Monte Carlo Simulation, Artificial Intelligence and Machine Learning-based Modelling and Optimization of Three-dimensional Electrochemical Treatment of Xenobiotic Dye Wastewater
Article
Ganthavee, Voravich, Fernando, Merenghege M. R. and Trzcinski, Antoine P.. 2024. " Monte Carlo Simulation, Artificial Intelligence and Machine Learning-based Modelling and Optimization of Three-dimensional Electrochemical Treatment of Xenobiotic Dye Wastewater." Environmental Processes. 11 (3). https://doi.org/10.1007/s40710-024-00719-1
Article Title | Monte Carlo Simulation, Artificial Intelligence and Machine Learning-based Modelling and Optimization of Three-dimensional Electrochemical Treatment of Xenobiotic Dye Wastewater |
---|---|
Article Category | Article |
Authors | Ganthavee, Voravich, Fernando, Merenghege M. R. and Trzcinski, Antoine P. |
Journal Title | Environmental Processes |
Journal Citation | 11 (3) |
Article Number | 41 |
Number of Pages | 31 |
Year | 2024 |
Publisher | Springer |
Place of Publication | Germany |
ISSN | 2198-7491 |
2198-7505 | |
Digital Object Identifier (DOI) | https://doi.org/10.1007/s40710-024-00719-1 |
Web Address (URL) | https://link.springer.com/article/10.1007/s40710-024-00719-1 |
Abstract | The present study investigates the synergistic performance of the three-dimensional electrochemical process to decolourise methyl orange (MO) dye pollutant from xenobiotic textile wastewater. The textile dye was treated using electrochemical technique with strong oxidizing potential, and additional adsorption technology was employed to effectively remove dye pollutants from wastewater. Approximately 98% of MO removal efficiency was achieved using 15 mA/cm2 of current density, 3.62 kWh/kg of energy consumption and 79.53% of current efficiency. The 50 mg/L MO pollutant was rapidly mineralized with a half-life of 4.66 min at a current density of 15 mA/cm2. Additionally, graphite intercalation compound (GIC) was electrically polarized in the three-dimensional electrochemical reactor to enhance the direct electrooxidation and.OH generation, thereby improving synergistic treatment efficiency. Decolourisation of MO-polluted wastewater was optimized by artificial intelligence (AI) and machine learning (ML) techniques such as Artificial Neural Networks (ANN), Support Vector Machine (SVM), and random forest (RF) algorithms. Statistical metrics indicated the superiority of the model followed this order: ANN > RF > SVM > Multiple regression. The optimization results of the process parameters by artificial neural network (ANN) and random forest (RF) approaches showed that a current density of 15 mA/cm2, electrolysis time of 30 min and initial MO concentration of 50 mg/L were the best operating parameters to maintain current and energy efficiencies of the electrochemical reactor. Finally, Monte Carlo simulations and sensitivity analysis showed that ANN yielded the best prediction efficiency with the lowest uncertainty and variability level, whereas the predictive outcome of random forest was slightly better. |
Keywords | Adsorption and electrochemical treatment; Dye removal ; Artifcial neural network; Support vector machine; Random forest ; Monte Carlo simulation |
Related Output | |
Is part of | Superior decomposition of xenobiotic dyes and pharmaceutical contaminants in wastewater using response surface methodology, artificial intelligence and machine learning for optimisation of a novel three-dimensional electrochemical technology |
Contains Sensitive Content | Does not contain sensitive content |
ANZSRC Field of Research 2020 | 400410. Wastewater treatment processes |
Public Notes | This article is part of a UniSQ Thesis by publication. See Related Output. |
Byline Affiliations | School of Agriculture and Environmental Science |
Permalink -
https://research.usq.edu.au/item/z99vq/-monte-carlo-simulation-artificial-intelligence-and-machine-learning-based-modelling-and-optimization-of-three-dimensional-electrochemical-treatment-of-xenobiotic-dye-wastewater
Download files
73
total views26
total downloads7
views this month1
downloads this month
Export as
Related outputs
Fermentation of sorghum with Aspergillus strains: A promising and sustainable pathway to enzyme production- comprehensive review
