CNG-diesel engine performance and exhaust emission analysis with the aid of artificial neural network
Article
Article Title | CNG-diesel engine performance and exhaust emission analysis with the aid of artificial neural network |
---|---|
ERA Journal ID | 4005 |
Article Category | Article |
Authors | Yusaf, Talal F. (Author), Buttsworth, D. R. (Author), Saleh, Khalid H. (Author) and Yousif, B. F. (Author) |
Journal Title | Applied Energy |
Journal Citation | 87 (5), pp. 1661-1669 |
Number of Pages | 9 |
Year | 2010 |
Publisher | Elsevier |
Place of Publication | United Kingdom |
ISSN | 0306-2619 |
1872-9118 | |
Digital Object Identifier (DOI) | https://doi.org/10.1016/j.apenergy.2009.10.009 |
Web Address (URL) | https://www.sciencedirect.com/science/article/pii/S0306261909004371 |
Abstract | This study investigates the use of artificial neural network (ANN) modelling to predict brake power, torque, break specific fuel consumption (BSFC), and exhaust emissions of a diesel engine modified to operate with a combination of both compressed natural gas CNG and diesel fuels. A single cylinder, four-stroke diesel engine was modified for the present work and was operated at different engine loads and speeds. The experimental results reveal that the mixtures of CNG and diesel fuel provided better engine performance and improved the emission characteristics compared with the pure diesel fuel. For the ANN modelling, the standard back-propagation algorithm was found to be the optimum choice for training the model. A multi-layer perception network was used for non-linear mapping between the input and output parameters. It was found that the ANN model is able to predict the engine performance and exhaust emissions with a correlation coefficient of 0.9884, 0.9838, 0.95707, and 0.9934 for the engine torque, BSFC, NOx and exhaust temperature, respectively. |
Keywords | CNG fuel; ANN; engine performance; engine emission |
ANZSRC Field of Research 2020 | 400205. Hybrid and electric vehicles and powertrains |
400202. Automotive engineering materials | |
400201. Automotive combustion and fuel engineering | |
Public Notes | Files associated with this item cannot be displayed due to copyright restrictions. |
Byline Affiliations | Department of Mechanical and Mechatronic Engineering |
University of Oxford, United Kingdom | |
Department of Agricultural, Civil and Environmental Engineering | |
University of Nottingham, United Kingdom |
https://research.usq.edu.au/item/9z493/cng-diesel-engine-performance-and-exhaust-emission-analysis-with-the-aid-of-artificial-neural-network
2473
total views12
total downloads9
views this month0
downloads this month
Export as
Related outputs
Hayabusa2 Surface and Shock Layer Radiation from the Australian Airborne Observation
Ravichandran, Ranjith, Birch, Byrenn, Lock, Andrew, Buttsworth, David and Zander, Fabian. 2025. "Hayabusa2 Surface and Shock Layer Radiation from the Australian Airborne Observation." Journal of Spacecraft and Rockets. https://doi.org/10.2514/1.A36225Mechanical and Tribological Performance of Epoxy Composites Reinforced with YSZ Waste Ceramics for Sustainable Green Engineering Applications
Alsaeed, Talal, Alajmi, Ayedh Eid, Alotaibi, Jasem Ghanem, Ganthavee, Voravich and Yousif, Belal F.. 2024. "Mechanical and Tribological Performance of Epoxy Composites Reinforced with YSZ Waste Ceramics for Sustainable Green Engineering Applications." Processes. 12 (11). https://doi.org/10.3390/pr12112609On the incorporation of waste ceramic powder into concrete
Alotaibi, Jasem G., Alajmi, Ayedh Eid, Alsaeed, Talal, Khalaf, Jamal A. and Yousif, Belal F.. 2024. "On the incorporation of waste ceramic powder into concrete." Frontiers in Mechanical Engineering. 10. https://doi.org/10.3389/fmech.2024.1469727Advances in improving tribological performance of titanium alloys and titanium matrix composites for biomedical applications: a critical review
Abakay, Eray, Armağa, Mustafa, Avcu, Yasemin Yıldıran, Guney, Mert, Yousif, B. F. and Avcu, Egemen. 2024. "Advances in improving tribological performance of titanium alloys and titanium matrix composites for biomedical applications: a critical review." Frontiers in Materials. 11. https://doi.org/10.3389/fmats.2024.1452288Aerodynamic Measurements of Hypersonic Free-Flight via Optical Tracking and Bayesian State Estimation
Lock, Andrew, Hack, Flynn, Jahn, Ingo, Armstrong, Gerard, Birch, Byrenn and Buttsworth, David. 2024. "Aerodynamic Measurements of Hypersonic Free-Flight via Optical Tracking and Bayesian State Estimation." AIAA Journal: devoted to aerospace research and development. https://doi.org/10.2514/1.J064061On the Influence of Engine Compression Ratio on Diesel Engine Performance and Emission Fueled with Biodiesel Extracted from Waste Cooking Oil
Alotaibi, Jasem Ghanem, Alajmi, Ayedh Eid, Alsaeed, Talal, Al-Lwayzy, Saddam H. and Yousif, Belal F.. 2024. "On the Influence of Engine Compression Ratio on Diesel Engine Performance and Emission Fueled with Biodiesel Extracted from Waste Cooking Oil." Energies. 17 (15). https://doi.org/10.3390/en17153844Reconfiguring the TUSQ Hypersonic Facility for Lower Mach Number Testing
Swann, Phillip, Buttsworth, David, Jahn, Ingo H., Frith, Robert, Moran, Jeremy, Webb, Alister and Noller, Lachlan. 2024. "Reconfiguring the TUSQ Hypersonic Facility for Lower Mach Number Testing." AIAA Aviation Forum and ASCEND 2024. Las Vegas, United States 29 Jul - 02 Aug 2024 United States. https://doi.org/10.2514/6.2024-4198Depressurisation Restart Method for a Hypersonic Inlet with Closed-Plenum Mass Flow Metering
Noller, Lachlan J., Zander, Fabian and Buttsworth, David. 2024. "Depressurisation Restart Method for a Hypersonic Inlet with Closed-Plenum Mass Flow Metering." AIAA Aviation Forum and ASCEND 2024. Las Vegas, United States 29 Jul - 02 Aug 2024 United States. https://doi.org/10.2514/6.2024-4116Mach 6 and Mach 7 conditions in TUSQ at Reynolds numbers up to 30 million
Moran, Jeremy, Buttsworth, David R. and Noller, Lachlan. 2024. "Mach 6 and Mach 7 conditions in TUSQ at Reynolds numbers up to 30 million." AIAA Aviation Forum and ASCEND 2024. Las Vegas, United States 29 Jul - 02 Aug 2024 United States. https://doi.org/10.2514/6.2024-4197Correction: Algayyim et al. Sugarcane Biomass as a Source of Biofuel for Internal Combustion Engines (Ethanol and Acetone-Butanol-Ethanol): A Review of Economic Challenges. Energies 2022, 15, 8644
Algayyim, Sattar Jabbar Murad, Yusaf, Talal, Hamza, Naseer H., Wandel, Andrew P., Fattah, I. M. Rizwanul, Laimon, Mohamd and Rahman, S. M. Ashrafur. 2024. "Correction: Algayyim et al. Sugarcane Biomass as a Source of Biofuel for Internal Combustion Engines (Ethanol and Acetone-Butanol-Ethanol): A Review of Economic Challenges. Energies 2022, 15, 8644 ." Energies. 17 (14). https://doi.org/10.3390/en17143521Seismoacoustic measurements of the OSIRIS-REx re-entry with an off-grid Raspberry PiShake
Fernando, Benjamin, Charalambous, Constantinos, Saliby, Christelle, Sansom, Eleanor, Larmat, Carene, Buttsworth, David, Hicks, Daniel, Johnson, Roy, Lewis, Kevin, McCleary, Meaghan, Petricca, Giuseppe, Schmerr, Nick, Zander, Fabian and Inman, Jennifer. 2024. "Seismoacoustic measurements of the OSIRIS-REx re-entry with an off-grid Raspberry PiShake." Seismica. 3 (1). https://doi.org/10.26443/seismica.v3i1.1154Free-Flight of a Propelled Model in a High-Speed Ground-Test Facility
McQuellin, Liam P., Noller, Lachlan J. and Buttsworth, David R.. 2024. "Free-Flight of a Propelled Model in a High-Speed Ground-Test Facility." AIAA SciTech Forum 2024. Orlando, FL, United States 08 - 12 Jan 2024 United States. https://doi.org/10.2514/6.2024-2889An Overview of Hydrogen Production Techniques: Challenges and Limiting Factors in Achieving Wide-scale Productivity
Ahmed, Idrees Hammoody, Alakoul, Karrar A., Al-Manea, Ahmed, Wetaify, Ahmed Rajih Hassan, Saleh, Khalid, Al-Rbaihat, Raed and Alahmer, Ali. 2024. "An Overview of Hydrogen Production Techniques: Challenges and Limiting Factors in Achieving Wide-scale Productivity." 3rd International Conference on Engineering and Science. Al-Samawa, Iraq 03 - 04 May 2024 United States. AIP Publishing. https://doi.org/10.1063/5.0191762Theoretical study of the effect of orientations and fibre volume on the thermal insulation capability of reinforced polymer composites
Shalwan, Abdullah, Alajmi, Abdalrahman and Yousif, Belal. 2024. "Theoretical study of the effect of orientations and fibre volume on the thermal insulation capability of reinforced polymer composites." Reviews on Advanced Materials Science. 63 (1). https://doi.org/10.1515/rams-2023-0190Simulation of an Ammonia-Carbon Dioxide Transcritical Cascade Refrigeration System
Hussen, Hasanen M., Rahman, Mokdad Hayawi, Majdi, Hasan Shakir and Saleh, Khalid. 2024. "Simulation of an Ammonia-Carbon Dioxide Transcritical Cascade Refrigeration System." Mathematical Modelling of Engineering Problems. 11 (4), pp. 943-952. https://doi.org/10.18280/mmep.110411Investigation of natural convection and entropy generation of non-Newtonian flow in molten polymer-filled odd-shaped cavities using finite difference lattice Boltzmann method
Ahmed, Saba Y., Al-Amir, Qusay Rasheed, Hamzah, Hameed K., Ali, Farooq H., Abed, Azher M. Abed, Al-Manea, Ahmed, Egab, Karim, Al-Rbaihat, Raed, Saleh, Khalid and Alahmer, Ali. 2024. "Investigation of natural convection and entropy generation of non-Newtonian flow in molten polymer-filled odd-shaped cavities using finite difference lattice Boltzmann method." Numerical Heat Transfer Part B: Fundamentals. https://doi.org/10.1080/10407790.2024.2349709Finite element analysis and experimental validation of polymer–metal contacts in block-on-ring configuration
Awwad, K. Y. Eayal, Fallahnezhad, Khosro, Yousif, B. F., Mostafa, Ahmad, Alajarmeh, Omar, Shalwan, A. and Zeng, Xuesen. 2024. "Finite element analysis and experimental validation of polymer–metal contacts in block-on-ring configuration." Friction. 12 (3), pp. 554-568. https://doi.org/10.1007/s40544-023-0795-xRevolutionizing procurement: Unveiling next-gen supplier strategies in UAE's oil & gas sector
Alhammadi, Ali, Yusaf, Talal, Soar, Jeffrey, Ali, Bashar Mahmood, Kadirgama, Kumaran and Yousif, Belal F. 2024. "Revolutionizing procurement: Unveiling next-gen supplier strategies in UAE's oil & gas sector." The Extractive Industries and Society. 17. https://doi.org/10.1016/j.exis.2024.101428Determination of deep bed drying rates for abattoir paunch waste using revised co-efficients for the Hukill equation
Spence, Jenny, Buttsworth, David, Carter, Brad and Amar, Abdurazaq. 2024. "Determination of deep bed drying rates for abattoir paunch waste using revised co-efficients for the Hukill equation." Thermal Science and Engineering Progress. 49, p. 102461. https://doi.org/10.1016/j.tsep.2024.102461Plenum-Based Mass Flow Metering
Noller, Lachlan, Zander, Fabian and Buttsworth, David. 2024. "Plenum-Based Mass Flow Metering." AIAA Journal: devoted to aerospace research and development. 62 (1), pp. 399-404. https://doi.org/10.2514/1.J063279Improved Surface Temperature Identification Method for Remote Observations of Sample Return Capsules
Birch, Byrenn, Buttsworth, David and Zander, Fabian. 2024. "Improved Surface Temperature Identification Method for Remote Observations of Sample Return Capsules." Journal of Spacecraft and Rockets. 61 (2). https://doi.org/10.2514/1.A35692Noncontact Estimation of the Onset of Ice Accretion in Turbofan Stators
Saleh, Khalid, Leis, John and Buttsworth, David. 2024. "Noncontact Estimation of the Onset of Ice Accretion in Turbofan Stators." IET Science, Measurement and Technology. 18 (6), pp. 271-279. https://doi.org/10.1049/smt2.12191Influence of Natural Gas and Hydrogen Properties on Internal Combustion Engine Performance, Combustion, and Emissions: A Review
Algayyim, Sattar Jabbar Murad, Saleh, Khalid, Wandel, Andrew P, Fattah, Islam Md Rizwanul, Yusaf, Talal and Alrazen, Hayder A.. 2024. "Influence of Natural Gas and Hydrogen Properties on Internal Combustion Engine Performance, Combustion, and Emissions: A Review." Fuel: the science and technology of fuel and energy. 632. https://doi.org/10.1016/j.fuel.2023.130844Trajectory Analysis of the Hayabusa2 Capsule from a Single Airborne Observation
Zander, Fabian, Buttsworth, David R., Birch, Byrenn and Payne, Allan. 2024. "Trajectory Analysis of the Hayabusa2 Capsule from a Single Airborne Observation." Journal of Spacecraft and Rockets. 61 (1). https://doi.org/10.2514/1.A35719Optical aerodynamic measurements of hypersonic free-flight using Bayesian state estimation
Lock, Andrew, Armstrong, Gerard, Hack, Flynn, Birch, Byrenn, Buttsworth, David and Jahn, Ingo. 2023. "Optical aerodynamic measurements of hypersonic free-flight using Bayesian state estimation." AIAA Aviation 2023 Forum . San Diego, United States 12 202 - 16 Jun 2023 United States. American Institute of Aeronautics and Astronautics. https://doi.org/10.2514/6.2023-3713Design of DC solar water pump for farmers in the Kurdistan region of Iraq
Mahmood, Raid A., Musa, Veyan A., Kassim, Muna S., Saleh, Khalid, Mahmood, Sajid A., Mahmood, Qaid A., Hassan, Amer M., Al-Manea, Ahmed R. and Abdulkareem, Lokman A.. 2023. "Design of DC solar water pump for farmers in the Kurdistan region of Iraq." 2nd International Conference on Engineering and Advanced Technology (ICEAT2022) . Istanbul, Turkey 28 - 29 Mar 2022 United States. AIP Publishing. https://doi.org/10.1063/5.0149197Simulation investigation of the oscillatory motion of two elliptic obstacles located within a quarter-circle cavity filled with Cu-Al2O3/water hybrid nanofluid
Al-Srayyih, Basil Mahdi, AlManea, Ahmed, Saleh, Khalid, Abed, Azher M., Al-Amir, Qusay Rasheed, Hamzah, Hameed K., Ali, Farooq H., Al-Rbaihat, Raed and Alahmer, Ali. 2023. "Simulation investigation of the oscillatory motion of two elliptic obstacles located within a quarter-circle cavity filled with Cu-Al2O3/water hybrid nanofluid." Numerical Heat Transfer Part A: Applications. https://doi.org/10.1080/10407782.2023.2279248Investigation on Three-Body Abrasion Resistance of Mild Steel Soil Slurry Condition-Simulating Agricultural Condition
Alsaeed, Talal, Alajmi, Ayedh Eid, Alotaibi, Jasem Ghanem and Yousif, Belal F.. 2023. "Investigation on Three-Body Abrasion Resistance of Mild Steel Soil Slurry Condition-Simulating Agricultural Condition." Advances in Materials Science and Engineering. 2023. https://doi.org/10.1155/2023/5616909Redefining procurement paradigms: A critical review of buyer-supplier dynamics in the global petroleum and natural gas industry
Alhammadi, Ali, Soar, Jeffrey, Yusaf, Talal, Ali, Bashar Mahmood and Kadirgama, Kumaran. 2023. "Redefining procurement paradigms: A critical review of buyer-supplier dynamics in the global petroleum and natural gas industry." The Extractive Industries and Society. 16. https://doi.org/10.1016/j.exis.2023.101351Investigating the lap shear adhesion of coir and glass-fibre reinforced epoxy bonding to mild steel with varying volume fractions
Alajmi, Ayedh Eid, Alsaeed, Talal, Alotaibi, Jasem Ghanem, Srelam, Jayanth Kumar and Yousif, Belal F.. 2023. "Investigating the lap shear adhesion of coir and glass-fibre reinforced epoxy bonding to mild steel with varying volume fractions." Frontiers in Mechanical Engineering. 9. https://doi.org/10.3389/fmech.2023.1232643Abrasive Wear Performance of Coir Fiber Reinforced Polymer Composite
Shalwana, A., Mallampati, S. and Yousif, Belal. 2023. "Abrasive Wear Performance of Coir Fiber Reinforced Polymer Composite." Tribology in Industry. 45 (3), pp. 532-541. https://doi.org/10.24874/ti.1500.06.23.07Performance evaluation of supersonic flow for variable geometry radial ejector through CFD models based on DES-turbulence models, GPR machine learning, and MPA optimization
Al-Rbaihat, Raed, Saleh, Khalid, Malpress, Ray, Buttsworth, David, Alahmer, Hussein and Alahmer, Ali. 2023. "Performance evaluation of supersonic flow for variable geometry radial ejector through CFD models based on DES-turbulence models, GPR machine learning, and MPA optimization." International Journal of Thermofluids. 20. https://doi.org/10.1016/j.ijft.2023.100487Experimental investigation of a novel variable geometry radial ejector
