Profile clusters of mood responses
Paper
Paper/Presentation Title | Profile clusters of mood responses |
---|---|
Presentation Type | Paper |
Authors | Parsons-Smith, Renee L. (Author), Terry, Peter C. (Author) and Machin, Tony (Author) |
Journal or Proceedings Title | Proceedings of the 28th International Congress of Applied Psychology (ICAP 2014) |
Year | 2014 |
Place of Publication | Paris, France |
Web Address (URL) of Paper | https://b-com.mci-group.com/Abstract/Statistics/AbstractStatisticsViewPage.aspx?AbstractID=183467 |
Conference/Event | 28th International Congress of Applied Psychology: From Crisis to Sustainable Well-Being (ICAP 2014) |
Event Details | 28th International Congress of Applied Psychology: From Crisis to Sustainable Well-Being (ICAP 2014) Event Date 08 to end of 13 Jul 2014 Event Location Paris, France |
Abstract | Research into mood and performance relationships has had a strong focus on psychometric testing, commonly referred to as mood profiling. Although mood profiling has been used extensively in applied psychology since the 1970s, there are no published investigations of whether distinct mood profile clusters can be identified among the general population. In the present investigation, an online mood profiling system (www.moodprofiling.com) was developed, based on the Brunel Mood Scale and the conceptual framework of Lane and Terry (2000). The mood responses of 2,364 participants were analysed using agglomerative, hierarchical cluster analysis, which identified six distinct and theoretically meaningful profiles. K-means clustering with a prescribed six-cluster solution was used to further refine the final parameter solution. The mood profiles identified in the cluster analysis were termed the iceberg (n = 695, 29.4%), inverse iceberg (n = 244, 10.3%), inverse Everest (n = 64, 2.7%), shark fin (n = 409, 17.3%), surface (n = 349, 14.8%), and submerged profiles (n = 603, 25.5%). A multivariate analysis of variance showed significant differences between clusters on each dimension of mood, being tension [F(5, 2358) = 615.96, p < .001], depression [F(5, 2358) = 874.00, p < .001], anger [F(5, 2358) = 715.04, p < .001], vigour [F(5, 2358) = 613.03, p < .001], fatigue [F(5, 2358) = 873.92, p < .001], and confusion [F(5, 2358) = 861.90, p < .001]. A chi-square test of goodness-of-fit indicated that gender [χ²(5, N = 2,364) = 25.48, p < .001], age [χ²(25, N = 2,364) = 78.30, p < .001], and education level [χ²(15, N = 2,364) = 41.86, p < .001], were unequally distributed across clusters. Further, a discriminant analysis showed that cluster membership could be correctly classified with a high degree of accuracy: iceberg (100%), inverse iceberg (92.2%), inverse Everest (98.4%), shark fin (94.4%), surface (82.8%), and submerged (98.3%). Identification of discrete mood profile clusters will assist in the interpretation of individual mood profiles by applied practitioners. |
Keywords | BRUMScluster; analysis; mood; profiles; psychometric |
ANZSRC Field of Research 2020 | 520406. Sensory processes, perception and performance |
520107. Sport and exercise psychology | |
520105. Psychological methodology, design and analysis | |
Public Notes | Only abstracts published in conference proceedings, as supplied here. Permanent restricted access to published version in accordance with the copyright policy of the publisher. |
Byline Affiliations | School of Psychology, Counselling and Community |
Institution of Origin | University of Southern Queensland |
https://research.usq.edu.au/item/q2z08/profile-clusters-of-mood-responses
1852
total views5
total downloads6
views this month0
downloads this month
Export as
Related outputs
Strike 3 … Out! Investigating Pre-Game Moods, Performance, and Mental Health of Softball Umpires
Houison, Ronald J., Lamont-Mills, Andrea, Kotiw, Michael and Terry, Peter C.. 2024. "Strike 3 … Out! Investigating Pre-Game Moods, Performance, and Mental Health of Softball Umpires." Sports. 12 (2). https://doi.org/10.3390/sports12020050Better Be Ready! Evacuation Experiences During a Bushfire Emergency
Anderson, Carina C., Rockloff, Susan F., Burton, Lucinda P., Terry, Victoria R., Jensen, Sally K. J, Nolan, Anne T. and Terry, Peter C.. 2024. "Better Be Ready! Evacuation Experiences During a Bushfire Emergency." Fire. 7 (12), p. 458. https://doi.org/10.3390/fire7120458Prevalence of Specific Mood Profile Clusters among Elite and Youth Athletes at a Brazilian Sports Club
Provenza de Miranda Rohlfs, Izabel Cristina, Noce, Franco, Wilke, Carolina, Terry, Victoria R., Parsons-Smith, Renée L. and Terry, Peter C.. 2024. "Prevalence of Specific Mood Profile Clusters among Elite and Youth Athletes at a Brazilian Sports Club." Sports. 12 (7). https://doi.org/10.3390/sports12070195Individual Resilience and Disaster-Specific Adaptation and Resilience Following a Bushfire Event in Regional Queensland
Rockloff, Susan F., Anderson, Carina C., Burton, Lucinda P., Terry, Victoria, Jensen, Sally K., Nolan, Anne and Terry, Peter C.. 2024. "Individual Resilience and Disaster-Specific Adaptation and Resilience Following a Bushfire Event in Regional Queensland." Sustainability. 16 (16), p. 7011. https://doi.org/10.3390/su16167011Students’ first professional placement: unravelling expectations and realities
Eastgate, Lindsay, Bialocerkowski, Andrea, Creed, Peter, Hood, Michelle, Machin, Michael Anthony, Brough, Paula and Winterbotham, Sonya. 2024. "Students’ first professional placement: unravelling expectations and realities." Education + Training. 66 (7). https://doi.org/10.1108/ET-07-2022-0273A Qualitative Study on Workplace Mental Wellbeing in the Singapore Context
Yip, C.C., Machin, T. and Goh, Yong Wah. 2024. "A Qualitative Study on Workplace Mental Wellbeing in the Singapore Context." Qualitative Report: an online journal dedicated to qualitative research since 1990. 29 (7), pp. 1981-2015. https://doi.org/10.46743/2160-3715/2024.6795Feeling the Stress: Salivary Cortisol Responses of Softball Umpires during National Championships
Houison, Ronald J, Lamont-Mills, Andrea, Kotiw, Michael and Terry, Peter C.. 2024. "Feeling the Stress: Salivary Cortisol Responses of Softball Umpires during National Championships." Sports. 12 (5). https://doi.org/10.3390/sports12050128Comparing ChatGPT With Experts’ Responses to Scenarios that Assess Psychological Literacy
Machin, M. Anthony, Machin, Tanya M. and Gasson, Natalie. 2024. "Comparing ChatGPT With Experts’ Responses to Scenarios that Assess Psychological Literacy." Psychology Learning and Teaching. 23 (2), pp. 265-280. https://doi.org/10.1177/14757257241241592Updated consensus statement on improving the mental health of high-performance athletes
Henriksen, Kristoffer, Schinke, Robert J., Moesch, Karin, McCann, Sean, Parham, William D., Larsen, Carsten Hvid and Terry, Peter C.. 2024. "Updated consensus statement on improving the mental health of high-performance athletes." Schinke, Robert J. (ed.) Mental Health in Sport and Physical Activity. United Kingdom. Routledge.Heading for the Frontline: Mood, Stress, Resilience, and Coping of Nursing Graduates during a Global Pandemic.
