. 2023. "Shape memory polymer composite circular and square hollow members for deployable structures ." Composites Part A: Applied Science and Manufacturing. 171. https://doi.org/10.1016/j.compositesa.2023.107559Can Bamboo Become a Sustainable Building Material in Australia?
Miranda, Mari, Thorpe, David and Nguyen, Andy. 2022. "Can Bamboo Become a Sustainable Building Material in Australia?" 8th International Sustainable Ecological Engineering Design for Society Conference (SEEDS 2022). Bristol, United Kingdom 31 Aug - 02 Sep 2022 Bristol, United Kingdom.Effective Sensitivity-Based Model Updating of Cable-Stayed Bridges Considering Monitoring Data Variability
Sharry, Thomas, Guan, Hong, Nguyen, Andy, Oh, Erwin and Hoang, Nam. 2022. "Effective Sensitivity-Based Model Updating of Cable-Stayed Bridges Considering Monitoring Data Variability." Guan, Hong, Chan, Tommy H. T. and Li, Jianchun (ed.) Recent Advances in Structural Health Monitoring Research in Australia. New York, United States. Nova Science Publishers. pp. 71-111Damage onset analysis of optimized shape memory polymer composites during programming into curved shapes
Emmanuel, K. D. C., Jeewantha, L. H. J., Herath, H. M. C. M., Epaarachchi, J. A. and Aravinthan, T.. 2022. "Damage onset analysis of optimized shape memory polymer composites during programming into curved shapes." Materialia. 26, pp. 1-11. https://doi.org/10.1016/j.mtla.2022.101599A computationally efficient crack detection approach based on deep learning assisted by stockwell transform and linear discriminant analysis
Nguyen, Andy, Nguyen, Canh Long, Gharehbaghi, Vahidreza, Perera, Ruveen, Brown, Jason, Yu, Yang and Kalbkhani, Hashem. 2022. "A computationally efficient crack detection approach based on deep learning assisted by stockwell transform and linear discriminant analysis." Structures. 45, pp. 1962-1970. https://doi.org/10.1016/j.istruc.2022.09.107Design optimisation of hollow box pultruded FRP profiles using mixed integer constrained Genetic algorithm
Alhawamdeh, Mohammad, Alajarmeh, Omar, Aravinthan, Thiru, Shelley, Tristan, Schubel, Peter, Mohammed, Ali and Zeng, Xuesen. 2022. "Design optimisation of hollow box pultruded FRP profiles using mixed integer constrained Genetic algorithm." Composite Structures. 302, pp. 1-21. https://doi.org/10.1016/j.compstruct.2022.116247Smart Automated Fault Detection for Improved Road Maintenance Planning in Australia
Brisolin, Jacob, Nguyen, Andy, Ullah, Fahim, Bourke, Allan, Khuc, Tung and Nsanabo, Vivien. 2022. "Smart Automated Fault Detection for Improved Road Maintenance Planning in Australia." Guan, Hong, Chan, Tommy H. T. and Li, Jianchun (ed.) Recent Advances in Structural Health Monitoring Research in Australia. New York, United States. Nova Science Publishers. pp. 245-278Computer vision-based classification of cracks on concrete bridges using machine learning techniques
Yu, Yang, Rashidi, Maria, Samali, Bijan, Mohammadi, Masoud and Nguyen, Andy. 2021. "Computer vision-based classification of cracks on concrete bridges using machine learning techniques." Cunha, A. and Caetano, E. (ed.) 10th International Conference on Structural Health Monitoring of Intelligent Infrastructure: Advanced Research and Real-World Applications (SHMII-10). Porto, Portugal 30 Jun - 02 Jul 2021 Winnipeg, Canada.Finite element model updating of a cable-stayed bridge using structural health monitoring data
Sharry, Thomas, Guan, Hong, Hoang, Nam, Nguyen, Andy and Oh, Erwin. 2021. "Finite element model updating of a cable-stayed bridge using structural health monitoring data." Cunha, A. and Caetano, E. (ed.) 10th International Conference on Structural Health Monitoring of Intelligent Infrastructure: Advanced Research and Real-World Applications (SHMII-10). Porto, Portugal 30 Jun - 02 Jul 2021 Winnipeg, Canada.Damage identification in concrete gravity dams using static and dynamic measurement data