Somadder, Pratul Dipta, Trzcinski, Antoine, Chen, Guangnan, Chow, Yvonne and Manan, Musaalbakri Abdul. 2025. "Fermentation of sorghum with Aspergillus strains: A promising and sustainable pathway to enzyme production- comprehensive review ." Renewable and Sustainable Energy Reviews. 213. https://doi.org/10.1016/j.rser.2025.115456Hydrodynamic performance of algal bioreactors
Zarei, Zahra, Malekshahi, Peyman, Morowvat, Mohammad Hossein and Trzcinski, Antoine P.. 2025. "Hydrodynamic performance of algal bioreactors." Jacob-Lopez, Eduardo, Zepka, Leila Queiroz and Depra, Mariany Costa (ed.) Algal bioreactors: Science, Engineering and technology of upstream processes. United States. Elsevier. pp. 121-133Degradation of Diclofenac Using Advanced Oxidation Processes: a Review
Ahmed, Riaz Ahmed, Lau, Lau Poh and Trzcinski, Antoine Prandota. 2025. "Degradation of Diclofenac Using Advanced Oxidation Processes: a Review ." Journal of Environmental Science and Health Part A: Toxic Hazardous Substances and Environmental Engineering. 60 (1), pp. 12-28. https://doi.org/10.1080/10934529.2025.2472116Superior decomposition of xenobiotic RB5 dye using three-dimensional electrochemical treatment: Response surface methodology modelling, artificial intelligence, and machine learning-based optimisation approaches
Ganthavee, Voravich and Trzcinski, Antoine P.. 2025. "Superior decomposition of xenobiotic RB5 dye using three-dimensional electrochemical treatment: Response surface methodology modelling, artificial intelligence, and machine learning-based optimisation approaches." Water Science and Engineering. 18 (1), pp. 1-10. https://doi.org/10.1016/j.wse.2024.05.003Removal of reactive black 5 in water using adsorption and electrochemical oxidation technology: kinetics, isotherms and mechanisms
Ganthavee, V. and Trzcinski, A. P.. 2025. "Removal of reactive black 5 in water using adsorption and electrochemical oxidation technology: kinetics, isotherms and mechanisms." International Journal of Environmental Science and Technology. 22 (2), pp. 1083-1106. https://doi.org/10.1007/s13762-024-05696-4Potential Utilisation of Organic Waste in Concrete at OT Mine: Exploring Mechanical Properties of Amended Concrete (Phase 2)
Mirzaghorbanali, Ali, McDougall, Kevin, Nourizadeh, Hadi, Karunasena, Karu, Trzcinski, Antoine, Entezam, Alireza and Ganthavee, Voravich. 2024. Potential Utilisation of Organic Waste in Concrete at OT Mine: Exploring Mechanical Properties of Amended Concrete (Phase 2). Australia. University of Southern Queensland. https://doi.org/10.26192/zx4q2Mechanical and Tribological Performance of Epoxy Composites Reinforced with YSZ Waste Ceramics for Sustainable Green Engineering Applications
Alsaeed, Talal, Alajmi, Ayedh Eid, Alotaibi, Jasem Ghanem, Ganthavee, Voravich and Yousif, Belal F.. 2024. "Mechanical and Tribological Performance of Epoxy Composites Reinforced with YSZ Waste Ceramics for Sustainable Green Engineering Applications." Processes. 12 (11). https://doi.org/10.3390/pr12112609Superior decomposition of xenobiotic dyes and pharmaceutical contaminants in wastewater using response surface methodology, artificial intelligence and machine learning for optimisation of a novel three-dimensional electrochemical technology
Ganthavee, Voravich. 2024. Superior decomposition of xenobiotic dyes and pharmaceutical contaminants in wastewater using response surface methodology, artificial intelligence and machine learning for optimisation of a novel three-dimensional electrochemical technology. PhD by Publication Doctor of Philosophy. University of Southern Queensland. https://doi.org/10.26192/zqyq2Editorial: Innovative treatment technologies for sustainable water and wastewater management
Mojiri, Amin, Trzcinski, Antoine P., Bashir, Mohammed J. K. and Abu Amr, Salem S.. 2024. "Editorial: Innovative treatment technologies for sustainable water and wastewater management ." Frontiers in Water. 6. https://doi.org/10.3389/frwa.2024.1388387Comparison of perfluorooctane sulfonate (PFOS), perfluorooctanoic acid (PFOA) and perfluorobutane sulfonate (PFBS) removal in a combined adsorption and electrochemical oxidation process
Trzcinski, Antoine P. and Harada, Kouji H.. 2024. "Comparison of perfluorooctane sulfonate (PFOS), perfluorooctanoic acid (PFOA) and perfluorobutane sulfonate (PFBS) removal in a combined adsorption and electrochemical oxidation process." Science of the Total Environment. 927. https://doi.org/10.1016/j.scitotenv.2024.172184A review of modified and hybrid anaerobic baffled reactors for municipal wastewater treatment with a focus on emerging contaminants