Terry, Victoria R, Parsons-Smith, , Renee L., Elliott, Jessica, Roderick, Geraldine, Luyke, Patricia and Terry, Peter C.. 2024. "Heading for the Frontline: Mood, Stress, Resilience, and Coping of Nursing Graduates during a Global Pandemic. " Sustainability. 16 (4), p. 1492. https://doi.org/10.3390/su16041492Pre-Event Self-Efficacy and Sports Performance: A Systematic Review with Meta-Analysis
Lochbaum, Marc, Sisneros, Cassandra, Cooper, Sydney and Terry, Peter C.. 2023. "Pre-Event Self-Efficacy and Sports Performance: A Systematic Review with Meta-Analysis." Sports. 11 (11). https://doi.org/10.3390/sports11110222Psychometric Characteristics of the Brazil Mood Scale among Youth and Elite Athletes Using Two Response Time Frames
Provenza de Miranda Rohlfs, Izabel Cristina, Noce, Franco, Gabbett, Tim J., Wilke, Carolina, Vido, Marcelo, Terry, Victoria R. and Terry, Peter C.. 2023. "Psychometric Characteristics of the Brazil Mood Scale among Youth and Elite Athletes Using Two Response Time Frames." Sports. 11 (12). https://doi.org/10.3390/sports11120244Monitoring female athlete wellbeing during international basketball competition: A case study from the 2023 FIBA Women’s Asia Cup
Bird, Stephen P., Parsons-Smith, Renée L. and Terry, Peter C.. 2023. "Monitoring female athlete wellbeing during international basketball competition: A case study from the 2023 FIBA Women’s Asia Cup." 2023 ASCA International Conference on Applied Strength and Conditioning. Gold Coast, Australia 02 - 04 Nov 2023 Australia.Testing the Psychometric Properties of an Arabic Version of the Brunel Mood Scale among Physical Education Students
Sahli, Hajer, Sahli, Faten, Saidane, Mouna, Rebhi, Mahmoud, Guelmami, Noomen, Trabelsi, Khaled, Jahrami, Haitham, Ammar, Achraf, Terry, Peter and Zghibi, Makram. 2023. "Testing the Psychometric Properties of an Arabic Version of the Brunel Mood Scale among Physical Education Students." European Journal of Investigation in Health Psychology and Education. 13 (8), pp. 1539-1552. https://doi.org/10.3390/ejihpe13080112Students managing work and study role boundaries: a person-centred approach
Creed, Peter A., Hood, Michelle, Bialocerkowski, Andrea, Machin, M. Anthony, Brough, Paula, Kim, Sujin, Winterbotham, Sonya and Eastgate, Lindsay. 2023. "Students managing work and study role boundaries: a person-centred approach." Frontiers in Psychology. 14. https://doi.org/10.3389/fpsyg.2023.1116031SPSS Explained
Hinton, Perry R., McMurray, Isabella, Brownlow, Charlotte and Terry, Peter C.. 2023. SPSS Explained. United Kingdom. Routledge.The higher degree research student experience in Australian universities: a systematic literature review
Brownlow, Charlotte, Eacersall, Douglas C., Martin, Neil and Parsons-Smith, Renée. 2023. "The higher degree research student experience in Australian universities: a systematic literature review." Higher Education Research and Development. 42 (7), pp. 1608-1623. https://doi.org/10.1080/07294360.2023.2183939Cross-Cultural Validation of the Malaysian Mood Scale and Tests of Between-Group Mood Differences
Lew, Philip Chun Foong, Parsons-Smith, Renée L., Lamont-Mills, Andrea and Terry, Peter C.. 2023. "Cross-Cultural Validation of the Malaysian Mood Scale and Tests of Between-Group Mood Differences ." International Journal of Environmental Research and Public Health. 20 (4), pp. 1-24. https://doi.org/10.3390/ijerph20043348The 4R Model of Mood and Emotion for Sustainable Mental Health in Organisational Settings
Beedie, Christopher J., Lane, Andrew M., Udberg, Robert and Terry, Peter C.. 2022. "The 4R Model of Mood and Emotion for Sustainable Mental Health in Organisational Settings." Sustainability. 14 (18), pp. 1-28. https://doi.org/10.3390/su141811670Consensus statement on improving the mental health of high performance athletes
Henriksen, Kristoffer, Schinke, Robert, Moesch, Karin, McCann, Sean, Parham, William, Larsen, Carsten Hvid and Terry, Peter. 2020. "Consensus statement on improving the mental health of high performance athletes." International Journal of Sport and Exercise Psychology. 18 (5), pp. 553-560. https://doi.org/10.1080/1612197X.2019.1570473Mood profiles of amateur triathletes: Implications for mental health and performance
Parsons-Smith, Renee L., Barkase, Sherry, Lovell, Geoff P., Vleck, Veronica and Terry, Peter C.. 2022. "Mood profiles of amateur triathletes: Implications for mental health and performance." Frontiers in Psychology. 13, pp. 1-14. https://doi.org/10.3389/fpsyg.2022.925992Ramadan observance is associated with higher fatigue and lower vigor in athletes: a systematic review and meta-analysis with meta-regression
Trabelsi, Khaled, Ammar, Achraf, Boujelbane, Mohamed Ali, Khacharem, Aimen, Elghoul, Yousri, Boukhris, Omar, Aziz, Abdul Rashid, Taheri, Morteza, Irandoust, Khadijeh, Khanfir, Saber, Chtourou, Hamdi, Clark, Cain C. T. and Terry, Peter C.. 2022. "Ramadan observance is associated with higher fatigue and lower vigor in athletes: a systematic review and meta-analysis with meta-regression." International Review of Sport and Exercise Psychology. https://doi.org/10.1080/1750984X.2022.2106790A global experiment on motivating social distancing during the COVID-19 pandemic
Psychological Science Accelerator Self-Determination Theory Coll, A, Machin, Michael A., Machin, Tanya, Martin, Neil I. and Brownlow, Charlotte. 2022. "A global experiment on motivating social distancing during the COVID-19 pandemic." University of Adelaide. 119 (22), pp. 1-11. https://doi.org/10.1073/pnas.2111091119Validation of a Lithuanian-Language Version of the Brunel Mood Scale: The BRUMS-LTU
Terry, Peter C., Skurvydas, Albertas, Lisinskiene, Ausra, Majauskiene, Daiva, Valanciene, Dovile, Cooper, Sydney and Lochbaum, Marc. 2022. "Validation of a Lithuanian-Language Version of the Brunel Mood Scale: The BRUMS-LTU." International Journal of Environmental Research and Public Health. 19 (8), pp. 1-16. https://doi.org/10.3390/ijerph19084867Revisiting the Self-Confidence and Sport Performance Relationship: A Systematic Review with Meta-Analysis
Lochbaum, Marc, Sherburn, Mackenzie, Sisneros, Cassandra, Cooper, Sydney, Lane, Andrew M. and Terry, Peter C.. 2022. "Revisiting the Self-Confidence and Sport Performance Relationship: A Systematic Review with Meta-Analysis." International Journal of Environmental Research and Public Health. 19 (11), pp. 1-19. https://doi.org/10.3390/ijerph19116381Engineering the World of Work: Organizations in an Era of Constant Change
Tziner, Aharon, Bareket-Bojmel, Liad, Fein, Erich C. and Machin, M. Anthony. Tziner, Aharon, Bareket-Bojmel, Liad, Fein, Erich C. and Machin, M. Anthony (ed.) 2022. Engineering the World of Work: Organizations in an Era of Constant Change. Massachusetts, United States. Edward Elgar Publishing.The Australian Handbook for Careers in Psychological Science
Machin, Tony, Machin, Tanya, Jeffries, Carla, Hoare, Nancey and Abel, Susan. Rowling, Samara and Andersen, Nikki (ed.) 2022. The Australian Handbook for Careers in Psychological Science. Australia. University of Southern Queensland.Physical Activity and Healthy Habits Influence Mood Profile Clusters in a Lithuanian Population
Terry, Peter C., Parsons-Smith, Renee L., Skurvydas, Albertas, Lisinskiene, Ausra, Majauskiene, Daiva, Valanciene, Dovile, Cooper, Sydney and Lochbaum, Marc. 2022. "Physical Activity and Healthy Habits Influence Mood Profile Clusters in a Lithuanian Population." Sustainability. 14 (16), pp. 1-16. https://doi.org/10.3390/su141610006Trauma-Informed School Programming: A Partnership Approach to Culturally Responsive Behavior Support
Bellamy, Tessa, Krishnamoorthy, Govind, Ayre, Kay, Berger, Emily, Machin, Tony and Rees, Bronwyn Elizabeth. 2022. "Trauma-Informed School Programming: A Partnership Approach to Culturally Responsive Behavior Support." Sustainability. 14 (7), pp. 1-17. https://doi.org/10.3390/su14073997Student work-study boundary flexibility and relationships with burnout and study engagement
Creed, Peter A., Hood, Michelle, Brough, Paula, Bialocerkowski, Andrea, Machin, M. Anthony, Winterbotham, Sonya and Eastgate, Lindsay. 2022. "Student work-study boundary flexibility and relationships with burnout and study engagement." Journal of Education and Work. 35 (3), pp. 256-271. https://doi.org/10.1080/13639080.2022.2048250Organisational career growth: implications for future perceived employability in students who work
Creed, Peter A., Hood, Michelle, Bagley, Louella, Brough, Paula, Bialocerkowski, Andrea, Machin, M. Anthony and Winterbotham, Sonya. 2022. "Organisational career growth: implications for future perceived employability in students who work." International Journal of Educational Research. 112, pp. 1-13. https://doi.org/10.1016/j.ijer.2022.101950Research Methods in the Psychological Sciences
Machin, Tony and Fein, Erich. 2022. "Research Methods in the Psychological Sciences." Machin, Tony, Machin, Tanya, Jeffries, Carla and Hoare, Nancey (ed.) The Australian Handbook for Careers in Psychological Science. Toowoomba, Australia. University of Southern Queensland. pp. 57-82A Systematic Review of Multilevel Influenced Risk-Taking in Helicopter and Small Airplane Normal Operations Operations
Harris, Matt R., Fein, Erich C. and Machin, M. Anthony. 2022. "A Systematic Review of Multilevel Influenced Risk-Taking in Helicopter and Small Airplane Normal Operations Operations." Frontiers in Public Health. 10, pp. 1-18. https://doi.org/10.3389/fpubh.2022.823276Sport psychology and performance meta-analyses: A systematic review of the literature
Lochbaum, Marc, Stoner, Elisabeth, Hefner, Tristen, Cooper, Sydney, Lane, Andrew M. and Terry, Peter C.. 2022. "Sport psychology and performance meta-analyses: A systematic review of the literature." PLoS One. 17 (2), pp. 1-22. https://doi.org/10.1371/journal.pone.0263408In the mood for triathlon