Le, N. T., Thambiratnam, D. P., Nguyen, A. and Chan, T. H. T.. 2021. "Damage identification in concrete gravity dams using static and dynamic measurement data." Cunha, A. and Caetano, E. (ed.) 10th International Conference on Structural Health Monitoring of Intelligent Infrastructure: Advanced Research and Real-World Applications (SHMII-10). Porto, Portugal 30 Jun - 02 Jul 2021 Winnipeg, Canada.Global best practices in dams & hydraulic structures: Alkali-Silica Reaction
Gharehbaghi, Vahid R. and Nguyen, Andy. 2021. "Global best practices in dams & hydraulic structures: Alkali-Silica Reaction." USHER in 2021: Redefining Resiliency in the New Normal. Philippines 23 Jul 2021Axial behaviour of precast concrete panels with hollow composite reinforcing systems
Al-Fakher, Usama, Manalo, Allan, Alajarmeh, Omar, Aravinthan, Thiru, Zhuge, Yan, Bai, Yu and Edoo, Azam. 2021. "Axial behaviour of precast concrete panels with hollow composite reinforcing systems." Structures. 32, pp. 76-86. https://doi.org/10.1016/j.istruc.2021.03.015A Critical Review on Structural Health Monitoring: Definitions, Methods, and Perspectives
Gharehbaghi, Vahid Reza, Farsangi, Ehsan Noroozinejad, Noori, Mohammad, Yang, T. Y., Li, Shaofan, Nguyen, Andy, Malaga‑Chuquitaype, Christian, Gardoni, Paolo and Mirjalili, Seyedali. 2021. "A Critical Review on Structural Health Monitoring: Definitions, Methods, and Perspectives." Archives of Computational Methods in Engineering. 29 (4), pp. 2209-2235. https://doi.org/10.1007/s11831-021-09665-9Shear behaviour of hollow precast concrete-composite structures
Al-Fakher, Usama, Manalo, Allan, Ferdous, Wahid, Alajarmeh, Omar, Aravinthan, Thiru, Zhuge, Yan, Bai, Yu and Edoo, Azam. 2021. "Shear behaviour of hollow precast concrete-composite structures." Materials and Structures. 54 (2), pp. 1-18. https://doi.org/10.1617/s11527-021-01618-9Bending and Shear Behaviour of Waste Rubber Concrete-Filled FRP Tubes with External Flanges
Ferdous, Wahid, Manalo, Allan, Alajarmeh, Omar S., Zhuge, Yan, Mohammed, Ali A., Bai, Yu, Aravinthan, Thiru and Schubel, Peter. 2021. "Bending and Shear Behaviour of Waste Rubber Concrete-Filled FRP Tubes with External Flanges." Polymers. 13 (15), pp. 1-18. https://doi.org/10.3390/polym13152500Bending behaviour of precast concrete slab with externally flanged hollow FRP tubes
Al-Fakher, Usama, Manalo, Allan, Ferdous, Wahid, Aravinthan, Thiru, Zhuge, Yan, Bai, Yu and Edoo, Azam. 2021. "Bending behaviour of precast concrete slab with externally flanged hollow FRP tubes." Engineering Structures. 241, pp. 1-10. https://doi.org/10.1016/j.engstruct.2021.112433Review on Local Buckling of Hollow Box FRP Profiles in Civil Structural Applications
Alhawamdeh, Mohammad, Alajarmeh, Omar, Aravinthan, Thiru, Shelley, Tristan, Schubel, Peter, Mohammed, Ali and Zeng, Xuesen. 2021. "Review on Local Buckling of Hollow Box FRP Profiles in Civil Structural Applications." Polymers. 13 (23), pp. 1-27. https://doi.org/10.3390/polym13234159Deterioration and damage identification in building structures using a novel feature selection method
Gharehbaghi, Vahid Reza, Farsangi, Ehsan Noroozinejad, Yang, T.Y. and Hajirasouliha, Iman. 2021. "Deterioration and damage identification in building structures using a novel feature selection method." Structures. 29, pp. 458-470. https://doi.org/10.1016/j.istruc.2020.11.040Modified plate frame interaction method for evaluation of steel plate shear walls with beam-connected web plates