Lau, Poh Lin and Trzcinski, Antoine P.. 2024. "A review of modified and hybrid anaerobic baffled reactors for municipal wastewater treatment with a focus on emerging contaminants." Environmental Science: Water Research and Technology. 10 (6), pp. 1335-1354. https://doi.org/10.1039/d3ew00822cArtificial intelligence and machine learning for the optimization of pharmaceutical wastewater treatment systems: a review
Ganthavee, Voravich and Trzcinski, Antoine Prandota. 2024. "Artificial intelligence and machine learning for the optimization of pharmaceutical wastewater treatment systems: a review." Environmental Chemistry Letters. 22 (5), pp. 2293-2318. https://doi.org/10.1007/s10311-024-01748-wCombined adsorption and electrochemical oxidation of perfluorooctanoic acid (PFOA) using graphite intercalated compound
Trzcinski, Antoine P. and Harada, Kouji. 2024. "Combined adsorption and electrochemical oxidation of perfluorooctanoic acid (PFOA) using graphite intercalated compound." Environmental Science and Pollution Research. 31 (13). https://doi.org/10.1007/s11356-024-32449-0Impact of polystyrene microplastics on the growth and photosynthetic efficiency of diatom Chaetoceros neogracile
Mojiri, Amin, Vishkaei, Mansoureh Nazari, Zhou, John L., Trzcinski, Antoine P., Lou, Ziyang, Kasmuri, Norhafezah, Rezania, Shahabaldin, Gholami, Ali, Vakili, Mohammadtaghi and Kazeroon, Reza Andasht. 2024. "Impact of polystyrene microplastics on the growth and photosynthetic efficiency of diatom Chaetoceros neogracile." Marine Environmental Research. 194. https://doi.org/10.1016/j.marenvres.2024.106343A review of bioreactor configurations for hydrogen production by cyanobacteria and microalgae
Zarei, Zahra, Malekshahi, Peyman, Morowvat, Mohammad Hossein and Trzcinski, Antoine P.. 2024. "A review of bioreactor configurations for hydrogen production by cyanobacteria and microalgae." International Journal of Hydrogen Energy. 49 (Part A), pp. 472-495. https://doi.org/10.1016/j.ijhydene.2023.09.108Potential utilisation of organic waste in concrete at OT mine: Project Progress Report – Phase 1
Mirzaghorbanali, Ali, McDougall, Kevin, Karunasena, Karu, Trzcinski, Antoine, Eberhard, Susette, Nourizadeh, Hadi and Weerasundara, Lakshika. 2023. Potential utilisation of organic waste in concrete at OT mine: Project Progress Report – Phase 1 . Australia. University of Southern Queensland. https://doi.org/10.26192/zx49zImproving the Quality of Set Yoghurt Using Milk Fat Globule Membrane Fragments
Ibitoye, Ibitoye, Phan, T. T. Q., Le, Duy Nghia, Dewettinck, K., Colgrave, Michelle L., Trzcinski, A. P. and Ly-Nguyen, B.. 2023. "Improving the Quality of Set Yoghurt Using Milk Fat Globule Membrane Fragments." Journal of Food Processing and Preservation. 2023. https://doi.org/10.1155/2023/6875570Removal of pharmaceutically active compounds from wastewater using adsorption coupled with electrochemical oxidation technology: A critical review
Ganthavee, Voravich and Trzcinski, Antoine P.. 2023. "Removal of pharmaceutically active compounds from wastewater using adsorption coupled with electrochemical oxidation technology: A critical review." Journal of Industrial and Engineering Chemistry. 126, pp. 20-35. https://doi.org/10.1016/j.jiec.2023.06.003Adsorption of PFOS onto graphite intercalated compound and analysis of degradation by-products during electro-chemical oxidation
Trzcinski, Antoine P. and Harada, Kouji. 2023. "Adsorption of PFOS onto graphite intercalated compound and analysis of degradation by-products during electro-chemical oxidation ." Chemosphere. 323. https://doi.org/10.1016/j.chemosphere.2023.138268Effect of hydrodynamic parameters on hydrogen production by Anabaena sp. in an internal‑loop airlift photobioreactor
Zarei, Zahra, Malekshahi, Peyman, Trzcinski, Antoine P. and Morowvat, Mohammad Hossein. 2023. "Effect of hydrodynamic parameters on hydrogen production by Anabaena sp. in an internal‑loop airlift photobioreactor." Brazilian Journal of Chemical Engineering. 40, p. 379–388. https://doi.org/10.1007/s43153-022-00245-3Investigation of Hydrodynamic Parameters in an Airlift Photobioreactor on CO2 Biofixation by Spirulina sp.
