Smart and Automated Infrastructure Management: A Deep Learning Approach for Crack Detection in Bridge Images
Article
Article Title | Smart and Automated Infrastructure Management: A Deep Learning Approach for Crack Detection in Bridge Images |
---|---|
ERA Journal ID | 41498 |
Article Category | Article |
Authors | Inam, Hina, Islam, Naeem Ul, Akram, Muhammad Usman and Ullah, Fahim |
Editors | Wei, K. |
Journal Title | Sustainability |
Journal Citation | 15 (3) |
Article Number | 1866 |
Number of Pages | 38 |
Year | 2023 |
Publisher | MDPI AG |
Place of Publication | Switzerland |
ISSN | 2071-1050 |
Digital Object Identifier (DOI) | https://doi.org/10.3390/su15031866 |
Web Address (URL) | https://www.mdpi.com/2071-1050/15/3/1866 |
Abstract | Artificial Intelligence (AI) and allied disruptive technologies have revolutionized the scientific world. However, civil engineering, in general, and infrastructure management, in particular, are lagging behind the technology adoption curves. Crack identification and assessment are important indicators to assess and evaluate the structural health of critical city infrastructures such as bridges. Historically, such critical infrastructure has been monitored through manual visual inspection. This process is costly, time-consuming, and prone to errors as it relies on the inspector’s knowledge and the gadgets’ precision. To save time and cost, automatic crack and damage detection in bridges and similar infrastructure is required to ensure its efficacy and reliability. However, an automated and reliable system does not exist, particularly in developing countries, presenting a gap targeted in this study. Accordingly, we proposed a two-phased deep learning-based framework for smart infrastructure management to assess the conditions of bridges in developing countries. In the first part of the study, we detected cracks in bridges using the dataset from Pakistan and the online-accessible SDNET2018 dataset. You only look once version 5 (YOLOv5) has been used to locate and classify cracks in the dataset images. To determine the main indicators (precision, recall, and mAP (0.5)), we applied each of the YOLOv5 s, m, and l models to the dataset using a ratio of 7:2:1 for training, validation, and testing, respectively. The mAP (Mean average precision) values of all the models were compared to evaluate their performance. The results show mAP values for the test set of the YOLOv5 s, m, and l as 97.8%, 99.3%, and 99.1%, respectively, indicating the superior performance of the YOLOv5 m model compared to the two counterparts. In the second portion of the study, segmentation of the crack is carried out using the U-Net model to acquire their exact pixels. Using the segmentation mask allocated to the attribute extractor, the pixel’s width, height, and area are measured and visualized on scatter plots and Boxplots to segregate different cracks. Furthermore, the segmentation part validated the output of the proposed YOLOv5 models. This study not only located and classified the cracks based on their severity level, but also segmented the crack pixels and measured their width, height, and area per pixel under different lighting conditions. It is one of the few studies targeting low-cost health assessment and damage detection in bridges of developing countries that otherwise struggle with regular maintenance and rehabilitation of such critical infrastructure. The proposed model can be used by local infrastructure monitoring and rehabilitation authorities for regular condition and health assessment of the bridges and similar infrastructure to move towards a smarter and automated damage assessment system. |
Keywords | bridges; crack assessment; crack detection; damage assessment; deep learning; image processing; smart infrastructure management; YOLOv5 |
Article Publishing Charge (APC) Amount Paid | 2200.0 |
Article Publishing Charge (APC) Funding | Researcher |
Contains Sensitive Content | Does not contain sensitive content |
ANZSRC Field of Research 2020 | 330201. Automation and technology in building and construction |
330202. Building construction management and project planning | |
Byline Affiliations | National University of Sciences and Technology, Pakistan |
School of Surveying and Built Environment |
https://research.usq.edu.au/item/w27vx/smart-and-automated-infrastructure-management-a-deep-learning-approach-for-crack-detection-in-bridge-images
Download files
120
total views259
total downloads5
views this month5
downloads this month
Export as
Related outputs
Impact of responsible leadership on construction employees' pro-environmental behavior: Exploring the mediating role of key variables
Maqsoom, Ahsen, Prasittisopin, Lapyote and Ullah, Fahim. 2025. "Impact of responsible leadership on construction employees' pro-environmental behavior: Exploring the mediating role of key variables." Sustainable Futures. 9. https://doi.org/10.1016/j.sftr.2025.100668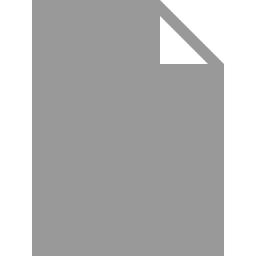