Parsons-Smith, Renee L., Barkase, Sherry, Lovell, Geoff P. and Terry, Peter C.. 2021. "In the mood for triathlon." Schinke, Robert J. and Chang, Yu-Kai (ed.) International Society of Sport Psychology: 15th World Congress (ISSP 2021). Taipei, Taiwan 30 Sep - 04 Oct 2021 United States.Psychometric re-evaluation of the brazil mood scale and evidence of mood profile clusters among youth athletes in Brazil
Brandao, Regina F., Correa, Marianna, Sermarine, Marcia, Angelo, Daniela L., Parsons-Smith, Renee L. and Terry, Peter C.. 2021. "Psychometric re-evaluation of the brazil mood scale and evidence of mood profile clusters among youth athletes in Brazil." Schinke, Robert J. and Chang, Yu-Kai (ed.) International Society of Sport Psychology: 15th World Congress (ISSP 2021). Taipei, Taiwan 30 Sep - 04 Oct 2021 United States.Mood profile clusters among Chinese athletes and nonathletes
Terry, Peter C., Parsons-Smith, Renee L., Zhang, Chun-Qing, Si, Gangyan and Chung, Pak- Kwong. 2021. "Mood profile clusters among Chinese athletes and nonathletes." Schinke, Robert J. and Chang, Yu-Kai (ed.) International Society of Sport Psychology: 15th World Congress (ISSP 2021). Taipei, Taiwan 30 Sep - 04 Oct 2021 United States.Developments in emotion and mood research in sport
Terry, Peter C.. 2021. "Developments in emotion and mood research in sport." Schinke, Robert J. and Chang, Yu-Kai (ed.) International Society of Sport Psychology: 15th World Congress (ISSP 2021). Taipei, Taiwan 30 Sep - 04 Oct 2021 United States.Role congruence and study engagement in mature-age students: a serial indirect effects model
Creed, Peter A., Hood, Michelle, Bialocerkowski, Andrea, Machin, M. Anthony, Brough, Paula, Bagley, Louella, Winterbotham Sonya and Eastgate, Lindsay. 2022. "Role congruence and study engagement in mature-age students: a serial indirect effects model." Journal of Education and Work. 35 (6-7), pp. 735-750. https://doi.org/10.1080/13639080.2022.2126967Risks to mental health of higher degree by research (HDR) students during a global pandemic
Brownlow, Charlotte, Eacersall, Douglas, Nelson, Charlie, Parsons-Smith, Renee and Terry, Peter. 2022. "Risks to mental health of higher degree by research (HDR) students during a global pandemic ." PLoS One. 17 (12). https://doi.org/10.1371/journal.pone.0279698Physical activity and mental well-being under COVID-19 lockdown: a cross-sectional multination study
Karageorghis, Costas I., Bird, Jonathan M., Hutchinson, Jasmin C., Hamer, Mark, Delevoye-Turrell, Yvonne N., Guerin, Segolene M. R., Mullin, Elizabeth M., Mellano, Kathleen T., Parsons-Smith, Renee L., Terry, Victoria R. and Terry, Peter C.. 2021. "Physical activity and mental well-being under COVID-19 lockdown: a cross-sectional multination study." BMC Public Health. 21 (1), pp. 1-13. https://doi.org/10.1186/s12889-021-10931-5History and development of the Asian-South Pacific Association of Sport Psychology from 1988 to 2020
Morris, Tony and Terry, Peter C.. 2021. "History and development of the Asian-South Pacific Association of Sport Psychology from 1988 to 2020." Asian Journal of Sport and Exercise Psychology. 1 (1), pp. 6-11. https://doi.org/10.1016/j.ajsep.2021.03.002Mood profiling for sustainable mental health among athletes
Terry, Peter C. and Parsons-Smith, Renee L.. 2021. "Mood profiling for sustainable mental health among athletes." Sustainability. 13 (11). https://doi.org/10.3390/su13116116Publishing trends in the International Journal of Sport Psychology during the First 50 years (1970-2019), with a particular focus on Asia and Oceania
Terry, Peter C., Parsons-Smith, Renee L., Quartiroli, Alessandro and Blackmore, Susan M.. 2021. "Publishing trends in the International Journal of Sport Psychology during the First 50 years (1970-2019), with a particular focus on Asia and Oceania." International Journal of Sport Psychology. 51 (5), pp. 493-513. https://doi.org/10.7352/IJSP.2020.51.493Influence of sex, age, and education on mood profile clusters
Terry, Peter C., Parsons-Smith, Renee, King, Rachel and Terry, Victoria R.. 2021. "Influence of sex, age, and education on mood profile clusters." PLoS One. 16 (2), pp. 1-16. https://doi.org/10.1371/journal.pone.0245341Why it pays to be nice: the impact of civil and uncivil behaviours on job satisfaction, engagement, psychological strain, and job turnover
Fogarty, Gerard J., Machin, Tony M., Sankey, Kim and Goh, Hong Eng. 2013. "Why it pays to be nice: the impact of civil and uncivil behaviours on job satisfaction, engagement, psychological strain, and job turnover." 10th Industrial and Organisational Psychology Conference (IOP 2013). Perth, Australia 03 - 06 Jul 2013 Melbourne, Australia.Developing a measure of respectful workplaces
Fogarty, Gerard J. and Machin, Tony M.. 2011. Developing a measure of respectful workplaces. Toowoomba, Australia. Unpublished.Psychometric properties of the Brunel Mood Scale among athletes and non-athletes in Singapore
Han, Christie S. Y., Parsons-Smith, Renee L., Fogarty, Gerard J. and Terry, Peter C.. 2022. "Psychometric properties of the Brunel Mood Scale among athletes and non-athletes in Singapore." International Journal of Sport and Exercise Psychology. 20 (3), pp. 698-714. https://doi.org/10.1080/1612197X.2021.1919740Foreword: Feelings in sport: theory, research, and practical implications for performance and well-being
Terry, Peter C.. 2020. "Foreword: Feelings in sport: theory, research, and practical implications for performance and well-being." Ruiz, Montse C. and Robazza, Claudio (ed.) Feelings in sport: theory, research, and practical implications for performance and well-being. Milton Park, United Kingdom. Routledge. pp. 1-4Mood profiling in Singapore: cross-cultural validation and potential applications of mood profile clusters
Han, Christie S. Y., Parsons-Smith, Renee L. and Terry, Peter C.. 2020. "Mood profiling in Singapore: cross-cultural validation and potential applications of mood profile clusters." Frontiers in Psychology. 11, pp. 1-9. https://doi.org/10.3389/fpsyg.2020.00665Mood responses associated with COVID-19 restrictions
Terry, Peter C., Parsons-Smith, Renee L. and Terry, Victoria R.. 2020. "Mood responses associated with COVID-19 restrictions." Frontiers in Psychology. 11, pp. 1-10. https://doi.org/10.3389/fpsyg.2020.589598Psychological Literacy: What Is It and How Do We Measure It?
Machin, M. Anthony and Machin, Tanya M.. 2019. "Psychological Literacy: What Is It and How Do We Measure It?" Baker, Suzanne (ed.) 126th Annual American Psychological Association Convention (APA 2018). San Francisco, United States 09 - 12 Aug 2018 Washington, D.C., United States.Identification of stress impact on personality density distributions
Lys, Brendan, Tao, Xiaohui, Machin, Tony, Zhang, Ji and Zhong, Ning. 2019. "Identification of stress impact on personality density distributions." Liang, Peipeng, Goel, Vinod and Shan, Chunlei (ed.) 12th International Conference on Brain Informatics (BI 2019). Haikou, China 13 - 15 Dec 2019 Cham, Switzerland. Springer. https://doi.org/10.1007/978-3-030-37078-7_26The impact of a Student vs Worker identity on work-study conflict and facilitation in university students
Machin, Tony, Winterbotham, Sonya, Creed, Peter, Hood, Michelle, Brough, Paula, Bialocerkowski, Andrea and Eastgate, Lindsay. 2019. "The impact of a Student vs Worker identity on work-study conflict and facilitation in university students." Nelson, Karen and Field, Rachael (ed.) 5th Students Transitions Achievement Retention & Success Conference (STARS 2019). Melbourne, Australia 07 - 10 Jul 2019Identification and Incidence of Mood Profile Clusters Among Sport Participants
Terry, P. and Parsons-Smith, R.. 2019. "Identification and Incidence of Mood Profile Clusters Among Sport Participants." 2019 ASICS SMA Conference (ASICS SMA 2019). Twin Waters, Australia 23 - 26 Oct 2019 Chatswood, Australia. Elsevier. https://doi.org/10.1016/j.jsams.2019.08.129Experiencing the Journey Together: The Role of Social Support during the Doctorate
Machin, Tanya, M. and Parsons-Smith, Renee L.. 2019. "Experiencing the Journey Together: The Role of Social Support during the Doctorate." Machin, Tanya, M., Clara, Marc and Danaher, Patrick Alan (ed.) Traversing the Doctorate: Reflections and Strategies from Students, Supervisors and Administrators. Cham, Switzerland. Springer. pp. 269-285Psychometric characteristics of the Brunel Mood Scale in a Singaporean context
Han, Christie, Parsons-Smith, Renee L., Fogarty, Gerard J. and Terry, Peter C.. 2019. "Psychometric characteristics of the Brunel Mood Scale in a Singaporean context." 15th European Congress of Sport and Exercise Psychology (FEPSAC Congress 2019). Münster, Germany 15 - 20 Jul 2019Effects of music in exercise and sport: a meta-analytic review
Terry, Peter C., Karageorghis, Costas I., Curran, Michelle L., Martin, Olwenn V. and Parsons-Smith, Renee L.. 2020. "Effects of music in exercise and sport: a meta-analytic review." Psychological Bulletin. 146 (2), pp. 91-117. https://doi.org/10.1037/bul0000216Influence of sex, age, and education on mood profile clusters
Terry, Peter, Parsons-Smith, Renee, Terry, Victoria and King, Rachel. 2020. Influence of sex, age, and education on mood profile clusters. Toowoomba. University of Southern Queensland. https://doi.org/10.26192/57rm-4t21The marriage of psychology and law: testamentary capacity
Zuscak, Simon, Coyle, Ian, Keyzer, Patrick and Machin, M. Anthony. 2019. "The marriage of psychology and law: testamentary capacity." Psychiatry Psychology and Law. 26 (4), pp. 614-643. https://doi.org/10.1080/13218719.2018.1557506Joint position stand of the ISSP, FEPSAC, ASPASP, and AASP on professional accreditation