Zarei, Zahra, Malekshahi, Peyman, Trzcinski, Antoine P. and Morowvat, Mohammad Hossein. 2022. "Investigation of Hydrodynamic Parameters in an Airlift Photobioreactor on CO2 Biofixation by Spirulina sp." Sustainability. 14. https://doi.org/10.3390/su14127503A review of modified and hybrid anaerobic baffled reactors for industrial wastewater treatment
Lau, Poh Lin and Trzcinski, Antoine. 2022. "A review of modified and hybrid anaerobic baffled reactors for industrial wastewater treatment." Water Science and Engineering. 15 (3), pp. 247-256. https://doi.org/10.1016/j.wse.2022.06.004Comment on: 'Associations of Prenatal Exposure to Per- and Polyfluoroalkyl Substances with the Neonatal Birth Size and Hormones in the Growth Hormone/Insulin-Like Growth Factor Axis': What Is the Origin of PFHxS Found in the Human Body?
Fujii, Yukiko, Harada, Kouji H. and Trzcinski, Antoine P.. 2022. "Comment on: 'Associations of Prenatal Exposure to Per- and Polyfluoroalkyl Substances with the Neonatal Birth Size and Hormones in the Growth Hormone/Insulin-Like Growth Factor Axis': What Is the Origin of PFHxS Found in the Human Body?" Environmental Science and Technology. 56 (8), pp. 5283-5284. https://doi.org/10.1021/acs.est.1c08190The impact of climate change on waterborne diseases in Pakistan
Noureen, Afshan, Aziz, Rabia, Ismail, Abdullah and Trzcinski, Antoine P.. 2022. "The impact of climate change on waterborne diseases in Pakistan." Sustainability and Climate Change. 15 (2), pp. 138-152. https://doi.org/10.1089/scc.2021.0070Conflict-related environmental damages on health: lessons learned from the past wars and ongoing Russian invasion of Ukraine
Harada, Kouji H., Soleman, Sani Rachman, Ang, Jeremy Sea Meng and Trzcinski, Antoine P.. 2022. "Conflict-related environmental damages on health: lessons learned from the past wars and ongoing Russian invasion of Ukraine." Environmental Health and Preventive Medicine. 27. https://doi.org/10.1265/ehpm.22-00122Discerning potable water sources using Monte Carlo based simulation for health risk assessment in Omu-Aran, Nigeria
Elemile, O.O., Ibitogbe, E.M., Obayomi, K.S., Trzcinski, P.A., Falana, B.M., Okon, E.M., Olajide, S.O., Arije, D., Tokula, B.E. and Ibitoye, O.O.. 2022. "Discerning potable water sources using Monte Carlo based simulation for health risk assessment in Omu-Aran, Nigeria." Groundwater for Sustainable Development. 19. https://doi.org/10.1016/j.gsd.2022.100843Quality of set yogurts made from raw milk and processed milk supplemented with enriched milk fat globule membrane in a two-stage homogenization process
Ibitoye, Joshua Oladapo, Ly-Nguyen, Binh, Le, Duy Nghia, Dewettinck, Koen, Trzcinski, Antoine P. and Phan, Thi Thanh Que. 2021. "Quality of set yogurts made from raw milk and processed milk supplemented with enriched milk fat globule membrane in a two-stage homogenization process." Foods. 10. https://doi.org/10.3390/foods10071534Investigation of methane production by anaerobic co-digestion of food waste, fats, oil and grease, and thickened waste activated sludge using automatic methane potential test system
Bahri, Nikita and Trzcinski, Antoine. 2017. "Investigation of methane production by anaerobic co-digestion of food waste, fats, oil and grease, and thickened waste activated sludge using automatic methane potential test system." 2017 MENS Research Conference. Toowoomba, AustraliaAdvanced biological, physical and chemical treatment of waste activated sludge