Mamazizi, Arman, Khani, Shafi, Gharehbaghi, V.R. and Farsangi, Ehsan Noroozinejad. 2022. "Modified plate frame interaction method for evaluation of steel plate shear walls with beam-connected web plates." Journal of Building Engineering. 45. https://doi.org/10.1016/j.jobe.2021.103682Rehabilitation of SDOF systems under air blast loading with a modified negative stiffness amplifying damper
Kiran, K. K., Farsangi, Ehsan Noroozinejad, Gharehbaghi, Vahidreza and Bogdanovic, Aleksandra. 2022. "Rehabilitation of SDOF systems under air blast loading with a modified negative stiffness amplifying damper." Journal of Building Pathology and Rehabilitation. 7 (1). https://doi.org/10.1007/s41024-022-00178-xAn innovative methodology for hybrid vibration control (MR+TMD) of buildings under seismic excitations
Lavassani, Seyed Hossein Hosseini, Shangapour, Saman, Homami, Peyman, Gharehbaghi, Vahidreza, Farsangi, Ehsan Noroozinejad and Yang, T.Y.. 2022. "An innovative methodology for hybrid vibration control (MR+TMD) of buildings under seismic excitations." Soil Dynamics and Earthquake Engineering. 155. https://doi.org/10.1016/j.soildyn.2022.107175Interpretation of simultaneously optimized fuzzy controller and active tuned mass damper parameters under Pulse-type ground motions
Lavassani, Seyed Hossein Hosseini, Ebadijalal, Mehrdad, Shahrouzi, Mohsen, Gharehbaghi, Vahidreza, Farsangi, Ehsan Noroozinejad and Yang, T.Y.. 2022. "Interpretation of simultaneously optimized fuzzy controller and active tuned mass damper parameters under Pulse-type ground motions." Engineering Structures. 261. https://doi.org/10.1016/j.engstruct.2022.114286A novel approach for deterioration and damage identification in building structures based on Stockwell-Transform and deep convolutional neural network
Gharehbaghi, Vahid Reza, Kalbkhani, Hashem, Farsangi, Ehsan Noroozinejad, Yang, T.Y., Nguyen, Andy, Mirjalili, Seyedali and Malaga‑Chuquitaype, Christian. 2022. "A novel approach for deterioration and damage identification in building structures based on Stockwell-Transform and deep convolutional neural network." Journal of Structural Integrity and Maintenance. 7 (2), pp. 136-150. https://doi.org/10.1080/24705314.2021.2018840Influence of Filler Properties on the Axial Behaviour of Pultruded FRP Tubes
Al-Saadi, Ali Umran, Aravinthan, Thiru and Lokuge, Weena. 2022. "Influence of Filler Properties on the Axial Behaviour of Pultruded FRP Tubes ." 10th International Conference on FRP Composites in Civil Engineering (CICE 2020/2021). Istanbul, Turkey 08 - 10 Dec 2021 Switzerland . Springer. https://doi.org/10.1007/978-3-030-88166-5_43Latest Advances in Finite Element Modelling and Model Updating of Cable-Stayed Bridges
Sharry, Thomas, Guan, Hong, Nguyen, Andy, Oh, Erwin and Hoang, Nam. 2022. "Latest Advances in Finite Element Modelling and Model Updating of Cable-Stayed Bridges." Infrastructures. 7 (1), pp. 1-30. https://doi.org/10.3390/infrastructures7010008Back-propagation neural network optimized by K-fold cross-validation for prediction of torsional strength of reinforced concrete beam
Lyu, Zhaoqiu, Yu, Yang, Samali, Bijan, Rashidi, Maria, Mohammadi, Masoud, Nguyen, Thuc N. and Nguyen, Andy. 2022. "Back-propagation neural network optimized by K-fold cross-validation for prediction of torsional strength of reinforced concrete beam." Materials. 15 (4). https://doi.org/10.3390/ma15041477Next Generation “Living” Laboratory for Engineering Education and Engagement