Schinke, Robert J., Si, Gangyan, Zhang, Liwei, Elbe, Anne-Marie, Watson, Jack, Harwood, Chris and Terry, Peter C.. 2018. "Joint position stand of the ISSP, FEPSAC, ASPASP, and AASP on professional accreditation." Psychology of Sport and Exercise. 38, pp. 107-115. https://doi.org/10.1016/j.psychsport.2018.06.005The day Australian cricket lost its integrity and a country reacted with shock and anger
Terry, Peter. 2018. "The day Australian cricket lost its integrity and a country reacted with shock and anger." The Conversation. 26 March 2018, pp. 1-3.This is bigger than Serena Williams: lessons from the 2018 US Open tennis
Terry, Peter. 2018. "This is bigger than Serena Williams: lessons from the 2018 US Open tennis." The Conversation. 11 September 2018, pp. 1-3.Effects of music on arousal during imagery in elite shooters: a pilot study
Kuan, Garry, Morris, Tony and Terry, Peter. 2017. "Effects of music on arousal during imagery in elite shooters: a pilot study." PLoS One. 12 (4), pp. 1-13. https://doi.org/10.1371/journal.pone.0175022Cross-cultural validation of mood profile clusters in a sport and exercise context
Quartiroli, Alessandro, Parsons-Smith, Renee L., Fogarty, Gerard J., Kuan, Garry and Terry, Peter C.. 2018. "Cross-cultural validation of mood profile clusters in a sport and exercise context." Frontiers in Psychology. 9, pp. 1-10. https://doi.org/10.3389/fpsyg.2018.01949The Heat Is On: Effects of Synchronous Music on Psychophysiological Parameters and Running Performance in Hot and Humid Conditions
Nikol, Luke, Kuan, Garry, Ong, Marilyn, Chang, Yu-Kai and Terry, Peter C.. 2018. "The Heat Is On: Effects of Synchronous Music on Psychophysiological Parameters and Running Performance in Hot and Humid Conditions." Frontiers in Psychology. 9, pp. 1-9. https://doi.org/10.3389/fpsyg.2018.01114Cross-cultural validation of mood profile clusters in a sport and exercise context
Quartiroli, Ale, Terry, Peter C., Fogarty, Gerard J. and Parsons-Smith, Renée L.. 2018. "Cross-cultural validation of mood profile clusters in a sport and exercise context." 8th Asian South Pacific Association of Sport Psychology, International Congress of Sport Psychology (ASPASP 2018): Crossing Borders and Expanding New Horizons for Sport and Exercise Psychology. Daegu, South Korea 29 Jun - 03 Jul 2018Effects of relaxing and arousing music during imagery training on dart-throwing performance, physiological arousal indices, and competitive state anxiety
Kuan, Garry, Morris, Tony, Kueh, Yee Cheng and Terry, Peter C.. 2018. "Effects of relaxing and arousing music during imagery training on dart-throwing performance, physiological arousal indices, and competitive state anxiety." Frontiers in Psychology. 9, pp. 1-12. https://doi.org/10.3389/fpsyg.2018.00014Development and initial validation of the Italian Mood Scale (ITAMS) for use in sport and exercise contexts
Quartiroli, Alessandro, Terry, Peter C. and Fogarty, Gerard J.. 2017. "Development and initial validation of the Italian Mood Scale (ITAMS) for use in sport and exercise contexts." Frontiers in Psychology. 8, pp. 1-10. https://doi.org/10.3389/fpsyg.2017.01483A framework for self-determination in massive open online courses: design for autonomy, competence, and relatedness
Martin, Neil I., Kelly, Nick and Terry, Peter C.. 2018. "A framework for self-determination in massive open online courses: design for autonomy, competence, and relatedness." Australasian Journal of Educational Technology. 34 (2), pp. 35-55. https://doi.org/10.14742/ajet.3722Face-to-face instruction combined with online resources improves retention of clinical skills among undergraduate nursing students
Terry, Victoria R., Terry, Peter C., Moloney, Clint and Bowtell, Les. 2018. "Face-to-face instruction combined with online resources improves retention of clinical skills among undergraduate nursing students." Nurse Education Today. 61, pp. 15-19. https://doi.org/10.1016/j.nedt.2017.10.014Work intensification, work–life interference, stress, and well-being in Australian workers
Fein, Erich C., Skinner, Natalie and Machin, M. Anthony. 2017. "Work intensification, work–life interference, stress, and well-being in Australian workers." International Studies of Management and Organization. 47 (4), pp. 360-371. https://doi.org/10.1080/00208825.2017.1382271Identification and description of novel mood profile clusters
Parsons-Smith, Renee L., Terry, Peter C. and Machin, M. Anthony. 2017. "Identification and description of novel mood profile clusters." Frontiers in Psychology. 8, pp. 1-13. https://doi.org/10.3389/fpsyg.2017.01958Restoration of organisational climate: an advanced (in)civility model and measure of experienced, instigated and observed workplace incivility
Smith, D., Dearman, D., Machin, M. A. and Beccaria, G.. 2017. "Restoration of organisational climate: an advanced (in)civility model and measure of experienced, instigated and observed workplace incivility." European Association of Work and Organizational Psychology 2017: Enabling Change Through Work and Organizational Psychology: Opportunities and Challenges for Research and Practice.. Dublin, Ireland 17 - 20 May 2017 Ireland.Self-determined leadership and motivation: relating two European constructs of engaging leadership and six dimensions of employee work motivation
Smith, D., Machin, M. A., Schaufeli, W. and Alban-Metcalfe, J.. 2017. "Self-determined leadership and motivation: relating two European constructs of engaging leadership and six dimensions of employee work motivation." European Association of Work and Organizational Psychology 2017: Enabling Change Through Work and Organizational Psychology: Opportunities and Challenges for Research and Practice.. Dublin, Ireland 17 - 20 May 2017 Ireland.The Organisational Socio-Ecological (In)Civility Model: framing multilevel (in)civility effects on employees within organisational climate and culture
Smith, D., Machin, M. A. and Beccaria, G.. 2017. "The Organisational Socio-Ecological (In)Civility Model: framing multilevel (in)civility effects on employees within organisational climate and culture." European Association of Work and Organizational Psychology 2017: Enabling Change Through Work and Organizational Psychology: Opportunities and Challenges for Research and Practice.. Dublin, Ireland 17 - 20 May 2017 Ireland.Want to win? Let music give you the edge
Terry, Peter. 2014. "Want to win? Let music give you the edge." The Conversation. 17 Oct 2014, pp. 1-6.Inside sport psychology
Karageorghis, Costas I. and Terry, Peter C.. 2011. Inside sport psychology. Champaign, IL. United States. Human Kinetics Publishers.Psychologia dla sportowcow
Karageorghis, Costas I. and Terry, Peter C.. 2014. Psychologia dla sportowcow. Poland. Insignis Media.Respectful workplace dimensions mediating relations between engaging leadership and employee outcomes
Machin, Tony, Dearman, Dale and Smith, David. 2017. "Respectful workplace dimensions mediating relations between engaging leadership and employee outcomes." 12th International Conference on Occupational Stress and Health. Minneapolis, MN, United States 07 - 10 Jun 2017 Washington DC, United States.Development and initial validation of an instrument to assess the motivational qualities of music in exercise and sport: the Brunel Music Rating Inventory
Karageorghis, Costas I., Terry, Peter C. and Lane, Andrew M.. 2010. "Development and initial validation of an instrument to assess the motivational qualities of music in exercise and sport: the Brunel Music Rating Inventory." Journal of Sports Sciences. 17 (9), pp. 713-724. https://doi.org/10.1080/026404199365579The use of arousing and relaxing classical music for novices and elite performers of fine-motor and power skill tasks
Kuan, Garry, Morris, Tony and Terry, Peter. 2014. "The use of arousing and relaxing classical music for novices and elite performers of fine-motor and power skill tasks." 28th International Congress of Applied Psychology: From Crisis to Sustainable Well-Being (ICAP 2014). Paris, France 08 - 13 Jul 2014 Paris, France.From classical conditioning to Michael Phelps: music applications in elite sport
Terry, Peter C.. 2014. "From classical conditioning to Michael Phelps: music applications in elite sport." 28th International Congress of Applied Psychology: From Crisis to Sustainable Well-Being (ICAP 2014). Paris, France 08 - 13 Jul 2014 Paris, France.Does music really make a difference? Meta-analytic review of a century of research
Terry, Peter C., Curran, M. and Karageorghis, Costas I.. 2014. "Does music really make a difference? Meta-analytic review of a century of research." 28th International Congress of Applied Psychology: From Crisis to Sustainable Well-Being (ICAP 2014). Paris, France 08 - 13 Jul 2014 Paris, France.A Test and Extension of Lane and Terry’s (2000) Conceptual Model of Mood-Performance Relationships Using a Large Internet Sample
Lane, Andrew M., Terry, Peter C., Devonport, Tracey J., Friesen, Andrew P. and Totterdell, Peter A.. 2017. "A Test and Extension of Lane and Terry’s (2000) Conceptual Model of Mood-Performance Relationships Using a Large Internet Sample." Frontiers in Psychology. 8, pp. 1-11. https://doi.org/10.3389/fpsyg.2017.00470Online mood profiling and self-regulation of affective responses
Lane, Andrew M. and Terry, Peter C.. 2016. "Online mood profiling and self-regulation of affective responses." Schinke, Robert J., McGannon, Kerry R. and Smith, Brett (ed.) Routledge international handbook of sport psychology. Milton Park, United Kingdom. Routledge. pp. 324-334Spor psikolojisi (Inside sport psychology)
Karageorghis, Costas I. and Terry, Peter C.. 2015. Spor psikolojisi (Inside sport psychology). Istanbul, Turkey. Nobel / Human Kinetics Publishers, Inc..Introduction to the special issue on providing sport psychology support for Olympic athletes: international perspectives