Trzcinski, Antoine Prandota. 2019. Advanced biological, physical and chemical treatment of waste activated sludge. Boca Raton, FL, United States. CRC Press.Sludge pretreatment methods for enhanced VS destruction and CH4 production
Trzcinski, A., Tian, X., Lin, L., Guo, Z. H., Zhou, Y. and Ng, Wun Jern. 2014. "Sludge pretreatment methods for enhanced VS destruction and CH4 production." Wastewater and Biosolids Treatment and Reuse (WBTR2014): Bridging modelling and experimental studies. Otranto, Italy 08 - 14 Jun 2014Effects of physico-chemical post-treatments on the semi-continuous anaerobic digestion of sewage sludge
Tian, Xinbo and Trzcinski, Antoine. 2018. "Effects of physico-chemical post-treatments on the semi-continuous anaerobic digestion of sewage sludge." Estokova, Adriana and Balintova, Magdalena (ed.) Advances in environmental engineering. Basel, Switzerland. MDPI AG. pp. 22-33Growth kinetics and lipid accumulation in Rhodosporidium toruloides
Trzcinski, Antoine. 2010. Growth kinetics and lipid accumulation in Rhodosporidium toruloides. Unpublished.A feasibility study into the bioprocessing of coffee residues
Trzcinski, Antoine. 2010. A feasibility study into the bioprocessing of coffee residues. Unpublished.Water balance Excel sheet to calculate water balance in a chemical plant
Trzcinski, Antoine. 2013. Water balance Excel sheet to calculate water balance in a chemical plant. Unpublished.Treatment of waste pickling baths from a batch hot-dip galvanizing plant
Trzcinski, Antoine. 2005. Treatment of waste pickling baths from a batch hot-dip galvanizing plant. Masters Thesis Master in Chemical Engineering. Catholic University of Louvain-la-Neuve.Excel program to design the main units in an algae biorefinery
Trzcinski, Antoine. 2011. Excel program to design the main units in an algae biorefinery. Unpublished.Developing novel biorefineries using food waste as substrate (Technical report 4)
Kiran, Esra Uckun, Trzcinski, Antoine P. and Liu, Yu. 2015. Developing novel biorefineries using food waste as substrate (Technical report 4). Singapore. National Environment Agency.Developing novel biorefineries using food waste as substrate (Technical report 3)
Kiran, Esra Uckun, Trzcinski, Antoine P. and Liu, Yu. 2014. Developing novel biorefineries using food waste as substrate (Technical report 3). Singapore. National Environment Agency.Developing novel biorefineries using food waste as substrate (Technical report 2)
Kiran, Esra Uckun, Trzcinski, Antoine P. and Liu, Yu. 2013. Developing novel biorefineries using food waste as substrate (Technical report 2). Singapore. National Environment Agency.Developing novel biorefineries using food waste as substrate (Technical report 1)
Kiran, Esra Uckun, Trzcinski, Antoine P. and Liu, Yu. 2013. Developing novel biorefineries using food waste as substrate (Technical report 1). Singapore. National Environment Agency.Anaerobic membrane bioreactor technology for solid waste stabilization
Trzcinski, Antoine Prandota. 2009. Anaerobic membrane bioreactor technology for solid waste stabilization. PhD Thesis Doctor of Philosophy. Imperial College London.Sample characterization and determination of anaerobic biodegradability of sludges drawn from biosorption plants located at Jurong WRP and at Ulu Pandan WRP (Final report)
Ng, Wun Jern, Trzcinski, Antoine P. and Ganda, Lily. 2015. Sample characterization and determination of anaerobic biodegradability of sludges drawn from biosorption plants located at Jurong WRP and at Ulu Pandan WRP (Final report). Unpublished.Analysis of samples and anaerobic biodegradability of sludges from Jurong and Ulu Pandan water reclamation plants (WRPs) (Report 8)