Nguyen, Andy, Le, Ngoc Thach, Landers, Richard, Byrne, Terry, McDougall, Kevin, Gharehbaghi, Vahid Reza, Brown, Jason, Nguyen, Canh Long and Karunasena, Warna. 2022. "Next Generation “Living” Laboratory for Engineering Education and Engagement." 33rd Annual Conference of the Australasian Association of Engineering Education (AAEE 2022). Sydney, Australia 04 - 07 Dec 2022 Australia.Comparative study of damage detection in symmetric and asymmetric buildings
Wang, Y., Thambiratnam, D. P., Chan, T. H. T. and Nguyen, A.. 2017. "Comparative study of damage detection in symmetric and asymmetric buildings." Motavalli, Masoud, Ilki, Alper, Havranek, Bernadette and Inci, Pinar (ed.) 4th Conference on Smart Monitoring, Assessment and Rehabilitation of Civil Structures (SMAR 2017). Zurich, Switzerland 13 - 15 Sep 2017 Zurich, Switzerland.Deterioration sensitive feature using enhanced AR Model residuals
Monavari, Benyamin, Chan, Tommy H. T., Nguyen, Andy and Thambiratnam, David P.. 2017. "Deterioration sensitive feature using enhanced AR Model residuals." Motavalli, Masoud, Ilki, Alper, Havranek, Bernadette and Inci, Pinar (ed.) 4th Conference on Smart Monitoring, Assessment and Rehabilitation of Civil Structures (SMAR 2017). Zurich, Switzerland 13 - 15 Sep 2017 Zurich, Switzerland.Failure analysis and design of grouted fibre-composite repair system for corroded steel pipes
Shamsuddoha, Md, Manalo, Allan, Aravinthan, Thiru, Islam, Md Mainul and Djukic, Luke. 2021. "Failure analysis and design of grouted fibre-composite repair system for corroded steel pipes." Engineering Failure Analysis. 119, pp. 1-15. https://doi.org/10.1016/j.engfailanal.2020.104979Thermomechanical and fire performance of DGEBA based shape memory polymer composites for constructions
Emmanuel, K. D. C., Herath, H. M. C. M., Jeewantha, L. H. J., Epaarachchi, J. A. and Aravinthan, T.. 2021. "Thermomechanical and fire performance of DGEBA based shape memory polymer composites for constructions." Construction and Building Materials. 303. https://doi.org/10.1016/j.conbuildmat.2021.124442Modelling flexural performance of hollow pultruded FRP profiles
Alhawamdeh, Mohammad, Alajarmeh, Omar, Aravinthan, Thiru, Shelley, Tristan, Schubel, Peter, Mohammad, Ali and Zeng, Xuesen. 2021. "Modelling flexural performance of hollow pultruded FRP profiles." Composite Structures. 276. https://doi.org/10.1016/j.compstruct.2021.114553Predicting elastic modulus degradation of alkali silica reaction affected concrete using soft computing techniques: a comparative study
Yu, Yang, Nguyen, Thuc N., Li, Jianchun, Sanchez, Leandro F. M. and Nguyen, Andy. 2021. "Predicting elastic modulus degradation of alkali silica reaction affected concrete using soft computing techniques: a comparative study." Construction and Building Materials. 274, pp. 1-21. https://doi.org/10.1016/j.conbuildmat.2020.122024Recycling of landfill wastes (tyres, plastics and glass) in construction – A review on global waste generation, performance, application and future opportunities
Ferdous, Wahid, Manalo, Allan, Siddique, Rafat, Mendis, Priyan, Zhuge, Yan, Wong, Hong S., Lokuge, Weena, Aravinthan, Thiru and Schubel, Peter. 2021. "Recycling of landfill wastes (tyres, plastics and glass) in construction – A review on global waste generation, performance, application and future opportunities." Resources, Conservation and Recycling. 173, pp. 1-13. https://doi.org/10.1016/j.resconrec.2021.105745Modelling hollow pultruded FRP profiles under axial compression: Local buckling and progressive failure
Alhawamdeh, Mohammad, Alajarmeh, Omar, Aravinthan, Thiru, Shelley, Tristan, Schubel, Peter, Kemp, Michael and Zeng, Xuesen. 2021. "Modelling hollow pultruded FRP profiles under axial compression: Local buckling and progressive failure." Composite Structures. 262, pp. 1-14. https://doi.org/10.1016/j.compstruct.2021.113650Synergistic effects of hygrothermal conditions and solar ultraviolet radiation on the properties of structural particulate-filled epoxy polymer coatings