Al-Rbaihat, Raed, Saleh, Khalid, Malpress, Ray and Buttsworth, David. 2023. "Experimental investigation of a novel variable geometry radial ejector." Applied Thermal Engineering. 233. https://doi.org/10.1016/j.applthermaleng.2023.121143Tribological and mechanical performances of newly developed eco-epoxy composites incorporating flax fibres and graphene nanoplatelets
Awwad, KY Eayal, Yousif, BF, Mostafa, Ahmad, Alajarmeh, Omar and Zeng, Xuesen. 2023. "Tribological and mechanical performances of newly developed eco-epoxy composites incorporating flax fibres and graphene nanoplatelets." Journal of Reinforced Plastics and Composites. 42 (17-18), p. 939–952. https://doi.org/10.1177/07316844221143451A 2022 τ-Herculid meteor cluster from an airborne experiment: automated detection, characterization, and consequences for meteoroids
Vaubaillon, J., Loir, C., Ciocan, C., Kandeepan, M., Millet, M., Cassagne, A., Lacassagne, L., Da Fonseca, P., Zander, F., Buttsworth, D., Loehle, S., Toth, J., Gray, S., Moingeon, A. and Rambaux, N.. 2023. "A 2022 τ-Herculid meteor cluster from an airborne experiment: automated detection, characterization, and consequences for meteoroids ." Astronomy and Astrophysics: a European journal. 670. https://doi.org/10.1051/0004-6361/202244993Sugarcane Biomass as a Source of Biofuel for Internal Combustion Engines (Ethanol and Acetone-Butanol-Ethanol): A Review of Economic Challenges
Algayyim, Sattar Jabbar Murad, Yusaf, Talal, Hamza, Naseer H., Wandel, Andrew P., Fattah, I. M. Rizwanul, Laimon, Mohamd and Rahman, S. M. Ashrafur. 2022. "Sugarcane Biomass as a Source of Biofuel for Internal Combustion Engines (Ethanol and Acetone-Butanol-Ethanol): A Review of Economic Challenges." Energies. 15 (22), pp. 1-17. https://doi.org/10.3390/en15228644CFD Simulations and Experimental Observation for Air-Water Two-phase Flow in a Vertical Pipe
Mahmood, Raid A., Saleh, Khalid, Musa, Veyan A., Massoud, Enass, Al-Lwayzy, Saddam H., Ibrahim, Ahmed Khalid, Mohammed, Ali Ghazi and Abdulkareem, Lokman A.. 2022. "CFD Simulations and Experimental Observation for Air-Water Two-phase Flow in a Vertical Pipe." Gongcheng Kexue yu Jishu. 54 (6), pp. 2363-2375.Experimental Study on the Efficiency Improvement of Flat Plate Solar Collectors Using Hybrid Nanofluids Graphene/Waste Cotton
Mahamude, Abu Shadate Faisal, Harun, Wan Sharuzi Wan, Kadirgama, Kumaran, Ramasamy, Devarajan, Farhana, Kaniz, Saleh, Khalid and Yusaf, Talal. 2022. "Experimental Study on the Efficiency Improvement of Flat Plate Solar Collectors Using Hybrid Nanofluids Graphene/Waste Cotton." Energies. 15 (7), pp. 1-27. https://doi.org/10.3390/en15072309Laser-Induced Diaphragm Rupture for Improved Sequencing and Repeatability in a Hypersonic Facility
Birch, Byrenn, Buttsworth, David and Zander, Fabian. 2023. "Laser-Induced Diaphragm Rupture for Improved Sequencing and Repeatability in a Hypersonic Facility." Journal of Spacecraft and Rockets. 60 (1), pp. 351-360. https://doi.org/10.2514/1.A35427Hayabusa2 Capsule Reentry: Visible and Near-IR Emission Spectroscopy from the Australian Airborne Observation
Birch, Byrenn, Zander, Fabian, Buttsworth, David, Noller, Lachlan and Payne, Allan. 2022. "Hayabusa2 Capsule Reentry: Visible and Near-IR Emission Spectroscopy from the Australian Airborne Observation." AIAA Aviation Forum 2022. Chicago, United States 27 Jun - 01 Jul 2022 United States. https://doi.org/10.2514/6.2022-3736Planning and Implementation of the Australian Airborne Observation of Hayabusa2
Zander, Fabian, Buttsworth, David R., Birch, Byrenn J. C. and Payne, Allan. 2022. "Planning and Implementation of the Australian Airborne Observation of Hayabusa2." AIAA Aviation Forum 2022. Chicago, United States 27 Jun - 01 Jul 2022 United States. https://doi.org/10.2514/6.2022-3583Machinability of Polymeric Composites and Future Reinforcements — A Review
Shalwan, Abdullah, Alsaeed, Talal and Yousif, Belal. 2022. "Machinability of Polymeric Composites and Future Reinforcements — A Review." Journal of Materials Science and Chemical Engineering. 10 (5), pp. 40-72. https://doi.org/10.4236/msce.2022.105004System dynamics modelling to assess the impact of renewable energy systems and energy efficiency on the performance of the energy sector
Laimon, Mohamd, Mai, Thanh, Goh, Steven and Yusaf, Talal. 2022. "System dynamics modelling to assess the impact of renewable energy systems and energy efficiency on the performance of the energy sector." Renewable Energy. 193, pp. 1041-1048. https://doi.org/10.1016/j.renene.2022.05.041A systems thinking approach to address sustainability challenges to the energy sector
Laimon, M., Yusaf, Talal, Mai, T., Goh, S. and Alrefae, Waleed. 2022. "A systems thinking approach to address sustainability challenges to the energy sector." International Journal of Thermofluids. 15, pp. 1-13. https://doi.org/10.1016/j.ijft.2022.100161Measurement of turbulent supersonic steam jet flow characteristics using TDLAS
Al-Manea, Ahmed, Buttsworth, D., Saleh, K., Malpress, R. and Leis, John. 2022. "Measurement of turbulent supersonic steam jet flow characteristics using TDLAS." Flow Measurement and Instrumentation. 87, pp. 1-10. https://doi.org/10.1016/j.flowmeasinst.2022.102212System Design and Preliminary Analysis of the UQ Near Infrared Spectroscopy Data of the Hayabusa2 Re-entry
James, Christopher M., Thompson, Matthew, Apirana, Steve F., Zander, Fabian, Buttsworth, David R. and Payne, Allan. 2022. "System Design and Preliminary Analysis of the UQ Near Infrared Spectroscopy Data of the Hayabusa2 Re-entry." AIAA SciTech Forum 2022. San Diego, United States 03 - 07 Jan 2022 United States. https://doi.org/10.2514/6.2022-2153Hayabusa2 Capsule Reentry: Australian Airborne Observation Emission Spectroscopy Calibration and Preliminary Analysis
Birch, Byrenn, Zander, Fabian, Buttsworth, David, Noller, Lachlan and Payne, Allan. 2022. "Hayabusa2 Capsule Reentry: Australian Airborne Observation Emission Spectroscopy Calibration and Preliminary Analysis." AIAA SciTech Forum 2022. San Diego, United States 03 - 07 Jan 2022 United States. https://doi.org/10.2514/6.2022-2151Theoretical Study of the Effect of Fibre Porosity on the Heat Conductivity of Reinforced Gypsum Composite Material
Shalwan, A., Alajmi, Abdalrahman and Yousif, B. F.. 2022. "Theoretical Study of the Effect of Fibre Porosity on the Heat Conductivity of Reinforced Gypsum Composite Material." Polymers. 14 (19), pp. 1-23. https://doi.org/10.3390/polym14193973Effects of exhaust gas recirculation (EGR) on a diesel engine fuelled with palm-biodiesel
Yasin, Mohd Hafizil Mat, Mamat, Rizalman, Yusop, Ahmad Fitri, Paruka, Perowansa, Yusaf, Talal and Najafi, Gholamhassan. 2015. "Effects of exhaust gas recirculation (EGR) on a diesel engine fuelled with palm-biodiesel." Energy Procedia. 75, pp. 30-36. https://doi.org/10.1016/j.egypro.2015.07.131Development of micro-scale biomass-fuelled CHP system using stirling engine
Arashnia, Ilva, Najafi, Gholamhassan, Ghobadian, Barat, Yusaf, Talal, Mamat, Rizalman and Kettner, Maurice. 2015. "Development of micro-scale biomass-fuelled CHP system using stirling engine." Energy Procedia. 75, pp. 1108-1113. https://doi.org/10.1016/j.egypro.2015.07.505Hypersonic Pitching Control Model Development
Stern, Nathan, Buttsworth, David, Choudhury, Rishabh and Birch, Byrenn. 2018. "Hypersonic Pitching Control Model Development." 22nd AIAA International Space Planes and Hypersonics Systems and Technologies Conference (AIAA 2018). Orlando, United States 17 - 19 Sep 2018 Reston, United States. https://doi.org/10.2514/6.2018-5267Pressure Fluctuations in a Hypersonic Ludwieg Tube with Free Piston Compression Heating
Birch, Byrenn J. C., Choudhury, Rishabh, Stern, Nathan and Buttsworth, David R.. 2018. "Pressure Fluctuations in a Hypersonic Ludwieg Tube with Free Piston Compression Heating." 22nd AIAA International Space Planes and Hypersonics Systems and Technologies Conference (AIAA 2018). Orlando, United States 17 - 19 Sep 2018 Reston, United States. https://doi.org/10.2514/6.2018-5266Testing of Ultra Fast Response, Durable Co-axial Thermocouples for High Enthalpy Impulse Facilities
James, Christopher M., Birch, Byrenn J. C., Smith, Daniel R., Cullen, Timothy G., Millard, Theodore, Vella, Samuel, Liu, Yu, Morgan, Richard G., Stern, Nathan and Buttsworth, David R.. 2019. "Testing of Ultra Fast Response, Durable Co-axial Thermocouples for High Enthalpy Impulse Facilities." AIAA Aviation Forum 2019. Dallas, United States 17 - 21 Jun 2019 United States. https://doi.org/10.2514/6.2019-3007Optimization of combustion in micro combined heat and power (mCHP) system with the biomass-Stirling engine using SiO2 and Al2O3 nanofluids
Najafi, G., Hoseini, S. S., De Goey, L. P. H. and Yusaf, Talal. 2020. "Optimization of combustion in micro combined heat and power (mCHP) system with the biomass-Stirling engine using SiO2 and Al2O3 nanofluids." Applied Thermal Engineering. 169 (114936), pp. 1-9. https://doi.org/10.1016/j.applthermaleng.2020.114936Analysis of Particulate Matter (PM) Emissions in Diesel Engines Using Palm Oil Biodiesel Blended with Diesel Fuel
Yusop, Ahmad Fitri, Mamat, Rizalman, Yusaf, Talal, Najafi, Gholamhassan, Yasin, Mohd Hafizil Mat and Khathri, Akasyah Mohd. 2018. "Analysis of Particulate Matter (PM) Emissions in Diesel Engines Using Palm Oil Biodiesel Blended with Diesel Fuel." Energies. 11 (5), pp. 1-25. https://doi.org/10.3390/en11051039Alcohol and ether as alternative fuels in spark ignition engine: A review
Awad, Omar I., Mamat, R., Ali, Obed M., Sidik, N. A. C., Yusaf, T., Kadirgama, K. and Kettner, Maurice. 2018. "Alcohol and ether as alternative fuels in spark ignition engine: A review." Renewable and Sustainable Energy Reviews. 82 (Part 3), pp. 2586-2605. https://doi.org/10.1016/j.rser.2017.09.074Ailanthus altissima (tree of heaven) seed oil: Characterisation and optimisation of ultrasonication-assisted biodiesel production
Hoseini, S. S., Najafi, G., Ghobadian, B., Mamat, R., Ebadi, M. T. and Yusaf, Talal. 2018. "Ailanthus altissima (tree of heaven) seed oil: Characterisation and optimisation of ultrasonication-assisted biodiesel production." Fuel: the science and technology of fuel and energy. 220, pp. 621-630. https://doi.org/10.1016/j.fuel.2018.01.094Economic and Environmental Impact of Energy Saving in Healthcare Buildings
Garcia-Sanz-Calcedo, Justo, Al-Kassir, Awf and Yusaf, Talal. 2018. "Economic and Environmental Impact of Energy Saving in Healthcare Buildings." Applied Sciences. 8 (3), pp. 1-13. https://doi.org/10.3390/app8030440The effect of inlet temperature and spark timing on thermo-mechanical, chemical and the total exergy of an SI engine using bioethanol-gasoline blends
Kiani, Mostafa Kiani Deh, Rostami, Sajad, Eslami, Maryam, Yusaf, Talal and Sendilvelan, Subramanian. 2018. "The effect of inlet temperature and spark timing on thermo-mechanical, chemical and the total exergy of an SI engine using bioethanol-gasoline blends." Energy Conversion and Management. 165, pp. 344-353. https://doi.org/10.1016/j.enconman.2018.03.066An Experimental Technology of Drying and Clean Combustion of Biomass Residues
Al-Kassir, Awf, Coelho, Pedro, Garcia-Sanz-Calcedo, Justo, Moral, Francisco J., Al-Karany, Raul Kassir and Yusaf, Talal. 2018. "An Experimental Technology of Drying and Clean Combustion of Biomass Residues." Applied Sciences. 8 (6), pp. 1-10. https://doi.org/10.3390/app8060905The Effects of Camelina 'Soheil' as a Novel Biodiesel Fuel on the Performance and Emission Characteristics of Diesel Engine
Hoseini, Seyed Salar, Najafi, Gholamhassam, Ghobadian, Barat, Yusaf, Talal and Ebadi, Mohammad Taghi. 2018. "The Effects of Camelina 'Soheil' as a Novel Biodiesel Fuel on the Performance and Emission Characteristics of Diesel Engine." Applied Sciences. 8 (6), pp. 1-20. https://doi.org/10.3390/app8061010Experimental and numerical analysis of flow and heat transfer characteristics of EGR cooler in diesel engine
Hoseini, S. S., Najafi, G., Ghobadian, Barat and Yusaf, Talal. 2018. "Experimental and numerical analysis of flow and heat transfer characteristics of EGR cooler in diesel engine." Applied Thermal Engineering. 140, pp. 745-758. https://doi.org/10.1016/j.applthermaleng.2018.05.058An overview of marine macroalgae as bioresource
Sudhakar, K., Mamat, R., Samykano, M., Azmi, W. H., Ishak, W. F. W. and Yusaf, Talal. 2018. "An overview of marine macroalgae as bioresource." Renewable and Sustainable Energy Reviews. 91, pp. 165-179. https://doi.org/10.1016/j.rser.2018.03.100Novel environmentally friendly fuel: The effects of nanographene oxide additives on the performance and emission characteristics of diesel engines fuelled with Ailanthus altissima biodiesel
Hoseini, S. S., Najafi, G., Ghobadian, B., Mamat, R., Ebadi, M. T. and Yusaf, Talal. 2018. "Novel environmentally friendly fuel: The effects of nanographene oxide additives on the performance and emission characteristics of diesel engines fuelled with Ailanthus altissima biodiesel." Renewable Energy. 125, pp. 283-294. https://doi.org/10.1016/j.renene.2018.02.104Characterization of biodiesel production (ultrasonic-assisted) from evening-primroses (Oenothera lamarckiana) as novel feedstock and its effect on CI engine parameters
Hoseini, S. S., Najafi, G., Ghobadian, B., Mamat, R., Ebadi, M. T. and Yusaf, Talal. 2019. "Characterization of biodiesel production (ultrasonic-assisted) from evening-primroses (Oenothera lamarckiana) as novel feedstock and its effect on CI engine parameters." Renewable Energy. 130, pp. 50-60. https://doi.org/10.1016/j.renene.2018.06.042The Impacts of Water Pricing and Non-Pricing Policies on Sustainable Water Resources Management: A Case of Ghorveh Plain at Kurdistan Province, Iran
Asaadi, Mohammad Ali, Mortazavi, Seyed Abolghasem, Zamani, Omid, Najafi, Gholam Hassan, Yusaf, Talal and Hoseini, Seyed Salar. 2019. "The Impacts of Water Pricing and Non-Pricing Policies on Sustainable Water Resources Management: A Case of Ghorveh Plain at Kurdistan Province, Iran." Energies. 12 (14), pp. 1-16. https://doi.org/10.3390/en12142667Optimization of an Ultrasonic-Assisted Biodiesel Production Process from One Genotype of Rapeseed (TERI (OE) R-983) as a Novel Feedstock Using Response Surface Methodology
Almasi, Sara, Ghobadian, Barat, Najafi, Gholam Hassan, Yusaf, Talal, Soufi, Masoud Dehghani and Hoseini, Seyed Salar. 2019. "Optimization of an Ultrasonic-Assisted Biodiesel Production Process from One Genotype of Rapeseed (TERI (OE) R-983) as a Novel Feedstock Using Response Surface Methodology." Energies. 12 (14), pp. 1-14. https://doi.org/10.3390/en12142656Performance Investigation of Solar ORC Using Different Nanofluids
Loni, Reyhaneh, Najafi, Gholamhassan, Asli-Ardeh, Ezzatollah Askari, Ghobadian, Barat, Le Roux, Willem G. and Yusaf, Talal. 2019. "Performance Investigation of Solar ORC Using Different Nanofluids." Applied Sciences. 9 (15), pp. 1-23. https://doi.org/10.3390/app9153048Performance and emission characteristics of a CI engine using nano particles additives in biodiesel-diesel blends and modeling with GP approach
Ghanbari, M., Najafi, G., Ghobadian, B., Yusaf, T., Carlucci, A. P. and Kiani Deh Kiani, M.. 2017. "Performance and emission characteristics of a CI engine using nano particles additives in biodiesel-diesel blends and modeling with GP approach." Fuel: the science and technology of fuel and energy. 202, pp. 699-716. https://doi.org/10.1016/j.fuel.2017.04.117Performance Assessment of a Solar Dryer System Using Small Parabolic Dish and Alumina/Oil Nanofluid: Simulation and Experimental Study
Arkian, Amir Hossein, Najafi, Gholamhassan, Gorjian, Shiva, Loni, Reyhaneh, Bellos, Evangelos and Yusaf, Talal. 2019. "Performance Assessment of a Solar Dryer System Using Small Parabolic Dish and Alumina/Oil Nanofluid: Simulation and Experimental Study." Energies. 12 (24), pp. 1-22. https://doi.org/10.3390/en12244747Effects of biodiesel fuel obtained from Salvia macrosiphon oil (ultrasonic-assisted) on performance and emissions of diesel engine
Hoseini, S. S., Najaf, G., Ghobadian, B., Rahimi, A., Yusaf, Talal, Mamat, Rizalman, Sidik, N. A. C. and Azmi, W. H.. 2017. "Effects of biodiesel fuel obtained from Salvia macrosiphon oil (ultrasonic-assisted) on performance and emissions of diesel engine." Energy. 131, pp. 289-296. https://doi.org/10.1016/j.energy.2017.04.150Effects of physicochemical properties of biodiesel fuel blends with alcohol on diesel engine performance and exhaust emissions: A review