Ng, Wun Jern, Trzcinski, Antoine P. and Ganda, Lily. 2015. Analysis of samples and anaerobic biodegradability of sludges from Jurong and Ulu Pandan water reclamation plants (WRPs) (Report 8). Unpublished.Analysis of samples and anaerobic biodegradability of sludges from Jurong and Ulu Pandan water reclamation plants (WRPs) (Report 5)
Ng, Wun Jern, Trzcinski, Antoine P. and Ganda, Lily. 2014. Analysis of samples and anaerobic biodegradability of sludges from Jurong and Ulu Pandan water reclamation plants (WRPs) (Report 5). Unpublished.Analysis of samples and anaerobic biodegradability of sludges from Jurong and Ulu Pandan water reclamation plants (WRPs) (Report 7)
Ng, Wun Jern, Trzcinski, Antoine P. and Ganda, Lily. 2015. Analysis of samples and anaerobic biodegradability of sludges from Jurong and Ulu Pandan water reclamation plants (WRPs) (Report 7). Unpublished.Analysis of samples and anaerobic biodegradability of sludges from Jurong and Ulu Pandan water reclamation plants (WRPs) (Report 6)
Ng, Wun Jern, Trzcinski, Antoine P. and Ganda, Lily. 2014. Analysis of samples and anaerobic biodegradability of sludges from Jurong and Ulu Pandan water reclamation plants (WRPs) (Report 6). Unpublished.Analysis of samples and anaerobic biodegradability of sludges from Jurong and Ulu Pandan water reclamation plants (WRPs) (Report 4)
Ng, Wun Jern, Trzcinski, Antoine P. and Ganda, Lily. 2014. Analysis of samples and anaerobic biodegradability of sludges from Jurong and Ulu Pandan water reclamation plants (WRPs) (Report 4). Unpublished.Analysis of samples and anaerobic biodegradability of sludges from Jurong and Ulu Pandan water reclamation plants (WRPs) (Report 3)
Ng, Wun Jern, Trzcinski, Antoine P. and Ganda, Lily. 2014. Analysis of samples and anaerobic biodegradability of sludges from Jurong and Ulu Pandan water reclamation plants (WRPs) (Report 3). Unpublished.Analysis of samples and anaerobic biodegradability of sludges from Jurong and Ulu Pandan water reclamation plants (Report 2)
Ng, Wun Jern, Trzcinski, Antoine P. and Ganda, Lily. 2013. Analysis of samples and anaerobic biodegradability of sludges from Jurong and Ulu Pandan water reclamation plants (Report 2). Unpublished.Analysis of samples and anaerobic biodegradability of sludges from Jurong and Ulu Pandan water reclamation plants (Report 1)
Ng, Wun Jern, Trzcinski, Antoine P. and Ganda, Lily. 2013. Analysis of samples and anaerobic biodegradability of sludges from Jurong and Ulu Pandan water reclamation plants (Report 1). Unpublished.Anaerobic digester feed stream conditioning for increasing biogas production (Technical report 8)
Ng, Wun Jern, Trzcinski, Antoine, Wang, Chong and Tian, Xinbo. 2014. Anaerobic digester feed stream conditioning for increasing biogas production (Technical report 8). Unpublished.Anaerobic digester feed stream conditioning for increasing biogas production (Technical report 7)
Ng, Wun Jern, Trzcinski, Antoine, Wang, Chong and Tian, Xinbo. 2013. Anaerobic digester feed stream conditioning for increasing biogas production (Technical report 7). Unpublished.Anaerobic digester feed stream conditioning for increasing biogas production (Technical report 6)
Ng, Wun Jern, Trzcinski, Antoine and Tian, Xinbo. 2013. Anaerobic digester feed stream conditioning for increasing biogas production (Technical report 6). Unpublished.Anaerobic digester feed stream conditioning for increasing biogas production (Technical report 5)
