Civil infrastructure damage and corrosion detection: an application of machine learning
Article
Article Title | Civil infrastructure damage and corrosion detection: an application of machine learning |
---|---|
ERA Journal ID | 210216 |
Article Category | Article |
Authors | Munawar, Hafiz Suliman (Author), Ullah, Fahim (Author), Shahzad, Danish (Author), Heravi, Amirhossein (Author), Qayyum, Siddra (Author) and Akram, Junaid (Author) |
Journal Title | Buildings |
Journal Citation | 12 (2) |
Article Number | 156 |
Number of Pages | 27 |
Year | 2022 |
Publisher | MDPI AG |
Place of Publication | Switzerland |
ISSN | 2075-5309 |
Digital Object Identifier (DOI) | https://doi.org/10.3390/buildings12020156 |
Web Address (URL) | https://www.mdpi.com/2075-5309/12/2/156 |
Abstract | Automatic detection of corrosion and associated damages to civil infrastructures such as bridges, buildings, and roads, from aerial images captured by an Unmanned Aerial Vehicle (UAV), helps one to overcome the challenges and shortcomings (objectivity and reliability) associated with the manual inspection methods. Deep learning methods have been widely reported in the literature for civil infrastructure corrosion detection. Among them, convolutional neural networks (CNNs) display promising applicability for the automatic detection of image features less affected by image noises. Therefore, in the current study, we propose a modified version of deep hierarchical CNN architecture, based on 16 convolution layers and cycle generative adversarial network (CycleGAN), to predict pixel-wise segmentation in an end-to-end manner using the images of Bolte Bridge and sky rail areas in Victoria (Melbourne). The convolutedly designed model network proposed in the study is based on learning and aggregation of multi-scale and multilevel features while moving from the low convolutional layers to the high-level layers, thus reducing the consistency loss in images due to the inclusion of CycleGAN. The standard approaches only use the last convolutional layer, but our proposed architecture differs from these approaches and uses multiple layers. Moreover, we have used guided filtering and Conditional Random Fields (CRFs) methods to refine the prediction results. Additionally, the effectiveness of the proposed architecture was assessed using benchmarking data of 600 images of civil infrastructure. Overall, the results show that the deep hierarchical CNN architecture based on 16 convolution layers produced advanced performances when evaluated for different methods, including the baseline, PSPNet, DeepLab, and SegNet. Overall, the extended method displayed the Global Accuracy (GA); Class Average Accuracy (CAC); mean Intersection Of the Union (IOU); Precision (P); Recall (R); and F-score values of 0.989, 0.931, 0.878, 0.849, 0.818 and 0.833, respectively. |
Keywords | artificial intelligence; building corrosion detection; building damage detection; civil infrastructure crack detection; civil infrastructure inspection; image processing; machine learning; unmanned aerial vehicles |
ANZSRC Field of Research 2020 | 330202. Building construction management and project planning |
460306. Image processing | |
400508. Infrastructure engineering and asset management | |
330201. Automation and technology in building and construction | |
400504. Construction engineering | |
Byline Affiliations | University of New South Wales |
School of Surveying and Built Environment | |
Saarland University, Germany | |
University of Sydney | |
Institution of Origin | University of Southern Queensland |
https://research.usq.edu.au/item/q7106/civil-infrastructure-damage-and-corrosion-detection-an-application-of-machine-learning
Download files
171
total views79
total downloads1
views this month1
downloads this month
Export as
Related outputs
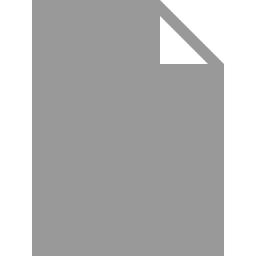