Zaharin, M. S. M, Abdullah, N. R., Najafi, G., Sharudin, H. and Yusaf, T.. 2017. "Effects of physicochemical properties of biodiesel fuel blends with alcohol on diesel engine performance and exhaust emissions: A review." Renewable and Sustainable Energy Reviews. 79, pp. 475-493. https://doi.org/10.1016/j.rser.2017.05.035Analysis of the Average Annual Consumption of Water in the Hospitals of Extremadura (Spain)
Garcia-Sanz-Calcedo, Justo, Lopez-Rodriguez, Fernando, Yusaf, Talal and Al-Kassir, Awf. 2017. "Analysis of the Average Annual Consumption of Water in the Hospitals of Extremadura (Spain)." Energies. 10 (4), pp. 1-10. https://doi.org/10.3390/en10040479Dependence of the Microporosity of Activated Carbons on the Lignocellulosic Composition of the Precursors
Roman, Silvia, Ledesma, Beatriz, Alvarez-Murillo, Andres, Al-Kassir, Awf and Yusaf, Talal. 2017. "Dependence of the Microporosity of Activated Carbons on the Lignocellulosic Composition of the Precursors." Energies. 10 (4), pp. 1-11. https://doi.org/10.3390/en10040542Treatment of Slaughterhouse Waste Water Mixed with Serum from Lacteal Industry of Extremadura in Spain to Produce Clean Energy
Marcos, A. C., Al-Kassir, A., Cuadros, Francisco and Yusaf, Talal. 2017. "Treatment of Slaughterhouse Waste Water Mixed with Serum from Lacteal Industry of Extremadura in Spain to Produce Clean Energy." Energies. 10 (6), pp. 1-15. https://doi.org/10.3390/en10060765Focused Laser Differential Interferometry for Hypersonic Flow Diagnostics
Birch, Byrenn, Buttsworth, David and Zander, Fabian. 2019. "Focused Laser Differential Interferometry for Hypersonic Flow Diagnostics." Alwahabi, Z. T. and Medwell, P. R. (ed.) 9th Australian Conference on Laser Diagnostics (IX ACLD). Adelaide, Australia 02 - 04 Dec 2019 Adelaide, Australia.Response surface methodology (RSM) based multi-objective optimization of fusel oil-gasoline blends at different water content in SI engine
Awad, Omar I., Mamat, R., Ali, Obed M., Azmi, W. H., Kadirgama, K., Yusri, I. M., Leman, A. M. and Yusaf, T.. 2017. "Response surface methodology (RSM) based multi-objective optimization of fusel oil-gasoline blends at different water content in SI engine." Energy Conversion and Management. 150, pp. 222-241. https://doi.org/10.1016/j.enconman.2017.07.047Energy content, bulk density, and the latent heat of vaporisation characteristics of abattoir paunch waste
Spence, Jennifer, Buttsworth, David and Carter, Brad. 2022. "Energy content, bulk density, and the latent heat of vaporisation characteristics of abattoir paunch waste." Energy. 248, pp. 1-6. https://doi.org/10.1016/j.energy.2022.123645Analysis of Reentry and Break-Up Forces from Impulse Facility Experiments and Numerical Rebuilding
Leiser, David, Loehle, Stefan, Zander, Fabian, Buttsworth, David R., Choudhury, Rishabh and Fasoulas, Stefanos. 2022. "Analysis of Reentry and Break-Up Forces from Impulse Facility Experiments and Numerical Rebuilding." Journal of Spacecraft and Rockets. 59 (4), pp. 1276-1288. https://doi.org/10.2514/1.A35204Supersonic Steam Ejectors: Comparison of Dry and Wet-steam CFD Simulation Models
Al-Manea, Ahmed and Saleh, Khalid. 2022. "Supersonic Steam Ejectors: Comparison of Dry and Wet-steam CFD Simulation Models." Journal of Engineering Science and Technology. 17 (2), pp. 1200-1212.A Comprehensive Review on Efficiency Enhancement of Solar Collectors Using Hybrid Nanofluids
Mahamude, Abu Shadate Faisal, Kamarulzaman, Muhamad Kamal, Harun, Wan Sharuzi Wan, Kadirgama, Kumaran, Ramasamy, Devarajan, Farhana, Kaniz, Bakar, Rosli Abu, Yusaf, Talal, Subramanion, Sivarao and Yousif, Belal. 2022. "A Comprehensive Review on Efficiency Enhancement of Solar Collectors Using Hybrid Nanofluids." Energies. 15 (4), pp. 1-25. https://doi.org/10.3390/en15041391Ice Crystal Icing Wind Tunnel: Characterisation and Experimental Results
Saleh, Khalid, Leis, John and Buttsworth, David. 2021. "Ice Crystal Icing Wind Tunnel: Characterisation and Experimental Results." 19th Australian International Aerospace Congress. Melbourne, Australia 29 Nov - 02 Dec 2021 Australia.Hydrogen Energy Demand Growth Prediction and Assessment (2021–2050) Using a System Thinking and System Dynamics Approach
Yusaf, Talal, Laimon, Mohamd, Alrefae, Waleed, Kadirgama, Kumaran, Al Dhahad, Hayder, Ramasamy, Devarajan, Kamarulzaman, Mohd Kamal and Yousif, Belal. 2022. "Hydrogen Energy Demand Growth Prediction and Assessment (2021–2050) Using a System Thinking and System Dynamics Approach." Applied Sciences. 12 (2), pp. 1-16. https://doi.org/10.3390/app12020781Two-Phase Flow Development of R134a in a Horizontal Pipe: Computational Investigation
Mahmood, Raid A., Saleh, Khalid, Musa, Veyan A., Massoud, Enass, Sharifian-Barforoush, Ahmad and Abdulkareem, Lokman A.. 2021. "Two-Phase Flow Development of R134a in a Horizontal Pipe: Computational Investigation." International Journal of Heat and Technology (Calore e Tecnologia). 39 (5), pp. 1532-1540. https://doi.org/10.18280/ijht.390515An Incident Shock Boundary Condition for Eilmer4
Hack, Flynn, Jahn, Ingo, Buttsworth, David and Zander, Fabian. 2022. "An Incident Shock Boundary Condition for Eilmer4." 23rd Australasian Fluid Mechanics Conference (AFMC2022). Sydney, Australia 04 - 08 Dec 2022 Australia. University of Sydney.Experimental analysis on the performance, combustion/emission characteristics of a DI diesel engine using hydrogen in dual fuel mode
Bakar, R.A., Widudo, Kadirgama, K., Ramasamy, D., Yusaf, Talal, Kamarulzaman, M.K., Sivaraos, Aslfattahi, Navid, Samylingam, L. and Alwayzy, Sadam H.. 2022. "Experimental analysis on the performance, combustion/emission characteristics of a DI diesel engine using hydrogen in dual fuel mode." International Journal of Hydrogen Energy. 52 (Part C), pp. 843-860. https://doi.org/10.1016/j.ijhydene.2022.04.129Experimental and numerical study to develop TRANSYS model for an active flat plate solar collector with an internally serpentine tube receiver
Al-Manea, Ahmed, Al-Rbaihat, Raed, Kadhim, Hakim T, Alahmer, Ali, Yusaf, Talal and Egab, Karim. 2022. "Experimental and numerical study to develop TRANSYS model for an active flat plate solar collector with an internally serpentine tube receiver." International Journal of Thermofluids. 15, pp. 1-9. https://doi.org/10.1016/j.ijft.2022.100189Numerical analysis of hybrid nanofluid natural convection in a wavy walled porous enclosure: Local thermal non-equilibrium model
Kadhim, Hakim T., Al-Manea, Ahmed, Al-Shamani, Ali Najah and Yusaf, Talal. 2022. "Numerical analysis of hybrid nanofluid natural convection in a wavy walled porous enclosure: Local thermal non-equilibrium model." International Journal of Thermofluids. 15, pp. 1-10. https://doi.org/10.1016/j.ijft.2022.100190Analysis of heat transfer and fluid flow in a microchannel heat sink with sidewall dimples and fillet profile
Okab, Abdullah K., Hasan, Hayder M., Hamzah, Mushtaq, Egab, Karim, Al-Manea, Ahmed and Yusaf, Talal. 2022. "Analysis of heat transfer and fluid flow in a microchannel heat sink with sidewall dimples and fillet profile." International Journal of Thermofluids. 15, pp. 1-8. https://doi.org/10.1016/j.ijft.2022.100192Transitional Boundary Layer Measurements in the University of Southern Queensland Hypersonic Wind Tunnel
Webb, Alister, Birch, Byrenn, Zander, Fabian and Buttsworth, David. 2022. "Transitional Boundary Layer Measurements in the University of Southern Queensland Hypersonic Wind Tunnel." 23rd Australasian Fluid Mechanics Conference (AFMC2022). Sydney, Australia 04 - 08 Dec 2022 Australia. University of Sydney.Mixing of Thermally Stratified Gas in Horizontal Tube Using Shock Waves Research Methodology
Grybko, Maciej, Malpress, Ray, Buttsworth, David, Zander, Fabian and Birch, Byrenn. 2022. "Mixing of Thermally Stratified Gas in Horizontal Tube Using Shock Waves Research Methodology." 23rd Australasian Fluid Mechanics Conference (AFMC2022). Sydney, Australia 04 - 08 Dec 2022 Australia. University of Sydney.Heated Barrel Mode for a Hypersonic Ludwieg Tube with Free-Piston Compression: Preliminary Performance
Birch, Byrenn, Malpress, Ray, Saleh, Khalid and Buttsworth, David. 2022. "Heated Barrel Mode for a Hypersonic Ludwieg Tube with Free-Piston Compression: Preliminary Performance." 23rd Australasian Fluid Mechanics Conference (AFMC2022). Sydney, Australia 04 - 08 Dec 2022 Australia. University of Sydney.Tunable Diode Laser Absorption Spectroscopy in a Supersonic Steam Jet
Al-Manea, Ahmed, Buttsworth, David, Leis, John and Saleh, Khalid. 2019. "Tunable Diode Laser Absorption Spectroscopy in a Supersonic Steam Jet." Alwahabi, Z. T. and Medwell, P. R. (ed.) 9th Australian Conference on Laser Diagnostics (IX ACLD). Adelaide, Australia 02 - 04 Dec 2019 Adelaide, Australia.Microwave technique for liquid water detection in icing applications
Leis, John, Buttsworth, David, Saeed, Ramiz, Saleh, Khalid, McGilvray, Matthew and Gillespie, David. 2019. "Microwave technique for liquid water detection in icing applications." 2019 International Conference on Icing of Aircraft, Engines, and Structures. Minneapolis, United States 17 - 21 Jun 2019 United States. https://doi.org/10.4271/2019-01-1930A new wind tunnel facility for ice crystal icing experiments
Saeed, Ramiz, Buttsworth, David and Saleh, Khalid. 2019. "A new wind tunnel facility for ice crystal icing experiments." 2019 International Conference on Icing of Aircraft, Engines, and Structures. Minneapolis, United States 17 - 21 Jun 2019 United States. https://doi.org/10.4271/2019-01-1926Hypersonic oscillating shock-wave/boundary-layer interaction on a flat plate
Currao, Gaetano M. D., McQuellin, Liam P., Neely, Andrew J., Gai, Sudhir L., O'Byrne, Sean, Zander, Fabian, Buttsworth, David R., McNamara, Jack J. and Jahn, Ingo. 2021. "Hypersonic oscillating shock-wave/boundary-layer interaction on a flat plate." AIAA Journal: devoted to aerospace research and development. 59 (3), pp. 940-959. https://doi.org/10.2514/1.J059590Tribological behavior of mild steel under canola biolubricant conditions
Shalwan, A., Yousif, B. F., Alajmi, F. H. and Alajmi, M.. 2021. "Tribological behavior of mild steel under canola biolubricant conditions." Advances in Tribology. 2021, pp. 1-13. https://doi.org/10.1155/2021/3795831Tribological studies of bamboo fibre reinforced epoxy composites using a BOD technique
Alajmi, Ayedh Eid, Alotaibi, Jasem Gh., Yousif, B. F. and Nirmal, Umar. 2021. "Tribological studies of bamboo fibre reinforced epoxy composites using a BOD technique." Polymers. 13 (15). https://doi.org/10.3390/polym13152444Epoxy and polyester composites’ characteristics under tribological loading conditions
Alotaibi, Jasem Ghanem, Alajmi, Ayedh Eid, Mehoub, Gabrel A. and Yousif, Belal F.. 2021. "Epoxy and polyester composites’ characteristics under tribological loading conditions." Polymer. 13. https://doi.org/10.3390/polym13142230Australian rapid-response airborne observation of the Hayabusa2 reentry
Zander, Fabian, Buttsworth, David R., Birch, Byrenn, Noller, Lachlan, Wright, Duncan, James, Christopher M., Thompson, Matthew, Apirana, Steven, Leis, John, Lobsey, Craig and Payne, Allan. 2021. "Australian rapid-response airborne observation of the Hayabusa2 reentry." Journal of Spacecraft and Rockets. 58 (6), pp. 1915-1919. https://doi.org/10.2514/1.A35062Fast-Response transient heat flux measurements in a plasma wind tunnel
Birch, Byrenn, Buttsworth, David, Loehle, Stefan and Hufgard, Fabian. 2021. "Fast-Response transient heat flux measurements in a plasma wind tunnel." International Journal of Heat and Mass Transfer. 173. https://doi.org/10.1016/j.ijheatmasstransfer.2021.121234CFD numerical and experimental investigation of two-phase flow development after an expansion device in a horizontal pipe
Mahmood, Raid Ahmed, Buttsworth, David, Malpress, Ray and Sharifian-Barforoush, Ahmed. 2021. "CFD numerical and experimental investigation of two-phase flow development after an expansion device in a horizontal pipe." Journal of Thermal Engineering. 7 (1), pp. 307- 323. https://doi.org/10.18186/thermal.850672Ice crystal icing wind tunnel at USQ: initial characterisation
Saeed, R. I., Saleh, Kh. and Buttsworth, D.. 2019. "Ice crystal icing wind tunnel at USQ: initial characterisation." Neely, Andrew (ed.) International Society for Air Breathing Engines (ISABE 2019). Canberra, Australia 22 - 27 Sep 2019 Indianapolis, United States.Tribological Performance of Materials Under Bio-Three Body Abrasion
Alotaibi, Jasem G., Omer, Ako K., Salih, Nbhan D. and Yousif, Belal F.. 2020. "Tribological Performance of Materials Under Bio-Three Body Abrasion." American Journal of Applied Sciences. 17 (1), pp. 161-170. https://doi.org/10.3844/ajassp.2020.161.170Effect of Fibre Content on Compressive and Flexural Properties of Coconut Fibre Reinforced Epoxy Composites
Alotaibi, Jasem G., Alajmi, Ayedh Eid, Yousif, Belal F. and Salih, Nbhan D.. 2020. "Effect of Fibre Content on Compressive and Flexural Properties of Coconut Fibre Reinforced Epoxy Composites." American Journal of Applied Sciences. 17 (1), pp. 141-155. https://doi.org/10.3844/ajassp.2020.141.155TUSQ hypersonic wind tunnel storage
Buttsworth, David. TUSQ hypersonic wind tunnel storage. Toowoomba. https://doi.org/10.26192/rps2-8e62Physical and mechanical properties of bamboo fibre/polyester composites subjected to moisture and hygrothermal conditions
Azwa, Z. N. and Yousif, B. F.. 2019. "Physical and mechanical properties of bamboo fibre/polyester composites subjected to moisture and hygrothermal conditions." Proceedings of the Institution of Mechanical Engineers Part L: Journal of Materials, Design and Applications. 233 (6). https://doi.org/10.1177/1464420717704221Experimental and numerical investigation of two-phase flow orientation direction change on a vertical flash tank separator
Mahmood, Raid Ahmed, Buttsworth, David and Malpress, Ray. 2019. "Experimental and numerical investigation of two-phase flow orientation direction change on a vertical flash tank separator." ISER 460th International Conference on Heat Transfer and Fluid Flow (ICHTFF 2019). Brisbane, Australia 08 - 09 Jan 2019Influence of Graphene Nanoplatelets on Mechanical Properties and Adhesive Wear Performance of Epoxy-Based Composites
Eayal Awwad, K. Y., Yousif, B. F., Fallahnezhad, Khosro, Saleh, Khalid and Zeng, Xuesen. 2021. "Influence of Graphene Nanoplatelets on Mechanical Properties and Adhesive Wear Performance of Epoxy-Based Composites." Friction. 9 (4), pp. 856-875. https://doi.org/10.1007/s40544-020-0453-5Tribological investigation of frictional behaviour of mild steel under canola bio-lubricant conditions
Shalwan, A., Yousif, B. F., Alajmi, F. H., Alrashdana, K. R. and Alajmi, M.. 2020. "Tribological investigation of frictional behaviour of mild steel under canola bio-lubricant conditions." Tribology in Industry. 42 (3), pp. 481-493. https://doi.org/10.24874/ti.895.05.20.09Ethylene Detection using Laser Modulated Photoacoustic Resonance at 1625nm
Leis, John and Buttsworth, David. 2019. "Ethylene Detection using Laser Modulated Photoacoustic Resonance at 1625nm." Alwahabi, Z.T. and Medwell, P.R. (ed.) 9th Australian Conference on Laser Diagnostics (IX ACLD). Adelaide, Australia 02 - 04 Dec 2019 Adelaide, Australia.An Experimental and Numerical Investigation into the Aerodynamics of the ISS Re-entry
Zander, F., Leiser, D., Choudhury, R., Loehle, S. and Buttsworth, D.R.. 2018. "An Experimental and Numerical Investigation into the Aerodynamics of the ISS Re-entry." Lau, T.C.W. and Kelso, R.M. (ed.) 21st Australasian Fluid Mechanics Conference (AFMC 2018). Adelaide, Australia 10 - 13 Dec 2018 Australia. Australasian Fluid Mechanics Society.Computational and Experimental Investigation of the Vertical Flash Tank Separator Part 1: Effect of Parameters on Separation Efficiency
Mahmood, Raid Ahmed, Buttsworth, David and Malpress, Ray. 2019. "Computational and Experimental Investigation of the Vertical Flash Tank Separator Part 1: Effect of Parameters on Separation Efficiency." International Journal of Air-Conditioning and Refrigeration. 27 (1), pp. 1950005-1. https://doi.org/10.1142/S2010132519500056Time-resolved stagnation temperature measurements in hypersonic flows using surface junction thermocouples