Mehrinejad Khotbehsara, Mojdeh, Manalo, Allan, Aravinthan, Thiru, Ferdous, Wahid, Benmokrane, Brahim and Nguyen, Kate T. Q.. 2021. "Synergistic effects of hygrothermal conditions and solar ultraviolet radiation on the properties of structural particulate-filled epoxy polymer coatings." Construction and Building Materials. 277. https://doi.org/10.1016/j.conbuildmat.2021.122336Effects of ultraviolet solar radiation on the properties of particulate-filled epoxy based polymer coating
Mehrinejad Khotbehsara, Mojdeh, Manalo, Allan, Aravinthan, Thiru, Turner, Joanna, Ferdous, Wahid and Hota, Gangarao. 2020. "Effects of ultraviolet solar radiation on the properties of particulate-filled epoxy based polymer coating." Polymer Degradation and Stability. 181, pp. 1-10. https://doi.org/10.1016/j.polymdegradstab.2020.109352Strengthening of headstocks in bridges with external post-tensioning
Aravinthan, Thiru. 2005. "Strengthening of headstocks in bridges with external post-tensioning." Andrews-Phaedonos, Fred, Corcoran, Brendan and Sanjayan, Jay (ed.) 22nd Biennial Conference of the Concrete Institute of Australia: Issues, Opportunities, Innovations. Melbourne, Australia 17 - 19 Oct 2005 Melbourne, Australia.Effect of thickness on the shape memory properties of bisphenol a epoxy based shape memory polymer composites
Emmanue, K. D. C., Jeewantha, J., Herath, M., Epaarachchi J. and Aravinthan T.. 2021. "Effect of thickness on the shape memory properties of bisphenol a epoxy based shape memory polymer composites." ASME 2021 Conference on Smart Materials, Adaptive Structures and Intelligent Systems (SMASIS2021). United States and Online United States. https://doi.org/10.1115/SMASIS2021-67111Effect of Deck Modelling Approaches on the Dynamic Analysis of a Cable-Stayed Bridge for Structural Health Monitoring
Sharry, Thomas, Guan, Hong, Nguyen, Andy, Hoang, Nam and Oh, Erwin. 2021. "Effect of Deck Modelling Approaches on the Dynamic Analysis of a Cable-Stayed Bridge for Structural Health Monitoring." Fifth Australasian Conference on Computational Mechanics (ACCM 2021). Sydney, Australia 13 - 15 Dec 2021 Australia. https://doi.org/10.26183/t5jn-8y77Compressive behaviour of hollow box pultruded FRP columns with continuous-wound fibres
Alajarmeh, Omar, Zeng, Xuesen, Aravinthan, Thiru, Shelley, Tristan, Alhawamdeh, Mohammad, Mohammed, Ali, Nicol, Lachlan, Vedernikov, Alexander, Safonov, Alexander and Schubel, Peter. 2021. "Compressive behaviour of hollow box pultruded FRP columns with continuous-wound fibres." Thin-Walled Structures. 168. https://doi.org/10.1016/j.tws.2021.108300Structural Deterioration Localisation Using Enhanced Autoregressive Time-Series Analysis
Monavari, Benyamin, Chan, Tommy H. T., Nguyen, Andy, Thambiratnam, David P. and Nguyen, Khac-Duy. 2020. "Structural Deterioration Localisation Using Enhanced Autoregressive Time-Series Analysis." International Journal of Structural Stability and Dynamics. 20 (10), pp. 2042013-1-2042013-27. https://doi.org/10.1142/S0219455420420134Locating and Quantifying Damage in Deck Type Arch Bridges Using Frequency Response Functions and Artificial Neural Networks
Jayasundara, N., Thambiratnam, D. P., Chan, T. H. T and Nguyen, A.. 2020. "Locating and Quantifying Damage in Deck Type Arch Bridges Using Frequency Response Functions and Artificial Neural Networks." International Journal of Structural Stability and Dynamics. 20 (10), pp. 2042010-1-2042010-25. https://doi.org/10.1142/S0219455420420109A Nonparametric Method for Identifying Structural Damage in Bridges Based on the Best-Fit Auto-Regressive Models