Terry, Peter C. and Si, Gangyan. 2015. "Introduction to the special issue on providing sport psychology support for Olympic athletes: international perspectives." International Journal of Sport and Exercise Psychology. 13 (1), pp. 1-3. https://doi.org/10.1080/1612197X.2015.992162The psychology of scrummaging
Mellalieu, Steve D. and Terry, Peter C.. 2012. "The psychology of scrummaging." Rodriguez, Enrique (ed.) The art of scrummaging. Australia. Enrique TOPO Rodriguez. pp. 105-113Online intravenous pump emulator: as effective as face-to-face simulation for training nursing students
Terry, Victoria R., Moloney, Clint, Bowtell, Leslie and Terry, Peter C.. 2016. "Online intravenous pump emulator: as effective as face-to-face simulation for training nursing students." Nurse Education Today. 40, pp. 198-203. https://doi.org/10.1016/j.nedt.2016.03.004Comparison of the effect of arousing and relaxing music during imagery training for power and fine motor skill sport tasks
Kuan, G., Morris, T. and Terry, P.. 2012. "Comparison of the effect of arousing and relaxing music during imagery training for power and fine motor skill sport tasks ." Brackenridge, Celia (ed.) 2nd International Congress of Science, Education and Medicine in Sport (ICSEMIS 2012): Sport: Inspiring a Learning Legacy. Glasgow, United Kingdom 19 - 24 Jul 2012 London, United Kingdom.Uptake and effects of a new sport program at a school in a disadvantaged locality
Hahn, A., Terry, P. and Simjanovic, M.. 2012. "Uptake and effects of a new sport program at a school in a disadvantaged locality." Journal of Science and Medicine in Sport. 15 (S1), pp. 117-117. https://doi.org/10.1016/j.jsams.2012.11.285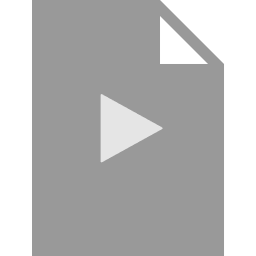
Chasing Olympic dreams and promoting healthy living in a digital world
Terry, Peter. 2012. "Chasing Olympic dreams and promoting healthy living in a digital world." Follow The Sun 2012: Online Learning Futures Festival (SUN 2012): Futures for Knowledge. Leicester, United Kingdom 27 - 30 Mar 2012 Leicester, United Kingdom.Relations between dimensions of empowering leadership and multidimensional work motivation
Machin, M. Anthony, James, Megan and Silcox, Amber. 2015. "Relations between dimensions of empowering leadership and multidimensional work motivation." 11th International Conference on Occupational Stress and Health: Sustainable Work, Sustainable Health, Sustainable Organizations 2015. Atlanta, United States 06 - 09 May 2015Maximising retention of nurses: Australian evidence
Hannigan, Delphine, Patrick, Jeff and Machin, Anthony. 2016. Maximising retention of nurses: Australian evidence. United States. Chamber & Row.In the mood: online mood profiling, mood response clusters, and mood-performance relationships in high-risk vocations
Parsons-Smith, Renee. 2015. In the mood: online mood profiling, mood response clusters, and mood-performance relationships in high-risk vocations. PhD Thesis Doctor of Philosophy. University of Southern Queensland.Analysing the role of job, interpersonal, and personal affective resources in alleviating anxiety and depression at work
Machin, T. and Slack-Smith, J.. 2015. "Analysing the role of job, interpersonal, and personal affective resources in alleviating anxiety and depression at work." 50th Australian Psychological Society Annual Conference (APS 2015): Celebrating the Past, Looking Toward the Future. Gold Coast, Australia 29 Sep - 02 Oct 2015Evidence base and applications of music in elite sport
Terry, Peter C.. 2014. "Evidence base and applications of music in elite sport." 1st International Conference on Applied Psychology: Psychology in Diverse Contexts (ICAP 2014). Colombo, Sri Lanka 22 - 24 Oct 2014 Colombo, Sri Lanka.Motives for Facebook use in an Australian sample
Machin, Tanya M., Jeffries, Carla H. and Machin, M. Anthony. 2014. "Motives for Facebook use in an Australian sample." Society of Australasian Social Psychologists Conference (SASP 2014). Canberra, Australia 10 - 12 Apr 2014 Australia.Sick and tired of being sick and tired: case study of an international kayaker's recovery from chronic fatigue syndrome and psychological preparation for the world championship
Terry, Peter C.. 2014. "Sick and tired of being sick and tired: case study of an international kayaker's recovery from chronic fatigue syndrome and psychological preparation for the world championship." Lane, Andrew M., Whyte, Gregory P., Godfrey, Richard J. and Loosemore, Mike (ed.) Case studies in sport science and medicine. United States. CreateSpace Independent Publishing. pp. 12-16Wrestling in Iran: where old meets new in sport psychology
Hamzeh, Khosro and Terry, Peter C.. 2014. "Wrestling in Iran: where old meets new in sport psychology." Terry, Peter C., Li-Wei, Zhang, Young-Ho, Kim, Morris, Tony and Hanrahan, Stephanie (ed.) Secrets of Asian sport psychology. Hong Kong. Asian-South Pacific Association of Sport Psychology. pp. 404-430Shooting in India
Terry, Peter C. and Cei, Alberto. 2014. "Shooting in India." Terry, Peter C., Li-Wei, Zhang, Young-Ho, Kim, Morris, Tony and Hanrahan, Stephanie (ed.) Secrets of Asian sport psychology. Hong Kong. Asian-South Pacific Association of Sport Psychology. pp. 263-294Secrets of Asian sport psychology [Introduction]
Terry, Peter C.. 2014. "Secrets of Asian sport psychology [Introduction]." Terry, Peter C., Li-Wei, Zhang, Young-Ho, Kim, Morris, Tony and Hanrahan, Stephanie (ed.) Secrets of Asian sport psychology. Hong Kong. Asian-South Pacific Association of Sport Psychology. pp. 1-9The impact of workplace incivility on the satisfaction of basic needs: the role of past experience
Machin, M. A. and Jeffries, C. H.. 2013. "The impact of workplace incivility on the satisfaction of basic needs: the role of past experience." 48th Australian Psychological Society Annual Conference (APS 2013): Psychology for a Healthy Nation. Cairns, Australia 08 - 12 Oct 2013 Melbourne, Australia.Combining survey and database technologies in support of a safety climate improvement strategy
Fogarty, Gerard J. and Machin, M. Anthony. 2008. "Combining survey and database technologies in support of a safety climate improvement strategy." Murphy, Peter J., Fogarty, Gerard J. and Swann, Jamie (ed.) 49th Annual Conference of the International Military Testing Association. Gold Coast, Australia 08 - 12 Oct 2007 Canberra, Australia.Psychometric re-evaluation of the revised version of the competitive state anxiety inventory-2
Terry, Peter C. and Munro, Angus. 2008. "Psychometric re-evaluation of the revised version of the competitive state anxiety inventory-2 ." Voudouris, Nicholas and Mrowinski, Vicky (ed.) 43rd Annual Australian Psychological Society Conference (APS 2008): Psychology Leading Change. Hobart, Australia 23 - 27 Sep 2008 Melbourne, Australia.Mood profiling of international athletes in Argentina: theoretical and applied developments
Wightman, Patricia, Terry, Peter C. and Giscafre, Nelly. 2011. "Mood profiling of international athletes in Argentina: theoretical and applied developments." 24th Annual Conference of the Association for Applied Sport Psychology (AASP 2009). Salt Lake City, United States 15 - 18 Sep 2009 Indianapolis, IN. United States.Music applications with elite athletes
Terry, P. C.. 2012. "Music applications with elite athletes." Brackenridge, Celia (ed.) International Convention on Science, Education and Medicine in Sport (ICSEMIS 2012): Sport: Inspiring a Learning Legacy. Glasgow, United Kingdom 19 - 24 Jul 2012 Berlin, Germany.Citius, altius, fortius: if only it were that simple [Keynote]
Terry, P. C.. 2012. "Citius, altius, fortius: if only it were that simple [Keynote]." Brackenridge, Celia (ed.) International Convention on Science, Education and Medicine in Sport (ICSEMIS 2012): Sport: Inspiring a Learning Legacy. Glasgow, United Kingdom 19 - 24 Jul 2012 Berlin, Germany.Effects of synchronous music among elite endurance athletes
Terry, Peter, Curran, Michelle, Saha, Alessandra Mecozzi and Bool, Ross. 2012. "Effects of synchronous music among elite endurance athletes." International Convention on Science, Education and Medicine in Sport (ICSEMIS 2012): Sport: Inspiring a Learning Legacy. Glasgow, United Kingdom 19 - 24 Jul 2012 London, United Kingdom.Applied sport psychology: beware the sun, Icarus
Terry, Peter C.. 2011. "Applied sport psychology: beware the sun, Icarus." Martin, Paul R., Cheung, Fanny M., Knowles, Michael C., Kyrios, Michael, Littlefield, Lyn, Overmier, J.Bruce and Prieto, Jose M. (ed.) IAAP Handbook of Applied Psychology. Oxford, UK. Wiley-Blackwell. pp. 386-410Employee participation in non-mandatory professional development – the role of core proactive motivation processes
Sankey, Kim S. and Machin, M. Anthony. 2014. "Employee participation in non-mandatory professional development – the role of core proactive motivation processes." International Journal of Training and Development. 18 (4), pp. 241-255. https://doi.org/10.1111/ijtd.12036Psychometric properties of the Brunel Mood Scale in Chinese adolescents and adults
Zhang, Chun-Qing, Si, Gangyan, Chung, Pak-Kwong, Du, Mengmeng and Terry, Peter C.. 2014. "Psychometric properties of the Brunel Mood Scale in Chinese adolescents and adults." Journal of Sports Sciences. 32 (15), pp. 1465-1476. https://doi.org/10.1080/02640414.2014.898184Effects of a sport programme (Box'Tag®) on disadvantaged youth participants
Terry, Peter C., Hahn, Allan and Simjanovic, Melina. 2014. "Effects of a sport programme (Box'Tag®) on disadvantaged youth participants." International Journal of Sport and Exercise Psychology. 12 (3), pp. 258-272. https://doi.org/10.1080/1612197X.2014.880263Development of a motivation-based taxonomy of adult sport participants using a self-determination theory perspective.
