Assessing Nitrate Contamination Risks in Groundwater: A Machine Learning Approach
Article
Article Title | Assessing Nitrate Contamination Risks in Groundwater: A Machine Learning Approach |
---|---|
ERA Journal ID | 211776 |
Article Category | Article |
Authors | Awais, Muhammad (Author), Aslam, Bilal (Author), Maqsoom, Ahsen (Author), Khalil, Umer (Author), Ullah, Fahim (Author), Azam, Sheheryar (Author) and Imran, Muhammad (Author) |
Journal Title | Applied Sciences |
Journal Citation | 11 (21), pp. 1-21 |
Article Number | 10034 |
Number of Pages | 21 |
Year | 2021 |
Publisher | MDPI AG |
Place of Publication | Switzerland |
ISSN | 2076-3417 |
Digital Object Identifier (DOI) | https://doi.org/10.3390/app112110034 |
Web Address (URL) | https://www.mdpi.com/2076-3417/11/21/10034 |
Abstract | Groundwater is one of the primary sources for the daily water requirements of the masses, but it is subjected to contamination due to the pollutants, such as nitrate, percolating through the soil with water. Especially in built-up areas, groundwater vulnerability and contamination are of major concern, and require appropriate consideration. The present study develops a novel framework for assessing groundwater nitrate contamination risk for the area along the Karakoram Highway, which is a part of the China Pakistan Economic Corridor (CPEC) route in northern Pakistan. A groundwater vulnerability map was prepared using the DRASTIC model. The nitrate concentration data from a previous study were used to formulate the nitrate contamination map. Three machine learning (ML) models, i.e., Support Vector Machine (SVM), Multivariate Discriminant Analysis (MDA), and Boosted Regression Trees (BRT), were used to analyze the probability of groundwater contamination incidence. Furthermore, groundwater contamination probability maps were obtained utilizing the ensemble modeling approach. The models were calibrated and validated through calibration trials, using the area under the receiver operating characteristic curve method (AUC), where a minimum AUC threshold value of 80% was achieved. Results indicated the accuracy of the models to be in the range of 0.82–0.87. The final groundwater contamination risk map highlights that 34% of the area is moderately vulnerable to groundwater contamination, and 13% of the area is exposed to high groundwater contamination risk. The findings of this study can facilitate decision-making regarding the location of future built-up areas properly in order to mitigate the nitrate contamination that can further reduce the associated health risks. |
Keywords | groundwater; machine learning; contamination risk mapping; policymaking; nitrate contamination |
ANZSRC Field of Research 2020 | 401104. Health and ecological risk assessment |
410504. Surface water quality processes and contaminated sediment assessment | |
461199. Machine learning not elsewhere classified | |
400411. Water treatment processes | |
Byline Affiliations | National University of Singapore |
Northern Arizona University, United States | |
COMSATS University Islamabad, Pakistan | |
University of Twente, Netherlands | |
School of Civil Engineering and Surveying | |
University of Greenwich, United Kingdom | |
Federation University | |
Institution of Origin | University of Southern Queensland |
https://research.usq.edu.au/item/q6vw5/assessing-nitrate-contamination-risks-in-groundwater-a-machine-learning-approach
Download files
172
total views69
total downloads2
views this month0
downloads this month
Export as
Related outputs
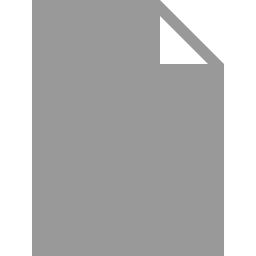