Birch, Byrenn, Buttsworth, David and Zander, Fabian. 2020. "Time-resolved stagnation temperature measurements in hypersonic flows using surface junction thermocouples." Experimental Thermal and Fluid Science. 119, pp. 1-13. https://doi.org/10.1016/j.expthermflusci.2020.110177Measurements of freestream density fluctuations in a hypersonic wind tunnel
Birch, Byrenn, Buttsworth, David and Zander, Fabian. 2020. "Measurements of freestream density fluctuations in a hypersonic wind tunnel." Experiments in Fluids: experimental methods and their applications to fluid flow. 61 (7), pp. 1-13. https://doi.org/10.1007/s00348-020-02992-wRobust approach for depth of anaesthesia assessment based on hybrid transform and statistical features
Diykh, Mohammed, Miften, Firas Sabar, Abdulla, Shahab, Saleh, Khalid and Green, Jonathan H.. 2020. "Robust approach for depth of anaesthesia assessment based on hybrid transform and statistical features." IET Science, Measurement and Technology. 14 (1), pp. 128-136. https://doi.org/10.1049/iet-smt.2018.5393Detached eddy simulation of an adjustable radial ejector
Al-Rbaihat, R., Malpress, R., Buttsworth, D. and Saleh, Kh.. 2018. "Detached eddy simulation of an adjustable radial ejector." Lau, T. C. W. and Kelso, R. M. (ed.) 21st Australasian Fluid Mechanics Conference (AFMC 2018). Adelaide, Australia 10 - 13 Dec 2018 Australia. Australasian Fluid Mechanics Society.Supersonic combustion of hydrogen in Mach 2 flows over an axisymmetric model
Zhao, Mengmeng, Buttsworth, David and Choudhury, Rishabh. 2018. "Supersonic combustion of hydrogen in Mach 2 flows over an axisymmetric model." 22nd AIAA International Space Planes and Hypersonics Systems and Technologies Conference (AIAA 2018). Orlando, United States 17 - 19 Sep 2018 United States. https://doi.org/10.2514/6.2018-5252Experimental and Numerical Investigation of Two-phase Flow Orientation Direction Change on a Vertical Flash Tank Separator
Mahmood, Raid Ahmed, Buttsworth, David and Malpress, Ray. 2019. "Experimental and Numerical Investigation of Two-phase Flow Orientation Direction Change on a Vertical Flash Tank Separator ." International Journal of Management and Applied Science (IJMAS). 5 (4), pp. 25-29.Energy sector development: system dynamics analysis
Laimon, Mohamd, Mai, Thanh, Goh, Steven and Yusaf, Talal. 2019. "Energy sector development: system dynamics analysis." Applied Sciences. 10 (1), pp. 1-19. https://doi.org/10.3390/app10010134Computational and experimental investigation of using an extractor in a vertical gravitational flash tank separator
Mahmood, R. A., Buttsworth, D. and Malpress, R.. 2019. "Computational and experimental investigation of using an extractor in a vertical gravitational flash tank separator." International Journal of Automotive and Mechanical Engineering. 16 (2), pp. 6706-6722.Pitching Model with Control Surface in Hypersonic Flow: Short Duration Ground Tests and Simulations
Stern Nathan and Buttsworth David R.. 2020. "Pitching Model with Control Surface in Hypersonic Flow: Short Duration Ground Tests and Simulations ." 23rd AIAA International Space Planes and Hypersonic Systems and Technologies Conference (AIAA 2020). Montreal, Quebec, Canada 10 - 12 Mar 2020 Canada. https://doi.org/10.2514/6.2020-2452Fractal dimension undirected correlation graph-based support vector machine model for identification of focal and non-focal electroencephalography signals
Diykh, Mohammed, Abdulla, Shahab, Saleh, Khalid and Deo, Ravinesh C.. 2019. "Fractal dimension undirected correlation graph-based support vector machine model for identification of focal and non-focal electroencephalography signals." Biomedical Signal Processing and Control. 54, pp. 1-10. https://doi.org/10.1016/j.bspc.2019.101611Sleep EEG signal analysis based on correlation graph similarity coupled with an ensemble extreme machine learning algorithm
Abdulla, Shahab, Diykh, Mohammed, Lafta, Raid Luaibi, Saleh, Khalid and Deo, Ravinesh C.. 2019. "Sleep EEG signal analysis based on correlation graph similarity coupled with an ensemble extreme machine learning algorithm." Expert Systems with Applications. 138, pp. 1-15. https://doi.org/10.1016/j.eswa.2019.07.007The influence of emulsified water fuel containing fresh water microalgae on diesel engine performance, combustion, vibration and emission
Al-lwayzy, Saddam H., Yusaf, Talal, Saleh, Khalid and Yousif, Belal. 2019. "The influence of emulsified water fuel containing fresh water microalgae on diesel engine performance, combustion, vibration and emission." Energies. 12 (13), pp. 1-17. https://doi.org/10.3390/en12132546Measurement of water vapour in axisymmetric jet development using TDLAS
Al-Manea, A., Buttsworth, D., Leis, J., Choudhury, R. and Saleh, K.. 2018. "Measurement of water vapour in axisymmetric jet development using TDLAS." Lau, T. C. W. and Kelso, R. M. (ed.) 21st Australasian Fluid Mechanics Conference (AFMC 2018). Adelaide, Australia 10 - 13 Dec 2018 Australia. Australasian Fluid Mechanics Society.Experimental and numerical study of OH* chemiluminescence in hydrogen diffusion flames
Zhao, Mengmeng, Buttsworth, David and Choudhury, Rishabh. 2018. "Experimental and numerical study of OH* chemiluminescence in hydrogen diffusion flames." Combustion and Flame. 197, pp. 369-377. https://doi.org/10.1016/j.combustflame.2018.08.019Oscillating shock impinging on a flat plate at Mach 6
Currao, Gaetano M. D., McQuellin, Liam P., Neely, Andrew J., Zander, Fabian, Buttsworth, David R., McNamara, Jack J. and Jahn, Ingo. 2019. "Oscillating shock impinging on a flat plate at Mach 6." AIAA Aviation Forum 2019. Dallas, United States 17 - 21 Jun 2019 United States. https://doi.org/10.2514/6.2019-3077Spacecraft Material Tests under Aerothermal and Mechanical Reentry Loads
Leiser, David, Lohle, Stefan, Zander, Fabian, Fasoulas, Stefanos, Buttsworth, David R. and Choudhury, Rishabh. 2019. "Spacecraft Material Tests under Aerothermal and Mechanical Reentry Loads." AIAA SciTech Forum 2019 . San Diego, United States 07 - 11 Jan 2019 United States. American Institute of Aeronautics and Astronautics. https://doi.org/10.2514/6.2019-0161Mixtures of n-butanol and iso-butanol blended with diesel: experimental investigation of combustion characteristics, engine performance and emission levels in a compression ignition engine
Algayyim, Sattar Jabbar Murad, Wandel, Andrew P. and Yusaf, Talal. 2021. "Mixtures of n-butanol and iso-butanol blended with diesel: experimental investigation of combustion characteristics, engine performance and emission levels in a compression ignition engine." Biofuels. 12 (4), pp. 439-448. https://doi.org/10.1080/17597269.2018.1493565Pulsed ultrasound as an energy saving mode for ultrasound treatment of surface water with terrestrial aquatic carbon
Al-juboori, Raed A., Yusaf, Talal and Bowtell, Les. 2018. "Pulsed ultrasound as an energy saving mode for ultrasound treatment of surface water with terrestrial aquatic carbon." Desalination and Water Treatment. 135, pp. 167-176. https://doi.org/10.5004/dwt.2018.23173Toughening of brittle polyester with functionalized halloysite nanocomposites
Albdiry, M. T. and Yousif, B. F.. 2019. "Toughening of brittle polyester with functionalized halloysite nanocomposites." Composites Part B: Engineering. 160, pp. 94-109. https://doi.org/10.1016/j.compositesb.2018.10.032The influence of fibre orientation on tribological performance of jute fibre reinforced epoxy composites considering different mat orientations
Alshammari, F. Z., Saleh, K. H., Yousif, B. F., Alajmi, A., Shalwan, A. and Alotaibi, J. G.. 2018. "The influence of fibre orientation on tribological performance of jute fibre reinforced epoxy composites considering different mat orientations." Tribology in Industry. 40 (3), pp. 335-348. https://doi.org/10.24874/ti.2018.40.03.01Fracture behaviour of bamboo fiber reinforced epoxy composites
Khan, Ziaullah, Yousif, B. F. and Islam, Mainul. 2017. "Fracture behaviour of bamboo fiber reinforced epoxy composites." Composites Part B: Engineering. 116, pp. 186-199. https://doi.org/10.1016/j.compositesb.2017.02.015Study of an axisymmetric backward facing step model in a supersonic flow
Zhao, Mengmeng, Buttsworth, David R., Choudhury, Rishabh, Malpress, Ray and Xing, Fei. 2016. "Study of an axisymmetric backward facing step model in a supersonic flow." 21st AIAA International Space Planes and Hypersonics Technologies Conference (Hypersonics 2017). Xiamen, China 06 - 09 Mar 2017 United States. https://doi.org/10.2514/6.2017-2181Determining the Convergence of Synchronous Measurements for Embedded Industrial Applications
Leis, John and Buttsworth, David. 2017. "Determining the Convergence of Synchronous Measurements for Embedded Industrial Applications." IEEE Transactions on Industrial Electronics. 64 (9), pp. 7392-7398. https://doi.org/10.1109/TIE.2016.2638798Butanol-acetone mixture blended with cottonseed biodiesel: spray characteristics evolution, combustion characteristics, engine performance and emission
Algayyim, Sattar Jabbar Murad, Wandel, Andrew P., Yusaf, Talal and Al-lwayzy, Saddam. 2019. "Butanol-acetone mixture blended with cottonseed biodiesel: spray characteristics evolution, combustion characteristics, engine performance and emission." Proceedings of the Combustion Institute. 37 (4), pp. 4729-4739. https://doi.org/10.1016/j.proci.2018.08.035Experimental investigation of hydrated butanol-acetone (BA) and diesel blend as alternative fuel for CI engines
Algayyim, Sattar Jabbar Murad, Wandel, Andrew P. and Yusaf, Talal. 2018. "Experimental investigation of hydrated butanol-acetone (BA) and diesel blend as alternative fuel for CI engines." 37th International Symposium on Combustion. Dublin, Ireland 29 Jul - 03 Aug 2018 Pittsburgh, PA, United States.Experimental and numerical investigation of spray characteristics of butanol-diesel blends
Algayyim, Sattar Jabbar Murad, Wandel, Andrew P. and Yusaf, Talal. 2017. "Experimental and numerical investigation of spray characteristics of butanol-diesel blends." Masri, A. R., Cleary, M., Dunn, M., Kourmatzis, A., Hawkes, E., Kook, S. and Chan, Q. N. (ed.) 11th Asia-Pacific Conference on Combustion. Sydney, Australia 10 - 14 Dec 2017 Australia.Experimental study of spray characteristics, engine performance and emission levels of acetone-butanol-ethanol mixture-diesel blends in a diesel engine
Algayyim, Sattar Jabbar Murad, Wandel, Andrew, Yusaf, Talal, Hamawand, Ihsan and Al-lwayzy, Saddam. 2017. "Experimental study of spray characteristics, engine performance and emission levels of acetone-butanol-ethanol mixture-diesel blends in a diesel engine." Masri, A. R., Cleary, M., Dunn, M., Kourmatzis, A., Hawkes, E., Kook, S. and Chan, Q. N. (ed.) 11th Asia-Pacific Conference on Combustion. Sydney, Australia 10 - 14 Dec 2017 Australia.The effect of butanol-acetone mixture-cottonseed biodiesel blend on spray characteristics, engine performance and emissions in diesel engine
Algayyim, Sattar Jabbar Murad, Wandel, Andrew P. and Yusaf, Talal. 2017. "The effect of butanol-acetone mixture-cottonseed biodiesel blend on spray characteristics, engine performance and emissions in diesel engine." Masri, A. R., Cleary, M., Dunn, M., Kourmatzis, A., Hawkes, E., Kook, S. and Chan, Q. N. (ed.) 11th Asia-Pacific Conference on Combustion. Sydney, Australia 10 - 14 Dec 2017 Australia.The impact of injector hole diameter on spray behaviour for butanol-diesel blends
Algayyim, Sattar Jabbar Murad, Wandel, Andrew P. and Yusaf, Talal. 2018. "The impact of injector hole diameter on spray behaviour for butanol-diesel blends." Energies. 11 (5), pp. 1298-1310. https://doi.org/10.3390/en11051298Impact of butanol-acetone mixture as a fuel additive on diesel engine performance and emissions
Algayyim, Sattar Jabbar Murad, Wandel, Andrew P., Yusaf, Talal, Al-Lwayzy, Saddam and Hamawand, Ihsan. 2018. "Impact of butanol-acetone mixture as a fuel additive on diesel engine performance and emissions." Fuel: the science and technology of fuel and energy. 227, pp. 118-126. https://doi.org/10.1016/j.fuel.2018.04.091Production and application of ABE as a biofuel
Algayyim, Sattar Jabbar Murad, Wandel, Andrew P., Yusaf, Talal and Hamawand, Ihsan. 2018. "Production and application of ABE as a biofuel." Renewable and Sustainable Energy Reviews. 82, pp. 1195-1214. https://doi.org/10.1016/j.rser.2017.09.082A critical review on processes and energy profile of the Australian meat processing industry
Hamawand, Ihsan, Ghadouani, Anas, Bundschuh, Jochen, Hamawand, Sara, Al Juboori, Raed A., Chakrabarty, Sayan and Yusaf, Talal. 2017. "A critical review on processes and energy profile of the Australian meat processing industry." Energies. 10 (5). https://doi.org/10.3390/en10050731The impact of n-butanol and iso-butanol as components of butanol-acetone (BA) mixture-diesel blend on spray, combustion characteristics, engine performance and emission in direct injection diesel engine
Algayyim, Sattar Jabbar Murad, Wandel, Andrew P., Yusaf, Talal and Hamawand, Ihsan. 2017. "The impact of n-butanol and iso-butanol as components of butanol-acetone (BA) mixture-diesel blend on spray, combustion characteristics, engine performance and emission in direct injection diesel engine." Energy. 140, pp. 1074-1086. https://doi.org/10.1016/j.energy.2017.09.044A Robust Method for Tuning Photoacoustic Gas Detectors
Leis, John and Buttsworth, David. 2018. "A Robust Method for Tuning Photoacoustic Gas Detectors." IEEE Transactions on Industrial Electronics. 65 (5), pp. 4338-4346. https://doi.org/10.1109/TIE.2017.2762636Investigation of ethanol production potential from lignocellulosic material without enzymatic hydrolysis using the ultrasound technique
Kandasamy, Manoj, Hamawand, Ihsan, Bowtell, Leslie, Seneweera, Saman, Chakrabarty, Sayan, Yusaf, Talal, Shakoor, Zaidoon, Algayyim, Sattar and Eberhard, Friederike. 2017. "Investigation of ethanol production potential from lignocellulosic material without enzymatic hydrolysis using the ultrasound technique." Energies. 10 (1), pp. 62-73. https://doi.org/10.3390/en10010062Analysis of hypersonic model pitching experiments in the TUSQ facility
Stern, Nathan, Jahn, Ingo, Choudbhury, Rishabh, Zander, Fabian, Steelant, Johan and Buttsworth, David R.. 2018. "Analysis of hypersonic model pitching experiments in the TUSQ facility." International Conference on High-Speed Vehicle Science and Technology 2018 (HiSST 2018). Moscow, Russia 26 - 29 Nov 2018Simulation in the Loop Control of a Planar Hypersonic Wing with a Rigid Control Surface
Jahn, I. H., Zander, F., Stern, N. and Buttsworth, D. R.. 2018. "Simulation in the Loop Control of a Planar Hypersonic Wing with a Rigid Control Surface." 21st Australasian Fluid Mechanics Conference (AFMC 2018). Adelaide, Australia 10 - 13 Dec 2018 Australia. Australasian Fluid Mechanics Society.Algae-biomass for fuel, electricity and agriculture
Bundschuh, Jochen, Yusaf, Talal, Maity, Jyoti Prakash, Nelson, Emily, Mamat, Rizalman and Mahlia, T. M. Indra. 2014. "Algae-biomass for fuel, electricity and agriculture ." Energy. 78, pp. 1-3. https://doi.org/10.1016/j.energy.2014.11.005Influence of chemical blends on palm oil methyl esters' cold flow properties and fuel characteristics
Ali, Obed M., Yusaf, Talal, Mamat, Rizalman, Abdullah, Nik R. and Abdullah, Abdul Adam. 2014. "Influence of chemical blends on palm oil methyl esters' cold flow properties and fuel characteristics." Energies. 7 (7), pp. 4364-4380. https://doi.org/10.3390/en7074364Sustainable energy and climate protection solutions in agriculture
Bundschuh, Jochen, Chen, Guangnan, Yusaf, Talal, Yan, Jinyue and Chen, Shulin. 2014. "Sustainable energy and climate protection solutions in agriculture ." Applied Energy. 114, pp. 735-736. https://doi.org/10.1016/j.apenergy.2013.11.037Optimization of biodiesel-diesel blended fuel properties and engine performance with ether additive using statistical analysis and response surface methods