Vlachopoulos, Symeon P., Karageorghis, Costas I. and Terry, Peter C.. 2001. "Development of a motivation-based taxonomy of adult sport participants using a self-determination theory perspective. " Papaioannou, A., Goudas, M. and Theodorakis, Y. (ed.) International Society of Sport Psychology 10th World Congress. Skiathos, Greece 28 May - 02 Jun 2001 Thessaloniki, Greece.Psychological wellbeing and the diathesis-stress hypothesis model: the role of psychological functioning and quality of relations in promoting subjective well-being in a life events study
Burns, Richard Andrew and Machin, Michael Anthony. 2013. "Psychological wellbeing and the diathesis-stress hypothesis model: the role of psychological functioning and quality of relations in promoting subjective well-being in a life events study." Personality and Individual Differences. 54 (3), pp. 321-326. https://doi.org/10.1016/j.paid.2012.09.017Perceptions of group cohesion and mood in sport teams
Terry, Peter C., Carron, Albert V., Pink, Mark J., Lane, Andrew M., Jones, Garland J. W. and Hall, Mark P.. 2000. "Perceptions of group cohesion and mood in sport teams." Group Dynamics: theory, research, and practice. 4 (3), pp. 244-253. https://doi.org/10.1037//1089-2699.4.3.244Combining theory, survey methodology, and database technologies in support of an organisational climate improvement strategy research
Fogarty, Gerard J. and Machin, Tony. 2013. "Combining theory, survey methodology, and database technologies in support of an organisational climate improvement strategy research." Lacey, Ron (ed.) Advances in organisational research methods and analysis, Australia. New York, United States. Chamber & Row. pp. 86-98Metadata in organisational surveys
Machin, Tony. 2013. "Metadata in organisational surveys." Lacey, Ron (ed.) Advances in organisational research methods and analysis. New York, United States. Chamber & Row. pp. 74-85Measuring psycho-physiological indicators to explore the use of music for enhancing mindfulness imagery experiences
Kuan, Garry, Morris, Tony and Terry, Peter. 2012. "Measuring psycho-physiological indicators to explore the use of music for enhancing mindfulness imagery experiences." Brackenridge, Celia (ed.) 2nd International Congress of Science, Education and Medicine in Sport (ICSEMIS 2012): Sport: Inspiring a Learning Legacy. Glasgow, United Kingdom 19 - 24 Jul 2012 London, United Kingdom.Eating attitudes, body shape perceptions and mood of elite rowers
Terry, Peter C., Lane, Andrew M. and Warren, Lucinda. 1999. "Eating attitudes, body shape perceptions and mood of elite rowers." Journal of Science and Medicine in Sport. 2 (1), pp. 67-77. https://doi.org/10.1016/S1440-2440(99)80185-6Motivation profiles in sport: a self-determination theory perspective
Vlachopoulos, Symeon P., Karageorghis, Costas I. and Terry, Peter C.. 2000. "Motivation profiles in sport: a self-determination theory perspective ." Research Quarterly for Exercise and Sport. 71 (4), pp. 387-397. https://doi.org/10.1080/02701367.2000.10608921Effects of synchronous music on elite athletes during training activities
Terry, P., Mecozzi, A. and Bool, R.. 2012. "Effects of synchronous music on elite athletes during training activities." Journal of Science and Medicine in Sport. 15 (1 (Supplement)), pp. 222-223. https://doi.org/10.1016/j.jsams.2012.11.540The BASES expert statement on use of music in exercise
Karageorghis, Costas I., Terry, Peter C., Lane, Andrew M., Bishop, Daniel T. and Priest, David-Lee. 2012. "The BASES expert statement on use of music in exercise." Journal of Sports Sciences. 30 (9), pp. 953-956. https://doi.org/10.1080/02640414.2012.676665Effects of synchronous music on treadmill running among elite triathletes
Terry, Peter C., Karageorghis, Costas I., Saha, Alessandra Mecozzi and D'Auria, Shaun. 2012. "Effects of synchronous music on treadmill running among elite triathletes." Journal of Science and Medicine in Sport. 15 (1), pp. 52-57. https://doi.org/10.1016/j.jsams.2011.06.003Development and initial validation of the Farsi Mood Scale
Terry, Peter C., Malekshahi, Maryam and Delva, Heather A.. 2012. "Development and initial validation of the Farsi Mood Scale." International Journal of Sport and Exercise Psychology. 10 (2), pp. 112-122. https://doi.org/10.1080/1612197X.2012.645133Employee and workplace well-being: a multi-level analysis of teacher personality and organizational climate in Norwegian teachers from rural, urban and city schools
Burns, Richard Andrew and Machin, Michael Anthony. 2013. "Employee and workplace well-being: a multi-level analysis of teacher personality and organizational climate in Norwegian teachers from rural, urban and city schools." Scandinavian Journal of Educational Research. 57 (3), pp. 309-324. https://doi.org/10.1080/00313831.2012.656281The new sport and exercise psychology companion [Introduction]
Morris, Tony and Terry, Peter. 2011. "The new sport and exercise psychology companion [Introduction]." Morris, Tony and Terry, Peter C. (ed.) The new sport and exercise psychology companion. Morgantown, WV. United States. Fitness Information Technology. pp. 1-8The new sport and exercise psychology companion [Conclusion]
Morris, Tony and Terry, Peter. 2011. "The new sport and exercise psychology companion [Conclusion]." Morris, Tony and Terry, Peter C. (ed.) The new sport and exercise psychology companion. Morgantown, WV. United States. Fitness Information Technology. pp. 625-628Chase that feeling: recent developments in music and sport research
Terry, Peter C., Curran, Michelle, Karageorghis, Costas I., Saha, Alessandra Mecozzi and D'Auria, Shaun. 2011. "Chase that feeling: recent developments in music and sport research." Liu, Suyen (ed.) ASPASP 2011: Turning a New Page: A Refreshing Look at Sports and Exercise Psychology from an Asian Perspective. Taipei, Taiwan 11 - 14 Nov 2011 Taipei, Taiwan.The new sport and exercise psychology companion
Morris, Tony and Terry, Peter C.. Morris, Tony and Terry, Peter C. (ed.) 2011. The new sport and exercise psychology companion. Morgantown, WV. United States. Fitness Information Technology.Music in sport and exercise
Terry, Peter C. and Karageorghis, Costas I.. 2011. "Music in sport and exercise." Morris, Tony and Terry, Peter C. (ed.) The new sport and exercise psychology companion. Morgantown, WV. United States. Fitness Information Technology. pp. 359-380Mood and emotions
Terry, Peter C. and Lane, Andrew M.. 2011. "Mood and emotions." Morris, Tony and Terry, Peter C. (ed.) The new sport and exercise psychology companion. Morgantown, WV. United States. Fitness Information Technology. pp. 63-87Understanding how the satisfaction of basic needs predicts motivation to participate in development opportunities
Machin, T.. 2011. "Understanding how the satisfaction of basic needs predicts motivation to participate in development opportunities." Byrne, Don (ed.) 46th Australian Psychological Society Annual Conference 2011. Canberra, Australia 04 - 08 Oct 2011 Melbourne, Australia.Factorial and concurrent validity of two anxiety measures and anxiety responses among vision-impaired people
Terry, P. and Barron, J.. 2011. "Factorial and concurrent validity of two anxiety measures and anxiety responses among vision-impaired people ." Byrne, Don (ed.) 46th Australian Psychological Society Annual Conference 2011. Canberra, Australia 04 - 08 Oct 2011 Melbourne, Australia.Ergogenic, psychological, and psychophyiological effects of synchronous music on treadmill running
Terry, Peter C., Karageorghis, Costas I., Mecozzi, Alessandra S. and D'Auria, Shaun I.. 2011. "Ergogenic, psychological, and psychophyiological effects of synchronous music on treadmill running ." Serpa, Sidonio, Teixeira, Nelson, Almeida, Maria and Rosado, Antonio (ed.) 13th FEPSAC European Congress of Sport Psychology: Sport and Exercise Psychology: Human Performance, Well-being and Health (ECSP 2011). Madeira, Portugal 12 - 17 Jul 2011 Madeira, Portugal.Effects of relaxing and arousing music on imagery for dart throwing
Kuan, Garry, Morris, Tony and Terry, Peter C.. 2011. "Effects of relaxing and arousing music on imagery for dart throwing." Serpa, Sidonio, Teixeira, Nelson, Almeida, Maria and Rosado, Antonio (ed.) 13th FEPSAC European Congress of Sport Psychology: Sport and Exercise Psychology: Human Performance, Well-being and Health (ECSP 2011). Madeira, Portugal 12 - 17 Jul 2011 Madeira, Portugal.Identifying interactive components of the horse-rider partnership during competition dressage
Bridgeman, D. J., Pretty, G. M. and Terry, P. C.. 2011. "Identifying interactive components of the horse-rider partnership during competition dressage." van Dierendonck, Machteld, de Cocq, Patricia and Visser, Kathalijne (ed.) 7th International Equitation Science Conference: Equitation Science: Principles and Practices - Science at Work. Hooge Mierde, Netherlands 26 - 29 Oct 2011 Wageningen, Netherlands.Effects of synchronous music use in ultra-distance events
Curran, M. and Terry, P.. 2011. "Effects of synchronous music use in ultra-distance events ." Journal of Science and Medicine in Sport. 14 (1 (Supplement)), pp. e83-e84. https://doi.org/10.1016/j.jsams.2011.11.172In the mood: development of an online mood profiling system
Terry, Peter C. and Lim, Julian. 2011. "In the mood: development of an online mood profiling system." Liu, Suyen (ed.) ASPASP 2011: Turning a New Page: A Refreshing Look at Sports and Exercise Psychology from an Asian Perspective. Taipei, Taiwan 11 - 14 Nov 2011 Taipei, Taiwan.Moving beyond the pleasure principle: within and between-occasion effects of employee eudaimonia within a school organizational climate context