Ng, Wun Jern, Trzcinski, Antoine, Wang, Chong and Tian, Xinbo. 2013. Anaerobic digester feed stream conditioning for increasing biogas production (Technical report 5). Unpublished.Anaerobic digester feed stream conditioning for increasing biogas production (Technical report 4)
Ng, Wun Jern, Trzcinski, Antoine, Wang, Chong and Tian, Xinbo. 2013. Anaerobic digester feed stream conditioning for increasing biogas production (Technical report 4). Unpublished.Anaerobic digestion design decision tool with economic analysis (NPV)
Trzcinski, A.. 2016. Anaerobic digestion design decision tool with economic analysis (NPV). Unpublished.Anaerobic digester feed stream conditioning for increasing biogas production (Technnical report 3)
Ng, Wun Jern, Trzcinski, Antoine, Wang, Chong and Tian, Xinbo. 2013. Anaerobic digester feed stream conditioning for increasing biogas production (Technnical report 3). Singapore. Nanyang Technological University.Anaerobic digester feed stream conditioning for increasing biogas production (Technical report 2)
Ng, Wun Jern, Trzcinski, Antoine, Wang, Chong and Tian, Xinbo. 2012. Anaerobic digester feed stream conditioning for increasing biogas production (Technical report 2). Singapore. Nanyang Technological University.Anaerobic digester feed stream conditioning for increasing biogas production (Technical report 1)
Ng, Wun Jern, Trzcinski, Antoine, Wang, Chong and Tian, Xinbo. 2012. Anaerobic digester feed stream conditioning for increasing biogas production (Technical report 1). Singpaore. Nanyang Technological University.Optimizing the synergistic effect of sodium hydroxide/ultrasound pre-treatment of sludge
Tian, Xinbo, Ng, Wun Jern and Trzcinski, Antoine P.. 2018. "Optimizing the synergistic effect of sodium hydroxide/ultrasound pre-treatment of sludge." Ultrasonics Sonochemistry. 48, pp. 432-440. https://doi.org/10.1016/j.ultsonch.2018.07.005Direct Sugarcane Bagasse to oil (SB2O)
Trzcinski, Antoine, Hernandez, Ernesto and Webb, Colin. 2011. Direct Sugarcane Bagasse to oil (SB2O). United Kingdom. University of Manchester.Added-value processing of ‘algae waste': 4th quarterly report
Trzcinski, Antoine, Hernandez, Ernesto and Webb, Colin. 2011. Added-value processing of ‘algae waste': 4th quarterly report. University of Manchester.Added-value processing of ‘algae waste': 3rd quarterly report
Trzcinski, Antoine, Hernandez, Ernesto and Webb, Colin. 2010. Added-value processing of ‘algae waste': 3rd quarterly report. United Kingdom. University of Manchester.Added-value processing of ‘algae waste': 2nd quarterly report
Trzcinski, Antoine, Hernandez, Ernesto and Webb, Colin. 2010. Added-value processing of ‘algae waste': 2nd quarterly report. United Kingdom. University of Manchester.Added-value processing of ‘algae waste': 1st quarterly repot
Trzcinski, Antoine, Hernandez, Erbesto and Webb, Colin. 2009. Added-value processing of ‘algae waste': 1st quarterly repot. United Kingdom. University of Manchester.Developing novel biorefineries using food waste as substrate
Kiran, Esra Uckun, Trzcinski, Antoine P. and Liu, Yu. 2014. "Developing novel biorefineries using food waste as substrate." 248th American Chemical Society National Meeting & Exposition. San Francisco, California 10 - 14 Aug 2014Biomass & biogas developments in Singapore
Trzcinski, A. and Ng, W. J.. 2014. "Biomass & biogas developments in Singapore." Biomass & Biogas Opportunities Seminar 2014. Singapore 20 Mar 2014The modern alchemy or How to convert Municipal Solid Wastes(MSW) into biogas?