Ali, Obed M., Mamat, Rizalman, Najafi, Gholamhassan, Yusaf, Talal and Ardebili, Seyed Mohammad Safieddin. 2015. "Optimization of biodiesel-diesel blended fuel properties and engine performance with ether additive using statistical analysis and response surface methods." Energies. 8 (12), pp. 14136-14150. https://doi.org/10.3390/en81212420TOPSIS multi-criteria decision modeling approach for biolubricant selection for two-stroke petrol engines
Soufi, Masoud Dehghani, Ghobadian, Barat, Najafi, Gholamhassan, Sabzimaleki, Mohammad Reza and Yusaf, Talal. 2015. "TOPSIS multi-criteria decision modeling approach for biolubricant selection for two-stroke petrol engines." Energies. 8 (12), pp. 13960-13970. https://doi.org/10.3390/en81212408Combustion of microalgae oil and ethanol blended with diesel fuel
Al-lwayzy, Saddam H. and Yusaf, Talal. 2015. "Combustion of microalgae oil and ethanol blended with diesel fuel." Energies. 8 (12), pp. 13985-13995. https://doi.org/10.3390/en81212409Glycerin, a biodiesel by-product with potentiality to produce hydrogen by steam gasification
Suero, Silvia Roman, Cano, Beatriz Ledesma, Alvarez-Murillo, Andres, Al-Kassir, Awf and Yusaf, Talal. 2015. "Glycerin, a biodiesel by-product with potentiality to produce hydrogen by steam gasification." Energies. 8 (11), pp. 12765-12775. https://doi.org/10.3390/en81112339SVM and ANFIS for prediction of performance and exhaust emissions of a SI engine with gasoline-ethanol blended fuels
Najafi, G., Ghobadian, B., Moosavian, A., Yusaf, T., Mamat, R., Kettner, M. and Azmi, W. H.. 2016. "SVM and ANFIS for prediction of performance and exhaust emissions of a SI engine with gasoline-ethanol blended fuels." Applied Thermal Engineering. 95, pp. 186-203. https://doi.org/10.1016/j.applthermaleng.2015.11.009Synthesis and optimization of Hevea brasiliensis and Ricinus communis as feedstock for biodiesel production: a comparative study
Silitonga, A. S., Masjuki, H. H., Ong, Hwai Chyuan, Kusumo, F., Yusaf, Talal and Mahlia, T. M. I.. 2016. "Synthesis and optimization of Hevea brasiliensis and Ricinus communis as feedstock for biodiesel production: a comparative study." Industrial Crops and Products. 85, pp. 274-286. https://doi.org/10.1016/j.indcrop.2016.03.017A temperature compensation technique for near-infrared methane gas threshold detection
Leis, John and Buttsworth, David. 2016. "A temperature compensation technique for near-infrared methane gas threshold detection." IEEE Transactions on Industrial Electronics. 63 (3), pp. 1813-1821. https://doi.org/10.1109/TIE.2015.2495292Impact of butanol-acetone mixture as an oxygenated fuel additive to diesel fuel on diesel engine performance and emissions
Algayyim, Sattar, Wandel, Andrew, Yusaf, Talal, Hamawand, Ihsan and Al-lwayzy, Saddam. 2016. "Impact of butanol-acetone mixture as an oxygenated fuel additive to diesel fuel on diesel engine performance and emissions." 36th International Symposium on Combustion. Seoul, Korea 31 Jul - 05 Aug 2016The experimental investigation of butanol-diesel blend on engine performance and emission levels in DI diesel engine
Algayyim, Sattar, Wandel, Andrew P., Yusaf, Talal, Hamawand, Ihsan and Al-lwayzy, Saddam. 2017. "The experimental investigation of butanol-diesel blend on engine performance and emission levels in DI diesel engine." 1st MoHESR and HCED Iraq Scholars Conference in Australasia 2017 (ISCA 2017). Melbourne, Australia 05 - 06 Dec 2017 Melbourne, Australia.Investigation into thin layer drying rates and equilibrium moisture content of abattoir paunch waste
Spence, Jennifer, Buttsworth, David, McCabe, Bernadette K., Baillie, Craig, Antille, Diogenes L. and Carter, Brad. 2018. "Investigation into thin layer drying rates and equilibrium moisture content of abattoir paunch waste." Renewable Energy. 124, pp. 95-102. https://doi.org/10.1016/j.renene.2017.07.082Absolute concentration measurements of OH* in an axisymmetric hydrogen-air premixed flame adjacent to a hot graphite model
Zhao, M. M., Choudhury, R., Malpress, R. and Buttsworth, D. R.. 2016. "Absolute concentration measurements of OH* in an axisymmetric hydrogen-air premixed flame adjacent to a hot graphite model." 20th Australasian Fluid Mechanics Conference (AFMC 2016). Perth, Australia 05 - 08 Dec 2016 Australia.SI: materials, design and tribology
Yousif, B. F.. 2013. "SI: materials, design and tribology." Materials and Design. 48. https://doi.org/10.1016/j.matdes.2013.01.009Design, fabrication and evaluation of gamma-type stirling engine to produce electricity from biomass for the micro-CHP system
Damirchi, Hojjat, Najafi, Gholam Hassan, Alizadehnia, Siamak, Ghobadian, Barat, Yusaf, Talal and Mamat, Rizalman. 2015. "Design, fabrication and evaluation of gamma-type stirling engine to produce electricity from biomass for the micro-CHP system." The 7th International Conference on Applied Energy - ICAE2015. Abu Dhabi, United Arab Emirates 28 - 31 Mar 2015 Netherlands. https://doi.org/10.1016/j.egypro.2015.07.240Energy conversion efficiency of pulsed ultrasound
Al-Juboori, Raed A., Yusaf, Talal and Bowtell, Leslie. 2015. "Energy conversion efficiency of pulsed ultrasound." The 7th International Conference on Applied Energy - ICAE2015. Abu Dhabi, United Arab Emirates 28 - 31 Mar 2015 Netherlands. Elsevier. https://doi.org/10.1016/j.egypro.2015.07.340The study of air fuel ratio for open furnace MILD combustion of biogas on bluff-body burner
Noor, M. M., Wandel, Andrew P. and Yusaf, T. F.. 2012. "The study of air fuel ratio for open furnace MILD combustion of biogas on bluff-body burner." 2012 Southern Regional Engineering Conference (SREC 2012). Toowoomba, Australia 01 Sep 2012 Canberra, Australia.Visible and near infrared spectroscopy of Hayabusa re-entry using semi-autonomous tracking
McIntyre, T. J., Khan, R., Eichmann, T. N., Upcroft, B. and Buttsworth, D.. 2012. "Visible and near infrared spectroscopy of Hayabusa re-entry using semi-autonomous tracking ." 50th AIAA Aerospace Sciences Meeting Including the New Horizons Forum and Aerospace Exposition: Advancing the Science of Flight Technology (AIAA 2012) . Nashville, United States 09 - 12 Jan 2012 Reston, VA. United States.Biofuel from microalgae: alternative, sustainable and renewable fuel
Najafi, Gholamhassan, Ghobadian, Barat and Yusaf, Talal F.. 2011. "Biofuel from microalgae: alternative, sustainable and renewable fuel ." 10th International Conference on Sustainable Energy Technologies (SET 2011). Istanbul, Turkey 04 - 07 Sep 2011Development of a photo-acoustic trace gas sensor
Kelly, C., Leis, J. and Buttsworth, D.. 2011. "Development of a photo-acoustic trace gas sensor." Mee, David J. and Hillock, Ian D. M. (ed.) 2011 Conference of the Australian Acoustical Society (Acoustics 2011): Breaking New Ground. Gold Coast, Australia 02 - 04 Nov 2011 Australia. Australian Acoustical Society.Evaluation of alternative energy sources for cotton production in Australia
Chen, Guangnan, Sandell, Gary, Yusaf, Talal and Baillie, Craig. 2013. "Evaluation of alternative energy sources for cotton production in Australia." SEAg 2013: Innovative Agricultural Technologies for a Sustainable Future. Barton, Australia 22 - 25 Sep 2013 Barton, ACT, Australia.Corrosion effects of CNT-nanofluids on different metals
Ismail, A. F., Anuar, A., Rashmi, W. and Yusaf, T.. 2014. "Corrosion effects of CNT-nanofluids on different metals." 3rd International Conference on Advanced Materials and Information Technology Processing (AMITP 2013). Los Angeles, United States 01 - 02 Dec 2013 Southampton, United Kingdom. https://doi.org/10.2495/AMITP20130171Wear and frictional behaviour of metals
Alotaibi, J. G., Yousif, B. F. and Yusaf, T. F.. 2014. "Wear and frictional behaviour of metals." Kida, Katsuyuki (ed.) 3rd International Conference on Advanced Materials and Engineering Materials (ICAMEM 2013). Singapore 14 - 15 Dec 2013 Zurich, Switzerland. https://doi.org/10.4028/www.scientific.net/AMR.893.430The development of MILD combustion open burner experimental setup
Noor, M. M., Wandel, Andrew P. and Yusaf, Talal. 2013. "The development of MILD combustion open burner experimental setup." Ishak, Mahadzir and Rahman, Md. Mustafizur (ed.) 2nd International Conference of Mechanical Engineering Research (ICMER 2013). Pahang, Malaysia 01 - 03 Jul 2013 Pahang, Malaysia.Detail guide for CFD on the simulation of biogas combustion in bluff-body mild burner
Noor, M. M., Wandel, Andrew P. and Yusaf, Talal. 2013. "Detail guide for CFD on the simulation of biogas combustion in bluff-body mild burner." Ishak, Mahadzir and Rahman, Md. Mustafizur (ed.) 2nd International Conference of Mechanical Engineering Research (ICMER 2013). Pahang, Malaysia 01 - 03 Jul 2013 Pahang, Malaysia.Mild combustion: the future for lean and clean combustion
Yusaf, Talal, Noor, M. M. and Wandel, Andrew P.. 2013. "Mild combustion: the future for lean and clean combustion." Ishak, Mahadzir and Rahman, Md. Mustafizur (ed.) 2nd International Conference of Mechanical Engineering Research (ICMER 2013). Pahang, Malaysia 01 - 03 Jul 2013 Pahang, Malaysia.Alternative energy sources fro cotton production
Baillie, Craig, Sandell, Gary, Chen, Guangnan and Yusaf, Talal. 2012. "Alternative energy sources fro cotton production." 16th Australian Cotton Conference (ACC 2012): Growing Better all the Time. Gold Coast, Australia 14 - 16 Aug 2012 Gold Coast, Australia.Investigation of the effect of additives to natural gas on heavy-duty SI engine combustion characteristics
Gharehghani, A., Hosseini, R. and Yusaf, T.. 2013. "Investigation of the effect of additives to natural gas on heavy-duty SI engine combustion characteristics." Journal of Mechanical Engineering and Sciences. 5, pp. 677-687. https://doi.org/10.15282/jmes.5.2013.14.0065Characterization of a diesel engine operating with a small proportion of methanol as a fuel additive in biodiesel blend
Mat Yasin, M. H., Yusaf, Talal, Mamat, R. and Yusop, A. Fitri. 2014. "Characterization of a diesel engine operating with a small proportion of methanol as a fuel additive in biodiesel blend." Applied Energy. 114, pp. 865-873. https://doi.org/10.1016/j.apenergy.2013.06.012An investigation on tensile, compression and flexural properties of natural fibre reinforced polyester composites
Yousif, B. F., Jye, W. K. and El-Tayeb, N. S. M.. 2007. "An investigation on tensile, compression and flexural properties of natural fibre reinforced polyester composites." 2007 ASME International Mechanical Engineering Congress and Exposition (IMECE 2007). Seattle, United States of America 11 - 15 Nov 2007 Boston, Massachusetts, USA.Transesterification of Nannochloropsis oculata microalga's oil to biodiesel using calcium methoxide catalyst
Teo, Siow Hwa, Islam, Aminul, Yusaf, Talal and Taufiq-Yap, Yun Hin. 2014. "Transesterification of Nannochloropsis oculata microalga's oil to biodiesel using calcium methoxide catalyst." Energy. 78, pp. 63-71. https://doi.org/10.1016/j.energy.2014.07.045Investigation on interfacial adhesion of date palm/epoxy using fragmentation technique
Shalwan, A. and Yousif, B. F.. 2014. "Investigation on interfacial adhesion of date palm/epoxy using fragmentation technique." Materials and Design. 53, pp. 928-937. https://doi.org/10.1016/j.matdes.2013.07.083Experimental investigation on the interfacial adhesion of date palm fibres with epoxy matrix
Alsaeed, T., Alajmi, A., Yousif, B. F., Ku, H. and Lau, Alan Kin-tak. 2012. "Experimental investigation on the interfacial adhesion of date palm fibres with epoxy matrix." Heslehurst, Rikard Benton and Byrne, Ann (ed.) Composites Australia and the CRC for Advanced Composite Structures Conference 2012: Diversity in Composites. Leura, Australia 15 - 16 Mar 2012 Melbourne, Australia.Numerical and experimental study of microorganism disruption using shock treatment
Yusaf, Talal and Al Juboori, Raed A.. 2015. "Numerical and experimental study of microorganism disruption using shock treatment." Journal of Biotechnology. 208 (Supplement), pp. S15-S15. https://doi.org/10.1016/j.jbiotec.2015.06.035Investigating natural organic carbon removal and structural alteration induced by pulsed ultrasound
Al-Juboori, Raed A., Yusaf, Talal, Aravinthan, Vasantha and Bowtell, Leslie. 2016. "Investigating natural organic carbon removal and structural alteration induced by pulsed ultrasound." Science of the Total Environment. 541, pp. 1019-1030. https://doi.org/10.1016/j.scitotenv.2015.09.143Insights into the scalability of magnetostrictive ultrasound technology for water treatment applications
Al-Juboori, Raed A., Bowtell, Leslie A., Yusaf, Talal and Aravinthan, Vasantha. 2016. "Insights into the scalability of magnetostrictive ultrasound technology for water treatment applications." Ultrasonics Sonochemistry. 28, pp. 357-366. https://doi.org/10.1016/j.ultsonch.2015.08.013Determination of drying characteristics of biomass residues to be used as a renewable fuel
Al Kassir, Awf, Yusaf, Talal, Al Karany, Raul Kassir and Hamawand, Ihsan. 2015. "Determination of drying characteristics of biomass residues to be used as a renewable fuel." Journal of Biotechnology. 208 (Supplement), pp. S15-S15. https://doi.org/10.1016/j.jbiotec.2015.06.034Single-zone zero-dimensional model study for diesel-fuelled homogeneous charge compression ignition (HCCI) engines using Cantera
Hairuddin, A. Aziz, Yusaf, Talal and Wandel, Andrew P.. 2016. "Single-zone zero-dimensional model study for diesel-fuelled homogeneous charge compression ignition (HCCI) engines using Cantera." International Journal of Automotive and Mechanical Engineering. 13 (2), pp. 3309-3328. https://doi.org/10.15282/ijame.13.2.2016.3.0275Diesel engine performance and exhaust gas emissions using microalgae Chlorella protothecoides biodiesel
Al-lwayzy, Saddam H. and Yusaf, Talal. 2017. "Diesel engine performance and exhaust gas emissions using microalgae Chlorella protothecoides biodiesel." Renewable Energy. 101, pp. 690-701. https://doi.org/10.1016/j.renene.2016.09.035Bioenergy from cotton industry wastes: a review and potential
Hamawand, Ihsan, Sandell, Gary, Pittaway, Pam, Chakrabarty, Sayan, Yusaf, Talal, Chen, Guangnan, Seneweera, Saman, Al-Lwayzy, Saddam, Bennett, John and Hopf, Joshua. 2016. "Bioenergy from cotton industry wastes: a review and potential." Renewable and Sustainable Energy Reviews. 66, pp. 435-448. https://doi.org/10.1016/j.rser.2016.08.033Temperature and heat flux measurement on hot models in short-duration facilities
Kraetzig, Benjamin, Buttsworth, David R., Zander, Fabian and Loehle, Stefan. 2014. "Temperature and heat flux measurement on hot models in short-duration facilities." Journal of Thermophysics and Heat Transfer. 29 (1), pp. 37-46. https://doi.org/10.2514/1.T4309An assessment of direct on-farm energy use for high value grain crops grown under different farming practices in Australia
Maraseni, Tek, Chen, Guangnan, Banhazi, Thomas, Bundschuh, Jochen and Yusaf, Talal. 2015. "An assessment of direct on-farm energy use for high value grain crops grown under different farming practices in Australia." Energies. 8 (11), pp. 13033-13046. https://doi.org/10.3390/en81112353Mixing layer effects on the entrainment ratio in steam ejectors through ideal gas computational simulations
Ariafar, Kavous, Buttsworth, David, Al-Doori, Ghassan and Sharifi, Navid. 2016. "Mixing layer effects on the entrainment ratio in steam ejectors through ideal gas computational simulations." Energy. 95, pp. 380-392. https://doi.org/10.1016/j.energy.2015.12.027Tracking ultrasonically structural changes of natural aquatic organic carbon: chemical fractionation and spectroscopic approaches
Al-Juboori, Raed A., Yusaf, Talal, Aravinthan, Vasantha and Bowtell, Leslie. 2016. "Tracking ultrasonically structural changes of natural aquatic organic carbon: chemical fractionation and spectroscopic approaches." Chemosphere. 145, pp. 231-248. https://doi.org/10.1016/j.chemosphere.2015.11.079Natural fibers and their characterization
Azwa, Z. N., Yousif, B. F., Manalo, A. C. and Karunasena, W.. 2016. "Natural fibers and their characterization." Campilho, R. D. S. G. (ed.) Natural fiber composites. Boca Raton, FL, United States. CRC Press. pp. 35-64Biolubricants and the potential of waste cooking oil
Alotaibi, J. G. and Yousif, B. F.. 2016. "Biolubricants and the potential of waste cooking oil." Davim, J. Paulo (ed.) Ecotribology, research developments. Switzerland. Springer. pp. 125-143Two-body abrasion of bamboo fibre/epoxy composites