Burns, Richard Andrew and Machin, Michael Anthony. 2012. "Moving beyond the pleasure principle: within and between-occasion effects of employee eudaimonia within a school organizational climate context." Journal of Vocational Behavior. 80 (1), pp. 118-128. https://doi.org/10.1016/j.jvb.2011.04.007Developing and implementing an action-oriented staff survey: Queensland Health and the 'Better Workplaces' initiative
Jury, Ceri, Machin, M. Anthony, Phillips, Jan, Goh, Hong Eng, Olsen, Shaney P. and Patrick, Jeff. 2009. "Developing and implementing an action-oriented staff survey: Queensland Health and the 'Better Workplaces' initiative." Australian Health Review. 33 (3), pp. 365-370. https://doi.org/10.1071/AH090365I’ve got the music in me: scientific basis and application of music in sport and exercise
Terry, Peter C. and Karageorghis, Costas I.. 2009. "I’ve got the music in me: scientific basis and application of music in sport and exercise." Meeting New Challenges and Bridging Cultural Gaps in Sport and Exercise Psychology (2009). Marrakesh, Morocco 17 - 21 Jun 2009 Marrakech, Morocco.Mood responses to athletic performance in extreme environments
Lane, Andrew M., Terry, Peter C., Stevens, Matthew J., Barney, Sam and Dinsdale, Sarah L.. 2004. "Mood responses to athletic performance in extreme environments." Journal of Sports Sciences. 22 (10), pp. 886-897. https://doi.org/10.1080/02640410400000165Relationships between pre-competition mood and swimming performance: test of a conceptual model with an emphasis on depressed mood
Janover, Marc A. and Terry, Peter C.. 2002. "Relationships between pre-competition mood and swimming performance: test of a conceptual model with an emphasis on depressed mood." 37th Annual Conference of the Australian Psychological Society. Gold Coast, Australia 27 Sep - 10 Oct 2002 United Kingdom. Taylor & Francis. https://doi.org/10.1111/j.1742-9536.2002.tb01871.xHierarchical confirmatory factor analysis of the Flow State Scale in exercise
Vlachopoulos, Symeon P., Karageorghis, Costas I. and Terry, Peter C.. 2000. "Hierarchical confirmatory factor analysis of the Flow State Scale in exercise ." Journal of Sports Sciences. 18 (10), pp. 815-823. https://doi.org/10.1080/026404100419874Mood profiling during Olympic qualifying judo competition: a case study testing transactional relationships
Stevens, Matthew J., Lane, Andrew M. and Terry, Peter C.. 2006. "Mood profiling during Olympic qualifying judo competition: a case study testing transactional relationships." Journal of Sports Science and Medicine. 5 (Special issue), pp. 143-151.The nature of mood: development of a conceptual model with a focus on depression
Lane, Andrew M. and Terry, Peter C.. 2000. "The nature of mood: development of a conceptual model with a focus on depression." Journal of Applied Sport Psychology. 12 (1), pp. 16-33. https://doi.org/10.1080/10413200008404211The profile of mood states and athletic performance: two meta-analyses
Beedie, Christopher J., Terry, Peter C. and Lane, Andrew M.. 2000. "The profile of mood states and athletic performance: two meta-analyses ." Journal of Applied Sport Psychology. 12 (1), pp. 49-68. https://doi.org/10.1080/10413200008404214Strategies for reflective cultural sport psychology practice
Terry, Peter C.. 2009. "Strategies for reflective cultural sport psychology practice ." Schinke, Robert and Hanrahan, Stephanie J. (ed.) Cultural sport psychology. Champaign, IL. United States. Human Kinetics Publishers. pp. 79-89Cohesion and teamwork
Munroe, Krista, Terry, Peter and Carron, Albert. 2002. "Cohesion and teamwork." Hale, Bruce D. and Collins, David J. (ed.) Rugby tough: focused for rugby. Champaign, IL. United States. Human Kinetics Publishers. pp. 137-153Use of music interventions with elite athletes
Terry, Peter C. and Karageorghis, Costas I.. 2007. "Use of music interventions with elite athletes." Theodorakis, Yannis, Goudas, Marios and Papaioannou, Athanasios (ed.) 12th European Congress of Sport Psychology: Sport & Exercise Psychology: Bridges between disciplines & cultures. Halkidiki, Greece 04 - 09 Sep 2007 Greece.The Sony Ericsson WTA tour 10 year age eligibility and professional development review
Otis, C. L., Crespo, M., Flygare, C. T., Johnston, P. R., Keber, A., Lloyd-Kolkin, D., Loehr, J., Martin, K., Pluim, B. M., Quinn, A., Roetert, P., Stroia, K. A. and Terry, P. C.. 2006. "The Sony Ericsson WTA tour 10 year age eligibility and professional development review." British Journal of Sports Medicine. 40 (5), pp. 464-468. https://doi.org/10.1136/bjsm.2005.023366Confirmatory factor analysis of the test of performance strategies (TOPS) among adolescent athletes
Lane, Andrew M., Harwood, Chris, Terry, Peter C. and Karageorghis, Costas I.. 2004. "Confirmatory factor analysis of the test of performance strategies (TOPS) among adolescent athletes." Journal of Sports Sciences. 22 (9), pp. 803-812. https://doi.org/10.1080/02640410410001716689Redesign and initial validation of an instrument to assess the motivational qualities of music in exercise: the Brunel Music Rating Inventory-2
Karageorghis, Costas I., Priest, David-Lee, Terry, Peter C., Chatzisarantis, Nikos L. D. and Lane, Andrew M.. 2006. "Redesign and initial validation of an instrument to assess the motivational qualities of music in exercise: the Brunel Music Rating Inventory-2." Journal of Sports Sciences. 24 (8), pp. 899-909. https://doi.org/10.1080/02640410500298107Perceptions of training-related factors and personal variables as predictors of transfer implementation intentions
Machin, M. Anthony and Fogarty, Gerard J.. 2003. "Perceptions of training-related factors and personal variables as predictors of transfer implementation intentions." Journal of Business and Psychology. 18 (1), pp. 51-71. https://doi.org/10.1023/A:1025082920860The role of satisfaction with occupational status, neuroticism, financial strain and categories of experience in predicting mental health in the unemployed
Creed, Peter A., Muller, Juanita J. and Machin, M. Anthony. 2001. "The role of satisfaction with occupational status, neuroticism, financial strain and categories of experience in predicting mental health in the unemployed." Personality and Individual Differences. 30 (3), pp. 435-447. https://doi.org/10.1016/S0191-8869(00)00035-0Understanding the differential benefits of training for the unemployed
Machin, M. Anthony and Creed, Peter A.. 2003. "Understanding the differential benefits of training for the unemployed." Australian Journal of Psychology. 55 (2), pp. 104-113. https://doi.org/10.1080/00049530412331312964Planning, managing, and optimizing transfer of training
Machin, M. Anthony. 2002. "Planning, managing, and optimizing transfer of training." Kraiger, Kurt (ed.) Creating, implementing, and managing effective training and development. San Francisco, United States. John Wiley & Sons. pp. 263-301Evaluating a fatigue management training program for coach drivers
Machin, M. Anthony. 2003. "Evaluating a fatigue management training program for coach drivers." Dorn, Lisa (ed.) Driver behaviour and training. Aldershot, UK. Ashgate Publishing Limited. pp. 75-83Factors influencing young drivers' risk perceptions and speeding behaviour
Machin, M. Anthony and Sankey, Kim S.. 2006. "Factors influencing young drivers' risk perceptions and speeding behaviour." King, Mark (ed.) Australasian Road Safety Research, Policing and Education Conference (2006). Gold Coast, Australia 25 - 27 Oct 2006 Gold Coast, Qld.Psychological predictors of injury among elite athletes
Galambos, S. A., Terry, P. C., Moyle, G. M. and Locke, S. A.. 2005. "Psychological predictors of injury among elite athletes." British Journal of Sports Medicine. 39 (6), pp. 351-354. https://doi.org/10.1136/bjsm.2005.018440Mood, self-set goals and examination performance: the moderating effect of depressed mood
Terry, Peter C., Lane, Andrew M., Nevill, Alan M. and Whyte, Gregory P.. 2005. "Mood, self-set goals and examination performance: the moderating effect of depressed mood." Personality and Individual Differences. 39 (1), pp. 143-153. https://doi.org/10.1016/j.paid.2004.12.015Distinctions between emotion and mood
Beedie, Christopher, Terry, Peter and Lane, Andrew. 2005. "Distinctions between emotion and mood." Cognition and Emotion. 19 (6), pp. 847-878. https://doi.org/10.1080/02699930541000057Influence of response time frame on mood assessment
Terry, Peter C., Stevens, Matthew J. and Lane, Andrew M.. 2005. "Influence of response time frame on mood assessment." Anxiety, Stress and Coping. 18 (3), pp. 279-285. https://doi.org/10.1080/10615800500134688Personality does not influence exercise-induced mood enhancement among female exercisers
Lane, Andrew M., Milton, Karen E. and Terry, Peter C.. 2005. "Personality does not influence exercise-induced mood enhancement among female exercisers." Journal of Sports Science and Medicine. 4 (3), pp. 223-228.Preferred modality influences on exercise-induced mood changes
Lane, Andrew M., Jackson, Andrew and Terry, Peter C.. 2005. "Preferred modality influences on exercise-induced mood changes." Journal of Sports Science and Medicine. 4 (2), pp. 195-200.Differential assessment of emotions and moods: development and validation of the emotion and mood components of anxiety questionnaire
Beedie, Christopher J., Terry, Peter C., Lane, Andrew M. and Devonport, Tracey J.. 2011. "Differential assessment of emotions and moods: development and validation of the emotion and mood components of anxiety questionnaire." Personality and Individual Differences. 50 (2), pp. 228-233. https://doi.org/10.1016/j.paid.2010.09.034The BASES expert statement on the use of music in exercise
Karageorghis, Costas I., Terry, Peter C., Lane, Andrew M., Bishop, Daniel T. and Priest, David-Lee. 2011. "The BASES expert statement on the use of music in exercise." The Sport and Exercise Scientist.It's nice to go travelling BUT...