Khuc, Tung, Nguyen, Phat Tien, Nguyen, Andy and Catbas, F. Necati. 2020. "A Nonparametric Method for Identifying Structural Damage in Bridges Based on the Best-Fit Auto-Regressive Models." International Journal of Structural Stability and Dynamics. 20 (10), pp. 1-17. https://doi.org/10.1142/S0219455420420122Locating and Quantifying Damage in Beam-like Structures Using Modal Flexibility-based Deflection Changes
Le, N. T., Nguyen, A., Thambiratnam, D. P., Chan, T. H. T and Khuc, T.. 2020. "Locating and Quantifying Damage in Beam-like Structures Using Modal Flexibility-based Deflection Changes." International Journal of Structural Stability and Dynamics. 20 (10), pp. 2042008-1-2042008-20. https://doi.org/10.1142/S0219455420420080Preface: Recent Research Advances on Structural Health Monitoring of Civil Engineering Structures
Chan, Tommy H. T., Li, Jun, Nguyen, Andy and Yu, Tao. 2020. "Preface: Recent Research Advances on Structural Health Monitoring of Civil Engineering Structures." International Journal of Structural Stability and Dynamics. 20 (10), pp. 2002002-1-2002002-3. https://doi.org/10.1142/S0219455420020022Mechanical and thermal properties of glass fiber–vinyl ester resin composite for pipeline repair exposed to hot-wet conditioning
Shamsuddoha, Md, Djukic, Luke P., Islam, Md Mainul, Aravinthan, Thiru and Manalo, Allan. 2017. "Mechanical and thermal properties of glass fiber–vinyl ester resin composite for pipeline repair exposed to hot-wet conditioning." Journal of Composite Materials. 51 (11), pp. 1605-1617. https://doi.org/10.1177/0021998316661869State-of-the-art review on FRP sandwich systems for lightweight civil infrastructure
Manalo, Allan, Aravinthan, Thiru, Fam, Amir and Benmokrane, Brahim. 2017. "State-of-the-art review on FRP sandwich systems for lightweight civil infrastructure." Journal of Composites for Construction. 21 (1), pp. 1-16. https://doi.org/10.1061/(ASCE)CC.1943-5614.0000729Supervised damage and deterioration detection in building structures using an enhanced autoregressive time-series approach
Gharehbaghi, Vahid Reza, Nguyen, Andy, Farsangi, Ehsan Noroozinejad and Yang, T. Y.. 2020. "Supervised damage and deterioration detection in building structures using an enhanced autoregressive time-series approach." Journal of Building Engineering. 30, pp. 1-16. https://doi.org/10.1016/j.jobe.2020.101292Numerical Investigation on Hollow Pultruded Fibre Reinforced Polymer Tube Columns
Al-Saadi, A.U., Aravinthan, T. and Lokuge, W.. 2020. "Numerical Investigation on Hollow Pultruded Fibre Reinforced Polymer Tube Columns." Wang, Chien Ming, Ho, Johnny C.M. and Kitipornchai, Sritawat (ed.) 25th Australasian Conference on Mechanics of Structures and Materials (ACMSM25). Brisbane, Australia 04 - 07 Dec 2018 Singapore. https://doi.org/10.1007/978-981-13-7603-0_45Ageing of particulate-filled epoxy resin under hygrothermal conditions
Mehrinejad Khotbehsara, Mojdeh, Manalo, Allan, Aravinthan, Thiru, Ferdous, Wahid, Nguyen, Kate T.Q. and Hota, Gangarao. 2020. "Ageing of particulate-filled epoxy resin under hygrothermal conditions." Construction and Building Materials. 249, pp. 1-14. https://doi.org/10.1016/j.conbuildmat.2020.118846Performance of an innovative composite railway sleeper
Ferdous, Wahid, Manalo, Allan and Aravinthan, Thiru. 2017. "Performance of an innovative composite railway sleeper." 2017 Advanced Composites Innovation Conference. Gold Coast, Australia 28 - 30 Mar 2017Assessment of flexural stiffness and load carrying capacity using substructural system