Trzcinski, A. P. and Stuckey, D. C.. 2006. "The modern alchemy or How to convert Municipal Solid Wastes(MSW) into biogas?" 7th UK Meeting IWA UK Young Researchers Conference 2006. Bath, United Kingdom 04 - 05 Apr 2006Feasibility of coupling ultrasound with chemical methods for sludge pre-treatment
Tian, Xinbo, Trzcinski, Antoine Prandota and Ng, Wun Jern. 2013. "Feasibility of coupling ultrasound with chemical methods for sludge pre-treatment." Postgraduate Workshop (2013). Darmstadt, Germany 25 Nov 2013Industrial wastewater treatment process
Trzcinski, Antoine P. and Ng, Wun Jern. 2013. "Industrial wastewater treatment process." 4th Global Ethanol Focus 2013: Sustainable Cooperation and Development Focus on Ethanol. Singapore 04 - 05 Sep 2013Optimizing the conversion of food waste to sugars using fungal enzymes
Kiran, Esra Uckun and Trzcinski, Antoine. 2017. "Optimizing the conversion of food waste to sugars using fungal enzymes." EC Microbiology. 8.3, pp. 98-112.Continuous treatment of the organic fraction of municipal solid waste in an anaerobic two-stage membrane process with liquid recycle
Trzcinski, A. P. and Stuckey, D. C.. 2009. "Continuous treatment of the organic fraction of municipal solid waste in an anaerobic two-stage membrane process with liquid recycle." Water Research. 43 (9), pp. 2449-2462. https://doi.org/10.1016/j.watres.2009.03.030Fate and behavior of dissolved organic matter in a submerged anoxic-aerobic membrane bioreactor (MBR)
Zhang, Dongqing, Trzcinski, Antoine Prandota, Luo, Jinxue, Stuckey, David C. and Tan, Soon Keat. 2018. "Fate and behavior of dissolved organic matter in a submerged anoxic-aerobic membrane bioreactor (MBR)." Environmental Science and Pollution Research. 25 (5), pp. 4289-4302. https://doi.org/10.1007/s11356-017-0586-xMicrobial stress mediated intercellular nanotubes in an anaerobic microbial consortium digesting cellulose
John, Martina, Trzcinski, Antoine Prandota, Zhou, Yan and Ng, Wun Jern. 2017. "Microbial stress mediated intercellular nanotubes in an anaerobic microbial consortium digesting cellulose." Scientific Reports. 7. https://doi.org/10.1038/s41598-017-18198-wMicrobial biomethane from solid waste: biotechnological process
Trzcinski, Antoine P. and Stuckey, David C.. 2018. "Microbial biomethane from solid waste: biotechnological process." Harzevili, Farshad Darvishi and Hiligsmann, Serge (ed.) Microbial fuels: technologies and applications. Boca Raton, FL, United States. CRC Press. pp. 77-151Biological treatment of leachate in a submerged anaerobic membrane bioreactor
Trzcinski, Antoine P.. 2017. "Biological treatment of leachate in a submerged anaerobic membrane bioreactor." Mattox, Maria R. (ed.) Leachates: biological treatment, toxicological characteristics and potential impacts on the environment. New York, United States. Nova Science Publishers. pp. 1-122Stepwise alkaline/ultrasound pretreatment of waste activated sludge prior to anaerobic digestion
Trzcinski, Antoine Prandota, Tian, Xinbo, Ng, Wun Jern and Wang, Chong. 2018. Stepwise alkaline/ultrasound pretreatment of waste activated sludge prior to anaerobic digestion. 10201405550UBioconversion of food waste to energy: a review
Kiran, Esra Uckun, Trzcinski, Antoine, Ng, Wun Jern and Liu, Yu. 2014. "Bioconversion of food waste to energy: a review." Fuel: the science and technology of fuel and energy. 134, pp. 389-399. https://doi.org/10.1016/j.fuel.2014.05.074Future scenarios modeling of urban stormwater management response to impacts of climate change and urbanization
Wang, Mo, Zhang, Dong Q., Su, Jin, Trzcinski, Antoine P., Dong, Jian W. and Tan, Soon K.. 2017. "Future scenarios modeling of urban stormwater management response to impacts of climate change and urbanization." CLEAN: Soil, Air, Water. 45 (10), pp. 1-10. https://doi.org/10.1002/clen.201700111Comparison of the effects and distribution of zinc oxide nanoparticles and zinc ions in activated sludge reactors
Zhang, Dongqing, Trzcinski, Antoine, Oh, Hyun-Suk, Chew, Evelyn, Liu, Yu, Tan, Soon Keat and Ng, Wun Jern. 2017. "Comparison of the effects and distribution of zinc oxide nanoparticles and zinc ions in activated sludge reactors." Journal of Environmental Science and Health Part A: Toxic Hazardous Substances and Environmental Engineering. 52 (11), pp. 1073-1081. https://doi.org/10.1080/10934529.2017.1338896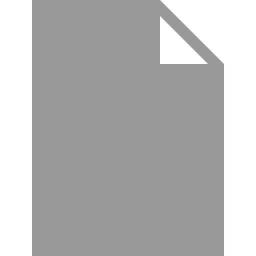