Oun, A. and Yousif, B. F.. 2016. "Two-body abrasion of bamboo fibre/epoxy composites." Davim, J. Paulo (ed.) Ecotribology, research developments. Switzerland. Springer. pp. 145-172Optimization of performance and exhaust emission parameters of a SI (spark ignition) engine with gasoline-ethanol blended fuels using response surface methodology
Najafi, Gholamhassan, Ghobadian, Barat, Yusaf, Talal, Ardebili, Seyed Mohammad Safieddin and Mamat, Rizalman. 2015. "Optimization of performance and exhaust emission parameters of a SI (spark ignition) engine with gasoline-ethanol blended fuels using response surface methodology." Energy. 90 (2), pp. 1815-1829. https://doi.org/10.1016/j.energy.2015.07.004Solar energy in Iran: current state and outlook
Najafi, G., Ghobadian, B., Mamat, R., Yusaf, T. and Azmi, W. H.. 2015. "Solar energy in Iran: current state and outlook." Renewable and Sustainable Energy Reviews. 49, pp. 931-942. https://doi.org/10.1016/j.rser.2015.04.056An experimental study on reactivity controlled compression ignition engine fueled with biodiesel/natural gas
Gharehghani, Ayatallah, Hosseini, Reza, Mirsalim, Mostafa, Jazayeri, S. Ali and Yusaf, Talal. 2015. "An experimental study on reactivity controlled compression ignition engine fueled with biodiesel/natural gas." Energy. 89, pp. 558-567. https://doi.org/10.1016/j.energy.2015.06.014A comparative study on the first and second law analysis and performance characteristics of a spark ignition engine using either natural gas or gasoline
Gharehghani, A., Hosseini, R., Mirsalim, M. and Yusaf, Talal F.. 2015. "A comparative study on the first and second law analysis and performance characteristics of a spark ignition engine using either natural gas or gasoline." Fuel: the science and technology of fuel and energy. 158, pp. 488-493. https://doi.org/10.1016/j.fuel.2015.05.067Convergence in synchronous measurements
Leis, John and Buttsworth, David. 2015. "Convergence in synchronous measurements." Wysocki, Beata J. and Wysocki, Tadeusz A. (ed.) 2014 8th International Conference on Signal Processing and Communication Systems (ICSPCS 2014). Gold Coast, Australia 15 - 17 Dec 2014 United States. https://doi.org/10.1109/ICSPCS.2014.7021090Influence of metal coating on sorghum milling process subjected to three body abrasion
Al-Sandooq, Jaafar Mahdi, Yousif, Belal and Jensen, T. A.. 2015. "Influence of metal coating on sorghum milling process subjected to three body abrasion." International Journal of Precision Technology. 5 (1), pp. 27-42. https://doi.org/10.1504/IJPTECH.2015.069972Effect of mixing on the performance of wet steam ejectors
Ariafar, Kavous, Buttsworth, David, Al-Doori, Ghassan and Malpress, Ray. 2015. "Effect of mixing on the performance of wet steam ejectors." Energy. 93 (2), pp. 2030-2041. https://doi.org/10.1016/j.energy.2015.10.082Editor's introduction to special theme issue [of International Journal of Precision Technology]: Precision engineering and tribology
Yousif, Belal. 2015. "Editor's introduction to special theme issue [of International Journal of Precision Technology]: Precision engineering and tribology." International Journal of Precision Technology. 5 (1).Exploring the correlations between common UV measurements and chemical fractionation for natural waters
Al-Juboori, Raed A., Yusaf, Talal and Pittaway, Pamela A.. 2016. "Exploring the correlations between common UV measurements and chemical fractionation for natural waters ." Desalination and Water Treatment. 57 (35), pp. 16324-16335. https://doi.org/10.1080/19443994.2015.1079805A computational study of operating range extension in a natural gas SI engine with the use of hydrogen
Gharehghani, A., Hosseini, R., Mirsalim, M. and Yusaf, Talal F.. 2015. "A computational study of operating range extension in a natural gas SI engine with the use of hydrogen." International Journal of Hydrogen Energy. 40 (17), pp. 5966-5975. https://doi.org/10.1016/j.ijhydene.2015.03.015A comparison of household carbon emission patterns of urban and rural China over the 17 year period (1995–2011)
Qu, Jiansheng, Maraseni, Tek, Liu, Lina, Zhang, Zhiqiang and Yusaf, Talal. 2015. "A comparison of household carbon emission patterns of urban and rural China over the 17 year period (1995–2011)." Energies. 8 (9), pp. 10537-10557. https://doi.org/10.3390/en80910537Impact of pulsed ultrasound on bacteria reduction of natural waters
Al-Juboori, Raed A., Aravinthan, Vasantha and Yusaf, Talal. 2015. "Impact of pulsed ultrasound on bacteria reduction of natural waters." Ultrasonics Sonochemistry. 27, pp. 137-147. https://doi.org/10.1016/j.ultsonch.2015.05.007The influence of straight vegetable oil fatty acid composition on compression ignition combustion and emissions
Hellier, Paul, Ladommatos, Nicos and Yusaf, Talal. 2015. "The influence of straight vegetable oil fatty acid composition on compression ignition combustion and emissions." Fuel: the science and technology of fuel and energy. 143, pp. 131-143. https://doi.org/10.1016/j.fuel.2014.11.021Assessing the application and downstream effects of pulsed mode ultrasound as a pre-treatment for alum coagulation
Al-Juboori, Raed A., Aravinthan, Vasantha, Yusaf, Talal and Bowtell, Leslie. 2016. "Assessing the application and downstream effects of pulsed mode ultrasound as a pre-treatment for alum coagulation." Ultrasonics Sonochemistry. 31, pp. 7-19. https://doi.org/10.1016/j.ultsonch.2015.11.028Experimental and Analytical Investigation of Hypersonic Fluid-Structure-Interactions on a Pitching Flat Plate Airfoil
Yamada, Kenji, Jahn, Ingo and Buttsworth, David. 2016. "Experimental and Analytical Investigation of Hypersonic Fluid-Structure-Interactions on a Pitching Flat Plate Airfoil." Applied Mechanics and Materials. 846, pp. 157-162. https://doi.org/10.4028/www.scientific.net/AMM.846.157Evaluating the impact of operating parameters on biocidal effects of pulsed ultrasound in natural water disinfection
Al Juboori, Raed A., Yusaf, Talal and Aravinthan, Vasantha. 2015. "Evaluating the impact of operating parameters on biocidal effects of pulsed ultrasound in natural water disinfection." Journal of Biotechnology. 208 (Supplement), pp. S17-S18. https://doi.org/10.1016/j.jbiotec.2015.06.042Brass, aluminium, and mild steel sliding against stainless steel under dry conditions
Alotaibi, J. G., Yousif, B. F. and Yusaf, T. F.. 2015. "Brass, aluminium, and mild steel sliding against stainless steel under dry conditions." International Journal of Precision Technology. 5 (2), pp. 85-96.Multimedia resources in engineering
Yousif, B. F., Basson, Marita and Hobohm, Carola. 2014. "Multimedia resources in engineering." Davim, J. Paulo (ed.) Modern mechanical engineering: research, development and education. Heidelberg, Germany. Springer. pp. 449-461Wear behaviour and mechanism of different metals sliding against stainless steel counterface
Alotaibi, J. G., Yousif, B. F. and Yusaf, T. F.. 2014. "Wear behaviour and mechanism of different metals sliding against stainless steel counterface." Proceedings of the Institution of Mechanical Engineers Part J: Journal of Engineering Tribology. 228 (6), pp. 692-704. https://doi.org/10.1177/1350650114527072Effect of SiC addition on mechanical and wear characteristics of WC-32(W-Ti)C-6Co cemented carbides
Albdiry, M. T. and Yousif, B. F.. 2014. "Effect of SiC addition on mechanical and wear characteristics of WC-32(W-Ti)C-6Co cemented carbides." International Journal of Precision Technology. 4 (1/2), pp. 110-121. https://doi.org/10.1504/IJPTECH.2014.060611Evaluating the effect of heat transfer on cell disruption in ultrasound processes
Yusaf, Talal. 2015. "Evaluating the effect of heat transfer on cell disruption in ultrasound processes." Annals of Microbiology. 65 (3), pp. 1447-1456. https://doi.org/10.1007/s13213-014-0983-zRole of silanized halloysite nanotubes on structural, mechanical properties and fracture toughness of thermoset nanocomposites
Albdiry, M. T. and Yousif, B. F.. 2014. "Role of silanized halloysite nanotubes on structural, mechanical properties and fracture toughness of thermoset nanocomposites." Materials and Design. 57, pp. 279-288. https://doi.org/10.1016/j.matdes.2013.12.017Engine performance and emissions using Jatropha curcas, Ceiba pentandra and Calophyllum inophyllum biodiesel in a CI diesel engine
Ong, Hwai Chyuan, Masjuki, H. H., Mahlia, T. M. I., Silitonga, A. S., Chong, W. T. and Yusaf, Talal. 2014. "Engine performance and emissions using Jatropha curcas, Ceiba pentandra and Calophyllum inophyllum biodiesel in a CI diesel engine." Energy. 69, pp. 427-445. https://doi.org/10.1016/j.energy.2014.03.035Growing algae using water from coal seam gas industry and harvesting using an innovative technique: a review and a potential
Hamawand, Ihsan, Yusaf, Talal and Hamawand, Sara. 2014. "Growing algae using water from coal seam gas industry and harvesting using an innovative technique: a review and a potential." Fuel: the science and technology of fuel and energy. 117 (Part A), pp. 422-430. https://doi.org/10.1016/j.fuel.2013.09.040An introduction to a homogeneous charge compression ignition engine
Hairuddin, A. A., Wandel, A. P. and Yusaf, T.. 2014. "An introduction to a homogeneous charge compression ignition engine." Journal of Mechanical Engineering and Sciences. 7, pp. 1042-1052. https://doi.org/10.15282/jmes.7.2014.3.0101Investigating the feasibility and the optimal location of pulsed ultrasound in surface water treatment schemes
Al-Juboori, Raed A., Yusaf, Talal, Aravinthan, Vasantha, Pittaway, Pamela A. and Bowtell, Leslie. 2016. "Investigating the feasibility and the optimal location of pulsed ultrasound in surface water treatment schemes." Desalination and Water Treatment. 57 (11), pp. 4769-4787. https://doi.org/10.1080/19443994.2014.996771Detection of potentially explosive methane levels using a solid-state infrared source
Leis, John, Buttsworth, David, Snook, Chris and Holmes, Graham. 2014. "Detection of potentially explosive methane levels using a solid-state infrared source." IEEE Transactions on Instrumentation and Measurement. 63 (12), pp. 3088-3095. https://doi.org/10.1109/TIM.2014.2327457Investigating corrosion effects and heat transfer enhancement in smaller size radiators using CNT-nanofluids
Rashmi, W., Ismail, A. F., Khalid, M., Anuar, A. and Yusaf, T.. 2014. "Investigating corrosion effects and heat transfer enhancement in smaller size radiators using CNT-nanofluids." Journal of Materials Science. 49 (13), pp. 4544-4551. https://doi.org/10.1007/s10853-014-8154-yStatistical diagnosis of the best weibull methods for wind power assessment for agricultural applications
Azad, Abul Kalam, Rasul, Mohammad Golam and Yusaf, Talal. 2014. "Statistical diagnosis of the best weibull methods for wind power assessment for agricultural applications." Energies. 7 (5), pp. 3056-3085. https://doi.org/10.3390/en7053056Ejector primary nozzle steam condensation: area ratio effects and mixing layer development
Ariafar, Kavous, Buttsworth, David, Sharifi, Navid and Malpress, Ray. 2014. "Ejector primary nozzle steam condensation: area ratio effects and mixing layer development ." Applied Thermal Engineering. 71 (1), pp. 519-527. https://doi.org/10.1016/j.applthermaleng.2014.06.038Influence of date palm fibre and graphite filler on mechanical and wear characteristics of epoxy composites
Shalwan, A. and Yousif, B. F.. 2014. "Influence of date palm fibre and graphite filler on mechanical and wear characteristics of epoxy composites." Materials and Design. 59, pp. 264-273. https://doi.org/10.1016/j.matdes.2014.02.066A review of hydrogen and natural gas addition in diesel HCCI engines
Hairuddin, A. Aziz, Yusaf, Talal and Wandel, Andrew P.. 2014. "A review of hydrogen and natural gas addition in diesel HCCI engines." Renewable and Sustainable Energy Reviews. 32, pp. 739-761. https://doi.org/10.1016/j.rser.2014.01.018Hot-wall reentry testing in hypersonic impulse facilities
Zander, F., Morgan, R. G., Sheikh, U., Buttsworth, D. R. and Teakle, P. R.. 2013. "Hot-wall reentry testing in hypersonic impulse facilities." AIAA Journal: devoted to aerospace research and development. 51 (2), pp. 476-484. https://doi.org/10.2514/1.J051867Hypersonic wind-tunnel free-flying experiments with onboard instrumentation
Mudford, Neil R., O'Byrne, Sean, Neely, Andrew, Buttsworth, David and Balage, Sudantha. 2015. "Hypersonic wind-tunnel free-flying experiments with onboard instrumentation." Journal of Spacecraft and Rockets. 52 (1), pp. 231-242. https://doi.org/10.2514/1.A32887Evolution of plasma species and heat – shield temperatures from Hayabusa Reentry Observation
Kraetzig, Benjamin, Lohle, Stefan and Buttsworth, David. 2014. "Evolution of plasma species and heat – shield temperatures from Hayabusa Reentry Observation." Journal of Spacecraft and Rockets. 51 (3), pp. 823-837. https://doi.org/10.2514/1.A32854Visible and near infrared spectroscopy of Hayabusa Reentry using semi-autonomous tracking
McIntyre, Timothy, Khan, Razmi, Eichmann, Troy, Upcroft, Ben and Buttsworth, David. 2014. "Visible and near infrared spectroscopy of Hayabusa Reentry using semi-autonomous tracking." Journal of Spacecraft and Rockets. 51 (1), pp. 31-38. https://doi.org/10.2514/1.A32497Energy characterisation of ultrasonic systems for industrial processes
Al-Juboori, Raed A., Yusaf, Talal, Bowtell, Leslie and Aravinthan, Vasantha. 2015. "Energy characterisation of ultrasonic systems for industrial processes." Ultrasonics. 57, pp. 18-30. https://doi.org/10.1016/j.ultras.2014.10.003Instantaneous heat flux simulation of a motored reciprocating engine: unsteady thermal boundary layer with variable turbulent thermal conductivity
Agrira, Abdalla, Buttsworth, David R. and Said, Mior A.. 2014. "Instantaneous heat flux simulation of a motored reciprocating engine: unsteady thermal boundary layer with variable turbulent thermal conductivity." Journal of Heat Transfer: Transactions of the ASME. 136 (3), pp. 031703 1-031703 9. https://doi.org/10.1115/1.4025639Coals seam gas (CSG) in agriculture - a review: technical and market analysis for Australia
Yusaf, Talal, Hamawand, Ihsan, Schmidt, Erik, Binnie, James, Rees, Steven and Chakrabarty, Sayan. 2014. "Coals seam gas (CSG) in agriculture - a review: technical and market analysis for Australia ." Sustainable Energy Technologies and Assessments. 8, pp. 149-158. https://doi.org/10.1016/j.seta.2014.08.005Pitot pressure measurements in a supersonic steam jet
Al-Doori, Ghassan and Buttsworth, David R.. 2014. "Pitot pressure measurements in a supersonic steam jet." Experimental Thermal and Fluid Science. 58, pp. 56-61. https://doi.org/10.1016/j.expthermflusci.2014.05.012Kenaf fibers for tribo-thermoplastic composites
Singh, Narish, Rilling, Dirk and Yousif, B. F.. 2010. "Kenaf fibers for tribo-thermoplastic composites." Ghia, Urmila (ed.) 2009 ASME International Mechanical Engineering Congress and Exposition (IMECE 2009). Florida, United States of America 13 - 19 Nov 2009 United States. https://doi.org/10.1115/IMECE2009-10167New technique measuring film thickness for tribological machines
Al-Haddad, A. A., Salih, Nbhan D., Wen, Chin Chee, Yusaf, T. F. and Yousif, B. F.. 2010. "New technique measuring film thickness for tribological machines." 2009 ASME International Mechanical Engineering Congress and Exposition (IMECE 2009). Florida, United States of America 13 - 19 Nov 2009 United States. https://doi.org/10.1115/IMECE2009-10160Air fuel ratio study for mixture of biogas and hydrogen on mild combustion
Noor, M. M., Wandel, Andrew P. and Yusaf, Talal. 2014. "Air fuel ratio study for mixture of biogas and hydrogen on mild combustion." International Journal of Automotive and Mechanical Engineering. 10 (1), pp. 2144-2154. https://doi.org/10.15282/ijame.9.2013.29.0180Effect of air-fuel ratio on temperature distribution and pollutants for biogas MILD combustion
Noor, M. M., Wandel, Andrew P. and Yusaf, Talal. 2014. "Effect of air-fuel ratio on temperature distribution and pollutants for biogas MILD combustion." International Journal of Automotive and Mechanical Engineering. 10 (1), pp. 1980-1992. https://doi.org/10.15282/ijame.10.2014.15.0166The simulation of biogas combustion in a MILD burner