Jamali, S., Koo, K. Y., Chan, T. H. T., Nguyen, A. and Thambiratnam, D. P.. 2018. "Assessment of flexural stiffness and load carrying capacity using substructural system." Chan, Tommy and Mahini, Saeed (ed.) 8th International Conference on Structural Health Monitoring of Intelligent Infrastructure (SHMII 2017): Structural Health Monitoring in Real-World Application. Brisbane, Australia 05 - 08 Dec 2017 Red Hook, NY, USA.Damage detection in arch bridges using vibration based damage detection techniques
Jayasundara, N., Thambiratnam, D. P., Chan, T. H. T. and Nguyen, A.. 2018. "Damage detection in arch bridges using vibration based damage detection techniques." Chan, Tommy and Mahini, Saeed (ed.) 8th International Conference on Structural Health Monitoring of Intelligent Infrastructure (SHMII 2017): Structural Health Monitoring in Real-World Application. Brisbane, Australia 05 - 08 Dec 2017 Red Hook, NY, USA.Finite element model updating of civil engineering infrastructures: a literature review
Moravej, H., Jamali, S., Chan, T. H. T. and Nguyen, A.. 2018. "Finite element model updating of civil engineering infrastructures: a literature review." Chan, Tommy and Mahini, Saeed (ed.) 8th International Conference on Structural Health Monitoring of Intelligent Infrastructure (SHMII 2017): Structural Health Monitoring in Real-World Application. Brisbane, Australia 05 - 08 Dec 2017 Red Hook, NY, USA.Time-series coefficient-based deterioration detection algorithm
Monavari, B., Chan, T. H. T., Nguyen, A. and Thambiratnam, D. P.. 2018. "Time-series coefficient-based deterioration detection algorithm." Chan, Tommy and Mahini, Saeed (ed.) 8th International Conference on Structural Health Monitoring of Intelligent Infrastructure (SHMII 2017): Structural Health Monitoring in Real-World Application. Brisbane, Australia 05 - 08 Dec 2017 Red Hook, NY, USA.Damage quantification in beam-like structures from modal flexibility change
Le, N. T., Thambiratnam, D. P., Chan, T. H. T and Nguyen, A.. 2018. "Damage quantification in beam-like structures from modal flexibility change." Chan, Tommy and Mahini, Saeed (ed.) 8th International Conference on Structural Health Monitoring of Intelligent Infrastructure (SHMII 2017): Structural Health Monitoring in Real-World Application. Brisbane, Australia 05 - 08 Dec 2017 Red Hook, NY, USA.Effect of elevated in-service temperature on the mechanical properties and microstructure of particulate-filled epoxy polymers
Mehrinejad Khotbehsara, Mojdeh, Manalo, Allan, Aravinthan, Thiru, Reddy, Kakarla Raghava, Ferdous, Wahid, Wong, Hong and Nazari, Ali. 2019. "Effect of elevated in-service temperature on the mechanical properties and microstructure of particulate-filled epoxy polymers." Polymer Degradation and Stability. 170, pp. 1-15. https://doi.org/10.1016/j.polymdegradstab.2019.108994Damage detection and quantification in deck type arch bridges using vibration based methods and artificial neural networks
Jayasundara, N., Thambiratnam, D. P., Chan, T. H. T. and Nguyen, A.. 2020. "Damage detection and quantification in deck type arch bridges using vibration based methods and artificial neural networks." Engineering Failure Analysis. 109, pp. 1-19. https://doi.org/10.1016/j.engfailanal.2019.104265Toward efficacy of piecewise polynomial truncated singular value decomposition algorithm in moving force identification
Chen, Zhen, Qin, Lifeng, Zhao, Shunbo, Chan, Tommy H. T. and Nguyen, Andy. 2019. "Toward efficacy of piecewise polynomial truncated singular value decomposition algorithm in moving force identification." Advances in Structural Engineering: an international journal. 22 (12), pp. 2687-2698. https://doi.org/10.1177/1369433219849817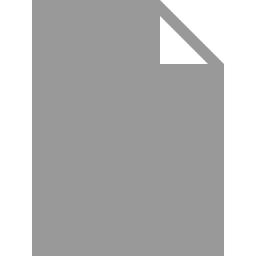