Terry, Peter C.. 2010. "It's nice to go travelling BUT..." Hanrahan, Stephanie J. and Andersen, Mark B. (ed.) Routledge handbook of applied sport psychology: a comprehensive guide for students and practitioners. Abingdon, Oxon. United Kingdom. Routledge. pp. 345-354Incidence of injury, psychological correlates, and injury prevention strategies for elite sport
Galambos, Sandor A., Terry, Peter C. and Moyle, Gene M.. 2006. "Incidence of injury, psychological correlates, and injury prevention strategies for elite sport." Katsikitis, Mary (ed.) 2006 Joint Conference of the Australian Psychological Society and the New Zealand Psychological Society. Auckland, New Zealand 26 - 30 Sep 2006 Melbourne, Australia.Understanding the unique contribution of aversion to risk taking in predicting drivers' self-reported speeding
Machin, M. Anthony and Plint, Janna E.. 2010. "Understanding the unique contribution of aversion to risk taking in predicting drivers' self-reported speeding." Dorn, Lisa (ed.) Driver behaviour and training. volume 4. Surrey, United Kingdom. Ashgate Publishing Limited. pp. 61-73The impact of reemployment on access to the latent and manifest benefits of employment and mental health
Hoare, P. Nancey and Machin, M. Anthony. 2010. "The impact of reemployment on access to the latent and manifest benefits of employment and mental health." Journal of Occupational and Organizational Psychology. 83 (3), pp. 759-770. https://doi.org/10.1348/096317909X472094Affective responses, emotional intelligence and examination performance of university undergraduates
Hulme, R. and Terry, Peter C.. 2010. "Affective responses, emotional intelligence and examination performance of university undergraduates." Mrowinski, Vicky, Kyrios, Michael and Voudouris, Nicholas (ed.) 27th International Congress of Applied Psychology (ICAP 2010). Melbourne, Australia 11 - 16 Jul 2010 Melbourne, Australia.Effects of synchronous music on treadmill running among elite triathletes
Mecozzi, Alessandra, Terry, Peter C., D'Auria, S. and Karageorghis, Costas I.. 2010. "Effects of synchronous music on treadmill running among elite triathletes." Mrowinski, Vicky, Kyrios, Michael and Voudouris, Nicholas (ed.) 27th International Congress of Applied Psychology (ICAP 2010). Melbourne, Australia 11 - 16 Jul 2010 Melbourne, Australia.Quantification of physiological arousal during familiar arousing music, unfamiliar arousing music, and unfamiliar relaxing music during imagery in elite shooters
Kuan, G., Morris, Tony and Terry, Peter C.. 2010. "Quantification of physiological arousal during familiar arousing music, unfamiliar arousing music, and unfamiliar relaxing music during imagery in elite shooters." Mrowinski, Vicky, Kyrios, Michael and Voudouris, Nicholas (ed.) 27th International Congress of Applied Psychology (ICAP 2010). Melbourne, Australia 11 - 16 Jul 2010 Melbourne, Australia.Music applications for athletes
Terry, Peter C.. 2010. "Music applications for athletes." Mrowinski, Vicky, Kyrios, Michael and Voudouris, Nicholas (ed.) 27th International Congress of Applied Psychology (ICAP 2010). Melbourne, Australia 11 - 16 Jul 2010 Melbourne, Australia.Keep on running: benefits of music for exercise and sport
Terry, Peter C. and Morris, Tony. 2010. "Keep on running: benefits of music for exercise and sport." Mrowinski, Vicky, Kyrios, Michael and Voudouris, Nicholas (ed.) 27th International Congress of Applied Psychology (ICAP 2010). Melbourne, Australia 11 - 16 Jul 2010 Melbourne, Australia.Mood profiling in sports psychology
Terry, Peter C.. 2010. "Mood profiling in sports psychology." Mrowinski, Vicky, Kyrios, Michael and Voudouris, Nicholas (ed.) 27th International Congress of Applied Psychology (ICAP 2010). Melbourne, Australia 11 - 16 Jul 2010 Melbourne, Australia.Applied sport psychology: beware the sun, Icarus
Terry, Peter C.. 2010. "Applied sport psychology: beware the sun, Icarus." Mrowinski, Vicky, Kyrios, Michael and Voudouris, Nicholas (ed.) 27th International Congress of Applied Psychology (ICAP 2010). Melbourne, Australia 11 - 16 Jul 2010 Melbourne, Australia.Coping styles, affective responses and examination performance of university undergraduates
Briggs, Elizabeth and Terry, Peter C.. 2010. "Coping styles, affective responses and examination performance of university undergraduates." Mrowinski, Vicky, Kyrios, Michael and Voudouris, Nicholas (ed.) 27th International Congress of Applied Psychology (ICAP 2010). Melbourne, Australia 11 - 16 Jul 2010 Melbourne, Australia.What you see is what you get: a meta-analytic review of the effects of imagery in sport and exercise domains
Curran, M. L. and Terry, Peter C.. 2010. "What you see is what you get: a meta-analytic review of the effects of imagery in sport and exercise domains." Mrowinski, Vicky, Kyrios, Michael and Voudouris, Nicholas (ed.) 27th International Congress of Applied Psychology (ICAP 2010). Melbourne, Australia 11 - 16 Jul 2010 Melbourne, Australia.The role of climate for transfer of training and intrinsic benefits in explaining employees' motivation to attend voluntary professional development activities
Sankey, Kim S. and Machin, M. Anthony. 2010. "The role of climate for transfer of training and intrinsic benefits in explaining employees' motivation to attend voluntary professional development activities." Mrowinski, Vicky, Kyrios, Michael and Voudouris, Nicholas (ed.) 27th International Congress of Applied Psychology (ICAP 2010). Melbourne, Australia 11 - 16 Jul 2010 Melbourne, Australia.Examining the validity of driver prototypes using driving-specific measures of personality and coping
Machin, M. Anthony. 2010. "Examining the validity of driver prototypes using driving-specific measures of personality and coping." Mrowinski, Vicky, Kyrios, Michael and Voudouris, Nicholas (ed.) 27th International Congress of Applied Psychology (ICAP 2010). Melbourne, Australia 11 - 16 Jul 2010 Melbourne, Australia.A structural model linking commitment to organizational change and change success
Machin, M. Anthony and Fogarty, Gerard J.. 2010. "A structural model linking commitment to organizational change and change success." Mrowinski, Vicky, Kyrios, Michael and Voudouris, Nicholas (ed.) 27th International Congress of Applied Psychology (ICAP 2010). Melbourne, Australia 11 - 16 Jul 2010 Melbourne, Australia.The impact of ageing on capacity for and decisions about driving
Machin, M. Anthony and Dorn, Lisa. 2010. "The impact of ageing on capacity for and decisions about driving." Mrowinski, Vicky, Kyrios, Michael and Voudouris, Nicholas (ed.) 27th International Congress of Applied Psychology (ICAP 2010). Melbourne, Australia 11 - 16 Jul 2010 Melbourne, Australia.Examining the validity of the problem solving inventory in Australia
Beccaria, Gavin and Machin, M. Anthony. 2010. "Examining the validity of the problem solving inventory in Australia." Mrowinski, Vicky, Kyrios, Michael and Voudouris, Nicholas (ed.) 27th International Congress of Applied Psychology (ICAP 2010). Melbourne, Australia 11 - 16 Jul 2010 Melbourne, Australia.Establishing links between organizational climate, employee well-being and historical patient outcomes
Machin, T., Goh, H. E., Patrick, J. and Jury, C.. 2010. "Establishing links between organizational climate, employee well-being and historical patient outcomes." Mrowinski, Vicky, Kyrios, Michael and Voudouris, Nicholas (ed.) 27th International Congress of Applied Psychology (ICAP 2010). Melbourne, Australia 11 - 16 Jul 2010 Melbourne, Australia.Improving employee engagement, well-being and performance: new tools, interventions, and solutions
Machin, M. Anthony and Fogarty, Gerard J.. 2010. "Improving employee engagement, well-being and performance: new tools, interventions, and solutions." Mrowinski, Vicky, Kyrios, Michael and Voudouris, Nicholas (ed.) 27th International Congress of Applied Psychology (ICAP 2010). Melbourne, Australia 11 - 16 Jul 2010 Melbourne, Australia.Identifying gender differences in the independent effects of personality and psychological well-being on two broad affect components of subjective well-being
Burns, R. A. and Machin, M. A.. 2010. "Identifying gender differences in the independent effects of personality and psychological well-being on two broad affect components of subjective well-being." Personality and Individual Differences. 48 (1), pp. 22-27. https://doi.org/10.1016/j.paid.2009.08.007Predicting job satisfaction and depression at work: how important are work-related factors?
Machin, M. Anthony. 2009. "Predicting job satisfaction and depression at work: how important are work-related factors?" Voudouris, Nicholas and Mrowinski, Vicky (ed.) 44th Australian Psychological Society Annual Conference 2009. Darwin, Australia 30 Sep - 04 Oct 2009 Melbourne, Australia.The importance of psychological well-being in organisational settings: moving beyond the pleasure principle
Burns, Richard A. and Machin, M. Anthony. 2009. "The importance of psychological well-being in organisational settings: moving beyond the pleasure principle." Voudouris, Nicholas and Mrowinski, Vicky (ed.) 44th Australian Psychological Society Annual Conference 2009. Darwin, Australia 30 Sep - 04 Oct 2009 Melbourne, Australia.Social problem-solving skills and mental health: a comparison of undergraduate cohorts
Beccaria, Gavin, Lee, Edna and Machin, M. Anthony. 2009. "Social problem-solving skills and mental health: a comparison of undergraduate cohorts." Voudouris, Nicholas and Mrowinski, Vicky (ed.) 44th Australian Psychological Society Annual Conference 2009. Darwin, Australia 30 Sep - 04 Oct 2009 Melbourne, Australia.Making a difference: the impact of sports science and medicine in women’s professional tennis
Terry, Peter C.. 2003. "Making a difference: the impact of sports science and medicine in women’s professional tennis ." 2003 Australian Conference of Science and Medicine in Sport and 3rd National Sports Injury Prevention Conference: Tackling the Barriers to Performance and Participation. Canberra, Australia 25 - 28 Oct 2003 Dickson, ACT, Australia.Psychological models of sporting injury: Janos Selye revisited
Galambos, S. A. and Terry, Peter C.. 2006. "Psychological models of sporting injury: Janos Selye revisited ." Journal of Science and Medicine in Sport. 9 (Supplement), pp. 34-34.Effects of a cognitive behavioural intervention on stress, recovery and performance
West, J., Galambos, S. A., Terry, Peter C. and Hooper, S.. 2007. "Effects of a cognitive behavioural intervention on stress, recovery and performance." Journal of Science and Medicine in Sport. 10 (1 (Supplement)), pp. 105-105.ThinkSmart routines and rowing ergometer performance
Lloyd, Michael and Terry, Peter C.. 2007. "ThinkSmart routines and rowing ergometer performance." Journal of Science and Medicine in Sport. 10 (1 (Supplement)), pp. 113-113. https://doi.org/10.1016/S1440-2440(07)70346-8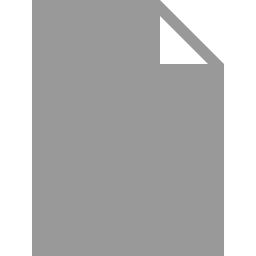