Noor, M. M., Wandel, Andrew P. and Yusaf, Talal. 2014. "The simulation of biogas combustion in a MILD burner." Journal of Mechanical Engineering and Sciences. 6 (1), pp. 995-1013.Design of film thickness instrument for fibre polymer composites tribological experiments
Yousif, B. F., Al-Haddad, A., Alotaibi, J. GH. and Yusaf, T. F.. 2014. "Design of film thickness instrument for fibre polymer composites tribological experiments." Journal of Engineering Science and Technology. 9 (2), pp. 154-166.Isokinetic total water content probe in a naturally aspirating configuration: initial aerodynamic design and testing
Davison, Craig, MacLeod, James D., Strapp, J. Walter and Buttsworth, David R.. 2008. "Isokinetic total water content probe in a naturally aspirating configuration: initial aerodynamic design and testing ." 46th AIAA Aerospace Sciences Meeting and Exhibit (AIAA 2008). Reno, United States 07 - 10 Jan 2008 Reston, VA. United States. https://doi.org/10.2514/6.2008-435Stagnation temperature in a cold hypersonic flow produced by a light free piston compression facility
Widodo, Agung and Buttsworth, David. 2013. "Stagnation temperature in a cold hypersonic flow produced by a light free piston compression facility." Experiments in Fluids: experimental methods and their applications to fluid flow. 54 (4), p. 1486. https://doi.org/10.1007/s00348-013-1486-6High-stress three-body abrasive wear of treated and untreated oil palm fibre-reinforced polyester composites
Yousif, B. F. and El-Tayeb, N. S. M.. 2008. "High-stress three-body abrasive wear of treated and untreated oil palm fibre-reinforced polyester composites." Proceedings of the Institution of Mechanical Engineers Part J: Journal of Engineering Tribology. 222 (5), pp. 637-646. https://doi.org/10.1243/13506501JET412On the effect of roller materials on the power window mechanism from a tribological perspective
Chee, S. B., Shalabi, Ammar Al, Chin, C. W. and Yousif, B. F.. 2009. "On the effect of roller materials on the power window mechanism from a tribological perspective." 2008 ASME International Mechanical Engineering Congress and Exposition (IMECE 2008). Boston, United States of America 31 Oct - 06 Nov 2008 New York, NY. United States. https://doi.org/10.1115/IMECE2008-66112On the mechanical properties of a treated betelnut fibre-reinforced polyester composite
Yousif, B. F. and Nirmal, U.. 2010. "On the mechanical properties of a treated betelnut fibre-reinforced polyester composite." Proceedings of the Institution of Mechanical Engineers Part C: Journal of Mechanical Engineering Science. 224 (9), pp. 1805-1814. https://doi.org/10.1243/09544062JMES1943Framework for orienting engineering undergraduate final year projects towards new product innovation process
Al-Shalabi, Ammar, Chee, S. B., Singh, Narish and Yousif, B. F.. 2008. "Framework for orienting engineering undergraduate final year projects towards new product innovation process." Soliman, Khalid S. (ed.) 10th Conference on Innovation and Knowledge Management in Business Globalization (IBIMA 2008). Kuala Lumpur, Malaysia 30 Jun - 01 Jul 2008 Norristown, PA. United States.The effect of treatment on tribo-performance of CFRP composites
Yousif, Belal F., Leong, Ong B., Ong, Low K. and Jye, Wong K.. 2009. "The effect of treatment on tribo-performance of CFRP composites." Recent Patents on Materials Science. 2 (1), pp. 67-74. https://doi.org/10.2174/1874464810902010067Wear and friction characteristics of CGRP composite under wet contact condition using two different test techniques
Yousif, B. F. and El-Tayeb, N. S. M.. 2008. "Wear and friction characteristics of CGRP composite under wet contact condition using two different test techniques." Wear. 265 (5-6), pp. 856-864. https://doi.org/10.1016/j.wear.2008.01.016Machinability of glass/date palm fibre epoxy composites
Alsaeed, T. and Yousif, B. F.. 2014. "Machinability of glass/date palm fibre epoxy composites." International Journal of Machining and Machinability of Materials. 16 (2), pp. 129-150. https://doi.org/10.1504/IJMMM.2014.064685An experimental investigation of the effective parameters on wet washing of biodiesel purification
Abbaszadeh, A., Ghobadian, B., Najafi, G. and Yusaf, T.. 2014. "An experimental investigation of the effective parameters on wet washing of biodiesel purification." International Journal of Automotive and Mechanical Engineering. 9 (1), pp. 1525-1537. https://doi.org/10.15282/ijame.9.2013.4.0126Smaller radiator with enhanced heat transfer using CNT-nanofluids
Ismail, A. F., Abd-Halim, B. A., Rashmi, W. and Yusaf, T.. 2014. "Smaller radiator with enhanced heat transfer using CNT-nanofluids ." Yang, G. (ed.) International Conference on Future Energy, Environment, and Materials (FEEM 2013). Hong Kong, China 24 - 25 Dec 2013 Southampton, United Kingdom. https://doi.org/10.2495/FEEM20130221Chlorella protothecoides microalgae as an alternative fuel for tractor diesel engines
Al-lwayzy, Saddam H. and Yusaf, Talal. 2013. "Chlorella protothecoides microalgae as an alternative fuel for tractor diesel engines." Energies. 6 (2), pp. 766-783. https://doi.org/10.3390/en6020766Correlation between frictional force, interface temperature and specific wear rate of fibre polymer composites
Arhaim, Y. H, Shalwan, A. and Yousif, B. F.. 2013. "Correlation between frictional force, interface temperature and specific wear rate of fibre polymer composites." Advanced Materials Research. 685 (685), pp. 45-49. https://doi.org/10.4028/www.scientific.net/AMR.685.45Hot wall testing methodology for impulse facilities
Zander, F., Morgan, R. G., Sheikh, U. A., Buttsworth, D. R. and Teakle, P. R.. 2013. "Hot wall testing methodology for impulse facilities." Stallings, David (ed.) 18th AIAA/3AF International Space Planes and Hypersonic Systems and Technologies Conference (AIAA 2012). Tours, France 24 - 28 Sep 2012 Reston, VA. United States. https://doi.org/10.2514/6.2012-5953In state of art: mechanical and tribological behaviour of polymeric composites based on natural fibres
Shalwan, A. and Yousif, B. F.. 2013. "In state of art: mechanical and tribological behaviour of polymeric composites based on natural fibres." Materials and Design. 48, pp. 14-24. https://doi.org/10.1016/j.matdes.2012.07.014Coal seam gas and associated water: a review paper
Hamawand, Ihsan, Yusaf, Talal and Hamawand, Sara. 2013. "Coal seam gas and associated water: a review paper." Renewable and Sustainable Energy Reviews. 22, pp. 550-560. https://doi.org/10.1016/j.rser.2013.02.030Near-ultraviolet emission spectroscopy of the Hayabusa reentry
Buttsworth, David, Morgan, Richard and Jenniskens, Peter. 2013. "Near-ultraviolet emission spectroscopy of the Hayabusa reentry." Journal of Spacecraft and Rockets. 50 (6), pp. 1109-1120. https://doi.org/10.2514/1.A32500Study and modelling drying of banana slices under superheated steam
Hamawand, Ihsan, Yusaf, Talal and Bennett, John. 2014. "Study and modelling drying of banana slices under superheated steam." Asia Pacific Journal of Chemical Engineering. 9 (4), pp. 591-603. https://doi.org/10.1002/apj.1788Biofuels from the fresh water microalgae Chlorella vulgaris (FWM-CV) for diesel engines
Al-lwayzy, Saddam H., Yusaf, Talal and Al-Juboori, Raed A.. 2014. "Biofuels from the fresh water microalgae Chlorella vulgaris (FWM-CV) for diesel engines." Energies. 7 (3), pp. 1829-1851. https://doi.org/10.3390/en7031829Fracture toughness and toughening mechanisms of unsaturated polyester-based clay nanocomposites
Albdiry, Mushtaq T., Yousif, Belal F. and Ku, Harry. 2013. "Fracture toughness and toughening mechanisms of unsaturated polyester-based clay nanocomposites." 13th International Conference on Fracture (ICF 2013). Beijing, China 16 - 21 Jun 2013 Anhui, China.Assessing a new approach to covered anaerobic pond design in the treatment of abattoir wastewater
McCabe, B. K., Harris, P., Baillie, C., Pittaway, P. and Yusaf, T.. 2013. "Assessing a new approach to covered anaerobic pond design in the treatment of abattoir wastewater." Australian Journal of Multi-Disciplinary Engineering. 10 (1), pp. 81-93. https://doi.org/10.7158/N12-AE07.2013.10.1.MILD combustion: the future for lean and clean combustion technology
Noor, M. M., Wandel, Andrew P. and Yusaf, Talal. 2014. "MILD combustion: the future for lean and clean combustion technology." International Review of Mechanical Engineering. 8 (1), pp. 251-257.Design and development of mild combustion burner
Noor, M. M., Wandel, Andrew P. and Yusaf, Talal. 2013. "Design and development of mild combustion burner." Journal of Mechanical Engineering and Sciences. 5, pp. 662-676. https://doi.org/10.15282/jmes.5.2013.13.0064A review on the degradability of polymeric composites based on natural fibres
Azwa, Z. N., Yousif, B. F., Manalo, A. C. and Karunasena, W.. 2013. "A review on the degradability of polymeric composites based on natural fibres." Materials and Design. 47, pp. 424-442. https://doi.org/10.1016/j.matdes.2012.11.025Effect of different heat transfer models on a diesel homogeneous charge compression ignition engine
Hairuddin, Abdul Aziz, Yusaf, Talal and Wandel, Andrew. 2013. "Effect of different heat transfer models on a diesel homogeneous charge compression ignition engine." International Journal of Automotive and Mechanical Engineering. 8 (1), pp. 1292-1304.Implementation of CMC in a 0-D single-zone model of a diesel HCCI engine
Hairuddin, A. Aziz, Yusaf, Talal and Wandel, Andrew P.. 2013. "Implementation of CMC in a 0-D single-zone model of a diesel HCCI engine." Zhu, Mingming, Yu, Yun, Vuthaluru, Hari, Zhang, Zhezi and Zhang, Dongke (ed.) 7th Australian Combustion Symposium (ACS 2013). Perth, Australia 06 - 08 Nov 2013 Sydney, Australia.Performance analysis of journal bearings using ultrasonic reflection
Kasolang, S., Ahmed, Diyar I., Dwyer-Joyce, R. S. and Yousif, B. F.. 2013. "Performance analysis of journal bearings using ultrasonic reflection." Tribology International. 64, pp. 78-84. https://doi.org/10.1016/j.triboint.2013.02.012Experimental study of microorganism disruption using shear stress
Yusaf, Talal. 2013. "Experimental study of microorganism disruption using shear stress." Biochemical Engineering Journal. 79, pp. 7-14. https://doi.org/10.1016/j.bej.2013.07.001The effect of methanol-diesel blended ratio on CI engine performance
Yusaf, T., Hamawand, I., Baker, P. and Najafi, G.. 2013. "The effect of methanol-diesel blended ratio on CI engine performance." International Journal of Automotive and Mechanical Engineering. 8 (1), pp. 1385-1395. https://doi.org/10.15282/ijame.8.2013.26.0114Effect of compressed natural gas mixing on the engine performance and emissions
Yusaf, T., Baker, P., Hamawand, I. and Noor, M. M.. 2013. "Effect of compressed natural gas mixing on the engine performance and emissions." International Journal of Automotive and Mechanical Engineering. 8 (1), pp. 1416-1429.Analysis of recirculation zone and ignition position of non-premixed bluff-body for biogas mild combustion
Noor, M. M., Wandel, Andrew P. and Yusaf, Talal. 2013. "Analysis of recirculation zone and ignition position of non-premixed bluff-body for biogas mild combustion." International Journal of Automotive and Mechanical Engineering. 8 (1), pp. 1176-1186.The analysis of recirculation zone and ignition position of non-premixed bluff-body for biogas mild combustion
Noor, M. M., Wandel, Andrew P. and Yusaf, Talal. 2013. "The analysis of recirculation zone and ignition position of non-premixed bluff-body for biogas mild combustion." Ishak, Mahadzir and Rahman, Md. Mustafizur (ed.) 2nd International Conference of Mechanical Engineering Research (ICMER 2013). Pahang, Malaysia 01 - 03 Jul 2013 Pahang, Malaysia.Sustainable composites
Yousif, Belal F., Islam, Md Mainul and Salit, Mohd Sapuan. 2013. "Sustainable composites." Advances in Materials Science and Engineering. https://doi.org/10.1155/2013/142718Particles motion in a cascading rotary drum dryer
Hamawand, Ihsan and Yusaf, Talal. 2014. "Particles motion in a cascading rotary drum dryer." Canadian Journal of Chemical Engineering. 92 (4), pp. 648-662. https://doi.org/10.1002/cjce.21845Impact fracture behaviour of silane-treated halloysite nanotubes-reinforced unsaturated polyester
Albdiry, M. T., Ku, H. and Yousif, B. F.. 2013. "Impact fracture behaviour of silane-treated halloysite nanotubes-reinforced unsaturated polyester." Engineering Failure Analysis. 35, pp. 718-725. https://doi.org/10.1016/j.engfailanal.2013.06.027Experimental investigation of thermal balance of a turbocharged SI engine operating on natural gas
Gharehghani, A., Koochak, M., Mirsalim, M. and Yusaf, Talal. 2013. "Experimental investigation of thermal balance of a turbocharged SI engine operating on natural gas." Applied Thermal Engineering. 60 (1-2), pp. 200-207. https://doi.org/10.1016/j.applthermaleng.2013.06.029Experimental investigation of the tractor engine performance using diesohol fuel
Shadidi, Behdad, Yusaf, Talal, Alizadeh, Hossein Haji Agha and Ghobadian, Barat. 2014. "Experimental investigation of the tractor engine performance using diesohol fuel." Applied Energy. 114, pp. 874-879. https://doi.org/10.1016/j.apenergy.2013.06.011Characteristics of kenaf fibre/epoxy composites subjected to thermal degradation
Azwa, Z. N. and Yousif, B. F.. 2013. "Characteristics of kenaf fibre/epoxy composites subjected to thermal degradation." Polymer Degradation and Stability. 98 (12), pp. 2752-2759. https://doi.org/10.1016/j.polymdegradstab.2013.10.008Nutrient removal of nursery and municipal wastewater using Chlorella vulgaris microalgae for lipid extraction
Aravinthan, Vasantha, Story, Nadine and Yusaf, Talal. 2014. "Nutrient removal of nursery and municipal wastewater using Chlorella vulgaris microalgae for lipid extraction." Desalination and Water Treatment. 52 (4-6), pp. 727-736. https://doi.org/10.1080/19443994.2013.826325Recycling of waste engine oils using a new washing agent
Hamawand, Ihsan, Yusaf, Talal and Rafat, Sardasht. 2013. "Recycling of waste engine oils using a new washing agent." Energies. 6 (2), pp. 1023-1049. https://doi.org/10.3390/en6021023Production of biodiesel from Sterculia foetida and its process optimization
Silitonga, A. S., Ong, H. C., Masjuki, H. H., Mahlia, T. M. I., Chong, W. T. and Yusaf, Talal F.. 2013. "Production of biodiesel from Sterculia foetida and its process optimization ." Fuel: the science and technology of fuel and energy. 111, pp. 478-484. https://doi.org/10.1016/j.fuel.2013.03.051Numerical study of oxygen dilution and temperature distribution of biogas combustion in Bluff-body MILD burner
Noor, M. M., Wandel, Andrew P. and Yusaf, Talal. 2013. "Numerical study of oxygen dilution and temperature distribution of biogas combustion in Bluff-body MILD burner." Masri, Assaad (ed.) 7th Australian Combustion Symposium (ACS 2013). Perth, Australia 06 - 08 Nov 2013 Perth, Australia.A case study for biogas generation from covered anaerobic ponds treating abattoir wastewater: investigation of pond performance and potential biogas production
McCabe, Bernadette K., Hamawand, Ihsan, Harris, Peter, Baillie, Craig and Yusaf, Talal. 2014. "A case study for biogas generation from covered anaerobic ponds treating abattoir wastewater: investigation of pond performance and potential biogas production." Applied Energy. 114, pp. 798-808. https://doi.org/10.1016/j.apenergy.2013.10.020Alternative methods of microorganism disruption for agricultural applications
Yusaf, Talal and Al-Juboori, Raed A.. 2014. "Alternative methods of microorganism disruption for agricultural applications ." Applied Energy. 114, pp. 909-923. https://doi.org/10.1016/j.apenergy.2013.08.085Fuzzy logic based model to predict maximum oil-film pressure in journal bearing
Ahmed, Diyar I., Kasolang, S., Khidhir, Basim A. and Yousif, B. F.. 2013. "Fuzzy logic based model to predict maximum oil-film pressure in journal bearing." Research Journal of Applied Sciences, Engineering and Technology. 6 (20), pp. 3871-3878.Thermal degredation study of kenaf fibre/epoxy composites using thermo gravimetric analysis
Azwa, Z. N. and Yousif, B. F.. 2013. "Thermal degredation study of kenaf fibre/epoxy composites using thermo gravimetric analysis." 3rd Malaysian Postgraduate Conference (MPC 2013). Sydney, Australia 04 - 05 Jul 2013 Sydney, Australia.The air fuel ratio study for the mixture of biogas and hydrogen on mild combustion
Noor, M. M., Wandel, Andrew P. and Yusaf, Talal. 2013. "The air fuel ratio study for the mixture of biogas and hydrogen on mild combustion." 3rd Malaysian Postgraduate Conference (MPC 2013). Sydney, Australia 04 - 05 Jul 2013 Sydney, Australia.Discretization of three dimensional non-uniform grid: conditional moment closure elliptic equation using finite difference method
Noor, M. M., Wandel, Andrew P. and Yusaf, Talal. 2013. "Discretization of three dimensional non-uniform grid: conditional moment closure elliptic equation using finite difference method." 3rd Malaysian Postgraduate Conference (MPC 2013). Sydney, Australia 04 - 05 Jul 2013 Sydney, Australia.A homogeneous charge compression ignition engine: an introduction
Hairuddin, A. Aziz, Wandel, Andrew P. and Yusaf, Talal. 2013. "A homogeneous charge compression ignition engine: an introduction." 3rd Malaysian Postgraduate Conference (MPC 2013). Sydney, Australia 04 - 05 Jul 2013 Sydney, Australia.Radiometric temperature analysis of the Hayabusa spacecraft re-entry
Eichmann, T. N., Khan, R., McIntyre, T. J., Jacobs, C., Porat, H., Buttsworth, D. and Upcroft, B.. 2012. "Radiometric temperature analysis of the Hayabusa spacecraft re-entry." Kontis, Konstantinos (ed.) 28th International Symposium on Shock Waves (ISSW 28). Manchester, United Kingdom 17 - 22 Jul 2011 Heidelberg, Germany. https://doi.org/10.1007/978-3-642-25688-2_77Adhesive wear characteristics of natural fiber-reinforced composites
Yousif, Belal F.. 2012. "Adhesive wear characteristics of natural fiber-reinforced composites." Davim, J. Paulo (ed.) Wear of advanced materials. London, United Kingdom. John Wiley & Sons. pp. 61-97Application of photogrammetry at USQ hypersonic wind tunnel
Chong, Albert K., Buttsworth, David, Mudford, Neil, Jokic, Michael, Balage, Sudantha and O'Bryne, Sean. 2012. "Application of photogrammetry at USQ hypersonic wind tunnel ." Short, Wayne and Cairns, Iver (ed.) 11th Australian Space Science Conference (ASSC 2011). Canberra, Australia 26 - 29 Sep 2011 Sydney, Australia.The potential of using date palm fibres as reinforcement for polymeric composites
Alsaeed, T., Yousif, B. F. and Ku, H.. 2013. "The potential of using date palm fibres as reinforcement for polymeric composites." Materials and Design. 43, pp. 177-184. https://doi.org/10.1016/j.matdes.2012.06.061Optically addressed pressure sensors for transient gas dynamics: calibration of a preliminary design
Sharifian, S. A. and Buttsworth, D. R.. 2001. "Optically addressed pressure sensors for transient gas dynamics: calibration of a preliminary design." Dally, B. B. (ed.) 14th Australasian Fluid Mechanics Conference (AFMC 2001) . Adelaide, South Australia 09 - 14 Dec 2001 Adelaide, South Australia.Reducing the mechanical hysteresis problem in optically-addressed diaphragm pressure sensors
Sharifian, S. A. and Buttsworth, D. R.. 2002. "Reducing the mechanical hysteresis problem in optically-addressed diaphragm pressure sensors." Tomasini, Enrico (ed.) SPIE 2002: Vibration Measurements by Laser Techniques: Advances and Applications. Ancona, Italy 18 - 21 Jun 2002 Washington, USA. https://doi.org/10.1117/12.468141Tensile properties of bagasse reinforced epoxy composites
Trada, M., Mandava, J., Ku, H. and Yousif, B.. 2012. "Tensile properties of bagasse reinforced epoxy composites." Jaafar, M., Azura, A. R., Leong, K. H. and Leong, A. Y. L. (ed.) 8th Asian-Australasian Conference on Composite Materials (ACCM 2012): Composites: Enabling Tomorrow's Industry Today. Kuala Lumpur, Malaysia 06 - 08 Nov 2012 Kowloon, Hong Kong.Sampling, quantization and computational aspects of the quadrature lock-in amplifier
Leis, John, Kelly, Christopher and Buttsworth, David. 2012. "Sampling, quantization and computational aspects of the quadrature lock-in amplifier." Wysocki, Beata J. and Wysocki, Tadeusz (ed.) 2012 6th International Conference on Signal Processing and Communication Systems (ICSPCS 2012). Gold Coast, Australia 12 - 14 Dec 2012 United States. IEEE (Institute of Electrical and Electronics Engineers). https://doi.org/10.1109/ICSPCS.2012.6507974Implementing multimedia resources in online learning and its effect on student understanding
Yousif, B. F., Basson, Marita and Hobohm, Carola. 2012. "Implementing multimedia resources in online learning and its effect on student understanding." Mann, Llewellyn and Daniel, Scott (ed.) 23rd Annual Conference of the Australasian Association for Engineering Education (AAEE 2012). Melbourne, Australia 03 - 05 Dec 2012 Melbourne, Australia.Near ultraviolet emission spectroscopy of the Hayabusa re-entry
Buttsworth, David, Jacobs, P., Morgan, R. G. and Jenniskens, Peter. 2012. "Near ultraviolet emission spectroscopy of the Hayabusa re-entry ." 50th AIAA Aerospace Sciences Meeting Including the New Horizons Forum and Aerospace Exposition: Advancing the Science of Flight Technology (AIAA 2012) . Nashville, United States 09 - 12 Jan 2012 Reston, VA. United States. https://doi.org/10.2514/6.2012-1297Tribological consideration in roller mill machines for agriculture applications
Al-Sandooq, J. M., Yousif, B. F. and Jensen, T. A.. 2012. "Tribological consideration in roller mill machines for agriculture applications." Surface Review and Letters. 19 (6), pp. 1250065-1. https://doi.org/10.1142/S0218625X12500655Characteristics of kenaf fibre immersed in different solutions
Yousif, B. F., Orupabo, C. and Azwa, Z. N.. 2012. "Characteristics of kenaf fibre immersed in different solutions." Journal of Natural Fibers. 9 (4), pp. 207-218. https://doi.org/10.1080/15440478.2012.733149Epoxy composite based on kenaf fibers for tribological applications under wet contact conditions
Yousif, B. F. and Chin, C. W.. 2012. "Epoxy composite based on kenaf fibers for tribological applications under wet contact conditions." Surface Review and Letters. 19 (5), pp. 1250050-1. https://doi.org/10.1142/S0218625X12500503Suitability of using coir fiber/polymeric composite for the design of liquid storage tanks
Yousif, B. F. and Ku, H.. 2012. "Suitability of using coir fiber/polymeric composite for the design of liquid storage tanks." Materials and Design. 36, pp. 847-853. https://doi.org/10.1016/j.matdes.2011.01.063The synergistic effect of hybrid flame retardants on pyrolysis behaviour of hybrid composite materials
Albdiry, M. T., Almosawi, A. I. and Yousif, B. F.. 2012. "The synergistic effect of hybrid flame retardants on pyrolysis behaviour of hybrid composite materials." Journal of Engineering Science and Technology. 7 (3), pp. 351-359.Tribo performance of T-BFRP composite subjected to dry/wet contact conditions
Nirmal, Umar, Hashim, Jamil, Rilling, Dirk, Brevern, P. V. and Yousif, B. F.. 2012. "Tribo performance of T-BFRP composite subjected to dry/wet contact conditions." Francis, Elizabeth (ed.) Natural polymers, biopolymers, biomaterials, and their composites, blends, and IPNs. Toronto, Canada. Apple Academic Press (CRC Press). pp. 311-325Flexural properties of treated and untreated kenaf/epoxy composites
Yousif, B. F., Shalwan, A., Chin, C. W. and Ming, K. C.. 2012. "Flexural properties of treated and untreated kenaf/epoxy composites." Materials and Design. 40, pp. 378-385. https://doi.org/10.1016/j.matdes.2012.04.017Investigating the efficiency of ultrasound for controlling bio-fouling in batch membrane systems
Yusaf, T. F. and Al-Juboori, Raed Ahmed. 2012. "Investigating the efficiency of ultrasound for controlling bio-fouling in batch membrane systems ." Noor, M. M., Baker, Paul and Yusaf, Talal F. (ed.) USQ Combustion Meeting 2012 (21st November). Toowoomba, Australia 21 Nov 2012 Toowoomba, Australia.The use of microalgae biodiesel in diesel engine: production, extraction and engine performance
Yusaf, T. F. and Al-lwayzy, Saddam H.. 2012. "The use of microalgae biodiesel in diesel engine: production, extraction and engine performance." Noor, M. M., Baker, Paul and Yusaf, Talal F. (ed.) USQ Combustion Meeting 2012 (21st November). Toowoomba, Australia 21 Nov 2012 Toowoomba, Australia.A review of mild combustion and open furnace design consideration
Noor, M. M., Wandel, Andrew P. and Yusaf, Talal. 2012. "A review of mild combustion and open furnace design consideration." International Journal of Automotive and Mechanical Engineering. 6 (1), pp. 730-754.Fibre composite railway sleeper design by using FE approach and optimization techniques
Awad, Ziad K. and Yusaf, Talal. 2012. "Fibre composite railway sleeper design by using FE approach and optimization techniques." Structural Engineering and Mechanics. 41 (2), pp. 231-242. https://doi.org/10.12989/sem.2012.41.2.231Artificial neural networks approach for the prediction of thermal balance of SI engine using ethanol-gasoline blends
Kiani, Mostafa Kiani Deh, Ghobadian, Barat, Ommi, Fathollah, Najafi, Gholamhassan and Yusaf, Talal. 2012. "Artificial neural networks approach for the prediction of thermal balance of SI engine using ethanol-gasoline blends." Quirchmayr, Gerald, Basl, Josef, You, Ilsun, Xu, Lida and Weippl, Edgar (ed.) 2012 International Cross-Domain Conference and Workshop on Availability, Reliability, and Security (CD-ARES 2012). Prague, Czech Republic 20 - 24 Aug 2012 Berlin, Germany. https://doi.org/10.1007/978-3-642-32498-7_3Design of newly fabricated tribological machine for wear and frictional experiments under dry/wet condition
Yousif, B. F.. 2013. "Design of newly fabricated tribological machine for wear and frictional experiments under dry/wet condition." Materials and Design. 48, pp. 2-13. https://doi.org/10.1016/j.matdes.2012.06.046Betelnut fibres as an alternative to glass fibres to reinforce thermoset composites: a comparative study
Nirmal, Umar, Hashim, Jamil, Lau, Saijod T. W., Yuhazri, M. Y. and Yousif, B. F.. 2012. "Betelnut fibres as an alternative to glass fibres to reinforce thermoset composites: a comparative study." Textile Research Journal. 82 (11), pp. 1107-1120. https://doi.org/10.1177/0040517512439945Morphological structures and tribological performance of unsaturated polyester based untreated/silane-treated halloysite nanotubes
Albdiry, M. T. and Yousif, B. F.. 2013. "Morphological structures and tribological performance of unsaturated polyester based untreated/silane-treated halloysite nanotubes." Materials and Design. 48, pp. 68-76. https://doi.org/10.1016/j.matdes.2012.08.035Initial development of ice crystal ice accretion at conditions related to turbofan operation at high Altitude
Saleh, Khalid Hashim. 2013. Initial development of ice crystal ice accretion at conditions related to turbofan operation at high Altitude. PhD Thesis Doctor of Philosophy. University of Southern Queensland.HCCI engine: numerical and experimental approach
Hairuddin, A. Aziz, Yusaf, Talal F. and Wandel, Andrew P.. 2012. "HCCI engine: numerical and experimental approach." Noor, M. M., Baker, Paul and Yusaf, Talal F. (ed.) USQ Combustion Meeting 2012 (26th September). Toowoomba, Australia 26 Sep 2012 Toowoomba, Australia.A critical review on the manufacturing processes in relation to the properties of nanoclay/polymer composites
Albdiry, M. T., Yousif, B. F., Ku, H. and Lau, K. T.. 2013. "A critical review on the manufacturing processes in relation to the properties of nanoclay/polymer composites." Journal of Composite Materials. 47 (9), pp. 1093-1115. https://doi.org/10.1177/0021998312445592Investigation of Biogas Moderate or Intense Low Oxygen Dilution (MILD) combustion on open furnace bluff-body burner
Noor, M. M., Wandel, Andrew P. and Yusaf, T. F.. 2012. "Investigation of Biogas Moderate or Intense Low Oxygen Dilution (MILD) combustion on open furnace bluff-body burner." Noor, M. M., Baker, Paul and Yusaf, Talal F. (ed.) USQ Combustion Meeting 2012 (29th August). Toowoomba, Australia 29 Aug 2012 Toowoomba, Australia.Modelling of non-premixed turbulent combustion of hydrogen using conditional moment closure method
Noor, M. M., Hairuddin, A. Aziz, Wandel, Andrew P. and Yusaf, T. F.. 2012. "Modelling of non-premixed turbulent combustion of hydrogen using conditional moment closure method." IOP Conference Series: Materials Science and Engineering. 36 (1), pp. 1-17. https://doi.org/10.1088/1757-899X/36/1/012036The modelling of the effect of air fuel ratio on unburned hydrocarbons for MILD combustion
Noor, M. M., Wandel, Andrew P. and Yusaf, T. F.. 2012. "The modelling of the effect of air fuel ratio on unburned hydrocarbons for MILD combustion." Noor, M. M., Rahman, M. M. and Ismail, J. (ed.) 2nd Malaysian Postgraduate Conference (MPC 2012). Gold Coast, Australia 07 - 09 Jul 2012 Sydney, Australia.A preliminary study of control parameters for open furnace mild combustion using CFD
Noor, M. M., Wandel, Andrew P. and Yusaf, T. F.. 2012. "A preliminary study of control parameters for open furnace mild combustion using CFD." Noor, M. M., Rahman, M. M. and Ismail, J. (ed.) 2nd Malaysian Postgraduate Conference (MPC 2012). Gold Coast, Australia 07 - 09 Jul 2012 Sydney, Australia.Mild combustion: a technical review towards open furnace combustion
Noor, M. M., Wandel, Andrew P. and Yusaf, T. F.. 2012. "Mild combustion: a technical review towards open furnace combustion." Noor, M. M., Rahman, M. M. and Ismail, J. (ed.) 2nd Malaysian Postgraduate Conference (MPC 2012). Gold Coast, Australia 07 - 09 Jul 2012 Sydney, Australia.Image-based visual servoing for the super-orbital re-entry of Hayabusa spacecraft
Khan, Razmi, Eichmann, Troy, Buttsworth, David and Upcroft, Ben. 2011. "Image-based visual servoing for the super-orbital re-entry of Hayabusa spacecraft ." 2011 Australasian Conference on Robotics and Automation (ACRA 2011) . Melbourne, Australia 07 - 09 Dec 2011 Sydney, Australia.Numerical investigation of influence of air and fuel dilution for open furnace MILD combustion burner
Noor, M. M., Wandel, Andrew P. and Yusaf, T. F.. 2012. "Numerical investigation of influence of air and fuel dilution for open furnace MILD combustion burner." 2012 Southern Regional Engineering Conference (SREC 2012). Toowoomba, Australia 01 Sep 2012 Canberra, Australia.Analytical and experimental study of pressure dynamics in a pulsed water jet device
Dehkhoda, Sevda, Hood, Michael, Alehossein, Habib and Buttsworth, David. 2012. "Analytical and experimental study of pressure dynamics in a pulsed water jet device." Flow, Turbulence and Combustion. 89 (1), pp. 97-119. https://doi.org/10.1007/s10494-012-9393-0Simplified digital lock-in amplifier algorithm
Leis, J., Martin, P. and Buttsworth, D.. 2012. "Simplified digital lock-in amplifier algorithm." Electronics Letters. 48 (5), pp. 259-261. https://doi.org/10.1049/el.2012.0193Numerical investigation of flow instability in shock tube due to shock wave-contact surface interactions
Al-Falahi, Amir, Yusoff, M. Z., Yusaf, Talal and Ahmed, Diyar I.. 2012. "Numerical investigation of flow instability in shock tube due to shock wave-contact surface interactions." International Journal of Numerical Methods for Heat and Fluid Flow. 22 (3), pp. 377-398. https://doi.org/10.1108/09615531211208079Biofouling in RO system: mechanisms, monitoring and controlling
Al-Juboori, Raed A. and Yusaf, Talal. 2012. "Biofouling in RO system: mechanisms, monitoring and controlling." Desalination. 302, pp. 1-23. https://doi.org/10.1016/j.desal.2012.06.016Identifying the optimum process parameters for ultrasonic cellular disruption of e. coli
Al-Juboori, Raed A. and Yusaf, Talal. 2012. "Identifying the optimum process parameters for ultrasonic cellular disruption of e. coli ." International Journal of Chemical Reactor Engineering. 10 (1), pp. 1-32. https://doi.org/10.1515/1542-6580.2937Predicting the combustion behaviour of a diesel HCCI engine using a zero-dimensional single-zone model
Hairuddin, A. Aziz, Yusaf, Talal F. and Wandel, Andrew P.. 2011. "Predicting the combustion behaviour of a diesel HCCI engine using a zero-dimensional single-zone model." Kennedy, Eric, Dlugogorski, Bogdan, Moghtaderi, Behdad and Masri, Assaad (ed.) 11th Australian Combustion Symposium (ACS 2011). Newcastle, Australia 29 Nov - 01 Dec 2011 Sydney, Australia.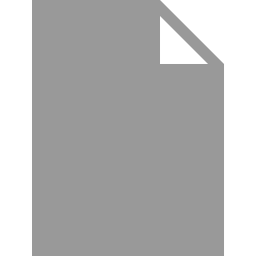